Commit
This commit does not belong to any branch on this repository, and may belong to a fork outside of the repository.
fix RowwiseMoments vectorization issue on CPU (pytorch#84404)
Originally `cpu/moments_utils.h` uses namespace of at::native::utils, this file contains `Vectorized<>`, in order to make it properly vectorized on different archs, need to use anonymous namespace or inline namespace. Otherwise it would be linked to scalar version of the code. This PR is to fix vectorization issue from `RowwiseMoments` which is used to calculate `mean` and `rstd` in norm layers. Attach benchmark data, generally fp32 will get 2-3x speedup and bf16 has larger speedup. This patch will improves layer_norm (input size 32x128x1024) float32 inference: * avx512 single socket: 2.1x ```bash before: LayerNorm((1024,), eps=1e-05, elementwise_affine=True) : 32x128x1024: fp32: 0.439 ms; bf16: 2.479 ms after: LayerNorm((1024,), eps=1e-05, elementwise_affine=True) : 32x128x1024: fp32: 0.210 ms; bf16: 0.770 ms ``` * avx512 single core: 3.2x ```bash before: LayerNorm((1024,), eps=1e-05, elementwise_affine=True) : 32x128x1024: fp32: 6.308 ms; bf16: 39.765 ms after: LayerNorm((1024,), eps=1e-05, elementwise_affine=True) : 32x128x1024: fp32: 2.661 ms; bf16: 12.267 ms ``` * avx2 single socket: 2.3x ```bash before: LayerNorm((1024,), eps=1e-05, elementwise_affine=True) : 32x128x1024: fp32: 1.248 ms; bf16: 8.487 ms after: LayerNorm((1024,), eps=1e-05, elementwise_affine=True) : 32x128x1024: fp32: 0.540 ms; bf16: 2.030 ms ``` * avx2 single core: 2.5x ```bash before: LayerNorm((1024,), eps=1e-05, elementwise_affine=True) : 32x128x1024: fp32: 10.792 ms; bf16: 66.366 ms after: LayerNorm((1024,), eps=1e-05, elementwise_affine=True) : 32x128x1024: fp32: 4.349 ms; bf16: 19.252 ms ``` Attached some original VTune profiling results here to further indicate the issue: 1. original bottlenecks 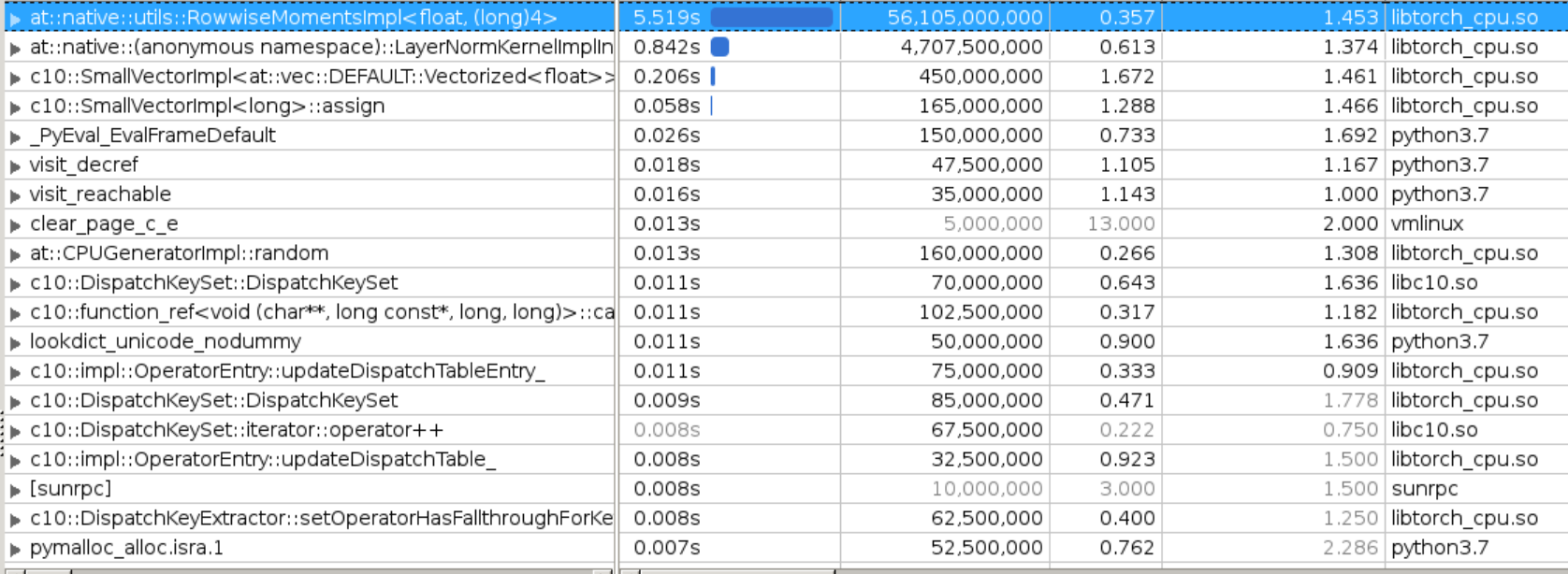 we can see `RowwiseMomentsImpl<>` takes majority of the runtime here. 2. Instruction level breakdown of `RowwiseMomentsImpl<>` 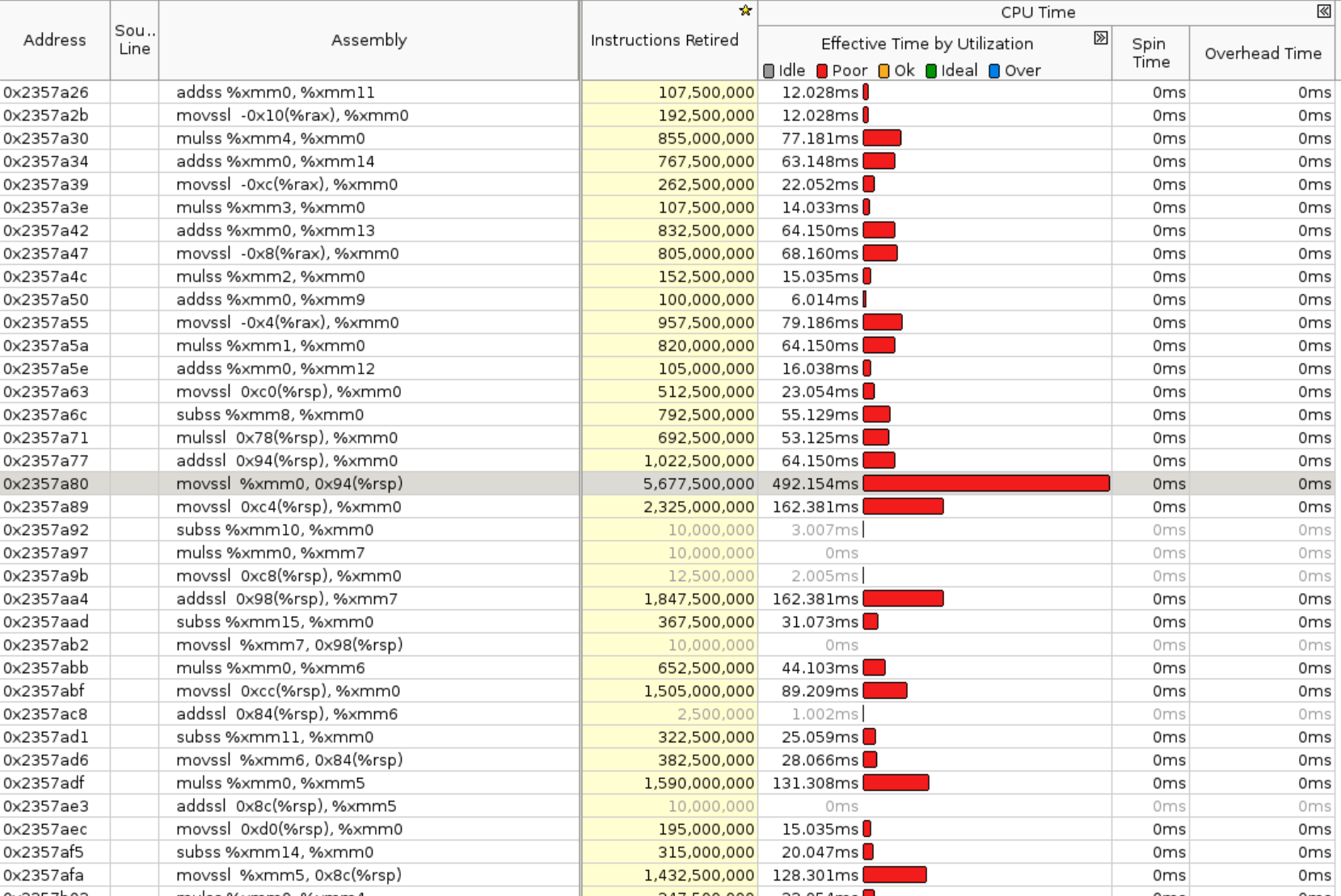 we can see it's all **scalar** instructions here. 3. after the fix, the bottlenecks 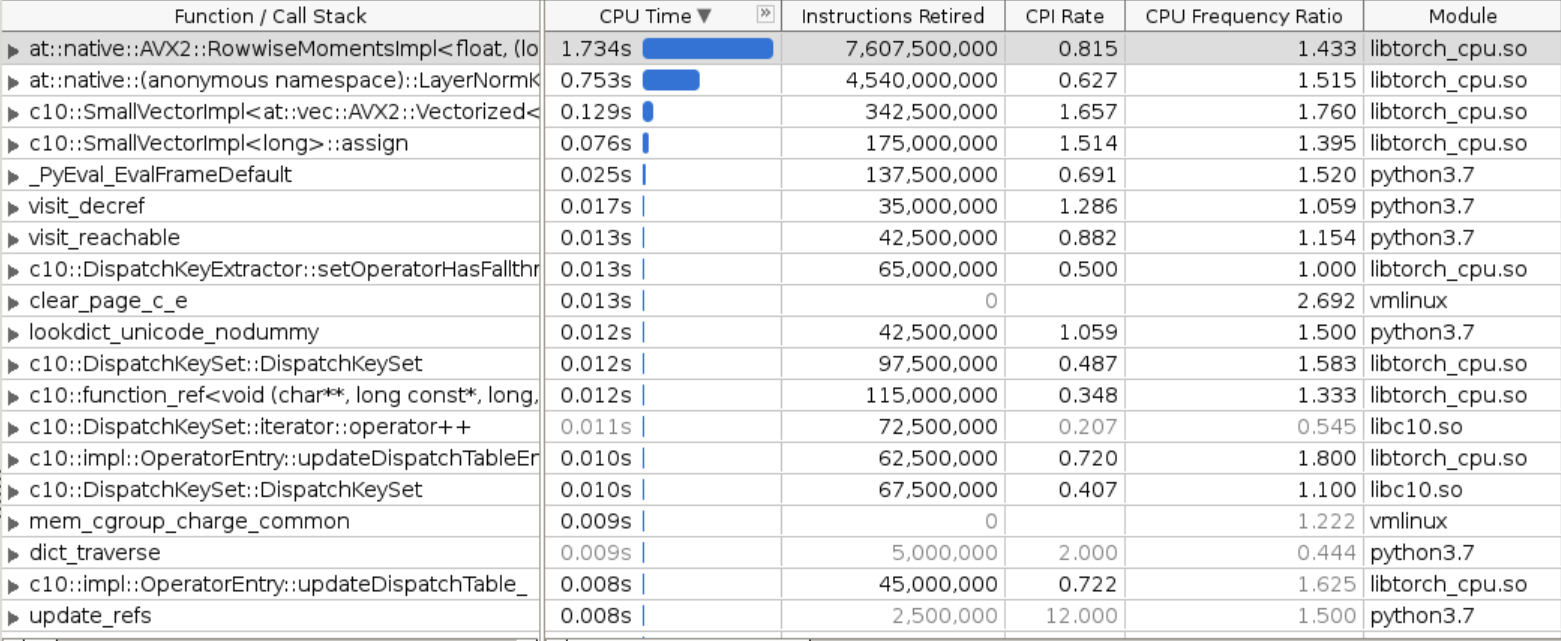 getting better. 4. after the fix, Instruction level breakdown of `RowwiseMomentsImpl<>` 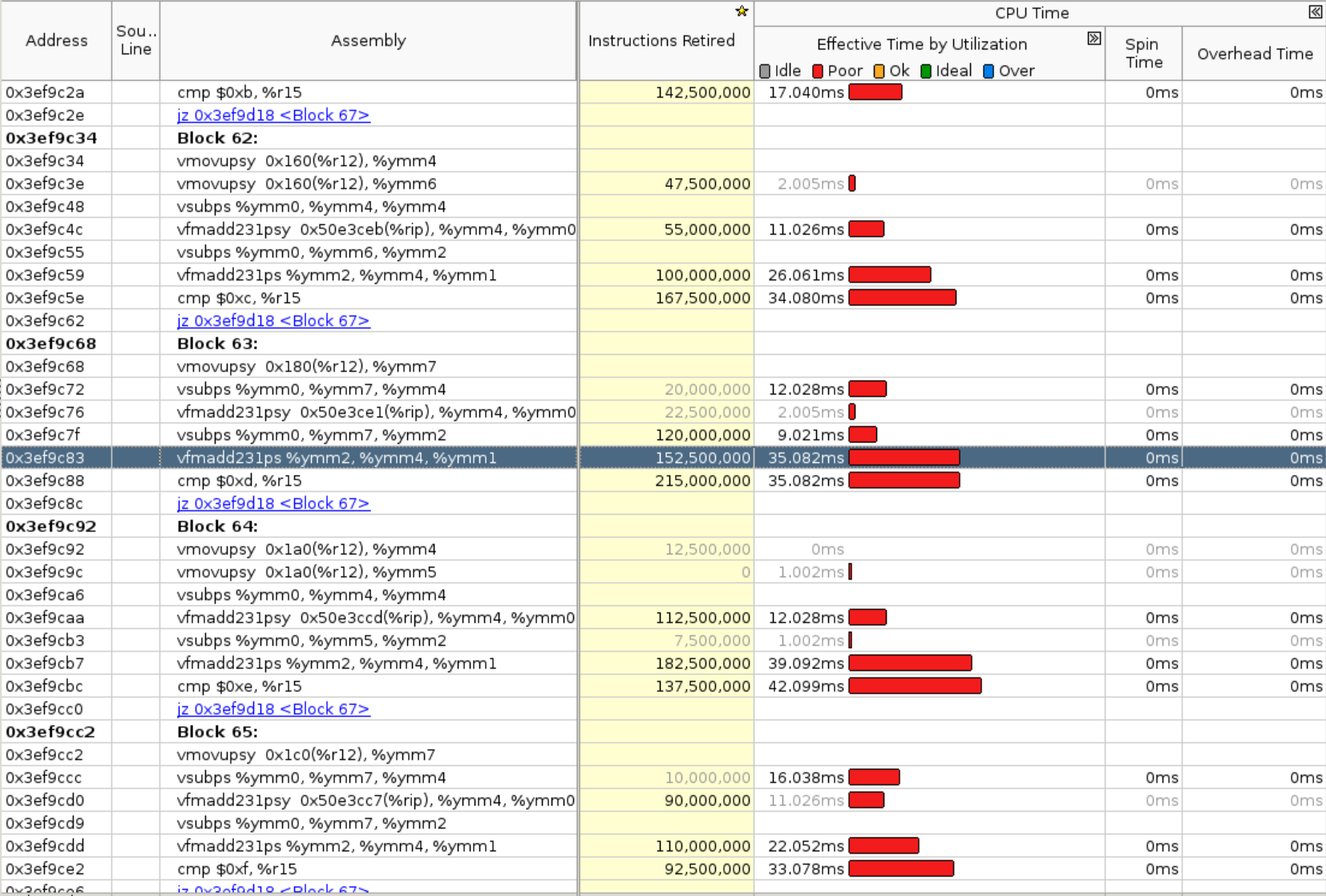 now it is all **vectorized** instructions. Pull Request resolved: pytorch#84404 Approved by: https://github.com/jgong5
- Loading branch information