forked from rasbt/LLMs-from-scratch
-
Notifications
You must be signed in to change notification settings - Fork 0
Commit
This commit does not belong to any branch on this repository, and may belong to a fork outside of the repository.
Add user interface to ch06 and ch07 (rasbt#366)
* Add user interface to ch06 and ch07 * pep8 * fix url
- Loading branch information
Showing
16 changed files
with
1,022 additions
and
58 deletions.
There are no files selected for viewing
This file contains bidirectional Unicode text that may be interpreted or compiled differently than what appears below. To review, open the file in an editor that reveals hidden Unicode characters.
Learn more about bidirectional Unicode characters
This file contains bidirectional Unicode text that may be interpreted or compiled differently than what appears below. To review, open the file in an editor that reveals hidden Unicode characters.
Learn more about bidirectional Unicode characters
This file contains bidirectional Unicode text that may be interpreted or compiled differently than what appears below. To review, open the file in an editor that reveals hidden Unicode characters.
Learn more about bidirectional Unicode characters
This file contains bidirectional Unicode text that may be interpreted or compiled differently than what appears below. To review, open the file in an editor that reveals hidden Unicode characters.
Learn more about bidirectional Unicode characters
This file contains bidirectional Unicode text that may be interpreted or compiled differently than what appears below. To review, open the file in an editor that reveals hidden Unicode characters.
Learn more about bidirectional Unicode characters
This file contains bidirectional Unicode text that may be interpreted or compiled differently than what appears below. To review, open the file in an editor that reveals hidden Unicode characters.
Learn more about bidirectional Unicode characters
This file contains bidirectional Unicode text that may be interpreted or compiled differently than what appears below. To review, open the file in an editor that reveals hidden Unicode characters.
Learn more about bidirectional Unicode characters
Original file line number | Diff line number | Diff line change |
---|---|---|
@@ -0,0 +1,39 @@ | ||
# Building a User Interface to Interact With the GPT-based Spam Classifier | ||
|
||
|
||
|
||
This bonus folder contains code for running a ChatGPT-like user interface to interact with the finetuned GPT-based spam classifier from chapter 6, as shown below. | ||
|
||
|
||
|
||
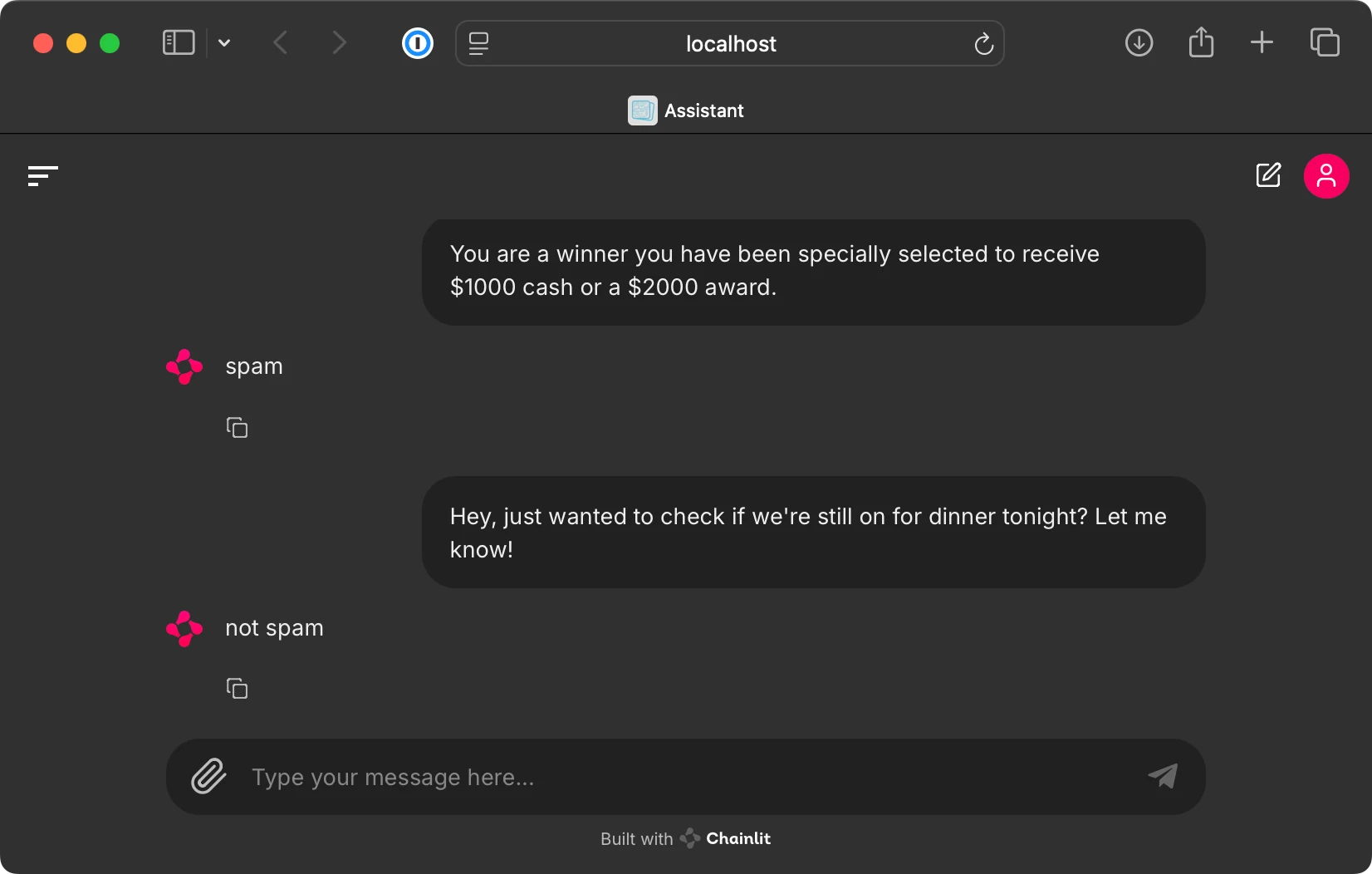 | ||
|
||
|
||
|
||
To implement this user interface, we use the open-source [Chainlit Python package](https://github.com/Chainlit/chainlit). | ||
|
||
| ||
## Step 1: Install dependencies | ||
|
||
First, we install the `chainlit` package via | ||
|
||
```bash | ||
pip install chainlit | ||
``` | ||
|
||
(Alternatively, execute `pip install -r requirements-extra.txt`.) | ||
|
||
| ||
## Step 2: Run `app` code | ||
|
||
The [`app.py`](app.py) file contains the UI code based. Open and inspect these files to learn more. | ||
|
||
This file loads and uses the GPT-2 classifier weights we generated in chapter 6. This requires that you execute the [`../01_main-chapter-code/ch06.ipynb`](../01_main-chapter-code/ch06.ipynb) file first. | ||
|
||
Excecute the following command from the terminal to start the UI server: | ||
|
||
```bash | ||
chainlit run app.py | ||
``` | ||
|
||
Running commands above should open a new browser tab where you can interact with the model. If the browser tab does not open automatically, inspect the terminal command and copy the local address into your browser address bar (usually, the address is `http://localhost:8000`). |
This file contains bidirectional Unicode text that may be interpreted or compiled differently than what appears below. To review, open the file in an editor that reveals hidden Unicode characters.
Learn more about bidirectional Unicode characters
Original file line number | Diff line number | Diff line change |
---|---|---|
@@ -0,0 +1,80 @@ | ||
# Copyright (c) Sebastian Raschka under Apache License 2.0 (see LICENSE.txt). | ||
# Source for "Build a Large Language Model From Scratch" | ||
# - https://www.manning.com/books/build-a-large-language-model-from-scratch | ||
# Code: https://github.com/rasbt/LLMs-from-scratch | ||
|
||
from pathlib import Path | ||
import sys | ||
|
||
import tiktoken | ||
import torch | ||
import chainlit | ||
|
||
from previous_chapters import ( | ||
classify_review, | ||
GPTModel | ||
) | ||
|
||
device = torch.device("cuda" if torch.cuda.is_available() else "cpu") | ||
|
||
|
||
def get_model_and_tokenizer(): | ||
""" | ||
Code to load finetuned GPT-2 model generated in chapter 6. | ||
This requires that you run the code in chapter 6 first, which generates the necessary model.pth file. | ||
""" | ||
|
||
GPT_CONFIG_124M = { | ||
"vocab_size": 50257, # Vocabulary size | ||
"context_length": 1024, # Context length | ||
"emb_dim": 768, # Embedding dimension | ||
"n_heads": 12, # Number of attention heads | ||
"n_layers": 12, # Number of layers | ||
"drop_rate": 0.1, # Dropout rate | ||
"qkv_bias": True # Query-key-value bias | ||
} | ||
|
||
tokenizer = tiktoken.get_encoding("gpt2") | ||
|
||
model_path = Path("..") / "01_main-chapter-code" / "review_classifier.pth" | ||
if not model_path.exists(): | ||
print( | ||
f"Could not find the {model_path} file. Please run the chapter 6 code" | ||
" (ch06.ipynb) to generate the review_classifier.pth file." | ||
) | ||
sys.exit() | ||
|
||
# Instantiate model | ||
model = GPTModel(GPT_CONFIG_124M) | ||
|
||
# Convert model to classifier as in section 6.5 in ch06.ipynb | ||
num_classes = 2 | ||
model.out_head = torch.nn.Linear(in_features=GPT_CONFIG_124M["emb_dim"], out_features=num_classes) | ||
|
||
# Then load model weights | ||
device = torch.device("cuda" if torch.cuda.is_available() else "cpu") | ||
checkpoint = torch.load(model_path, map_location=device, weights_only=True) | ||
model.load_state_dict(checkpoint) | ||
model.to(device) | ||
model.eval() | ||
|
||
return tokenizer, model | ||
|
||
|
||
# Obtain the necessary tokenizer and model files for the chainlit function below | ||
tokenizer, model = get_model_and_tokenizer() | ||
|
||
|
||
@chainlit.on_message | ||
async def main(message: chainlit.Message): | ||
""" | ||
The main Chainlit function. | ||
""" | ||
user_input = message.content | ||
device = torch.device("cuda" if torch.cuda.is_available() else "cpu") | ||
|
||
label = classify_review(user_input, model, tokenizer, device, max_length=120) | ||
|
||
await chainlit.Message( | ||
content=f"{label}", # This returns the model response to the interface | ||
).send() |
Oops, something went wrong.