forked from rasbt/LLMs-from-scratch
-
Notifications
You must be signed in to change notification settings - Fork 3
Commit
This commit does not belong to any branch on this repository, and may belong to a fork outside of the repository.
- Loading branch information
Showing
10 changed files
with
275 additions
and
1 deletion.
There are no files selected for viewing
This file contains bidirectional Unicode text that may be interpreted or compiled differently than what appears below. To review, open the file in an editor that reveals hidden Unicode characters.
Learn more about bidirectional Unicode characters
This file contains bidirectional Unicode text that may be interpreted or compiled differently than what appears below. To review, open the file in an editor that reveals hidden Unicode characters.
Learn more about bidirectional Unicode characters
Original file line number | Diff line number | Diff line change |
---|---|---|
@@ -0,0 +1,13 @@ | ||
# 第 5 章:对未标记数据进行预训练 | ||
|
||
### 主要章节代码 | ||
|
||
- [ch05.ipynb](ch05.ipynb) 包含本章中出现的所有代码 | ||
- [previous_chapters.py](previous_chapters.py) 是一个 Python 模块,其中包含前几章中的 `MultiHeadAttention` 模块和 `GPTModel` 类,我们在 [ch05.ipynb](ch05.ipynb) 中导入它们以预训练 GPT 模型 | ||
- [gpt_download.py](gpt_download.py) 包含用于下载预训练 GPT 模型权重的实用函数 | ||
- [exercise-solutions.ipynb](exercise-solutions.ipynb) 包含本章的练习解决方案 | ||
|
||
### 可选代码 | ||
|
||
- [gpt_train.py](gpt_train.py) 是一个独立的 Python 脚本文件,其中包含我们在[ch05.ipynb](ch05.ipynb) 用于训练 GPT 模型(你可以将其视为总结本章的代码文件) | ||
- [gpt_generate.py](gpt_generate.py) 是一个独立的 Python 脚本文件,其中包含我们在 [ch05.ipynb](ch05.ipynb) 中实现的代码,用于加载和使用来自 OpenAI 的预训练模型权重 |
This file contains bidirectional Unicode text that may be interpreted or compiled differently than what appears below. To review, open the file in an editor that reveals hidden Unicode characters.
Learn more about bidirectional Unicode characters
Original file line number | Diff line number | Diff line change |
---|---|---|
@@ -0,0 +1,5 @@ | ||
# 加载预训练权重的替代方法 | ||
|
||
此文件夹包含替代权重加载策略,以防 OpenAI 无法提供权重。 | ||
|
||
- [weight-loading-hf-transformers.ipynb](weight-loading-hf-transformers.ipynb):包含通过“transformers”库从 Hugging Face Model Hub 加载权重的代码 |
This file contains bidirectional Unicode text that may be interpreted or compiled differently than what appears below. To review, open the file in an editor that reveals hidden Unicode characters.
Learn more about bidirectional Unicode characters
Original file line number | Diff line number | Diff line change |
---|---|---|
@@ -0,0 +1,172 @@ | ||
# 在 Project Gutenberg 数据集上预训练 GPT | ||
|
||
此目录中的代码包含用于在 Project Gutenberg 提供的免费书籍上训练小型 GPT 模型的代码。 | ||
|
||
正如 Project Gutenberg 网站所述,“绝大多数 Project Gutenberg 电子书在美国属于公共领域。” | ||
|
||
请阅读 [Project Gutenberg 权限、许可和其他常见请求](https://www.gutenberg.org/policy/permission.html) 页面,了解有关使用 Project Gutenberg 提供的资源的更多信息。 | ||
|
||
| ||
## 如何使用此代码 | ||
|
||
| ||
|
||
### 1) 下载数据集 | ||
|
||
在本节中,我们使用来自 [`pgcorpus/gutenberg`](https://github.com/pgcorpus/gutenberg) GitHub 存储库的代码从 Project Gutenberg 下载书籍。 | ||
|
||
截至撰写本文时,这将需要大约 50 GB 的磁盘空间,大约需要 10-15 小时,但具体时间可能更长,具体取决于 Project Gutenberg 从那时起的发展程度。 | ||
|
||
| ||
#### Linux 和 macOS 用户的下载说明 | ||
|
||
Linux 和 macOS 用户可以按照以下步骤下载数据集(如果您是 Windows 用户,请参阅下面的说明): | ||
|
||
1. 将 `03_bonus_pretraining_on_gutenberg` 文件夹设置为工作目录,以在此文件夹中本地克隆 `gutenberg` 存储库(这对于运行提供的脚本 `prepare_dataset.py` 和 `pretraining_simple.py` 是必要的)。例如,当在 `LLMs-from-scratch` 存储库文件夹中时,通过以下方式导航到 *03_bonus_pretraining_on_gutenberg* 文件夹: | ||
```bash | ||
cd ch05/03_bonus_pretraining_on_gutenberg | ||
``` | ||
|
||
2. 克隆其中的 `gutenberg` 存储库: | ||
```bash | ||
git clone https://github.com/pgcorpus/gutenberg.git | ||
``` | ||
|
||
3. 导航到本地克隆的 `gutenberg` 存储库文件夹: | ||
```bash | ||
cd gutenberg | ||
``` | ||
|
||
4. 从 `gutenberg` 存储库文件夹安装 *requirements.txt* 中定义的所需软件包: | ||
```bash | ||
pip install -r requirements.txt | ||
``` | ||
|
||
5. 下载数据: | ||
```bash | ||
python get_data.py | ||
``` | ||
|
||
6. 返回`03_bonus_pretraining_on_gutenberg` 文件夹 | ||
```bash | ||
cd .. | ||
``` | ||
|
||
| ||
#### 针对 Windows 用户的特殊说明 | ||
|
||
[`pgcorpus/gutenberg`](https://github.com/pgcorpus/gutenberg) 代码兼容 Linux 和 macOS。但是,Windows 用户必须进行一些小调整,例如在 `subprocess` 调用中添加 `shell=True` 并替换 `rsync`。 | ||
|
||
或者,在 Windows 上运行此代码的更简单的方法是使用“Windows Subsystem for Linux”(WSL) 功能,该功能允许用户在 Windows 中使用 Ubuntu 运行 Linux 环境。有关更多信息,请阅读 [Microsoft 的官方安装说明](https://learn.microsoft.com/en-us/windows/wsl/install) 和 [教程](https://learn.microsoft.com/en-us/training/modules/wsl-introduction/)。 | ||
|
||
使用 WSL 时,请确保已安装 Python 3(通过 `python3 --version` 检查,或者例如使用 `sudo apt-get install -y python3.10` 安装 Python 3.10)并在那里安装以下软件包: | ||
|
||
```bash | ||
sudo apt-get update && \ | ||
sudo apt-get upgrade -y && \ | ||
sudo apt-get install -y python3-pip && \ | ||
sudo apt-get install -y python-is-python3 && \ | ||
sudo apt-get install -y rsync | ||
``` | ||
|
||
> [!NOTE] | ||
> 有关如何设置 Python 和安装软件包的说明,可在 [可选 Python 设置首选项](../../setup/01_optional-python-setup-preferences/README.md) 和 [安装 Python库](../../setup/02_installing-python-libraries/README.zh.md)。 | ||
> | ||
> 可选地,此存储库提供了一个运行 Ubuntu 的 Docker 映像。有关如何使用提供的 Docker 映像运行容器的说明,请参阅 [可选 Docker 环境](../../setup/03_optional-docker-environment/README.zh.md)。 | ||
| ||
### 2) 准备数据集 | ||
|
||
接下来,运行 `prepare_dataset.py` 脚本,该脚本将(截至撰写本文时,60,173 个)文本文件连接成更少的较大文件,以便更有效地传输和访问它们: | ||
|
||
```bash | ||
python prepare_dataset.py \ | ||
--data_dir gutenberg/data/raw \ | ||
--max_size_mb 500 \ | ||
--output_dir gutenberg_preprocessed | ||
``` | ||
|
||
``` | ||
... | ||
跳过 gutenberg/data/raw/PG29836_raw.txt,因为它不主要包含英文文本。跳过 gutenberg/data/raw/PG16527_raw.txt,因为它不主要包含英文文本。100%|████████████████████████████████████████████████████████| 57250/57250 [25:04<00:00, 38.05it/s] | ||
42 个文件保存在 /Users/sebastian/Developer/LLMs-from-scratch/ch05/03_bonus_pretraining_on_gutenberg/gutenberg_preprocessed | ||
``` | ||
|
||
> [!TIP] | ||
> 请注意,生成的文件以纯文本格式存储,并且为了简单起见未预先标记。但是,如果您计划更频繁地使用数据集或进行多个时期的训练,您可能需要更新代码以将数据集存储在预先标记的形式中以节省计算时间。有关更多信息,请参阅本页底部的 *设计决策和改进*。 | ||
> [!TIP] | ||
> 您可以选择较小的文件大小,例如示例,50 MB。这将产生更多文件,但对于在少量文件上进行更快的预训练运行以进行测试可能很有用。 | ||
| ||
### 3) 运行预训练脚本 | ||
|
||
您可以按如下方式运行预训练脚本。请注意,为了便于说明,附加命令行参数以默认值显示: | ||
|
||
```bash | ||
python pretraining_simple.py \ | ||
--data_dir "gutenberg_preprocessed" \ | ||
--n_epochs 1 \ | ||
--batch_size 4 \ | ||
--output_dir model_checkpoints | ||
``` | ||
|
||
输出将按以下方式格式化: | ||
|
||
> 文件总数:3 | ||
> 标记文件 1/3:data_small/combined_1.txt | ||
> 训练... | ||
> 第 1 步(步骤 0):训练损失 9.694,Val 损失 9.724 | ||
> 第 1 步(步骤 100):训练损失 6.672,Val 损失 6.683 | ||
> 第 1 步(步骤 200):训练损失 6.543,Val 损失 6.434 | ||
> 第 1 步(步骤 300):训练损失5.772,Val 损失 6.313 | ||
> Ep 1(步骤 400):训练损失 5.547,Val 损失 6.249 | ||
> Ep 1(步骤 500):训练损失 6.182,Val 损失 6.155 | ||
> Ep 1(步骤 600):训练损失 5.742,Val 损失 6.122 | ||
> Ep 1(步骤 700):训练损失 6.309,Val 损失 5.984 | ||
> Ep 1(步骤 800):训练损失 5.435,Val 损失 5.975 | ||
> Ep 1(步骤 900):训练损失 5.582,Val 损失 5.935 | ||
> ... | ||
> Ep 1(步骤 31900):训练损失 3.664,Val 损失 3.946 | ||
> Ep 1 (步骤 32000):训练损失 3.493,Val 损失 3.939 | ||
> 第 1 步 (步骤 32100):训练损失 3.940,Val 损失 3.961 | ||
> 已保存 model_checkpoints/model_pg_32188.pth | ||
> 处理书籍 3 小时 46 分 55 秒 | ||
> 总耗时 3 小时 46 分 55 秒 | ||
> 剩余书籍的预计到达时间:7 小时 33 分 50 秒 | ||
> 标记文件 2/3:data_small/combined_2.txt | ||
> 训练... | ||
> 第 1 步 (步骤 32200):训练损失 2.982,Val 损失 4.094 | ||
> 第 1 步 (步骤 32300):训练损失 3.920,Val 损失 4.097 | ||
> ... | ||
| ||
> [!TIP] | ||
> 实际上,如果您使用的是 macOS 或 Linux,我建议使用 `tee` 命令将日志输出保存到 `log.txt` 文件,并将其打印在终端上: | ||
```bash | ||
python -u pretraining_simple.py | tee log.txt | ||
``` | ||
|
||
| ||
> [!WARNING] | ||
> 请注意,在 V100 GPU 上对 `gutenberg_preprocessed` 文件夹中约 500 Mb 的文本文件之一进行训练大约需要 4 个小时。 | ||
> 该文件夹包含 47 个文件,大约需要 200 小时(超过 1 周)才能完成。您可能希望在较少的文件上运行它。 | ||
| ||
## 设计决策和改进 | ||
|
||
请注意,此代码侧重于保持简单和最小化,以用于教育目的。可以通过以下方式改进代码,以提高建模性能和训练效率: | ||
|
||
1. 修改 `prepare_dataset.py` 脚本,从每个书籍文件中删除 Gutenberg 样板文本。 | ||
|
||
2. 更新数据准备和加载实用程序,以预先标记数据集并将其保存为标记形式,这样在调用预训练脚本时就不必每次都重新标记。 | ||
|
||
3. 更新 `train_model_simple` 脚本,添加 [附录 D:为训练循环添加花哨功能](../../appendix-D/01_main-chapter-code/appendix-D.ipynb) 中介绍的功能,即余弦衰减、线性预热和梯度剪裁。 | ||
|
||
4. 更新预训练脚本以保存优化器状态(请参阅第 5 章中的 *5.4 在 PyTorch 中加载和保存权重* 部分;[ch05.ipynb](../../ch05/01_main-chapter-code/ch05.ipynb)),并添加加载现有模型和优化器检查点并在训练运行中断时继续训练的选项。 | ||
5. 添加更高级的记录器(例如,权重和偏差)以实时查看损失和验证曲线 | ||
6. 添加分布式数据并行 (DDP) 并在多个 GPU 上训练模型(请参阅附录 A 中的 *A.9.3 使用多个 GPU 进行训练* 部分;[DDP-script.py](../../appendix-A/01_main-chapter-code/DDP-script.py))。 | ||
7. 将 `previous_chapter.py` 脚本中从头开始的 `MultiheadAttention` 类与 [高效多头注意力实现](../../ch03/02_bonus_efficient-multihead-attention/mha-implementations.ipynb) 奖励部分中实现的高效 `MHAPyTorchScaledDotProduct` 类进行交换,该类通过 PyTorch 的 `nn. functional.scaled_dot_product_attention` 函数使用 Flash Attention。 | ||
8. 通过 [torch.compile](https://pytorch.org/tutorials/intermediate/torch_compile_tutorial.html) (`model = torch.compile`) 或 [thunder](https://github.com/Lightning-AI/lightning-thunder) (`model = thunder.jit(model)`) 优化模型,从而加快训练速度。 | ||
9. 实现梯度低秩投影 (GaLore),进一步加快预训练过程。只需将 `AdamW` 优化器替换为 [GaLore Python 库](https://github.com/jiaweizzhao/GaLore) 中提供的 `GaLoreAdamW` 即可实现。 |
This file contains bidirectional Unicode text that may be interpreted or compiled differently than what appears below. To review, open the file in an editor that reveals hidden Unicode characters.
Learn more about bidirectional Unicode characters
Original file line number | Diff line number | Diff line change |
---|---|---|
@@ -0,0 +1,5 @@ | ||
# 为训练循环添加花哨功能 | ||
|
||
主要章节使用了一个相对简单的训练函数,以保持代码的可读性,并使第 5 章符合页数限制。我们还可以添加线性预热、余弦衰减计划和梯度裁剪,以提高训练稳定性和收敛性。 | ||
|
||
您可以在 [附录 D:为训练循环添加花哨功能](../../appendix-D/01_main-chapter-code/appendix-D.ipynb) 中找到此更复杂的训练函数的代码。 |
This file contains bidirectional Unicode text that may be interpreted or compiled differently than what appears below. To review, open the file in an editor that reveals hidden Unicode characters.
Learn more about bidirectional Unicode characters
Original file line number | Diff line number | Diff line change |
---|---|---|
@@ -0,0 +1,6 @@ | ||
# 优化预训练的超参数 | ||
|
||
[hparam_search.py](hparam_search.py) 脚本基于 [附录 D:为训练循环添加花哨功能](../../appendix-D/01_main-chapter-code/appendix-D.ipynb) 中的扩展训练函数,旨在通过网格搜索找到最佳超参数。 | ||
|
||
>[!NOTE] | ||
此脚本将需要很长时间才能运行。您可能希望减少顶部 `HPARAM_GRID` 字典中探索的超参数配置数量。 |
This file contains bidirectional Unicode text that may be interpreted or compiled differently than what appears below. To review, open the file in an editor that reveals hidden Unicode characters.
Learn more about bidirectional Unicode characters
Original file line number | Diff line number | Diff line change |
---|---|---|
@@ -0,0 +1,42 @@ | ||
# 构建用户界面以与预训练的 LLM 交互 | ||
|
||
此奖励文件夹包含用于运行类似 ChatGPT 的用户界面的代码,以便与第 5 章中的预训练 LLM 交互,如下所示。 | ||
|
||
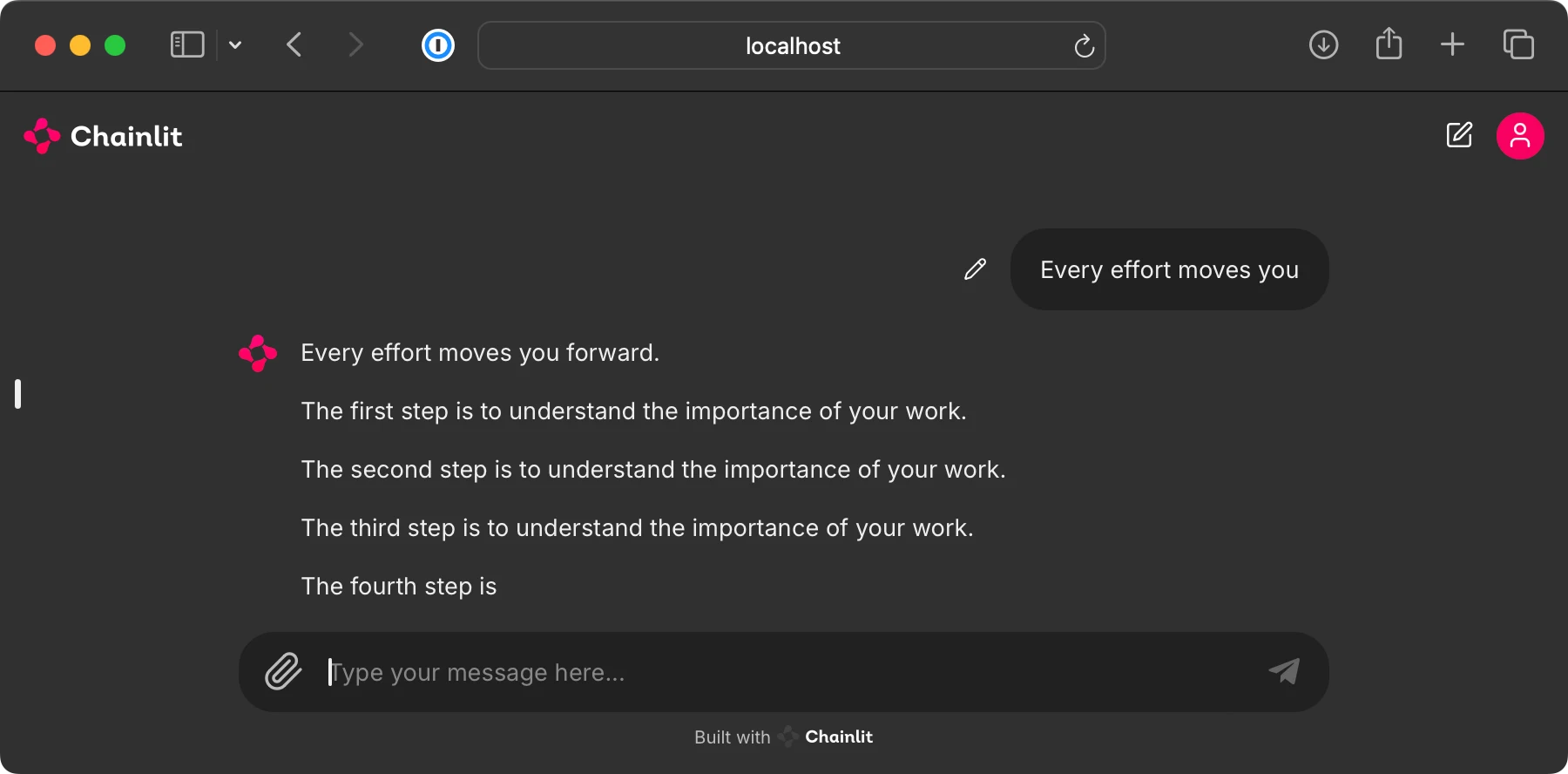 | ||
|
||
为了实现此用户界面,我们使用开源 [Chainlit Python 包](https://github.com/Chainlit/chainlit)。 | ||
|
||
| ||
## 步骤 1:安装依赖项 | ||
|
||
首先,我们通过 | ||
|
||
```bash | ||
pip install chainlit | ||
``` | ||
|
||
安装 `chainlit` 包(或者,执行 `pip install -r requirements-extra.txt`。) | ||
|
||
| ||
## 第 2 步:运行 `app` 代码 | ||
|
||
此文件夹包含 2 个文件: | ||
|
||
1. [`app_orig.py`](app_orig.py):此文件加载并使用来自 OpenAI 的原始 GPT-2 权重。 | ||
2. [`app_own.py`](app_own.py):此文件加载并使用我们在第 5 章中生成的 GPT-2 权重。这需要您先执行 [`../01_main-chapter-code/ch05.ipynb`](../01_main-chapter-code/ch05.ipynb) 文件。 | ||
|
||
(打开并检查这些文件以了解更多信息。) | ||
|
||
从终端运行以下命令之一以启动 UI 服务器: | ||
|
||
```bash | ||
chainlit run app_orig.py | ||
``` | ||
|
||
或 | ||
|
||
```bash | ||
chainlit run app_own.py | ||
``` | ||
|
||
运行上述命令之一应打开一个新的浏览器选项卡,您可以在其中与模型进行交互。如果浏览器选项卡未自动打开,请检查终端命令并将本地地址复制到浏览器地址栏中(通常,地址为 `http://localhost:8000`)。 |
This file contains bidirectional Unicode text that may be interpreted or compiled differently than what appears below. To review, open the file in an editor that reveals hidden Unicode characters.
Learn more about bidirectional Unicode characters
Original file line number | Diff line number | Diff line change |
---|---|---|
@@ -0,0 +1,9 @@ | ||
# 将 GPT 转换为 Llama | ||
|
||
此文件夹包含将第 4 章和第 5 章中的 GPT 实现转换为 Meta AI 的 Llama 架构的代码,建议阅读顺序如下: | ||
|
||
- [converting-gpt-to-llama2.ipynb](converting-gpt-to-llama2.ipynb):包含将 GPT 逐步转换为 Llama 2 7B 的代码,并从 Meta AI 加载预训练权重 | ||
- [converting-llama2-to-llama3.ipynb](converting-llama2-to-llama3.ipynb):包含将 Llama 2 模型转换为 Llama 3、Llama 3.1 和 Llama 3.2 的代码 | ||
- [standalone-llama32.ipynb](standalone-llama32.ipynb):实现 Llama 3.2 的独立笔记本 | ||
|
||
<img src="https://sebastianraschka.com/images/LLMs-from-scratch-images/bonus/gpt-to-llama/gpt-and-all-llamas.webp"> |
This file contains bidirectional Unicode text that may be interpreted or compiled differently than what appears below. To review, open the file in an editor that reveals hidden Unicode characters.
Learn more about bidirectional Unicode characters
Original file line number | Diff line number | Diff line change |
---|---|---|
@@ -0,0 +1,5 @@ | ||
# 内存高效模型权重加载 | ||
|
||
此文件夹包含代码,用于说明如何更高效地加载模型权重 | ||
|
||
- [memory-efficient-state-dict.ipynb](memory-efficient-state-dict.ipynb):包含代码,用于通过 PyTorch 的 `load_state_dict` 方法更高效地加载模型权重 |
This file contains bidirectional Unicode text that may be interpreted or compiled differently than what appears below. To review, open the file in an editor that reveals hidden Unicode characters.
Learn more about bidirectional Unicode characters
Original file line number | Diff line number | Diff line change |
---|---|---|
@@ -0,0 +1,17 @@ | ||
# 第 5 章:对未标记数据进行预训练 | ||
|
||
| ||
## 主要章节代码 | ||
|
||
- [01_main-chapter-code](01_main-chapter-code/README.zh.md) 包含主要章节代码 | ||
|
||
| ||
## 奖励材料 | ||
|
||
- [02_alternative_weight_loading](02_alternative_weight_loading/README.zh.md) 包含用于在 OpenAI 无法获取模型权重的情况下从其他位置加载 GPT 模型权重的代码 | ||
- [03_bonus_pretraining_on_gutenberg](03_bonus_pretraining_on_gutenberg/README.zh.md) 包含用于在 Project Gutenberg 的整个书籍语料库上对 LLM 进行更长时间预训练的代码 | ||
- [04_learning_rate_schedulers](04_learning_rate_schedulers/README.zh.md) 包含实现更复杂训练函数的代码,包括学习率调度程序和梯度裁剪 | ||
- [05_bonus_hparam_tuning](05_bonus_hparam_tuning/README.zh.md) 包含可选的超参数调整脚本 | ||
- [06_user_interface](06_user_interface/README.zh.md) 实现交互式用户界面以与预训练的 LLM 进行交互 | ||
- [07_gpt_to_llama](07_gpt_to_llama/README.zh.md) 包含将 GPT 架构实现转换为 Llama 3.2 的分步指南,并从 Meta AI 加载预训练权重 | ||
- [08_memory_efficient_weight_loading](08_memory_efficient_weight_loading/README.zh.md) 包含一个额外的笔记本,展示了如何更有效地通过 PyTorch 的 `load_state_dict` 方法加载模型权重 |