diff --git a/LICENSE b/LICENSE
new file mode 100644
index 0000000..5b13748
--- /dev/null
+++ b/LICENSE
@@ -0,0 +1,201 @@
+ Apache License
+ Version 2.0, January 2004
+ http://www.apache.org/licenses/
+
+ TERMS AND CONDITIONS FOR USE, REPRODUCTION, AND DISTRIBUTION
+
+ 1. Definitions.
+
+ "License" shall mean the terms and conditions for use, reproduction,
+ and distribution as defined by Sections 1 through 9 of this document.
+
+ "Licensor" shall mean the copyright owner or entity authorized by
+ the copyright owner that is granting the License.
+
+ "Legal Entity" shall mean the union of the acting entity and all
+ other entities that control, are controlled by, or are under common
+ control with that entity. For the purposes of this definition,
+ "control" means (i) the power, direct or indirect, to cause the
+ direction or management of such entity, whether by contract or
+ otherwise, or (ii) ownership of fifty percent (50%) or more of the
+ outstanding shares, or (iii) beneficial ownership of such entity.
+
+ "You" (or "Your") shall mean an individual or Legal Entity
+ exercising permissions granted by this License.
+
+ "Source" form shall mean the preferred form for making modifications,
+ including but not limited to software source code, documentation
+ source, and configuration files.
+
+ "Object" form shall mean any form resulting from mechanical
+ transformation or translation of a Source form, including but
+ not limited to compiled object code, generated documentation,
+ and conversions to other media types.
+
+ "Work" shall mean the work of authorship, whether in Source or
+ Object form, made available under the License, as indicated by a
+ copyright notice that is included in or attached to the work
+ (an example is provided in the Appendix below).
+
+ "Derivative Works" shall mean any work, whether in Source or Object
+ form, that is based on (or derived from) the Work and for which the
+ editorial revisions, annotations, elaborations, or other modifications
+ represent, as a whole, an original work of authorship. For the purposes
+ of this License, Derivative Works shall not include works that remain
+ separable from, or merely link (or bind by name) to the interfaces of,
+ the Work and Derivative Works thereof.
+
+ "Contribution" shall mean any work of authorship, including
+ the original version of the Work and any modifications or additions
+ to that Work or Derivative Works thereof, that is intentionally
+ submitted to Licensor for inclusion in the Work by the copyright owner
+ or by an individual or Legal Entity authorized to submit on behalf of
+ the copyright owner. For the purposes of this definition, "submitted"
+ means any form of electronic, verbal, or written communication sent
+ to the Licensor or its representatives, including but not limited to
+ communication on electronic mailing lists, source code control systems,
+ and issue tracking systems that are managed by, or on behalf of, the
+ Licensor for the purpose of discussing and improving the Work, but
+ excluding communication that is conspicuously marked or otherwise
+ designated in writing by the copyright owner as "Not a Contribution."
+
+ "Contributor" shall mean Licensor and any individual or Legal Entity
+ on behalf of whom a Contribution has been received by Licensor and
+ subsequently incorporated within the Work.
+
+ 2. Grant of Copyright License. Subject to the terms and conditions of
+ this License, each Contributor hereby grants to You a perpetual,
+ worldwide, non-exclusive, no-charge, royalty-free, irrevocable
+ copyright license to reproduce, prepare Derivative Works of,
+ publicly display, publicly perform, sublicense, and distribute the
+ Work and such Derivative Works in Source or Object form.
+
+ 3. Grant of Patent License. Subject to the terms and conditions of
+ this License, each Contributor hereby grants to You a perpetual,
+ worldwide, non-exclusive, no-charge, royalty-free, irrevocable
+ (except as stated in this section) patent license to make, have made,
+ use, offer to sell, sell, import, and otherwise transfer the Work,
+ where such license applies only to those patent claims licensable
+ by such Contributor that are necessarily infringed by their
+ Contribution(s) alone or by combination of their Contribution(s)
+ with the Work to which such Contribution(s) was submitted. If You
+ institute patent litigation against any entity (including a
+ cross-claim or counterclaim in a lawsuit) alleging that the Work
+ or a Contribution incorporated within the Work constitutes direct
+ or contributory patent infringement, then any patent licenses
+ granted to You under this License for that Work shall terminate
+ as of the date such litigation is filed.
+
+ 4. Redistribution. You may reproduce and distribute copies of the
+ Work or Derivative Works thereof in any medium, with or without
+ modifications, and in Source or Object form, provided that You
+ meet the following conditions:
+
+ (a) You must give any other recipients of the Work or
+ Derivative Works a copy of this License; and
+
+ (b) You must cause any modified files to carry prominent notices
+ stating that You changed the files; and
+
+ (c) You must retain, in the Source form of any Derivative Works
+ that You distribute, all copyright, patent, trademark, and
+ attribution notices from the Source form of the Work,
+ excluding those notices that do not pertain to any part of
+ the Derivative Works; and
+
+ (d) If the Work includes a "NOTICE" text file as part of its
+ distribution, then any Derivative Works that You distribute must
+ include a readable copy of the attribution notices contained
+ within such NOTICE file, excluding those notices that do not
+ pertain to any part of the Derivative Works, in at least one
+ of the following places: within a NOTICE text file distributed
+ as part of the Derivative Works; within the Source form or
+ documentation, if provided along with the Derivative Works; or,
+ within a display generated by the Derivative Works, if and
+ wherever such third-party notices normally appear. The contents
+ of the NOTICE file are for informational purposes only and
+ do not modify the License. You may add Your own attribution
+ notices within Derivative Works that You distribute, alongside
+ or as an addendum to the NOTICE text from the Work, provided
+ that such additional attribution notices cannot be construed
+ as modifying the License.
+
+ You may add Your own copyright statement to Your modifications and
+ may provide additional or different license terms and conditions
+ for use, reproduction, or distribution of Your modifications, or
+ for any such Derivative Works as a whole, provided Your use,
+ reproduction, and distribution of the Work otherwise complies with
+ the conditions stated in this License.
+
+ 5. Submission of Contributions. Unless You explicitly state otherwise,
+ any Contribution intentionally submitted for inclusion in the Work
+ by You to the Licensor shall be under the terms and conditions of
+ this License, without any additional terms or conditions.
+ Notwithstanding the above, nothing herein shall supersede or modify
+ the terms of any separate license agreement you may have executed
+ with Licensor regarding such Contributions.
+
+ 6. Trademarks. This License does not grant permission to use the trade
+ names, trademarks, service marks, or product names of the Licensor,
+ except as required for reasonable and customary use in describing the
+ origin of the Work and reproducing the content of the NOTICE file.
+
+ 7. Disclaimer of Warranty. Unless required by applicable law or
+ agreed to in writing, Licensor provides the Work (and each
+ Contributor provides its Contributions) on an "AS IS" BASIS,
+ WITHOUT WARRANTIES OR CONDITIONS OF ANY KIND, either express or
+ implied, including, without limitation, any warranties or conditions
+ of TITLE, NON-INFRINGEMENT, MERCHANTABILITY, or FITNESS FOR A
+ PARTICULAR PURPOSE. You are solely responsible for determining the
+ appropriateness of using or redistributing the Work and assume any
+ risks associated with Your exercise of permissions under this License.
+
+ 8. Limitation of Liability. In no event and under no legal theory,
+ whether in tort (including negligence), contract, or otherwise,
+ unless required by applicable law (such as deliberate and grossly
+ negligent acts) or agreed to in writing, shall any Contributor be
+ liable to You for damages, including any direct, indirect, special,
+ incidental, or consequential damages of any character arising as a
+ result of this License or out of the use or inability to use the
+ Work (including but not limited to damages for loss of goodwill,
+ work stoppage, computer failure or malfunction, or any and all
+ other commercial damages or losses), even if such Contributor
+ has been advised of the possibility of such damages.
+
+ 9. Accepting Warranty or Additional Liability. While redistributing
+ the Work or Derivative Works thereof, You may choose to offer,
+ and charge a fee for, acceptance of support, warranty, indemnity,
+ or other liability obligations and/or rights consistent with this
+ License. However, in accepting such obligations, You may act only
+ on Your own behalf and on Your sole responsibility, not on behalf
+ of any other Contributor, and only if You agree to indemnify,
+ defend, and hold each Contributor harmless for any liability
+ incurred by, or claims asserted against, such Contributor by reason
+ of your accepting any such warranty or additional liability.
+
+ END OF TERMS AND CONDITIONS
+
+ APPENDIX: How to apply the Apache License to your work.
+
+ To apply the Apache License to your work, attach the following
+ boilerplate notice, with the fields enclosed by brackets "[]"
+ replaced with your own identifying information. (Don't include
+ the brackets!) The text should be enclosed in the appropriate
+ comment syntax for the file format. We also recommend that a
+ file or class name and description of purpose be included on the
+ same "printed page" as the copyright notice for easier
+ identification within third-party archives.
+
+ Copyright 2021 Xiao Liu
+
+ Licensed under the Apache License, Version 2.0 (the "License");
+ you may not use this file except in compliance with the License.
+ You may obtain a copy of the License at
+
+ http://www.apache.org/licenses/LICENSE-2.0
+
+ Unless required by applicable law or agreed to in writing, software
+ distributed under the License is distributed on an "AS IS" BASIS,
+ WITHOUT WARRANTIES OR CONDITIONS OF ANY KIND, either express or implied.
+ See the License for the specific language governing permissions and
+ limitations under the License.
diff --git a/PT-Retrieval/.gitignore b/PT-Retrieval/.gitignore
new file mode 100644
index 0000000..952b01e
--- /dev/null
+++ b/PT-Retrieval/.gitignore
@@ -0,0 +1,9 @@
+checkpoints/
+encoded_files/
+.cache/
+beir_eval/datasets/
+beir_eval/preprocessed_data/
+mlruns/
+results/
+data/
+!dpr/data/
\ No newline at end of file
diff --git a/PT-Retrieval/LICENSE b/PT-Retrieval/LICENSE
new file mode 100755
index 0000000..50f2e65
--- /dev/null
+++ b/PT-Retrieval/LICENSE
@@ -0,0 +1,399 @@
+Attribution-NonCommercial 4.0 International
+
+=======================================================================
+
+Creative Commons Corporation ("Creative Commons") is not a law firm and
+does not provide legal services or legal advice. Distribution of
+Creative Commons public licenses does not create a lawyer-client or
+other relationship. Creative Commons makes its licenses and related
+information available on an "as-is" basis. Creative Commons gives no
+warranties regarding its licenses, any material licensed under their
+terms and conditions, or any related information. Creative Commons
+disclaims all liability for damages resulting from their use to the
+fullest extent possible.
+
+Using Creative Commons Public Licenses
+
+Creative Commons public licenses provide a standard set of terms and
+conditions that creators and other rights holders may use to share
+original works of authorship and other material subject to copyright
+and certain other rights specified in the public license below. The
+following considerations are for informational purposes only, are not
+exhaustive, and do not form part of our licenses.
+
+ Considerations for licensors: Our public licenses are
+ intended for use by those authorized to give the public
+ permission to use material in ways otherwise restricted by
+ copyright and certain other rights. Our licenses are
+ irrevocable. Licensors should read and understand the terms
+ and conditions of the license they choose before applying it.
+ Licensors should also secure all rights necessary before
+ applying our licenses so that the public can reuse the
+ material as expected. Licensors should clearly mark any
+ material not subject to the license. This includes other CC-
+ licensed material, or material used under an exception or
+ limitation to copyright. More considerations for licensors:
+ wiki.creativecommons.org/Considerations_for_licensors
+
+ Considerations for the public: By using one of our public
+ licenses, a licensor grants the public permission to use the
+ licensed material under specified terms and conditions. If
+ the licensor's permission is not necessary for any reason--for
+ example, because of any applicable exception or limitation to
+ copyright--then that use is not regulated by the license. Our
+ licenses grant only permissions under copyright and certain
+ other rights that a licensor has authority to grant. Use of
+ the licensed material may still be restricted for other
+ reasons, including because others have copyright or other
+ rights in the material. A licensor may make special requests,
+ such as asking that all changes be marked or described.
+ Although not required by our licenses, you are encouraged to
+ respect those requests where reasonable. More_considerations
+ for the public:
+ wiki.creativecommons.org/Considerations_for_licensees
+
+=======================================================================
+
+Creative Commons Attribution-NonCommercial 4.0 International Public
+License
+
+By exercising the Licensed Rights (defined below), You accept and agree
+to be bound by the terms and conditions of this Creative Commons
+Attribution-NonCommercial 4.0 International Public License ("Public
+License"). To the extent this Public License may be interpreted as a
+contract, You are granted the Licensed Rights in consideration of Your
+acceptance of these terms and conditions, and the Licensor grants You
+such rights in consideration of benefits the Licensor receives from
+making the Licensed Material available under these terms and
+conditions.
+
+Section 1 -- Definitions.
+
+ a. Adapted Material means material subject to Copyright and Similar
+ Rights that is derived from or based upon the Licensed Material
+ and in which the Licensed Material is translated, altered,
+ arranged, transformed, or otherwise modified in a manner requiring
+ permission under the Copyright and Similar Rights held by the
+ Licensor. For purposes of this Public License, where the Licensed
+ Material is a musical work, performance, or sound recording,
+ Adapted Material is always produced where the Licensed Material is
+ synched in timed relation with a moving image.
+
+ b. Adapter's License means the license You apply to Your Copyright
+ and Similar Rights in Your contributions to Adapted Material in
+ accordance with the terms and conditions of this Public License.
+
+ c. Copyright and Similar Rights means copyright and/or similar rights
+ closely related to copyright including, without limitation,
+ performance, broadcast, sound recording, and Sui Generis Database
+ Rights, without regard to how the rights are labeled or
+ categorized. For purposes of this Public License, the rights
+ specified in Section 2(b)(1)-(2) are not Copyright and Similar
+ Rights.
+ d. Effective Technological Measures means those measures that, in the
+ absence of proper authority, may not be circumvented under laws
+ fulfilling obligations under Article 11 of the WIPO Copyright
+ Treaty adopted on December 20, 1996, and/or similar international
+ agreements.
+
+ e. Exceptions and Limitations means fair use, fair dealing, and/or
+ any other exception or limitation to Copyright and Similar Rights
+ that applies to Your use of the Licensed Material.
+
+ f. Licensed Material means the artistic or literary work, database,
+ or other material to which the Licensor applied this Public
+ License.
+
+ g. Licensed Rights means the rights granted to You subject to the
+ terms and conditions of this Public License, which are limited to
+ all Copyright and Similar Rights that apply to Your use of the
+ Licensed Material and that the Licensor has authority to license.
+
+ h. Licensor means the individual(s) or entity(ies) granting rights
+ under this Public License.
+
+ i. NonCommercial means not primarily intended for or directed towards
+ commercial advantage or monetary compensation. For purposes of
+ this Public License, the exchange of the Licensed Material for
+ other material subject to Copyright and Similar Rights by digital
+ file-sharing or similar means is NonCommercial provided there is
+ no payment of monetary compensation in connection with the
+ exchange.
+
+ j. Share means to provide material to the public by any means or
+ process that requires permission under the Licensed Rights, such
+ as reproduction, public display, public performance, distribution,
+ dissemination, communication, or importation, and to make material
+ available to the public including in ways that members of the
+ public may access the material from a place and at a time
+ individually chosen by them.
+
+ k. Sui Generis Database Rights means rights other than copyright
+ resulting from Directive 96/9/EC of the European Parliament and of
+ the Council of 11 March 1996 on the legal protection of databases,
+ as amended and/or succeeded, as well as other essentially
+ equivalent rights anywhere in the world.
+
+ l. You means the individual or entity exercising the Licensed Rights
+ under this Public License. Your has a corresponding meaning.
+
+Section 2 -- Scope.
+
+ a. License grant.
+
+ 1. Subject to the terms and conditions of this Public License,
+ the Licensor hereby grants You a worldwide, royalty-free,
+ non-sublicensable, non-exclusive, irrevocable license to
+ exercise the Licensed Rights in the Licensed Material to:
+
+ a. reproduce and Share the Licensed Material, in whole or
+ in part, for NonCommercial purposes only; and
+
+ b. produce, reproduce, and Share Adapted Material for
+ NonCommercial purposes only.
+
+ 2. Exceptions and Limitations. For the avoidance of doubt, where
+ Exceptions and Limitations apply to Your use, this Public
+ License does not apply, and You do not need to comply with
+ its terms and conditions.
+
+ 3. Term. The term of this Public License is specified in Section
+ 6(a).
+
+ 4. Media and formats; technical modifications allowed. The
+ Licensor authorizes You to exercise the Licensed Rights in
+ all media and formats whether now known or hereafter created,
+ and to make technical modifications necessary to do so. The
+ Licensor waives and/or agrees not to assert any right or
+ authority to forbid You from making technical modifications
+ necessary to exercise the Licensed Rights, including
+ technical modifications necessary to circumvent Effective
+ Technological Measures. For purposes of this Public License,
+ simply making modifications authorized by this Section 2(a)
+ (4) never produces Adapted Material.
+
+ 5. Downstream recipients.
+
+ a. Offer from the Licensor -- Licensed Material. Every
+ recipient of the Licensed Material automatically
+ receives an offer from the Licensor to exercise the
+ Licensed Rights under the terms and conditions of this
+ Public License.
+
+ b. No downstream restrictions. You may not offer or impose
+ any additional or different terms or conditions on, or
+ apply any Effective Technological Measures to, the
+ Licensed Material if doing so restricts exercise of the
+ Licensed Rights by any recipient of the Licensed
+ Material.
+
+ 6. No endorsement. Nothing in this Public License constitutes or
+ may be construed as permission to assert or imply that You
+ are, or that Your use of the Licensed Material is, connected
+ with, or sponsored, endorsed, or granted official status by,
+ the Licensor or others designated to receive attribution as
+ provided in Section 3(a)(1)(A)(i).
+
+ b. Other rights.
+
+ 1. Moral rights, such as the right of integrity, are not
+ licensed under this Public License, nor are publicity,
+ privacy, and/or other similar personality rights; however, to
+ the extent possible, the Licensor waives and/or agrees not to
+ assert any such rights held by the Licensor to the limited
+ extent necessary to allow You to exercise the Licensed
+ Rights, but not otherwise.
+
+ 2. Patent and trademark rights are not licensed under this
+ Public License.
+
+ 3. To the extent possible, the Licensor waives any right to
+ collect royalties from You for the exercise of the Licensed
+ Rights, whether directly or through a collecting society
+ under any voluntary or waivable statutory or compulsory
+ licensing scheme. In all other cases the Licensor expressly
+ reserves any right to collect such royalties, including when
+ the Licensed Material is used other than for NonCommercial
+ purposes.
+
+Section 3 -- License Conditions.
+
+Your exercise of the Licensed Rights is expressly made subject to the
+following conditions.
+
+ a. Attribution.
+
+ 1. If You Share the Licensed Material (including in modified
+ form), You must:
+
+ a. retain the following if it is supplied by the Licensor
+ with the Licensed Material:
+
+ i. identification of the creator(s) of the Licensed
+ Material and any others designated to receive
+ attribution, in any reasonable manner requested by
+ the Licensor (including by pseudonym if
+ designated);
+
+ ii. a copyright notice;
+
+ iii. a notice that refers to this Public License;
+
+ iv. a notice that refers to the disclaimer of
+ warranties;
+
+ v. a URI or hyperlink to the Licensed Material to the
+ extent reasonably practicable;
+
+ b. indicate if You modified the Licensed Material and
+ retain an indication of any previous modifications; and
+
+ c. indicate the Licensed Material is licensed under this
+ Public License, and include the text of, or the URI or
+ hyperlink to, this Public License.
+
+ 2. You may satisfy the conditions in Section 3(a)(1) in any
+ reasonable manner based on the medium, means, and context in
+ which You Share the Licensed Material. For example, it may be
+ reasonable to satisfy the conditions by providing a URI or
+ hyperlink to a resource that includes the required
+ information.
+
+ 3. If requested by the Licensor, You must remove any of the
+ information required by Section 3(a)(1)(A) to the extent
+ reasonably practicable.
+
+ 4. If You Share Adapted Material You produce, the Adapter's
+ License You apply must not prevent recipients of the Adapted
+ Material from complying with this Public License.
+
+Section 4 -- Sui Generis Database Rights.
+
+Where the Licensed Rights include Sui Generis Database Rights that
+apply to Your use of the Licensed Material:
+
+ a. for the avoidance of doubt, Section 2(a)(1) grants You the right
+ to extract, reuse, reproduce, and Share all or a substantial
+ portion of the contents of the database for NonCommercial purposes
+ only;
+
+ b. if You include all or a substantial portion of the database
+ contents in a database in which You have Sui Generis Database
+ Rights, then the database in which You have Sui Generis Database
+ Rights (but not its individual contents) is Adapted Material; and
+
+ c. You must comply with the conditions in Section 3(a) if You Share
+ all or a substantial portion of the contents of the database.
+
+For the avoidance of doubt, this Section 4 supplements and does not
+replace Your obligations under this Public License where the Licensed
+Rights include other Copyright and Similar Rights.
+
+Section 5 -- Disclaimer of Warranties and Limitation of Liability.
+
+ a. UNLESS OTHERWISE SEPARATELY UNDERTAKEN BY THE LICENSOR, TO THE
+ EXTENT POSSIBLE, THE LICENSOR OFFERS THE LICENSED MATERIAL AS-IS
+ AND AS-AVAILABLE, AND MAKES NO REPRESENTATIONS OR WARRANTIES OF
+ ANY KIND CONCERNING THE LICENSED MATERIAL, WHETHER EXPRESS,
+ IMPLIED, STATUTORY, OR OTHER. THIS INCLUDES, WITHOUT LIMITATION,
+ WARRANTIES OF TITLE, MERCHANTABILITY, FITNESS FOR A PARTICULAR
+ PURPOSE, NON-INFRINGEMENT, ABSENCE OF LATENT OR OTHER DEFECTS,
+ ACCURACY, OR THE PRESENCE OR ABSENCE OF ERRORS, WHETHER OR NOT
+ KNOWN OR DISCOVERABLE. WHERE DISCLAIMERS OF WARRANTIES ARE NOT
+ ALLOWED IN FULL OR IN PART, THIS DISCLAIMER MAY NOT APPLY TO YOU.
+
+ b. TO THE EXTENT POSSIBLE, IN NO EVENT WILL THE LICENSOR BE LIABLE
+ TO YOU ON ANY LEGAL THEORY (INCLUDING, WITHOUT LIMITATION,
+ NEGLIGENCE) OR OTHERWISE FOR ANY DIRECT, SPECIAL, INDIRECT,
+ INCIDENTAL, CONSEQUENTIAL, PUNITIVE, EXEMPLARY, OR OTHER LOSSES,
+ COSTS, EXPENSES, OR DAMAGES ARISING OUT OF THIS PUBLIC LICENSE OR
+ USE OF THE LICENSED MATERIAL, EVEN IF THE LICENSOR HAS BEEN
+ ADVISED OF THE POSSIBILITY OF SUCH LOSSES, COSTS, EXPENSES, OR
+ DAMAGES. WHERE A LIMITATION OF LIABILITY IS NOT ALLOWED IN FULL OR
+ IN PART, THIS LIMITATION MAY NOT APPLY TO YOU.
+
+ c. The disclaimer of warranties and limitation of liability provided
+ above shall be interpreted in a manner that, to the extent
+ possible, most closely approximates an absolute disclaimer and
+ waiver of all liability.
+
+Section 6 -- Term and Termination.
+
+ a. This Public License applies for the term of the Copyright and
+ Similar Rights licensed here. However, if You fail to comply with
+ this Public License, then Your rights under this Public License
+ terminate automatically.
+
+ b. Where Your right to use the Licensed Material has terminated under
+ Section 6(a), it reinstates:
+
+ 1. automatically as of the date the violation is cured, provided
+ it is cured within 30 days of Your discovery of the
+ violation; or
+
+ 2. upon express reinstatement by the Licensor.
+
+ For the avoidance of doubt, this Section 6(b) does not affect any
+ right the Licensor may have to seek remedies for Your violations
+ of this Public License.
+
+ c. For the avoidance of doubt, the Licensor may also offer the
+ Licensed Material under separate terms or conditions or stop
+ distributing the Licensed Material at any time; however, doing so
+ will not terminate this Public License.
+
+ d. Sections 1, 5, 6, 7, and 8 survive termination of this Public
+ License.
+
+Section 7 -- Other Terms and Conditions.
+
+ a. The Licensor shall not be bound by any additional or different
+ terms or conditions communicated by You unless expressly agreed.
+
+ b. Any arrangements, understandings, or agreements regarding the
+ Licensed Material not stated herein are separate from and
+ independent of the terms and conditions of this Public License.
+
+Section 8 -- Interpretation.
+
+ a. For the avoidance of doubt, this Public License does not, and
+ shall not be interpreted to, reduce, limit, restrict, or impose
+ conditions on any use of the Licensed Material that could lawfully
+ be made without permission under this Public License.
+
+ b. To the extent possible, if any provision of this Public License is
+ deemed unenforceable, it shall be automatically reformed to the
+ minimum extent necessary to make it enforceable. If the provision
+ cannot be reformed, it shall be severed from this Public License
+ without affecting the enforceability of the remaining terms and
+ conditions.
+
+ c. No term or condition of this Public License will be waived and no
+ failure to comply consented to unless expressly agreed to by the
+ Licensor.
+
+ d. Nothing in this Public License constitutes or may be interpreted
+ as a limitation upon, or waiver of, any privileges and immunities
+ that apply to the Licensor or You, including from the legal
+ processes of any jurisdiction or authority.
+
+=======================================================================
+
+Creative Commons is not a party to its public
+licenses. Notwithstanding, Creative Commons may elect to apply one of
+its public licenses to material it publishes and in those instances
+will be considered the “Licensor.” The text of the Creative Commons
+public licenses is dedicated to the public domain under the CC0 Public
+Domain Dedication. Except for the limited purpose of indicating that
+material is shared under a Creative Commons public license or as
+otherwise permitted by the Creative Commons policies published at
+creativecommons.org/policies, Creative Commons does not authorize the
+use of the trademark "Creative Commons" or any other trademark or logo
+of Creative Commons without its prior written consent including,
+without limitation, in connection with any unauthorized modifications
+to any of its public licenses or any other arrangements,
+understandings, or agreements concerning use of licensed material. For
+the avoidance of doubt, this paragraph does not form part of the
+public licenses.
+
+Creative Commons may be contacted at creativecommons.org.
\ No newline at end of file
diff --git a/PT-Retrieval/README.md b/PT-Retrieval/README.md
new file mode 100755
index 0000000..27c04f4
--- /dev/null
+++ b/PT-Retrieval/README.md
@@ -0,0 +1,207 @@
+# Parameter-Efficient Prompt Tuning Makes Generalized and Calibrated Neural Text Retrievers
+
+Parameter-efficient learning can boost cross-domain and cross-topic generalization and calibration. [[Paper]](https://arxiv.org/pdf/2207.07087.pdf)
+
+
+
+
+
+## Setup
+Create the python environment via `Anaconda3`:
+```bash
+conda create -n pt-retrieval -y python=3.8.13
+conda activate pt-retrieval
+```
+
+Install the necessary python packages. Change `cudatoolkit` version according to your environment (`11.3` in our experiment).
+
+```bash
+conda install -n pt-retrieval pytorch==1.12.0 torchvision==0.13.0 torchaudio==0.12.0 cudatoolkit=11.3 -c pytorch
+pip install -r requirements.txt
+```
+
+(Optional) To train with Adapter, install Adapter-transformers
+
+```bash
+pip install -U adapter-transformers
+```
+
+## Data
+
+### OpenQA
+
+We use the preprocessed data provided by DPR which can be downloaded from the cloud using `download_data.py`. One needs to specify the resource name to be downloaded. Run `python download_data.py'`to see all options.
+
+```bash
+python data/download_data.py \
+ --resource {key from download_data.py's RESOURCES_MAP}
+```
+
+The keys of the five datasets and retrieval corpus we used in our experiments are:
+
+- `data.retriever.nq` and `data.retriever.qas.nq`
+- `data.retriever.trivia` and `data.retriever.qas.trivia`
+- `data.retriever.squad1` and `data.retriever.qas.squad1`
+- `data.retriever.webq` and `data.retriever.qas.webq`
+- `data.retriever.curatedtre` and `data.retriever.qas.curatedtrec`
+- `data.wikipedia_split.psgs_w100`
+
+**NOTE**: The resource name matching is prefix-based. So if you need to download all training data, just use ` --resource data.retriever`.
+
+### BEIR
+
+Run the evaluation script for DPR on BEIR and the script will download the dataset automatically. The decompressed data will be saved at `./beir_eval/datasets`.
+
+Or you can download the data manually via:
+
+```bash
+wget https://public.ukp.informatik.tu-darmstadt.de/thakur/BEIR/datasets/$dataset.zip
+```
+
+### OAG-QA
+
+OAG-QA is the largest public topic-specific passage retrieval dataset, which consists of 17,948 unique queries from 22 scientific disciplines and 87 fine-grained topics.
+The queries and reference papers are collected from professional forums such as [Zhihu](https://zhihu.com) and [StackExchange](https://stackexchange.com), and mapped to papers in the [Open Academic Graph](https://www.aminer.cn/oag-2-1) with rich meta-information (e.g. abstract, field-of-study (FOS)).
+
+Download OAG-QA from the link: [OAG-QA](https://drive.google.com/file/d/1jEAzWq_J0cXz1pvT9nQ2s2ooGdcR8r6-/view?usp=sharing), and unzip it to `./data/oagqa-topic-v2`
+
+
+## Training
+
+### DPR
+
+Five training modes are supported in the code and the corresponding training scripts are provided:
+
+- Fine-tuning
+- P-tuning v2
+- BitFit
+- Adapter
+- Lester et al. & P-tuning
+
+Run training scripts in `./run_scripts`, for example:
+
+```bash
+bash run_scripts/run_train_dpr_multidata_ptv2.sh
+```
+
+### ColBERT
+
+P-Tuning v2 and the original finetuning are supported in colbert.
+
+```bash
+cd colbert
+bash scripts/run_train_colbert_ptv2.sh
+```
+
+
+
+## Checkpoints for Reproduce
+Download the checkpoints we use in the experiments to reproduce our results in the paper.
+Change `--model_file ./checkpoints/$filename/$checkpoint` in each evaluation script `*.sh` from `./eval_scripts` to load them.
+
+| Checkpoints | DPR | ColBERT |
+| ----------- | ------------------------------------------------------------ | ------------------------------------------------------------ |
+| P-tuning v2 | [Download](https://drive.google.com/file/d/1jVVndoHScqMMcJOBcJi2lVQvIhKAwaGN/view?usp=sharing) | [Download](https://drive.google.com/file/d/1JZYmRKoobs4vfaIXsEX_DNkluKmXxmzD/view?usp=sharing) |
+| Fine-tune | [Download](https://dl.fbaipublicfiles.com/dpr/checkpoint/retriver/multiset/hf_bert_base.cp) | [Download](https://public.ukp.informatik.tu-darmstadt.de/thakur/BEIR/models/ColBERT/msmarco.psg.l2.zip) |
+
+
+
+## Evaluation
+
+### DPR
+
+#### 1. OpenQA
+
+Inference is divied as two steps.
+
+- **Step 1**: Generate representation vectors for the static documents dataset via:
+
+```bash
+bash eval_scripts/generate_wiki_embeddings.sh
+```
+
+- **Step 2**: Retrieve for the validation question set from the entire set of candidate documents and calculate the top-k retrieval accuracy. To select the validation dataset, you can replace `$dataset` with `nq`, `trivia`, `webq` or `curatedtrec`.
+
+```bash
+bash eval_scripts/evaluate_on_openqa.sh $dataset $top-k
+```
+
+#### 2. BEIR
+
+Evaluate DPR on BEIR via:
+
+```bash
+bash eval_scripts/evaluate_on_beir.sh $dataset
+```
+
+You can choose `$dataset` from 15 datasets from BEIR, which can be referred to [BEIR](https://github.com/beir-cellar/beir#beers-available-datasets).
+
+#### 3. OAG-QA
+
+There are 87 topics in OAG-QA and you can choose any topic to run the evaluation via:
+
+```bash
+bash eval_scripts/evaluate_on_oagqa.sh $topic $top-k
+```
+
+### ColBERT
+
+#### 1. BEIR
+
+Similar to the BEIR evaluation for DPR, run the script to evaluate ColBERT on BEIR:
+
+```bash
+cd colbert
+bash scripts/evalute_on_beir.sh $dataset
+```
+
+
+
+## Calibration for DPR
+
+#### OAG-QA
+
+Plot the calibration curve and calculate ECE via:
+
+```bash
+bash calibration_on_openqa.sh $dataset
+```
+
+#### BEIR
+
+Plot the calibration curve and calculate ECE via:
+
+```bash
+bash eval_scripts/calibration_on_beir.sh $dataset
+```
+
+
+
+## Citation
+If you find this paper and repo useful, please consider citing us in your work
+
+```
+@article{WLTam2022PT-Retrieval,
+ author = {Weng Lam Tam and
+ Xiao Liu and
+ Kaixuan Ji and
+ Lilong Xue and
+ Xingjian Zhang and
+ Yuxiao Dong and
+ Jiahua Liu and
+ Maodi Hu and
+ Jie Tang},
+ title = {Parameter-Efficient Prompt Tuning Makes Generalized and Calibrated
+ Neural Text Retrievers},
+ journal = {CoRR},
+ volume = {abs/2207.07087},
+ year = {2022},
+ url = {https://doi.org/10.48550/arXiv.2207.07087},
+ doi = {10.48550/arXiv.2207.07087},
+ eprinttype = {arXiv},
+ eprint = {2207.07087},
+ timestamp = {Tue, 19 Jul 2022 17:45:18 +0200},
+ biburl = {https://dblp.org/rec/journals/corr/abs-2207-07087.bib},
+ bibsource = {dblp computer science bibliography, https://dblp.org}
+}
+```
diff --git a/PT-Retrieval/beir_eval/__init__.py b/PT-Retrieval/beir_eval/__init__.py
new file mode 100755
index 0000000..e69de29
diff --git a/PT-Retrieval/beir_eval/evaluate_dpr_on_beir.py b/PT-Retrieval/beir_eval/evaluate_dpr_on_beir.py
new file mode 100755
index 0000000..2e4e811
--- /dev/null
+++ b/PT-Retrieval/beir_eval/evaluate_dpr_on_beir.py
@@ -0,0 +1,90 @@
+from beir import util, LoggingHandler
+from beir.datasets.data_loader import GenericDataLoader
+from beir.retrieval.evaluation import EvaluateRetrieval
+from beir.retrieval.search.dense import DenseRetrievalExactSearch as DRES
+
+from models import DPRForBeir
+
+import sys
+from pathlib import Path
+BASE_DIR = Path.resolve(Path(__file__)).parent.parent
+sys.path.append(str(BASE_DIR))
+print(BASE_DIR)
+
+
+from dpr.options import add_encoder_params, setup_args_gpu, \
+ add_tokenizer_params, add_cuda_params, add_tuning_params
+
+import argparse
+import logging
+import pathlib, os
+import random
+
+#### Just some code to print debug information to stdout
+logging.basicConfig(format='%(asctime)s - %(message)s',
+ datefmt='%Y-%m-%d %H:%M:%S',
+ level=logging.INFO,
+ handlers=[LoggingHandler()])
+#### /print debug information to stdout
+
+parser = argparse.ArgumentParser()
+
+add_encoder_params(parser)
+add_tokenizer_params(parser)
+add_cuda_params(parser)
+add_tuning_params(parser)
+
+parser.add_argument('--batch_size', type=int, default=32, help="Batch size for the passage encoder forward pass")
+parser.add_argument('--dataset', type=str)
+parser.add_argument('--split', type=str, default="text")
+
+args = parser.parse_args()
+args.dpr_mode = False
+args.fix_ctx_encoder = False
+args.use_projection = False
+
+setup_args_gpu(args)
+
+model = DRES(DPRForBeir(args), batch_size=args.batch_size)
+
+dataset = args.dataset
+
+data_path = os.path.join(pathlib.Path(__file__).parent.absolute(), "datasets", dataset)
+
+logging.info("evaluating dataset %s..." % dataset)
+logging.info(data_path)
+if not os.path.exists(data_path):
+ #### Download NFCorpus dataset and unzip the dataset
+ url = "https://public.ukp.informatik.tu-darmstadt.de/thakur/BEIR/datasets/{}.zip".format(dataset)
+ out_dir = os.path.join(pathlib.Path(__file__).parent.absolute(), "datasets")
+ util.download_and_unzip(url, out_dir)
+ data_path = os.path.join(out_dir, dataset)
+
+#### Provide the data path where nfcorpus has been downloaded and unzipped to the data loader
+# data folder would contain these files:
+# (1) nfcorpus/corpus.jsonl (format: jsonlines)
+# (2) nfcorpus/queries.jsonl (format: jsonlines)
+# (3) nfcorpus/qrels/test.tsv (format: tsv ("\t"))
+corpus, queries, qrels = GenericDataLoader(data_folder=data_path).load(args.split)
+
+retriever = EvaluateRetrieval(model, score_function="dot")
+
+#### Retrieve dense results (format of results is identical to qrels)
+results = retriever.retrieve(corpus, queries)
+
+#### Evaluate your retrieval using NDCG@k, MAP@K ...
+
+logging.info("Retriever evaluation for k in: {}".format(retriever.k_values))
+ndcg, _map, recall, precision = retriever.evaluate(qrels, results, retriever.k_values)
+
+#### Print top-k documents retrieved ####
+top_k = 10
+
+query_id, ranking_scores = random.choice(list(results.items()))
+scores_sorted = sorted(ranking_scores.items(), key=lambda item: item[1], reverse=True)
+# logging.info("Query : %s\n" % queries[query_id])
+
+# for rank in range(top_k):
+# doc_id = scores_sorted[rank][0]
+# # Format: Rank x: ID [Title] Body
+# logging.info("Rank %d: %s [%s] - %s\n" % (rank+1, doc_id, corpus[doc_id].get("title"), corpus[doc_id].get("text")))
diff --git a/PT-Retrieval/beir_eval/models.py b/PT-Retrieval/beir_eval/models.py
new file mode 100755
index 0000000..8a02cfe
--- /dev/null
+++ b/PT-Retrieval/beir_eval/models.py
@@ -0,0 +1,100 @@
+# from transformers import DPRContextEncoder, DPRContextEncoderTokenizerFast
+# from transformers import DPRQuestionEncoder, DPRQuestionEncoderTokenizerFast
+from typing import Union, List, Dict, Tuple
+from tqdm.autonotebook import trange
+import torch
+
+import sys
+from pathlib import Path
+BASE_DIR = Path.resolve(Path(__file__)).parent.parent
+sys.path.append(str(BASE_DIR))
+print(BASE_DIR)
+
+
+from dpr.models import init_encoder_components
+from dpr.options import add_encoder_params, setup_args_gpu, print_args, set_encoder_params_from_state
+from dpr.utils.model_utils import setup_for_distributed_mode, get_model_obj, load_states_from_checkpoint,move_to_device
+
+
+class DPRForBeir():
+ def __init__(self, args, **kwargs):
+ if not args.adapter:
+ saved_state = load_states_from_checkpoint(args.model_file)
+ set_encoder_params_from_state(saved_state.encoder_params, args)
+
+ args.sequence_length = 512
+ tensorizer, encoder, _ = init_encoder_components("dpr", args, inference_only=True)
+ encoder, _ = setup_for_distributed_mode(encoder, None, args.device, args.n_gpu,
+ args.local_rank,
+ args.fp16,
+ args.fp16_opt_level)
+
+ self.device = args.device
+
+ self.tensorizer = tensorizer
+
+ model_to_load = get_model_obj(encoder).question_model
+ model_to_load.eval()
+ if args.adapter:
+ adapter_name = model_to_load.load_adapter(args.model_file+".q")
+ model_to_load.set_active_adapters(adapter_name)
+ else:
+ encoder_name = "question_model."
+ prefix_len = len(encoder_name)
+ if args.prefix or args.prompt:
+ encoder_name += "prefix_encoder."
+ q_state = {key[prefix_len:]: value for (key, value) in saved_state.model_dict.items() if
+ key.startswith(encoder_name)}
+ model_to_load.load_state_dict(q_state, strict=not args.prefix and not args.prompt)
+ self.q_model = model_to_load
+
+
+ model_to_load = get_model_obj(encoder).ctx_model
+ model_to_load.eval()
+ if args.adapter:
+ adapter_name = model_to_load.load_adapter(args.model_file+".ctx")
+ model_to_load.set_active_adapters(adapter_name)
+ else:
+ encoder_name = "ctx_model."
+ prefix_len = len(encoder_name)
+ if args.prefix or args.prompt:
+ encoder_name += "prefix_encoder."
+ ctx_state = {key[prefix_len:]: value for (key, value) in saved_state.model_dict.items() if
+ key.startswith(encoder_name)}
+ model_to_load.load_state_dict(ctx_state, strict=not args.prefix and not args.prompt)
+ self.ctx_model = model_to_load
+
+ def encode_queries(self, queries: List[str], batch_size: int = 16, **kwargs) -> torch.Tensor:
+ query_embeddings = []
+ with torch.no_grad():
+ for start_idx in trange(0, len(queries), batch_size):
+ batch_token_tensors = [self.tensorizer.text_to_tensor(q) for q in
+ queries[start_idx:start_idx + batch_size]]
+ q_ids_batch = move_to_device(torch.stack(batch_token_tensors, dim=0), self.device)
+ q_seg_batch = move_to_device(torch.zeros_like(q_ids_batch), self.device)
+ q_attn_mask = move_to_device(self.tensorizer.get_attn_mask(q_ids_batch), self.device)
+ _, out, _ = self.q_model(q_ids_batch, q_seg_batch, q_attn_mask)
+
+ query_embeddings.extend(out.cpu())
+
+ result = torch.stack(query_embeddings)
+ return result
+
+ def encode_corpus(self, corpus: List[Dict[str, str]], batch_size: int = 8, **kwargs) -> torch.Tensor:
+
+ corpus_embeddings = []
+ with torch.no_grad():
+ for start_idx in trange(0, len(corpus), batch_size):
+ batch_token_tensors = [self.tensorizer.text_to_tensor(ctx["text"], title=ctx["title"]) for ctx in
+ corpus[start_idx:start_idx + batch_size]]
+
+ ctx_ids_batch = move_to_device(torch.stack(batch_token_tensors, dim=0), self.device)
+ ctx_seg_batch = move_to_device(torch.zeros_like(ctx_ids_batch), self.device)
+ ctx_attn_mask = move_to_device(self.tensorizer.get_attn_mask(ctx_ids_batch), self.device)
+ _, out, _ = self.ctx_model(ctx_ids_batch, ctx_seg_batch, ctx_attn_mask)
+ out = out.cpu()
+ corpus_embeddings.extend([out[i].view(-1) for i in range(out.size(0))])
+
+
+ result = torch.stack(corpus_embeddings)
+ return result
\ No newline at end of file
diff --git a/PT-Retrieval/calibration/calibration_beir.py b/PT-Retrieval/calibration/calibration_beir.py
new file mode 100644
index 0000000..5e1d458
--- /dev/null
+++ b/PT-Retrieval/calibration/calibration_beir.py
@@ -0,0 +1,85 @@
+import os
+import sys
+import logging
+import argparse
+from pathlib import Path
+
+from beir import util, LoggingHandler
+from beir.datasets.data_loader import GenericDataLoader
+from beir.retrieval.evaluation import EvaluateRetrieval
+from beir.retrieval.search.dense import DenseRetrievalExactSearch as DRES
+
+BASE_DIR = Path.resolve(Path(__file__)).parent.parent
+sys.path.append(str(BASE_DIR))
+
+from beir_eval.models import DPRForBeir
+from calibration.utils import calculate_ece, calculate_erce, plot_calibration_curve
+from dpr.options import add_encoder_params, setup_args_gpu, set_seed, add_tokenizer_params, add_cuda_params, add_tuning_params
+
+#### Just some code to print debug information to stdout
+logging.basicConfig(format='%(asctime)s - %(message)s',
+ datefmt='%Y-%m-%d %H:%M:%S',
+ level=logging.INFO,
+ handlers=[LoggingHandler()])
+
+def main(args):
+ model = DRES(DPRForBeir(args), batch_size=256)
+
+ dataset = args.dataset
+
+ data_path = "beir_eval/datasets/%s" % dataset
+
+ logging.info("evaluating dataset %s..." % dataset)
+
+ if not os.path.exists(data_path):
+ #### Download NFCorpus dataset and unzip the dataset
+ url = "https://public.ukp.informatik.tu-darmstadt.de/thakur/BEIR/datasets/{}.zip".format(dataset)
+ out_dir = "beir_eval/datasets"
+ util.download_and_unzip(url, out_dir)
+ data_path = os.path.join(out_dir, dataset)
+
+ corpus, queries, qrels = GenericDataLoader(data_folder=data_path).load(split=args.split)
+
+ retriever = EvaluateRetrieval(model, score_function="dot")
+
+ #### Retrieve dense results (format of results is identical to qrels)
+ results = retriever.retrieve(corpus, queries)
+
+ ece = calculate_ece(qrels, results, args)
+ print("ECE = %.3f" % ece)
+
+ ece = calculate_erce(qrels, results, args)
+ print("ERCE = %.3f" % ece)
+
+ # nq2, webq, trivia, curatedtrec
+ plot_calibration_curve(qrels, results, args)
+
+
+if __name__ == '__main__':
+
+#### /print debug information to stdout
+
+ parser = argparse.ArgumentParser()
+
+ add_encoder_params(parser)
+ add_tokenizer_params(parser)
+ add_cuda_params(parser)
+ add_tuning_params(parser)
+
+ parser.add_argument('--batch_size', type=int, default=32, help="Batch size for the passage encoder forward pass")
+ parser.add_argument('--dataset', type=str)
+ parser.add_argument('--split', type=str, default="text")
+ parser.add_argument('--n_non_rel', type=int, default=5)
+ parser.add_argument('--n_ece_docs', type=int, default=5)
+ parser.add_argument('--n_erce_docs', type=int, default=20)
+
+
+ args = parser.parse_args()
+ args.dpr_mode = False
+ args.fix_ctx_encoder = False
+ args.use_projection = False
+
+ setup_args_gpu(args)
+
+ main(args)
+
diff --git a/PT-Retrieval/calibration/calibration_ece_openqa.py b/PT-Retrieval/calibration/calibration_ece_openqa.py
new file mode 100755
index 0000000..7879f60
--- /dev/null
+++ b/PT-Retrieval/calibration/calibration_ece_openqa.py
@@ -0,0 +1,202 @@
+#!/usr/bin/env python3
+# Copyright (c) Facebook, Inc. and its affiliates.
+# All rights reserved.
+#
+# This source code is licensed under the license found in the
+# LICENSE file in the root directory of this source tree.
+
+"""
+ Command line tool to get dense results and validate them
+"""
+import argparse
+import os
+import csv
+import glob
+import logging
+import time
+from scipy.special import softmax
+
+from utils import calibration_curve_with_ece
+
+from dpr.models import init_encoder_components
+from dpr.options import add_encoder_params, setup_args_gpu, print_args, set_encoder_params_from_state, \
+ add_tokenizer_params, add_cuda_params, add_tuning_params
+from dpr.utils.model_utils import setup_for_distributed_mode, get_model_obj, load_states_from_checkpoint
+from dpr.indexer.faiss_indexers import DenseIndexer, DenseHNSWFlatIndexer, DenseFlatIndexer
+
+from dense_retriever import DenseRetriever, parse_qa_csv_file, validate, load_passages, save_results, iterate_encoded_files
+
+csv.field_size_limit(10000000)
+
+logger = logging.getLogger()
+logger.setLevel(logging.INFO)
+if (logger.hasHandlers()):
+ logger.handlers.clear()
+console = logging.StreamHandler()
+logger.addHandler(console)
+
+
+def main(args):
+ args.qa_file = f"data/retriever/qas/{args.dataset}-test.csv"
+ if args.dataset == "curatedtrec":
+ args.match = "regex"
+
+ total_time = time.time()
+ if not args.adapter:
+ saved_state = load_states_from_checkpoint(args.model_file)
+ set_encoder_params_from_state(saved_state.encoder_params, args)
+
+ tensorizer, encoder, _ = init_encoder_components("dpr", args, inference_only=True)
+
+ encoder = encoder.question_model
+
+ encoder, _ = setup_for_distributed_mode(encoder, None, args.device, args.n_gpu,
+ args.local_rank,
+ args.fp16)
+ encoder.eval()
+
+ # load weights from the model file
+ model_to_load = get_model_obj(encoder)
+ logger.info('Loading saved model state ...')
+ if args.adapter:
+ adapter_name = model_to_load.load_adapter(args.model_file+".q")
+ model_to_load.set_active_adapters(adapter_name)
+ else:
+ encoder_name = "question_model."
+ prefix_len = len(encoder_name)
+ if args.prefix or args.prompt:
+ encoder_name += "prefix_encoder."
+ question_encoder_state = {key[prefix_len:]: value for (key, value) in saved_state.model_dict.items() if
+ key.startswith(encoder_name)}
+ model_to_load.load_state_dict(question_encoder_state, strict=not args.prefix and not args.prompt)
+ vector_size = model_to_load.get_out_size()
+ logger.info('Encoder vector_size=%d', vector_size)
+
+ if args.hnsw_index:
+ index = DenseHNSWFlatIndexer(vector_size, args.index_buffer)
+ else:
+ index = DenseFlatIndexer(vector_size, args.index_buffer)
+
+ retriever = DenseRetriever(encoder, args.batch_size, tensorizer, index)
+
+
+ # index all passages
+ ctx_files_pattern = args.encoded_ctx_file
+ input_paths = glob.glob(ctx_files_pattern)
+
+ index_path = "_".join(input_paths[0].split("_")[:-1])
+ if args.save_or_load_index and (os.path.exists(index_path) or os.path.exists(index_path + ".index.dpr")):
+ retriever.index.deserialize_from(index_path)
+ else:
+ logger.info('Reading all passages data from files: %s', input_paths)
+ retriever.index.index_data(input_paths)
+ if args.save_or_load_index:
+ retriever.index.serialize(index_path)
+ # get questions & answers
+ questions = []
+ question_answers = []
+
+ for ds_item in parse_qa_csv_file(args.qa_file):
+ question, answers = ds_item
+ questions.append(question)
+ question_answers.append(answers)
+
+ sample_time = time.time()
+ questions_tensor = retriever.generate_question_vectors(questions)
+
+ # get top k results
+ top_ids_and_scores = retriever.get_top_docs(questions_tensor.numpy(), args.n_docs)
+
+ logger.info('sample time: %f sec.', time.time() - sample_time)
+ all_passages = load_passages(args.ctx_file)
+
+ logger.info('total time: %f sec.', time.time() - total_time)
+ if len(all_passages) == 0:
+ raise RuntimeError('No passages data found. Please specify ctx_file param properly.')
+
+ check_index = 1 if args.oagqa else 0
+ questions_doc_hits, _ = validate(all_passages, question_answers, top_ids_and_scores, args.validation_workers,
+ args.match, check_index)
+
+ ece = calculate_ece(top_ids_and_scores, questions_doc_hits)
+ print("ECE = %.3f" % ece)
+
+
+def calculate_ece(top_ids_and_scores, questions_doc_hits):
+ y_true = []
+ y_prob = []
+
+ for ids_and_scores, doc_hits in zip(top_ids_and_scores, questions_doc_hits):
+ _, scores = ids_and_scores
+ scores = scores[:args.n_docs]
+ doc_hits = doc_hits[:args.n_docs]
+ scores = softmax(scores)
+ for pred_score, hit in zip(scores, doc_hits):
+ y_true.append(int(hit))
+ y_prob.append(pred_score)
+
+ _, _, ece = calibration_curve_with_ece(y_true, y_prob, n_bins=10)
+ return ece
+
+# def calculate_erce(top_ids_and_scores, questions_doc_hits):
+# y_true = []
+# y_prob = []
+
+# for ids_and_scores, doc_hits in zip(top_ids_and_scores, questions_doc_hits):
+# _, scores = ids_and_scores
+# pos_scores = []
+# neg_scores = []
+
+# for pred_score, hit in zip(scores[:args.n_docs], doc_hits[:args.n_docs]):
+# if hit:
+# pos_scores.append(pred_score)
+# else:
+# neg_scores.append(pred_score)
+# if len(pos_scores) == 0:
+# for pred_score, hit in zip(scores, doc_hits):
+# if hit:
+# pos_scores.append(pred_score)
+# break
+
+# for pscore in pos_scores:
+# for nscore in neg_scores:
+# pos_score, neg_score = softmax([pscore, nscore])
+# diff = pos_score - neg_score
+# y_prob.append(abs(diff))
+# y_true.append(int(diff > 0))
+
+# _, _, erce = calibration_curve(y_true, y_prob, n_bins=10)
+# return erce
+
+if __name__ == '__main__':
+ parser = argparse.ArgumentParser()
+
+ add_encoder_params(parser)
+ add_tokenizer_params(parser)
+ add_cuda_params(parser)
+ add_tuning_params(parser)
+
+ parser.add_argument('--dataset', type=str, choices=["nq", "trivia", "webq", "curatedtrec"])
+ parser.add_argument('--ctx_file', required=True, type=str, default=None,
+ help="All passages file in the tsv format: id \\t passage_text \\t title")
+ parser.add_argument('--encoded_ctx_file', type=str, default=None,
+ help='Glob path to encoded passages (from generate_dense_embeddings tool)')
+ parser.add_argument('--match', type=str, default='string', choices=['regex', 'string'],
+ help="Answer matching logic type")
+ parser.add_argument('--n-docs', type=int, default=200, help="Amount of top docs to return")
+ parser.add_argument('--validation_workers', type=int, default=16,
+ help="Number of parallel processes to validate results")
+ parser.add_argument('--batch_size', type=int, default=32, help="Batch size for question encoder forward pass")
+ parser.add_argument('--index_buffer', type=int, default=50000,
+ help="Temporal memory data buffer size (in samples) for indexer")
+ parser.add_argument("--hnsw_index", action='store_true', help='If enabled, use inference time efficient HNSW index')
+ parser.add_argument("--save_or_load_index", action='store_true', help='If enabled, save index')
+ parser.add_argument("--oagqa", action='store_true')
+
+ args = parser.parse_args()
+
+ assert args.model_file, 'Please specify --model_file checkpoint to init model weights'
+
+ setup_args_gpu(args)
+ print_args(args)
+ main(args)
diff --git a/PT-Retrieval/calibration/calibration_plot_openqa.py b/PT-Retrieval/calibration/calibration_plot_openqa.py
new file mode 100644
index 0000000..1960d9b
--- /dev/null
+++ b/PT-Retrieval/calibration/calibration_plot_openqa.py
@@ -0,0 +1,70 @@
+import os
+import sys
+import logging
+import argparse
+from pathlib import Path
+
+from beir import LoggingHandler
+from beir.datasets.data_loader import GenericDataLoader
+from beir.retrieval.evaluation import EvaluateRetrieval
+from beir.retrieval.search.dense import DenseRetrievalExactSearch as DRES
+
+BASE_DIR = Path.resolve(Path(__file__)).parent.parent
+sys.path.append(str(BASE_DIR))
+
+from beir_eval.models import DPRForBeir
+from calibration.utils import plot_calibration_curve
+from calibration.calibration_beir import plot_calibration_curve
+from dpr.options import add_encoder_params, setup_args_gpu, set_seed, add_tokenizer_params, add_cuda_params, add_tuning_params
+from convert_openqa_data import convert
+
+#### Just some code to print debug information to stdout
+logging.basicConfig(format='%(asctime)s - %(message)s',
+ datefmt='%Y-%m-%d %H:%M:%S',
+ level=logging.INFO,
+ handlers=[LoggingHandler()])
+
+def main(args):
+ model = DRES(DPRForBeir(args), batch_size=256)
+
+ dataset = args.dataset
+
+ data_path = "beir_eval/datasets/openqa-%s" % dataset
+
+ logging.info("evaluating dataset %s..." % dataset)
+
+ if not os.path.exists(data_path):
+ logging.info("Convert OpenQA data to corpus, queries and qrels")
+ convert(dataset)
+
+ corpus, queries, qrels = GenericDataLoader(data_folder=data_path).load(split="test")
+
+ retriever = EvaluateRetrieval(model, score_function="dot")
+
+ results = retriever.retrieve(corpus, queries)
+
+ plot_calibration_curve(qrels, results, args)
+
+
+if __name__ == '__main__':
+ parser = argparse.ArgumentParser()
+
+ add_encoder_params(parser)
+ add_tokenizer_params(parser)
+ add_cuda_params(parser)
+ add_tuning_params(parser)
+
+ parser.add_argument('--batch_size', type=int, default=32, help="Batch size for the passage encoder forward pass")
+ parser.add_argument('--dataset', type=str, choices=["nq", "trivia", "webq", "curatedtrec"])
+ parser.add_argument('--n_non_rel', type=int, default=5)
+
+ args = parser.parse_args()
+ args.dpr_mode = False
+ args.fix_ctx_encoder = False
+ args.use_projection = False
+
+ setup_args_gpu(args)
+ # set_seed(args)
+
+ main(args)
+
diff --git a/PT-Retrieval/calibration/utils.py b/PT-Retrieval/calibration/utils.py
new file mode 100644
index 0000000..7ce5346
--- /dev/null
+++ b/PT-Retrieval/calibration/utils.py
@@ -0,0 +1,159 @@
+
+import sys
+from pathlib import Path
+BASE_DIR = Path.resolve(Path(__file__)).parent.parent
+sys.path.append(str(BASE_DIR))
+print(BASE_DIR)
+
+import logging
+import numpy as np
+from scipy.special import softmax
+
+from beir import LoggingHandler
+
+from sklearn.utils import column_or_1d
+from sklearn.utils.validation import check_consistent_length
+from sklearn.metrics._base import _check_pos_label_consistency
+from sklearn.calibration import CalibrationDisplay, calibration_curve
+
+import matplotlib.pyplot as plt
+
+logging.basicConfig(format='%(asctime)s - %(message)s',
+ datefmt='%Y-%m-%d %H:%M:%S',
+ level=logging.INFO,
+ handlers=[LoggingHandler()])
+
+def calibration_curve_with_ece(
+ y_true,
+ y_prob,
+ *,
+ pos_label=None,
+ n_bins=5,
+ strategy="uniform",
+):
+ y_true = column_or_1d(y_true)
+ y_prob = column_or_1d(y_prob)
+ check_consistent_length(y_true, y_prob)
+ pos_label = _check_pos_label_consistency(pos_label, y_true)
+
+ if y_prob.min() < 0 or y_prob.max() > 1:
+ raise ValueError("y_prob has values outside [0, 1].")
+
+ labels = np.unique(y_true)
+ if len(labels) > 2:
+ raise ValueError(
+ f"Only binary classification is supported. Provided labels {labels}."
+ )
+ y_true = y_true == pos_label
+
+ if strategy == "quantile": # Determine bin edges by distribution of data
+ quantiles = np.linspace(0, 1, n_bins + 1)
+ bins = np.percentile(y_prob, quantiles * 100)
+ elif strategy == "uniform":
+ bins = np.linspace(0.0, 1.0, n_bins + 1)
+ else:
+ raise ValueError(
+ "Invalid entry to 'strategy' input. Strategy "
+ "must be either 'quantile' or 'uniform'."
+ )
+
+ binids = np.searchsorted(bins[1:-1], y_prob)
+
+ bin_sums = np.bincount(binids, weights=y_prob, minlength=len(bins))
+ bin_true = np.bincount(binids, weights=y_true, minlength=len(bins))
+ bin_total = np.bincount(binids, minlength=len(bins))
+
+ nonzero = bin_total != 0
+ prob_true = bin_true[nonzero] / bin_total[nonzero]
+ prob_pred = bin_sums[nonzero] / bin_total[nonzero]
+ ece = np.sum(np.abs(prob_true - prob_pred) * (bin_total[nonzero] / len(y_true)))
+
+ return prob_true, prob_pred, ece
+
+def calculate_ece(qrels, results, args):
+ questions = qrels.keys()
+ y_true = []
+ y_prob = []
+ for qid in questions:
+ rels = [ k for k, v in qrels[qid].items() if v ]
+ sorted_result = sorted(results[qid].items(), key=lambda x: x[1], reverse=True)
+ prob = []
+ for pid, score in sorted_result[:args.n_ece_docs]:
+ prob.append(score)
+ y_true.append(1 if pid in rels else 0)
+ y_prob.extend(softmax(prob))
+
+ _, _, ece = calibration_curve_with_ece(y_true, y_prob, n_bins=10)
+ return ece
+
+def calculate_erce(qrels, results, args):
+ questions = qrels.keys()
+ y_true = []
+ y_prob = []
+ for qid in questions:
+ rels = set([k for k, v in qrels[qid].items() if v])
+ sorted_result = sorted(results[qid].items(), key=lambda x: x[1], reverse=True)
+ pos_scores = []
+ neg_scores = []
+ # VERSION 1
+ for pid, score in sorted_result[:args.n_erce_docs]:
+ if pid in rels:
+ pos_scores.append(score)
+ else:
+ neg_scores.append(score)
+ if len(pos_scores) == 0:
+ for pid, score in sorted_result:
+ if pid in rels:
+ pos_scores.append(score)
+ break
+ # VERSION 2
+ # for pid, score in sorted_result:
+ # if pid in rels:
+ # pos_scores.append(score)
+ # break
+ # if len(pos_scores) == 0: pos_scores.append(0.0)
+ # for pid, score in sorted_result[:args.n_erce_docs]:
+ # if pid not in rels:
+ # neg_scores.append(score)
+
+ for pscore in pos_scores:
+ for nscore in neg_scores:
+ pos_score, neg_score = softmax([pscore, nscore])
+ diff = pos_score - neg_score
+ y_prob.append(abs(diff))
+ y_true.append(int(diff > 0))
+
+ _, _, erce = calibration_curve_with_ece(y_true, y_prob, n_bins=10)
+ return erce
+
+def plot_calibration_curve(qrels, results, args):
+ questions = qrels.keys()
+ y_true = []
+ y_prob = []
+
+ for qid in questions:
+ rels = set([k for k, v in qrels[qid].items() if v])
+ result = set(results[qid].keys())
+ pos_docs = list(result.intersection(rels))
+ if len(pos_docs) == 0:
+ continue
+ neg_docs = list(result - rels)
+
+ pos_pid = np.random.choice(pos_docs, 1)[0]
+ neg_pid = np.random.choice(neg_docs, args.n_non_rel, replace=False)
+ pos_score = results[qid][pos_pid]
+ neg_scores = [ results[qid][i] for i in neg_pid ]
+ scores = softmax([pos_score] + neg_scores)
+
+ y_true.extend([1] + [0] * args.n_non_rel)
+ y_prob.extend(scores)
+
+
+ prob_true, prob_pred = calibration_curve(y_true, y_prob, n_bins=10)
+
+ disp = CalibrationDisplay(prob_true, prob_pred, y_prob)
+ disp.plot()
+
+ method = "pt" if args.prefix else "ft"
+ filename = f"{args.dataset}-{args.n_non_rel}-{method}"
+ plt.savefig(f'results/{filename}.png')
diff --git a/PT-Retrieval/colbert/.gitignore b/PT-Retrieval/colbert/.gitignore
new file mode 100644
index 0000000..2286c76
--- /dev/null
+++ b/PT-Retrieval/colbert/.gitignore
@@ -0,0 +1,16 @@
+experiments/
+checkpoints/
+data/
+logs/
+output/
+
+# Byte-compiled / optimized / DLL files
+__pycache__/
+*.py[cod]
+*$py.class
+
+# Jupyter Notebook
+.ipynb_checkpoints
+
+# mac
+.DS_Store
diff --git a/PT-Retrieval/colbert/LICENSE b/PT-Retrieval/colbert/LICENSE
new file mode 100644
index 0000000..9072f0b
--- /dev/null
+++ b/PT-Retrieval/colbert/LICENSE
@@ -0,0 +1,21 @@
+MIT License
+
+Copyright (c) 2019, 2020 Stanford Future Data Systems
+
+Permission is hereby granted, free of charge, to any person obtaining a copy
+of this software and associated documentation files (the "Software"), to deal
+in the Software without restriction, including without limitation the rights
+to use, copy, modify, merge, publish, distribute, sublicense, and/or sell
+copies of the Software, and to permit persons to whom the Software is
+furnished to do so, subject to the following conditions:
+
+The above copyright notice and this permission notice shall be included in all
+copies or substantial portions of the Software.
+
+THE SOFTWARE IS PROVIDED "AS IS", WITHOUT WARRANTY OF ANY KIND, EXPRESS OR
+IMPLIED, INCLUDING BUT NOT LIMITED TO THE WARRANTIES OF MERCHANTABILITY,
+FITNESS FOR A PARTICULAR PURPOSE AND NONINFRINGEMENT. IN NO EVENT SHALL THE
+AUTHORS OR COPYRIGHT HOLDERS BE LIABLE FOR ANY CLAIM, DAMAGES OR OTHER
+LIABILITY, WHETHER IN AN ACTION OF CONTRACT, TORT OR OTHERWISE, ARISING FROM,
+OUT OF OR IN CONNECTION WITH THE SOFTWARE OR THE USE OR OTHER DEALINGS IN THE
+SOFTWARE.
diff --git a/PT-Retrieval/colbert/colbert/__init__.py b/PT-Retrieval/colbert/colbert/__init__.py
new file mode 100644
index 0000000..e69de29
diff --git a/PT-Retrieval/colbert/colbert/beir_eval.py b/PT-Retrieval/colbert/colbert/beir_eval.py
new file mode 100644
index 0000000..8a130db
--- /dev/null
+++ b/PT-Retrieval/colbert/colbert/beir_eval.py
@@ -0,0 +1,76 @@
+from beir import util, LoggingHandler
+from beir.datasets.data_loader import GenericDataLoader
+from beir.retrieval.evaluation import EvaluateRetrieval
+
+import pathlib, os, csv, random
+import sys
+import argparse
+import logging
+
+#### Just some code to print debug information to stdout
+logging.basicConfig(format='%(asctime)s - %(message)s',
+ datefmt='%Y-%m-%d %H:%M:%S',
+ level=logging.INFO,
+ handlers=[LoggingHandler()])
+
+csv.field_size_limit(sys.maxsize)
+
+def tsv_reader(input_filepath):
+ reader = csv.reader(open(input_filepath, encoding="utf-8"), delimiter="\t", quoting=csv.QUOTE_MINIMAL)
+ for idx, row in enumerate(reader):
+ yield idx, row
+
+def main(dataset, split, data_dir, collection, rankings, k_values):
+ #### Provide the data_dir where nfcorpus has been downloaded and unzipped
+ if data_dir == None:
+ url = "https://public.ukp.informatik.tu-darmstadt.de/thakur/BEIR/datasets/{}.zip".format(dataset)
+ out_dir = os.path.join(pathlib.Path(__file__).parent.absolute(), "datasets")
+ data_dir = util.download_and_unzip(url, out_dir)
+
+ #### Provide the data_dir where nfcorpus has been downloaded and unzipped
+ corpus, queries, qrels = GenericDataLoader(data_folder=data_dir).load(split=split)
+
+ inv_map, results = {}, {}
+
+ #### Document mappings (from original string to position in tsv file ####
+ for idx, row in tsv_reader(collection):
+ inv_map[str(idx)] = row[0]
+
+ #### Results ####
+ for _, row in tsv_reader(rankings):
+ qid, doc_id, rank = row[0], row[1], int(row[2])
+ if qid != inv_map[str(doc_id)]:
+ if qid not in results:
+ results[qid] = {inv_map[str(doc_id)]: 1 / (rank + 1)}
+ else:
+ results[qid][inv_map[str(doc_id)]] = 1 / (rank + 1)
+
+ #### Evaluate your retrieval using NDCG@k, MAP@K ...
+ evaluator = EvaluateRetrieval()
+ ndcg, _map, recall, precision = evaluator.evaluate(qrels, results, k_values)
+ mrr = EvaluateRetrieval.evaluate_custom(qrels, results, k_values, metric='mrr')
+
+ #### Print top-k documents retrieved ####
+ top_k = 10
+
+ query_id, ranking_scores = random.choice(list(results.items()))
+ scores_sorted = sorted(ranking_scores.items(), key=lambda item: item[1], reverse=True)
+ logging.info("Query : %s\n" % queries[query_id])
+
+ # for rank in range(top_k):
+ # doc_id = scores_sorted[rank][0]
+ # # Format: Rank x: ID [Title] Body
+ # logging.info("Rank %d: %s [%s] - %s\n" % (rank+1, doc_id, corpus[doc_id].get("title"), corpus[doc_id].get("text")))
+
+
+if __name__ == '__main__':
+ parser = argparse.ArgumentParser()
+ parser.add_argument('--dataset', type=str, help="BEIR Dataset Name, eg. nfcorpus")
+ parser.add_argument('--split', type=str, default="test")
+ parser.add_argument('--data_dir', type=str, default=None, help='Path to a BEIR repository (incase already downloaded or custom)')
+ parser.add_argument('--collection', type=str, help='Path to the ColBERT collection file')
+ parser.add_argument('--rankings', required=True, type=str, help='Path to the ColBERT generated rankings file')
+ parser.add_argument('--k_values', nargs='+', type=int, default=[1,3,5,10,100])
+ args = parser.parse_args()
+ main(**vars(args))
+
diff --git a/PT-Retrieval/colbert/colbert/data_prep.py b/PT-Retrieval/colbert/colbert/data_prep.py
new file mode 100644
index 0000000..9e59e3d
--- /dev/null
+++ b/PT-Retrieval/colbert/colbert/data_prep.py
@@ -0,0 +1,63 @@
+from beir import util, LoggingHandler
+from beir.datasets.data_loader import GenericDataLoader
+
+import pathlib, os, csv, random
+import sys
+import logging
+import argparse
+from tqdm import tqdm
+
+random.seed(42)
+
+#### Just some code to print debug information to stdout
+logging.basicConfig(format='%(asctime)s - %(message)s',
+ datefmt='%Y-%m-%d %H:%M:%S',
+ level=logging.INFO,
+ handlers=[LoggingHandler()])
+#### /print debug information to stdout
+
+def preprocess(text):
+ return text.replace("\r", " ").replace("\t", " ").replace("\n", " ")
+
+def main(dataset, split, data_dir, collection, queries):
+
+ if data_dir == None:
+ #### Download nfcorpus.zip dataset and unzip the dataset
+ url = "https://public.ukp.informatik.tu-darmstadt.de/thakur/BEIR/datasets/{}.zip".format(dataset)
+ out_dir = os.path.join(pathlib.Path(__file__).parent.absolute(), "datasets")
+ data_dir = util.download_and_unzip(url, out_dir)
+ logging.info("Downloaded {} BEIR dataset: {}".format(dataset, out_dir))
+
+ logging.info("Coverting {} split of {} dataset...".format(split.upper(), dataset))
+
+ #### Provide the data_dir where nfcorpus has been downloaded and unzipped
+ corpus, _queries, qrels = GenericDataLoader(data_folder=data_dir).load(split=split)
+ corpus_ids = list(corpus)
+
+ #### Create output directories for collection and queries
+ os.makedirs("/".join(collection.split("/")[:-1]), exist_ok=True)
+ os.makedirs("/".join(queries.split("/")[:-1]), exist_ok=True)
+
+ logging.info("Preprocessing Corpus and Saving to {} ...".format(collection))
+ with open(collection, 'w') as fIn:
+ writer = csv.writer(fIn, delimiter="\t", quoting=csv.QUOTE_MINIMAL)
+ for doc_id in tqdm(corpus_ids, total=len(corpus_ids)):
+ doc = corpus[doc_id]
+ writer.writerow([doc_id,(preprocess(doc.get("title", "")) + " " + preprocess(doc.get("text", ""))).strip()])
+
+ logging.info("Preprocessing Corpus and Saving to {} ...".format(queries))
+ with open(queries, 'w') as fIn:
+ writer = csv.writer(fIn, delimiter="\t", quoting=csv.QUOTE_MINIMAL)
+ for qid, query in tqdm(_queries.items(), total=len(_queries)):
+ writer.writerow([qid, query])
+
+
+if __name__ == '__main__':
+ parser = argparse.ArgumentParser()
+ parser.add_argument('--dataset', type=str, help="BEIR Dataset Name, eg. nfcorpus")
+ parser.add_argument('--split', type=str, default="test")
+ parser.add_argument('--data_dir', type=str, default=None, help='Path to a BEIR repository (incase already downloaded or custom)')
+ parser.add_argument('--collection', type=str, help='Path to store BEIR collection tsv file')
+ parser.add_argument('--queries', type=str, help='Path to store BEIR queries tsv file')
+ args = parser.parse_args()
+ main(**vars(args))
\ No newline at end of file
diff --git a/PT-Retrieval/colbert/colbert/evaluation/__init__.py b/PT-Retrieval/colbert/colbert/evaluation/__init__.py
new file mode 100644
index 0000000..e69de29
diff --git a/PT-Retrieval/colbert/colbert/evaluation/load_model.py b/PT-Retrieval/colbert/colbert/evaluation/load_model.py
new file mode 100644
index 0000000..11b9c9b
--- /dev/null
+++ b/PT-Retrieval/colbert/colbert/evaluation/load_model.py
@@ -0,0 +1,45 @@
+import os
+import ujson
+import torch
+import random
+
+from collections import defaultdict, OrderedDict
+
+from transformers import BertConfig
+
+from colbert.parameters import DEVICE
+from colbert.modeling.colbert import ColBERT
+from colbert.modeling.prefix import PrefixColBERT
+from colbert.utils.utils import print_message, load_checkpoint
+
+
+def load_model(args, do_print=True):
+ if args.prefix:
+ config = BertConfig.from_pretrained('bert-base-uncased', cache_dir=".cache")
+ config.pre_seq_len = args.pre_seq_len
+ config.prefix_hidden_size = args.prefix_hidden_size
+ config.prefix_mlp = args.prefix_mlp
+ colbert = PrefixColBERT.from_pretrained('bert-base-uncased', config=config,
+ query_maxlen=args.query_maxlen,
+ doc_maxlen=args.doc_maxlen,
+ dim=args.dim,
+ similarity_metric=args.similarity,
+ mask_punctuation=args.mask_punctuation)
+ else:
+ config = BertConfig.from_pretrained('bert-base-uncased', cache_dir=".cache")
+ colbert = ColBERT.from_pretrained('bert-base-uncased',
+ config=config,
+ query_maxlen=args.query_maxlen,
+ doc_maxlen=args.doc_maxlen,
+ dim=args.dim,
+ similarity_metric=args.similarity,
+ mask_punctuation=args.mask_punctuation)
+ colbert = colbert.to(DEVICE)
+
+ print_message("#> Loading model checkpoint.", condition=do_print)
+
+ checkpoint = load_checkpoint(args.checkpoint, colbert, do_print=do_print)
+
+ colbert.eval()
+
+ return colbert, checkpoint
diff --git a/PT-Retrieval/colbert/colbert/evaluation/loaders.py b/PT-Retrieval/colbert/colbert/evaluation/loaders.py
new file mode 100644
index 0000000..ef7bfff
--- /dev/null
+++ b/PT-Retrieval/colbert/colbert/evaluation/loaders.py
@@ -0,0 +1,196 @@
+import os
+import ujson
+import torch
+import random
+
+from collections import defaultdict, OrderedDict
+
+from colbert.parameters import DEVICE
+from colbert.modeling.colbert import ColBERT
+from colbert.utils.utils import print_message, load_checkpoint
+from colbert.evaluation.load_model import load_model
+from colbert.utils.runs import Run
+
+
+def load_queries(queries_path):
+ queries = OrderedDict()
+
+ print_message("#> Loading the queries from", queries_path, "...")
+
+ with open(queries_path) as f:
+ for line in f:
+ qid, query, *_ = line.strip().split('\t')
+ # qid = int(qid)
+
+ assert (qid not in queries), ("Query QID", qid, "is repeated!")
+ queries[qid] = query
+
+ print_message("#> Got", len(queries), "queries. All QIDs are unique.\n")
+
+ return queries
+
+
+def load_qrels(qrels_path):
+ if qrels_path is None:
+ return None
+
+ print_message("#> Loading qrels from", qrels_path, "...")
+
+ qrels = OrderedDict()
+ with open(qrels_path, mode='r', encoding="utf-8") as f:
+ for line in f:
+ qid, x, pid, y = map(int, line.strip().split('\t'))
+ assert x == 0 and y == 1
+ qrels[qid] = qrels.get(qid, [])
+ qrels[qid].append(pid)
+
+ assert all(len(qrels[qid]) == len(set(qrels[qid])) for qid in qrels)
+
+ avg_positive = round(sum(len(qrels[qid]) for qid in qrels) / len(qrels), 2)
+
+ print_message("#> Loaded qrels for", len(qrels), "unique queries with",
+ avg_positive, "positives per query on average.\n")
+
+ return qrels
+
+
+def load_topK(topK_path):
+ queries = OrderedDict()
+ topK_docs = OrderedDict()
+ topK_pids = OrderedDict()
+
+ print_message("#> Loading the top-k per query from", topK_path, "...")
+
+ with open(topK_path) as f:
+ for line_idx, line in enumerate(f):
+ if line_idx and line_idx % (10*1000*1000) == 0:
+ print(line_idx, end=' ', flush=True)
+
+ qid, pid, query, passage = line.split('\t')
+ # qid, pid = int(qid), int(pid)
+
+ assert (qid not in queries) or (queries[qid] == query)
+ queries[qid] = query
+ topK_docs[qid] = topK_docs.get(qid, [])
+ topK_docs[qid].append(passage)
+ topK_pids[qid] = topK_pids.get(qid, [])
+ topK_pids[qid].append(pid)
+
+ print()
+
+ assert all(len(topK_pids[qid]) == len(set(topK_pids[qid])) for qid in topK_pids)
+
+ Ks = [len(topK_pids[qid]) for qid in topK_pids]
+
+ print_message("#> max(Ks) =", max(Ks), ", avg(Ks) =", round(sum(Ks) / len(Ks), 2))
+ print_message("#> Loaded the top-k per query for", len(queries), "unique queries.\n")
+
+ return queries, topK_docs, topK_pids
+
+
+def load_topK_pids(topK_path, qrels):
+ topK_pids = defaultdict(list)
+ topK_positives = defaultdict(list)
+
+ print_message("#> Loading the top-k PIDs per query from", topK_path, "...")
+
+ with open(topK_path) as f:
+ for line_idx, line in enumerate(f):
+ if line_idx and line_idx % (10*1000*1000) == 0:
+ print(line_idx, end=' ', flush=True)
+
+ qid, pid, *rest = line.strip().split('\t')
+ # qid, pid = int(qid), int(pid)
+
+ topK_pids[qid].append(pid)
+
+ assert len(rest) in [1, 2, 3]
+
+ if len(rest) > 1:
+ *_, label = rest
+ label = int(label)
+ assert label in [0, 1]
+
+ if label >= 1:
+ topK_positives[qid].append(pid)
+
+ print()
+
+ assert all(len(topK_pids[qid]) == len(set(topK_pids[qid])) for qid in topK_pids)
+ assert all(len(topK_positives[qid]) == len(set(topK_positives[qid])) for qid in topK_positives)
+
+ # Make them sets for fast lookups later
+ topK_positives = {qid: set(topK_positives[qid]) for qid in topK_positives}
+
+ Ks = [len(topK_pids[qid]) for qid in topK_pids]
+
+ print_message("#> max(Ks) =", max(Ks), ", avg(Ks) =", round(sum(Ks) / len(Ks), 2))
+ print_message("#> Loaded the top-k per query for", len(topK_pids), "unique queries.\n")
+
+ if len(topK_positives) == 0:
+ topK_positives = None
+ else:
+ assert len(topK_pids) >= len(topK_positives)
+
+ for qid in set.difference(set(topK_pids.keys()), set(topK_positives.keys())):
+ topK_positives[qid] = []
+
+ assert len(topK_pids) == len(topK_positives)
+
+ avg_positive = round(sum(len(topK_positives[qid]) for qid in topK_positives) / len(topK_pids), 2)
+
+ print_message("#> Concurrently got annotations for", len(topK_positives), "unique queries with",
+ avg_positive, "positives per query on average.\n")
+
+ assert qrels is None or topK_positives is None, "Cannot have both qrels and an annotated top-K file!"
+
+ if topK_positives is None:
+ topK_positives = qrels
+
+ return topK_pids, topK_positives
+
+
+def load_collection(collection_path):
+ print_message("#> Loading collection...")
+
+ collection = []
+
+ with open(collection_path) as f:
+ for line_idx, line in enumerate(f):
+ if line_idx % (1000*1000) == 0:
+ print(f'{line_idx // 1000 // 1000}M', end=' ', flush=True)
+
+ pid, passage, *rest = line.strip().split('\t')
+ assert pid == 'id' or int(pid) == line_idx
+
+ if len(rest) >= 1:
+ title = rest[0]
+ passage = title + ' | ' + passage
+
+ collection.append(passage)
+
+ print()
+
+ return collection
+
+
+def load_colbert(args, do_print=True):
+ colbert, checkpoint = load_model(args, do_print)
+
+ # TODO: If the parameters below were not specified on the command line, their *checkpoint* values should be used.
+ # I.e., not their purely (i.e., training) default values.
+
+ for k in ['query_maxlen', 'doc_maxlen', 'dim', 'similarity', 'amp']:
+ if 'arguments' in checkpoint and hasattr(args, k):
+ if k in checkpoint['arguments'] and checkpoint['arguments'][k] != getattr(args, k):
+ a, b = checkpoint['arguments'][k], getattr(args, k)
+ Run.warn(f"Got checkpoint['arguments']['{k}'] != args.{k} (i.e., {a} != {b})")
+
+ if 'arguments' in checkpoint:
+ if args.rank < 1:
+ print(ujson.dumps(checkpoint['arguments'], indent=4))
+
+ if do_print:
+ print('\n')
+
+ return colbert, checkpoint
diff --git a/PT-Retrieval/colbert/colbert/evaluation/metrics.py b/PT-Retrieval/colbert/colbert/evaluation/metrics.py
new file mode 100644
index 0000000..c9d1f54
--- /dev/null
+++ b/PT-Retrieval/colbert/colbert/evaluation/metrics.py
@@ -0,0 +1,114 @@
+import ujson
+
+from collections import defaultdict
+from colbert.utils.runs import Run
+
+
+class Metrics:
+ def __init__(self, mrr_depths: set, recall_depths: set, success_depths: set, total_queries=None):
+ self.results = {}
+ self.mrr_sums = {depth: 0.0 for depth in mrr_depths}
+ self.recall_sums = {depth: 0.0 for depth in recall_depths}
+ self.success_sums = {depth: 0.0 for depth in success_depths}
+ self.total_queries = total_queries
+
+ self.max_query_idx = -1
+ self.num_queries_added = 0
+
+ def add(self, query_idx, query_key, ranking, gold_positives):
+ self.num_queries_added += 1
+
+ assert query_key not in self.results
+ assert len(self.results) <= query_idx
+ assert len(set(gold_positives)) == len(gold_positives)
+ assert len(set([pid for _, pid, _ in ranking])) == len(ranking)
+
+ self.results[query_key] = ranking
+
+ positives = [i for i, (_, pid, _) in enumerate(ranking) if pid in gold_positives]
+
+ if len(positives) == 0:
+ return
+
+ for depth in self.mrr_sums:
+ first_positive = positives[0]
+ self.mrr_sums[depth] += (1.0 / (first_positive+1.0)) if first_positive < depth else 0.0
+
+ for depth in self.success_sums:
+ first_positive = positives[0]
+ self.success_sums[depth] += 1.0 if first_positive < depth else 0.0
+
+ for depth in self.recall_sums:
+ num_positives_up_to_depth = len([pos for pos in positives if pos < depth])
+ self.recall_sums[depth] += num_positives_up_to_depth / len(gold_positives)
+
+ def print_metrics(self, query_idx):
+ for depth in sorted(self.mrr_sums):
+ print("MRR@" + str(depth), "=", self.mrr_sums[depth] / (query_idx+1.0))
+
+ for depth in sorted(self.success_sums):
+ print("Success@" + str(depth), "=", self.success_sums[depth] / (query_idx+1.0))
+
+ for depth in sorted(self.recall_sums):
+ print("Recall@" + str(depth), "=", self.recall_sums[depth] / (query_idx+1.0))
+
+ def log(self, query_idx):
+ assert query_idx >= self.max_query_idx
+ self.max_query_idx = query_idx
+
+ Run.log_metric("ranking/max_query_idx", query_idx, query_idx)
+ Run.log_metric("ranking/num_queries_added", self.num_queries_added, query_idx)
+
+ for depth in sorted(self.mrr_sums):
+ score = self.mrr_sums[depth] / (query_idx+1.0)
+ Run.log_metric("ranking/MRR." + str(depth), score, query_idx)
+
+ for depth in sorted(self.success_sums):
+ score = self.success_sums[depth] / (query_idx+1.0)
+ Run.log_metric("ranking/Success." + str(depth), score, query_idx)
+
+ for depth in sorted(self.recall_sums):
+ score = self.recall_sums[depth] / (query_idx+1.0)
+ Run.log_metric("ranking/Recall." + str(depth), score, query_idx)
+
+ def output_final_metrics(self, path, query_idx, num_queries):
+ assert query_idx + 1 == num_queries
+ assert num_queries == self.total_queries
+
+ if self.max_query_idx < query_idx:
+ self.log(query_idx)
+
+ self.print_metrics(query_idx)
+
+ output = defaultdict(dict)
+
+ for depth in sorted(self.mrr_sums):
+ score = self.mrr_sums[depth] / (query_idx+1.0)
+ output['mrr'][depth] = score
+
+ for depth in sorted(self.success_sums):
+ score = self.success_sums[depth] / (query_idx+1.0)
+ output['success'][depth] = score
+
+ for depth in sorted(self.recall_sums):
+ score = self.recall_sums[depth] / (query_idx+1.0)
+ output['recall'][depth] = score
+
+ with open(path, 'w') as f:
+ ujson.dump(output, f, indent=4)
+ f.write('\n')
+
+
+def evaluate_recall(qrels, queries, topK_pids):
+ if qrels is None:
+ return
+
+ assert set(qrels.keys()) == set(queries.keys())
+ recall_at_k = [len(set.intersection(set(qrels[qid]), set(topK_pids[qid]))) / max(1.0, len(qrels[qid]))
+ for qid in qrels]
+ recall_at_k = sum(recall_at_k) / len(qrels)
+ recall_at_k = round(recall_at_k, 3)
+ print("Recall @ maximum depth =", recall_at_k)
+
+
+# TODO: If implicit qrels are used (for re-ranking), warn if a recall metric is requested + add an asterisk to output.
diff --git a/PT-Retrieval/colbert/colbert/evaluation/ranking.py b/PT-Retrieval/colbert/colbert/evaluation/ranking.py
new file mode 100644
index 0000000..2d844ed
--- /dev/null
+++ b/PT-Retrieval/colbert/colbert/evaluation/ranking.py
@@ -0,0 +1,88 @@
+import os
+import random
+import time
+import torch
+import torch.nn as nn
+
+from itertools import accumulate
+from math import ceil
+
+from colbert.utils.runs import Run
+from colbert.utils.utils import print_message
+
+from colbert.evaluation.metrics import Metrics
+from colbert.evaluation.ranking_logger import RankingLogger
+from colbert.modeling.inference import ModelInference
+
+from colbert.evaluation.slow import slow_rerank
+
+
+def evaluate(args):
+ args.inference = ModelInference(args.colbert, amp=args.amp)
+ qrels, queries, topK_pids = args.qrels, args.queries, args.topK_pids
+
+ depth = args.depth
+ collection = args.collection
+ if collection is None:
+ topK_docs = args.topK_docs
+
+ def qid2passages(qid):
+ if collection is not None:
+ return [collection[pid] for pid in topK_pids[qid][:depth]]
+ else:
+ return topK_docs[qid][:depth]
+
+ metrics = Metrics(mrr_depths={10, 100}, recall_depths={50, 200, 1000},
+ success_depths={5, 10, 20, 50, 100, 1000},
+ total_queries=len(queries))
+
+ ranking_logger = RankingLogger(Run.path, qrels=qrels)
+
+ args.milliseconds = []
+
+ with ranking_logger.context('ranking.tsv', also_save_annotations=(qrels is not None)) as rlogger:
+ with torch.no_grad():
+ keys = sorted(list(queries.keys()))
+ random.shuffle(keys)
+
+ for query_idx, qid in enumerate(keys):
+ query = queries[qid]
+
+ print_message(query_idx, qid, query, '\n')
+
+ if qrels and args.shortcircuit and len(set.intersection(set(qrels[qid]), set(topK_pids[qid]))) == 0:
+ continue
+
+ ranking = slow_rerank(args, query, topK_pids[qid], qid2passages(qid))
+
+ rlogger.log(qid, ranking, [0, 1])
+
+ if qrels:
+ metrics.add(query_idx, qid, ranking, qrels[qid])
+
+ for i, (score, pid, passage) in enumerate(ranking):
+ if pid in qrels[qid]:
+ print("\n#> Found", pid, "at position", i+1, "with score", score)
+ print(passage)
+ break
+
+ metrics.print_metrics(query_idx)
+ metrics.log(query_idx)
+
+ print_message("#> checkpoint['batch'] =", args.checkpoint['batch'], '\n')
+ print("rlogger.filename =", rlogger.filename)
+
+ if len(args.milliseconds) > 1:
+ print('Slow-Ranking Avg Latency =', sum(args.milliseconds[1:]) / len(args.milliseconds[1:]))
+
+ print("\n\n")
+
+ print("\n\n")
+ # print('Avg Latency =', sum(args.milliseconds[1:]) / len(args.milliseconds[1:]))
+ print("\n\n")
+
+ print('\n\n')
+ if qrels:
+ assert query_idx + 1 == len(keys) == len(set(keys))
+ metrics.output_final_metrics(os.path.join(Run.path, 'ranking.metrics'), query_idx, len(queries))
+ print('\n\n')
diff --git a/PT-Retrieval/colbert/colbert/evaluation/ranking_logger.py b/PT-Retrieval/colbert/colbert/evaluation/ranking_logger.py
new file mode 100644
index 0000000..7a11fa7
--- /dev/null
+++ b/PT-Retrieval/colbert/colbert/evaluation/ranking_logger.py
@@ -0,0 +1,57 @@
+import os
+
+from contextlib import contextmanager
+from colbert.utils.utils import print_message, NullContextManager
+from colbert.utils.runs import Run
+
+
+class RankingLogger():
+ def __init__(self, directory, qrels=None, log_scores=False):
+ self.directory = directory
+ self.qrels = qrels
+ self.filename, self.also_save_annotations = None, None
+ self.log_scores = log_scores
+
+ @contextmanager
+ def context(self, filename, also_save_annotations=False):
+ assert self.filename is None
+ assert self.also_save_annotations is None
+
+ filename = os.path.join(self.directory, filename)
+ self.filename, self.also_save_annotations = filename, also_save_annotations
+
+ print_message("#> Logging ranked lists to {}".format(self.filename))
+
+ with open(filename, 'w') as f:
+ self.f = f
+ with (open(filename + '.annotated', 'w') if also_save_annotations else NullContextManager()) as g:
+ self.g = g
+ try:
+ yield self
+ finally:
+ pass
+
+ def log(self, qid, ranking, is_ranked=True, print_positions=[]):
+ print_positions = set(print_positions)
+
+ f_buffer = []
+ g_buffer = []
+
+ for rank, (score, pid, passage) in enumerate(ranking):
+ is_relevant = self.qrels and int(pid in self.qrels[qid])
+ rank = rank+1 if is_ranked else -1
+
+ possibly_score = [score] if self.log_scores else []
+
+ f_buffer.append('\t'.join([str(x) for x in [qid, pid, rank] + possibly_score]) + "\n")
+ if self.g:
+ g_buffer.append('\t'.join([str(x) for x in [qid, pid, rank, is_relevant]]) + "\n")
+
+ if rank in print_positions:
+ prefix = "** " if is_relevant else ""
+ prefix += str(rank)
+ print("#> ( QID {} ) ".format(qid) + prefix + ") ", pid, ":", score, ' ', passage)
+
+ self.f.write(''.join(f_buffer))
+ if self.g:
+ self.g.write(''.join(g_buffer))
diff --git a/PT-Retrieval/colbert/colbert/evaluation/slow.py b/PT-Retrieval/colbert/colbert/evaluation/slow.py
new file mode 100644
index 0000000..a0c3bb8
--- /dev/null
+++ b/PT-Retrieval/colbert/colbert/evaluation/slow.py
@@ -0,0 +1,21 @@
+import os
+
+def slow_rerank(args, query, pids, passages):
+ colbert = args.colbert
+ inference = args.inference
+
+ Q = inference.queryFromText([query])
+
+ D_ = inference.docFromText(passages, bsize=args.bsize)
+ scores = colbert.score(Q, D_).cpu()
+
+ scores = scores.sort(descending=True)
+ ranked = scores.indices.tolist()
+
+ ranked_scores = scores.values.tolist()
+ ranked_pids = [pids[position] for position in ranked]
+ ranked_passages = [passages[position] for position in ranked]
+
+ assert len(ranked_pids) == len(set(ranked_pids))
+
+ return list(zip(ranked_scores, ranked_pids, ranked_passages))
diff --git a/PT-Retrieval/colbert/colbert/filter_model.py b/PT-Retrieval/colbert/colbert/filter_model.py
new file mode 100644
index 0000000..b7d2233
--- /dev/null
+++ b/PT-Retrieval/colbert/colbert/filter_model.py
@@ -0,0 +1,91 @@
+
+
+
+import os
+import random
+import torch
+
+from colbert.utils.parser import Arguments
+from colbert.utils.runs import Run
+
+from colbert.evaluation.loaders import load_colbert, load_qrels, load_queries
+from colbert.indexing.faiss import get_faiss_index_name
+from colbert.ranking.retrieval import retrieve
+from colbert.ranking.batch_retrieval import batch_retrieve
+from colbert.utils.utils import print_message, save_checkpoint
+from colbert.utils.utils import print_message, load_checkpoint
+from transformers import BertConfig
+
+from colbert.parameters import DEVICE
+from colbert.modeling.colbert import ColBERT
+from colbert.modeling.prefix import PrefixColBERT
+from colbert.utils.utils import print_message, load_checkpoint
+from transformers import AdamW
+from collections import OrderedDict, defaultdict
+
+def main():
+ random.seed(12345)
+
+ parser = Arguments(description='End-to-end retrieval and ranking with ColBERT.')
+
+ parser.add_model_parameters()
+ parser.add_model_inference_parameters()
+ parser.add_ranking_input()
+ parser.add_retrieval_input()
+
+ parser.add_argument('--faiss_name', dest='faiss_name', default=None, type=str)
+ parser.add_argument('--faiss_depth', dest='faiss_depth', default=1024, type=int)
+ parser.add_argument('--part-range', dest='part_range', default=None, type=str)
+ parser.add_argument('--batch', dest='batch', default=False, action='store_true')
+ parser.add_argument('--depth', dest='depth', default=1000, type=int)
+ parser.add_argument('--ranking_dir', dest='ranking_dir', default=None, type=str)
+
+ args = parser.parse()
+
+ args.depth = args.depth if args.depth > 0 else None
+
+ if args.part_range:
+ part_offset, part_endpos = map(int, args.part_range.split('..'))
+ args.part_range = range(part_offset, part_endpos)
+
+ # colbert, checkpoint = load_colbert(args)
+ if args.prefix:
+ config = BertConfig.from_pretrained('bert-base-uncased', cache_dir=".cache")
+ config.pre_seq_len = args.pre_seq_len
+ config.prefix_hidden_size = args.prefix_hidden_size
+ config.prefix_mlp = args.prefix_mlp
+ colbert = PrefixColBERT.from_pretrained('bert-base-uncased', config=config,
+ query_maxlen=args.query_maxlen,
+ doc_maxlen=args.doc_maxlen,
+ dim=args.dim,
+ similarity_metric=args.similarity,
+ mask_punctuation=args.mask_punctuation)
+ else:
+ config = BertConfig.from_pretrained('bert-base-uncased', cache_dir=".cache")
+ colbert = ColBERT.from_pretrained('bert-base-uncased',
+ config=config,
+ query_maxlen=args.query_maxlen,
+ doc_maxlen=args.doc_maxlen,
+ dim=args.dim,
+ similarity_metric=args.similarity,
+ mask_punctuation=args.mask_punctuation)
+
+ colbert = colbert.to(DEVICE)
+ pretrain_state_dict = OrderedDict()
+ for k, v in colbert.state_dict().items():
+ pretrain_state_dict[k] = torch.clone(v)
+ print(filter(lambda p: p.requires_grad, colbert.parameters()))
+ optimizer = AdamW(filter(lambda p: p.requires_grad, colbert.parameters()), lr=1e-3, eps=1e-8)
+ checkpoint = load_checkpoint(args.checkpoint, colbert, optimizer=optimizer)
+
+ for k, v in pretrain_state_dict.items():
+ print(k)
+ print(bool(torch.all(v == colbert.state_dict().get(k))))
+ print(args.checkpoint)
+ print(checkpoint['arguments'])
+ name = "checkpoints/ptv2.colbert.msmarco.cosine.1e-3.64.filtered/colbert-360000.dnn"
+ save_checkpoint(name, 0, 360000, colbert, optimizer, checkpoint['arguments'], True)
+
+
+if __name__ == "__main__":
+ main()
diff --git a/PT-Retrieval/colbert/colbert/index.py b/PT-Retrieval/colbert/colbert/index.py
new file mode 100644
index 0000000..aead2ae
--- /dev/null
+++ b/PT-Retrieval/colbert/colbert/index.py
@@ -0,0 +1,59 @@
+import os
+import ujson
+import random
+
+from colbert.utils.runs import Run
+from colbert.utils.parser import Arguments
+import colbert.utils.distributed as distributed
+
+from colbert.utils.utils import print_message, create_directory
+from colbert.indexing.encoder import CollectionEncoder
+
+
+def main():
+ random.seed(12345)
+
+ parser = Arguments(description='Precomputing document representations with ColBERT.')
+
+ parser.add_model_parameters()
+ parser.add_model_inference_parameters()
+ parser.add_indexing_input()
+
+ parser.add_argument('--chunksize', dest='chunksize', default=6.0, required=False, type=float) # in GiBs
+
+ args = parser.parse()
+
+ with Run.context():
+ args.index_path = os.path.join(args.index_root, args.index_name)
+ assert not os.path.exists(args.index_path), args.index_path
+
+ distributed.barrier(args.rank)
+
+ if args.rank < 1:
+ create_directory(args.index_root)
+ create_directory(args.index_path)
+
+ distributed.barrier(args.rank)
+
+ process_idx = max(0, args.rank)
+ encoder = CollectionEncoder(args, process_idx=process_idx, num_processes=args.nranks)
+ encoder.encode()
+
+ distributed.barrier(args.rank)
+
+ # Save metadata.
+ if args.rank < 1:
+ metadata_path = os.path.join(args.index_path, 'metadata.json')
+ print_message("Saving (the following) metadata to", metadata_path, "..")
+ print(args.input_arguments)
+
+ with open(metadata_path, 'w') as output_metadata:
+ ujson.dump(args.input_arguments.__dict__, output_metadata)
+
+ distributed.barrier(args.rank)
+
+
+if __name__ == "__main__":
+ main()
+
+# TODO: Add resume functionality
diff --git a/PT-Retrieval/colbert/colbert/index_faiss.py b/PT-Retrieval/colbert/colbert/index_faiss.py
new file mode 100644
index 0000000..daa928a
--- /dev/null
+++ b/PT-Retrieval/colbert/colbert/index_faiss.py
@@ -0,0 +1,43 @@
+import os
+import random
+import math
+
+from colbert.utils.runs import Run
+from colbert.utils.parser import Arguments
+from colbert.indexing.faiss import index_faiss
+from colbert.indexing.loaders import load_doclens
+
+
+def main():
+ random.seed(12345)
+
+ parser = Arguments(description='Faiss indexing for end-to-end retrieval with ColBERT.')
+ parser.add_index_use_input()
+
+ parser.add_argument('--sample', dest='sample', default=None, type=float)
+ parser.add_argument('--slices', dest='slices', default=1, type=int)
+
+ args = parser.parse()
+ assert args.slices >= 1
+ assert args.sample is None or (0.0 < args.sample < 1.0), args.sample
+
+ with Run.context():
+ args.index_path = os.path.join(args.index_root, args.index_name)
+ assert os.path.exists(args.index_path), args.index_path
+
+ num_embeddings = sum(load_doclens(args.index_path))
+ print("#> num_embeddings =", num_embeddings)
+
+ if args.partitions is None:
+ args.partitions = 1 << math.ceil(math.log2(8 * math.sqrt(num_embeddings)))
+ print('\n\n')
+ Run.warn("You did not specify --partitions!")
+ Run.warn("Default computation chooses", args.partitions,
+ "partitions (for {} embeddings)".format(num_embeddings))
+ print('\n\n')
+
+ index_faiss(args)
+
+
+if __name__ == "__main__":
+ main()
diff --git a/PT-Retrieval/colbert/colbert/indexing/__init__.py b/PT-Retrieval/colbert/colbert/indexing/__init__.py
new file mode 100644
index 0000000..e69de29
diff --git a/PT-Retrieval/colbert/colbert/indexing/encoder.py b/PT-Retrieval/colbert/colbert/indexing/encoder.py
new file mode 100644
index 0000000..1117bec
--- /dev/null
+++ b/PT-Retrieval/colbert/colbert/indexing/encoder.py
@@ -0,0 +1,191 @@
+import os
+import time
+import torch
+import ujson
+import numpy as np
+
+import itertools
+import threading
+import queue
+
+from colbert.modeling.inference import ModelInference
+from colbert.evaluation.loaders import load_colbert
+from colbert.utils.utils import print_message
+
+from colbert.indexing.index_manager import IndexManager
+
+
+class CollectionEncoder():
+ def __init__(self, args, process_idx, num_processes):
+ self.args = args
+ self.collection = args.collection
+ self.process_idx = process_idx
+ self.num_processes = num_processes
+
+ assert 0.5 <= args.chunksize <= 128.0
+ max_bytes_per_file = args.chunksize * (1024*1024*1024)
+
+ max_bytes_per_doc = (self.args.doc_maxlen * self.args.dim * 2.0)
+
+ # Determine subset sizes for output
+ minimum_subset_size = 10_000
+ maximum_subset_size = max_bytes_per_file / max_bytes_per_doc
+ maximum_subset_size = max(minimum_subset_size, maximum_subset_size)
+ self.possible_subset_sizes = [int(maximum_subset_size)]
+
+ self.print_main("#> Local args.bsize =", args.bsize)
+ self.print_main("#> args.index_root =", args.index_root)
+ self.print_main(f"#> self.possible_subset_sizes = {self.possible_subset_sizes}")
+
+ self._load_model()
+ self.indexmgr = IndexManager(args.dim)
+ self.iterator = self._initialize_iterator()
+
+ def _initialize_iterator(self):
+ return open(self.collection)
+
+ def _saver_thread(self):
+ for args in iter(self.saver_queue.get, None):
+ self._save_batch(*args)
+
+ def _load_model(self):
+ self.colbert, self.checkpoint = load_colbert(self.args, do_print=(self.process_idx == 0))
+ self.colbert = self.colbert.cuda()
+ self.colbert.eval()
+
+ self.inference = ModelInference(self.colbert, amp=self.args.amp)
+
+ def encode(self):
+ self.saver_queue = queue.Queue(maxsize=3)
+ thread = threading.Thread(target=self._saver_thread)
+ thread.start()
+
+ t0 = time.time()
+ local_docs_processed = 0
+
+ for batch_idx, (offset, lines, owner) in enumerate(self._batch_passages(self.iterator)):
+ if owner != self.process_idx:
+ continue
+
+ t1 = time.time()
+ batch = self._preprocess_batch(offset, lines)
+ embs, doclens = self._encode_batch(batch_idx, batch)
+
+ t2 = time.time()
+ self.saver_queue.put((batch_idx, embs, offset, doclens))
+
+ t3 = time.time()
+ local_docs_processed += len(lines)
+ overall_throughput = compute_throughput(local_docs_processed, t0, t3)
+ this_encoding_throughput = compute_throughput(len(lines), t1, t2)
+ this_saving_throughput = compute_throughput(len(lines), t2, t3)
+
+ self.print(f'#> Completed batch #{batch_idx} (starting at passage #{offset}) \t\t'
+ f'Passages/min: {overall_throughput} (overall), ',
+ f'{this_encoding_throughput} (this encoding), ',
+ f'{this_saving_throughput} (this saving)')
+ self.saver_queue.put(None)
+
+ self.print("#> Joining saver thread.")
+ thread.join()
+
+ def _batch_passages(self, fi):
+ """
+ Must use the same seed across processes!
+ """
+ np.random.seed(0)
+
+ offset = 0
+ for owner in itertools.cycle(range(self.num_processes)):
+ batch_size = np.random.choice(self.possible_subset_sizes)
+
+ L = [line for _, line in zip(range(batch_size), fi)]
+
+ if len(L) == 0:
+ break # EOF
+
+ yield (offset, L, owner)
+ offset += len(L)
+
+ if len(L) < batch_size:
+ break # EOF
+
+ self.print("[NOTE] Done with local share.")
+
+ return
+
+ def _preprocess_batch(self, offset, lines):
+ endpos = offset + len(lines)
+
+ batch = []
+
+ for line_idx, line in zip(range(offset, endpos), lines):
+ line_parts = line.strip().split('\t')
+
+ try:
+ pid, passage, *other = line_parts
+
+ assert len(passage) >= 1
+
+ if len(other) >= 1:
+ title, *_ = other
+ passage = title + ' | ' + passage
+
+ except:
+ print_message(line_parts)
+ passage = ""
+
+ batch.append(passage)
+ # assert pid == 'id' or int(pid) == line_idx
+
+ return batch
+
+ def _encode_batch(self, batch_idx, batch):
+ with torch.no_grad():
+ embs = self.inference.docFromText(batch, bsize=self.args.bsize, keep_dims=False)
+ assert type(embs) is list
+ assert len(embs) == len(batch)
+
+ local_doclens = [d.size(0) for d in embs]
+ embs = torch.cat(embs)
+
+ return embs, local_doclens
+
+ def _save_batch(self, batch_idx, embs, offset, doclens):
+ start_time = time.time()
+
+ output_path = os.path.join(self.args.index_path, "{}.pt".format(batch_idx))
+ output_sample_path = os.path.join(self.args.index_path, "{}.sample".format(batch_idx))
+ doclens_path = os.path.join(self.args.index_path, 'doclens.{}.json'.format(batch_idx))
+
+ # Save the embeddings.
+ self.indexmgr.save(embs, output_path)
+ self.indexmgr.save(embs[torch.randint(0, high=embs.size(0), size=(embs.size(0) // 20,))], output_sample_path)
+
+ # Save the doclens.
+ with open(doclens_path, 'w') as output_doclens:
+ ujson.dump(doclens, output_doclens)
+
+ throughput = compute_throughput(len(doclens), start_time, time.time())
+ self.print_main("#> Saved batch #{} to {} \t\t".format(batch_idx, output_path),
+ "Saving Throughput =", throughput, "passages per minute.\n")
+
+ def print(self, *args):
+ print_message("[" + str(self.process_idx) + "]", "\t\t", *args)
+
+ def print_main(self, *args):
+ if self.process_idx == 0:
+ self.print(*args)
+
+
+def compute_throughput(size, t0, t1):
+ throughput = size / (t1 - t0) * 60
+
+ if throughput > 1000 * 1000:
+ throughput = throughput / (1000*1000)
+ throughput = round(throughput, 1)
+ return '{}M'.format(throughput)
+
+ throughput = throughput / (1000)
+ throughput = round(throughput, 1)
+ return '{}k'.format(throughput)
diff --git a/PT-Retrieval/colbert/colbert/indexing/faiss.py b/PT-Retrieval/colbert/colbert/indexing/faiss.py
new file mode 100644
index 0000000..c0d5564
--- /dev/null
+++ b/PT-Retrieval/colbert/colbert/indexing/faiss.py
@@ -0,0 +1,116 @@
+import os
+import math
+import faiss
+import torch
+import numpy as np
+
+import threading
+import queue
+
+from colbert.utils.utils import print_message, grouper
+from colbert.indexing.loaders import get_parts
+from colbert.indexing.index_manager import load_index_part
+from colbert.indexing.faiss_index import FaissIndex
+
+
+def get_faiss_index_name(args, offset=None, endpos=None):
+ partitions_info = '' if args.partitions is None else f'.{args.partitions}'
+ range_info = '' if offset is None else f'.{offset}-{endpos}'
+
+ return f'ivfpq{partitions_info}{range_info}.faiss'
+
+
+def load_sample(samples_paths, sample_fraction=None):
+ sample = []
+
+ for filename in samples_paths:
+ print_message(f"#> Loading {filename} ...")
+ part = load_index_part(filename)
+ if sample_fraction:
+ part = part[torch.randint(0, high=part.size(0), size=(int(part.size(0) * sample_fraction),))]
+ sample.append(part)
+
+ sample = torch.cat(sample).float().numpy()
+
+ print("#> Sample has shape", sample.shape)
+
+ return sample
+
+
+def prepare_faiss_index(slice_samples_paths, partitions, sample_fraction=None):
+ training_sample = load_sample(slice_samples_paths, sample_fraction=sample_fraction)
+
+ dim = training_sample.shape[-1]
+ index = FaissIndex(dim, partitions)
+
+ print_message("#> Training with the vectors...")
+
+ index.train(training_sample)
+
+ print_message("Done training!\n")
+
+ return index
+
+
+SPAN = 3
+
+
+def index_faiss(args):
+ print_message("#> Starting..")
+
+ parts, parts_paths, samples_paths = get_parts(args.index_path)
+
+ if args.sample is not None:
+ assert args.sample, args.sample
+ print_message(f"#> Training with {round(args.sample * 100.0, 1)}% of *all* embeddings (provided --sample).")
+ samples_paths = parts_paths
+
+ num_parts_per_slice = math.ceil(len(parts) / args.slices)
+
+ for slice_idx, part_offset in enumerate(range(0, len(parts), num_parts_per_slice)):
+ part_endpos = min(part_offset + num_parts_per_slice, len(parts))
+
+ slice_parts_paths = parts_paths[part_offset:part_endpos]
+ slice_samples_paths = samples_paths[part_offset:part_endpos]
+
+ if args.slices == 1:
+ faiss_index_name = get_faiss_index_name(args)
+ else:
+ faiss_index_name = get_faiss_index_name(args, offset=part_offset, endpos=part_endpos)
+
+ output_path = os.path.join(args.index_path, faiss_index_name)
+ print_message(f"#> Processing slice #{slice_idx+1} of {args.slices} (range {part_offset}..{part_endpos}).")
+ print_message(f"#> Will write to {output_path}.")
+
+ assert not os.path.exists(output_path), output_path
+
+ index = prepare_faiss_index(slice_samples_paths, args.partitions, args.sample)
+
+ loaded_parts = queue.Queue(maxsize=1)
+
+ def _loader_thread(thread_parts_paths):
+ for filenames in grouper(thread_parts_paths, SPAN, fillvalue=None):
+ sub_collection = [load_index_part(filename) for filename in filenames if filename is not None]
+ sub_collection = torch.cat(sub_collection)
+ sub_collection = sub_collection.float().numpy()
+ loaded_parts.put(sub_collection)
+
+ thread = threading.Thread(target=_loader_thread, args=(slice_parts_paths,))
+ thread.start()
+
+ print_message("#> Indexing the vectors...")
+
+ for filenames in grouper(slice_parts_paths, SPAN, fillvalue=None):
+ print_message("#> Loading", filenames, "(from queue)...")
+ sub_collection = loaded_parts.get()
+
+ print_message("#> Processing a sub_collection with shape", sub_collection.shape)
+ index.add(sub_collection)
+
+ print_message("Done indexing!")
+
+ index.save(output_path)
+
+ print_message(f"\n\nDone! All complete (for slice #{slice_idx+1} of {args.slices})!")
+
+ thread.join()
diff --git a/PT-Retrieval/colbert/colbert/indexing/faiss_index.py b/PT-Retrieval/colbert/colbert/indexing/faiss_index.py
new file mode 100644
index 0000000..7bb3a50
--- /dev/null
+++ b/PT-Retrieval/colbert/colbert/indexing/faiss_index.py
@@ -0,0 +1,58 @@
+import sys
+import time
+import math
+import faiss
+import torch
+
+import numpy as np
+
+from colbert.indexing.faiss_index_gpu import FaissIndexGPU
+from colbert.utils.utils import print_message
+
+
+class FaissIndex():
+ def __init__(self, dim, partitions):
+ self.dim = dim
+ self.partitions = partitions
+
+ self.gpu = FaissIndexGPU()
+ self.quantizer, self.index = self._create_index()
+ self.offset = 0
+
+ def _create_index(self):
+ quantizer = faiss.IndexFlatL2(self.dim) # faiss.IndexHNSWFlat(dim, 32)
+ index = faiss.IndexIVFPQ(quantizer, self.dim, self.partitions, 16, 8)
+
+ return quantizer, index
+
+ def train(self, train_data):
+ print_message(f"#> Training now (using {self.gpu.ngpu} GPUs)...")
+
+ if self.gpu.ngpu > 0:
+ self.gpu.training_initialize(self.index, self.quantizer)
+
+ s = time.time()
+ self.index.train(train_data)
+ print(time.time() - s)
+
+ if self.gpu.ngpu > 0:
+ self.gpu.training_finalize()
+
+ def add(self, data):
+ print_message(f"Add data with shape {data.shape} (offset = {self.offset})..")
+
+ if self.gpu.ngpu > 0 and self.offset == 0:
+ self.gpu.adding_initialize(self.index)
+
+ if self.gpu.ngpu > 0:
+ self.gpu.add(self.index, data, self.offset)
+ else:
+ self.index.add(data)
+
+ self.offset += data.shape[0]
+
+ def save(self, output_path):
+ print_message(f"Writing index to {output_path} ...")
+
+ self.index.nprobe = 10 # just a default
+ faiss.write_index(self.index, output_path)
diff --git a/PT-Retrieval/colbert/colbert/indexing/faiss_index_gpu.py b/PT-Retrieval/colbert/colbert/indexing/faiss_index_gpu.py
new file mode 100644
index 0000000..2d4205c
--- /dev/null
+++ b/PT-Retrieval/colbert/colbert/indexing/faiss_index_gpu.py
@@ -0,0 +1,138 @@
+"""
+ Heavily based on: https://github.com/facebookresearch/faiss/blob/master/benchs/bench_gpu_1bn.py
+"""
+
+
+import sys
+import time
+import math
+import faiss
+import torch
+
+import numpy as np
+from colbert.utils.utils import print_message
+
+
+class FaissIndexGPU():
+ def __init__(self):
+ self.ngpu = faiss.get_num_gpus()
+
+ if self.ngpu == 0:
+ return
+
+ self.tempmem = 1 << 33
+ self.max_add_per_gpu = 1 << 25
+ self.max_add = self.max_add_per_gpu * self.ngpu
+ self.add_batch_size = 65536
+
+ self.gpu_resources = self._prepare_gpu_resources()
+
+ def _prepare_gpu_resources(self):
+ print_message(f"Preparing resources for {self.ngpu} GPUs.")
+
+ gpu_resources = []
+
+ for _ in range(self.ngpu):
+ res = faiss.StandardGpuResources()
+ if self.tempmem >= 0:
+ res.setTempMemory(self.tempmem)
+ gpu_resources.append(res)
+
+ return gpu_resources
+
+ def _make_vres_vdev(self):
+ """
+ return vectors of device ids and resources useful for gpu_multiple
+ """
+
+ assert self.ngpu > 0
+
+ vres = faiss.GpuResourcesVector()
+ vdev = faiss.IntVector()
+
+ for i in range(self.ngpu):
+ vdev.push_back(i)
+ vres.push_back(self.gpu_resources[i])
+
+ return vres, vdev
+
+ def training_initialize(self, index, quantizer):
+ """
+ The index and quantizer should be owned by caller.
+ """
+
+ assert self.ngpu > 0
+
+ s = time.time()
+ self.index_ivf = faiss.extract_index_ivf(index)
+ self.clustering_index = faiss.index_cpu_to_all_gpus(quantizer)
+ self.index_ivf.clustering_index = self.clustering_index
+ print(time.time() - s)
+
+ def training_finalize(self):
+ assert self.ngpu > 0
+
+ s = time.time()
+ self.index_ivf.clustering_index = faiss.index_gpu_to_cpu(self.index_ivf.clustering_index)
+ print(time.time() - s)
+
+ def adding_initialize(self, index):
+ """
+ The index should be owned by caller.
+ """
+
+ assert self.ngpu > 0
+
+ self.co = faiss.GpuMultipleClonerOptions()
+ self.co.useFloat16 = True
+ self.co.useFloat16CoarseQuantizer = False
+ self.co.usePrecomputed = False
+ self.co.indicesOptions = faiss.INDICES_CPU
+ self.co.verbose = True
+ self.co.reserveVecs = self.max_add
+ self.co.shard = True
+ assert self.co.shard_type in (0, 1, 2)
+
+ self.vres, self.vdev = self._make_vres_vdev()
+ self.gpu_index = faiss.index_cpu_to_gpu_multiple(self.vres, self.vdev, index, self.co)
+
+ def add(self, index, data, offset):
+ assert self.ngpu > 0
+
+ t0 = time.time()
+ nb = data.shape[0]
+
+ for i0 in range(0, nb, self.add_batch_size):
+ i1 = min(i0 + self.add_batch_size, nb)
+ xs = data[i0:i1]
+
+ self.gpu_index.add_with_ids(xs, np.arange(offset+i0, offset+i1))
+
+ if self.max_add > 0 and self.gpu_index.ntotal > self.max_add:
+ self._flush_to_cpu(index, nb, offset)
+
+ print('\r%d/%d (%.3f s) ' % (i0, nb, time.time() - t0), end=' ')
+ sys.stdout.flush()
+
+ if self.gpu_index.ntotal > 0:
+ self._flush_to_cpu(index, nb, offset)
+
+ assert index.ntotal == offset+nb, (index.ntotal, offset+nb, offset, nb)
+ print(f"add(.) time: %.3f s \t\t--\t\t index.ntotal = {index.ntotal}" % (time.time() - t0))
+
+ def _flush_to_cpu(self, index, nb, offset):
+ print("Flush indexes to CPU")
+
+ for i in range(self.ngpu):
+ index_src_gpu = faiss.downcast_index(self.gpu_index if self.ngpu == 1 else self.gpu_index.at(i))
+ index_src = faiss.index_gpu_to_cpu(index_src_gpu)
+
+ index_src.copy_subset_to(index, 0, offset, offset+nb)
+ index_src_gpu.reset()
+ index_src_gpu.reserveMemory(self.max_add)
+
+ if self.ngpu > 1:
+ try:
+ self.gpu_index.sync_with_shard_indexes()
+ except:
+ self.gpu_index.syncWithSubIndexes()
diff --git a/PT-Retrieval/colbert/colbert/indexing/index_manager.py b/PT-Retrieval/colbert/colbert/indexing/index_manager.py
new file mode 100644
index 0000000..a79fac7
--- /dev/null
+++ b/PT-Retrieval/colbert/colbert/indexing/index_manager.py
@@ -0,0 +1,22 @@
+import torch
+import faiss
+import numpy as np
+
+from colbert.utils.utils import print_message
+
+
+class IndexManager():
+ def __init__(self, dim):
+ self.dim = dim
+
+ def save(self, tensor, path_prefix):
+ torch.save(tensor, path_prefix)
+
+
+def load_index_part(filename, verbose=True):
+ part = torch.load(filename)
+
+ if type(part) == list: # for backward compatibility
+ part = torch.cat(part)
+
+ return part
diff --git a/PT-Retrieval/colbert/colbert/indexing/loaders.py b/PT-Retrieval/colbert/colbert/indexing/loaders.py
new file mode 100644
index 0000000..9c5e969
--- /dev/null
+++ b/PT-Retrieval/colbert/colbert/indexing/loaders.py
@@ -0,0 +1,34 @@
+import os
+import torch
+import ujson
+
+from math import ceil
+from itertools import accumulate
+from colbert.utils.utils import print_message
+
+
+def get_parts(directory):
+ extension = '.pt'
+
+ parts = sorted([int(filename[: -1 * len(extension)]) for filename in os.listdir(directory)
+ if filename.endswith(extension)])
+
+ assert list(range(len(parts))) == parts, parts
+
+ # Integer-sortedness matters.
+ parts_paths = [os.path.join(directory, '{}{}'.format(filename, extension)) for filename in parts]
+ samples_paths = [os.path.join(directory, '{}.sample'.format(filename)) for filename in parts]
+
+ return parts, parts_paths, samples_paths
+
+
+def load_doclens(directory, flatten=True):
+ parts, _, _ = get_parts(directory)
+
+ doclens_filenames = [os.path.join(directory, 'doclens.{}.json'.format(filename)) for filename in parts]
+ all_doclens = [ujson.load(open(filename)) for filename in doclens_filenames]
+
+ if flatten:
+ all_doclens = [x for sub_doclens in all_doclens for x in sub_doclens]
+
+ return all_doclens
diff --git a/PT-Retrieval/colbert/colbert/modeling/__init__.py b/PT-Retrieval/colbert/colbert/modeling/__init__.py
new file mode 100644
index 0000000..e69de29
diff --git a/PT-Retrieval/colbert/colbert/modeling/colbert.py b/PT-Retrieval/colbert/colbert/modeling/colbert.py
new file mode 100644
index 0000000..7b47024
--- /dev/null
+++ b/PT-Retrieval/colbert/colbert/modeling/colbert.py
@@ -0,0 +1,68 @@
+import string
+import torch
+import torch.nn as nn
+
+from transformers import BertPreTrainedModel, BertModel, BertTokenizerFast
+from colbert.parameters import DEVICE
+
+
+class ColBERT(BertPreTrainedModel):
+ def __init__(self, config, query_maxlen, doc_maxlen, mask_punctuation, dim=128, similarity_metric='cosine'):
+
+ super(ColBERT, self).__init__(config)
+
+ self.query_maxlen = query_maxlen
+ self.doc_maxlen = doc_maxlen
+ self.similarity_metric = similarity_metric
+ self.dim = dim
+
+ self.mask_punctuation = mask_punctuation
+ self.skiplist = {}
+
+ if self.mask_punctuation:
+ self.tokenizer = BertTokenizerFast.from_pretrained('bert-base-uncased')
+ self.skiplist = {w: True
+ for symbol in string.punctuation
+ for w in [symbol, self.tokenizer.encode(symbol, add_special_tokens=False)[0]]}
+
+ self.bert = BertModel(config)
+ self.linear = nn.Linear(config.hidden_size, dim, bias=False)
+
+ self.init_weights()
+
+ def forward(self, Q, D):
+ return self.score(self.query(*Q), self.doc(*D))
+
+ def query(self, input_ids, attention_mask):
+ input_ids, attention_mask = input_ids.to(DEVICE), attention_mask.to(DEVICE)
+ Q = self.bert(input_ids, attention_mask=attention_mask)[0]
+ Q = self.linear(Q)
+
+ return torch.nn.functional.normalize(Q, p=2, dim=2)
+
+ def doc(self, input_ids, attention_mask, keep_dims=True):
+ input_ids, attention_mask = input_ids.to(DEVICE), attention_mask.to(DEVICE)
+ D = self.bert(input_ids, attention_mask=attention_mask)[0]
+ D = self.linear(D)
+
+ mask = torch.tensor(self.mask(input_ids), device=DEVICE).unsqueeze(2).float()
+ D = D * mask
+
+ D = torch.nn.functional.normalize(D, p=2, dim=2)
+
+ if not keep_dims:
+ D, mask = D.cpu().to(dtype=torch.float16), mask.cpu().bool().squeeze(-1)
+ D = [d[mask[idx]] for idx, d in enumerate(D)]
+
+ return D
+
+ def score(self, Q, D):
+ if self.similarity_metric == 'cosine':
+ return (Q @ D.permute(0, 2, 1)).max(2).values.sum(1)
+
+ assert self.similarity_metric == 'l2'
+ return (-1.0 * ((Q.unsqueeze(2) - D.unsqueeze(1))**2).sum(-1)).max(-1).values.sum(-1)
+
+ def mask(self, input_ids):
+ mask = [[(x not in self.skiplist) and (x != 0) for x in d] for d in input_ids.cpu().tolist()]
+ return mask
diff --git a/PT-Retrieval/colbert/colbert/modeling/inference.py b/PT-Retrieval/colbert/colbert/modeling/inference.py
new file mode 100644
index 0000000..e60f4f0
--- /dev/null
+++ b/PT-Retrieval/colbert/colbert/modeling/inference.py
@@ -0,0 +1,87 @@
+import torch
+
+from colbert.modeling.colbert import ColBERT
+from colbert.modeling.tokenization import QueryTokenizer, DocTokenizer
+from colbert.utils.amp import MixedPrecisionManager
+from colbert.parameters import DEVICE
+
+
+class ModelInference():
+ def __init__(self, colbert: ColBERT, amp=False):
+ assert colbert.training is False
+
+ self.colbert = colbert
+ self.query_tokenizer = QueryTokenizer(colbert.query_maxlen)
+ self.doc_tokenizer = DocTokenizer(colbert.doc_maxlen)
+
+ self.amp_manager = MixedPrecisionManager(amp)
+
+ def query(self, *args, to_cpu=False, **kw_args):
+ with torch.no_grad():
+ with self.amp_manager.context():
+ Q = self.colbert.query(*args, **kw_args)
+ return Q.cpu() if to_cpu else Q
+
+ def doc(self, *args, to_cpu=False, **kw_args):
+ with torch.no_grad():
+ with self.amp_manager.context():
+ D = self.colbert.doc(*args, **kw_args)
+ return D.cpu() if to_cpu else D
+
+ def queryFromText(self, queries, bsize=None, to_cpu=False):
+ if bsize:
+ batches = self.query_tokenizer.tensorize(queries, bsize=bsize)
+ batches = [self.query(input_ids, attention_mask, to_cpu=to_cpu) for input_ids, attention_mask in batches]
+ return torch.cat(batches)
+
+ input_ids, attention_mask = self.query_tokenizer.tensorize(queries)
+ return self.query(input_ids, attention_mask)
+
+ def docFromText(self, docs, bsize=None, keep_dims=True, to_cpu=False):
+ if bsize:
+ batches, reverse_indices = self.doc_tokenizer.tensorize(docs, bsize=bsize)
+
+ batches = [self.doc(input_ids, attention_mask, keep_dims=keep_dims, to_cpu=to_cpu)
+ for input_ids, attention_mask in batches]
+
+ if keep_dims:
+ D = _stack_3D_tensors(batches)
+ return D[reverse_indices]
+
+ D = [d for batch in batches for d in batch]
+ return [D[idx] for idx in reverse_indices.tolist()]
+
+ input_ids, attention_mask = self.doc_tokenizer.tensorize(docs)
+ return self.doc(input_ids, attention_mask, keep_dims=keep_dims)
+
+ def score(self, Q, D, mask=None, lengths=None, explain=False):
+ if lengths is not None:
+ assert mask is None, "don't supply both mask and lengths"
+
+ mask = torch.arange(D.size(1), device=DEVICE) + 1
+ mask = mask.unsqueeze(0) <= lengths.to(DEVICE).unsqueeze(-1)
+
+ scores = (D @ Q)
+ scores = scores if mask is None else scores * mask.unsqueeze(-1)
+ scores = scores.max(1)
+
+ if explain:
+ assert False, "TODO"
+
+ return scores.values.sum(-1).cpu()
+
+
+def _stack_3D_tensors(groups):
+ bsize = sum([x.size(0) for x in groups])
+ maxlen = max([x.size(1) for x in groups])
+ hdim = groups[0].size(2)
+
+ output = torch.zeros(bsize, maxlen, hdim, device=groups[0].device, dtype=groups[0].dtype)
+
+ offset = 0
+ for x in groups:
+ endpos = offset + x.size(0)
+ output[offset:endpos, :x.size(1)] = x
+ offset = endpos
+
+ return output
diff --git a/PT-Retrieval/colbert/colbert/modeling/prefix.py b/PT-Retrieval/colbert/colbert/modeling/prefix.py
new file mode 100644
index 0000000..6af272e
--- /dev/null
+++ b/PT-Retrieval/colbert/colbert/modeling/prefix.py
@@ -0,0 +1,261 @@
+import string
+import torch
+import torch.nn as nn
+
+from colbert.parameters import DEVICE
+
+from transformers import BertPreTrainedModel, BertModel, BertTokenizerFast
+from transformers.modeling_outputs import BaseModelOutputWithPoolingAndCrossAttentions
+
+class PrefixEncoder(torch.nn.Module):
+ r'''
+ The torch.nn model to encode the prefix
+
+ Input shape: (batch-size, prefix-length)
+
+ Output shape: (batch-size, prefix-length, 2*layers*hidden)
+ '''
+ def __init__(self, config):
+ super().__init__()
+ self.prefix_mlp = config.prefix_mlp
+ print(f" > Initializing PrefixEncoder: prefix_mlp:{config.prefix_mlp} prefix_hidden_size={config.prefix_hidden_size}")
+
+ if self.prefix_mlp:
+ # Use a two-layer MLP to encode the prefix
+ self.embedding = torch.nn.Embedding(config.pre_seq_len, config.hidden_size)
+ self.trans = torch.nn.Sequential(
+ torch.nn.Linear(config.hidden_size, config.prefix_hidden_size),
+ torch.nn.Tanh(),
+ torch.nn.Linear(config.prefix_hidden_size, config.num_hidden_layers * 2 * config.hidden_size)
+ )
+ else:
+ self.embedding = torch.nn.Embedding(config.pre_seq_len, config.num_hidden_layers * 2 * config.hidden_size)
+
+ def forward(self, prefix: torch.Tensor):
+ if self.prefix_mlp:
+ prefix_tokens = self.embedding(prefix)
+ past_key_values = self.trans(prefix_tokens)
+ else:
+ past_key_values = self.embedding(prefix)
+ return past_key_values
+
+
+class BertModelModifed(BertModel):
+ def __init__(self, config, add_pooling_layer=True):
+ super().__init__(config)
+
+ def forward(
+ self,
+ input_ids=None,
+ attention_mask=None,
+ token_type_ids=None,
+ position_ids=None,
+ head_mask=None,
+ inputs_embeds=None,
+ encoder_hidden_states=None,
+ encoder_attention_mask=None,
+ past_key_values=None,
+ use_cache=None,
+ output_attentions=None,
+ output_hidden_states=None,
+ return_dict=None,
+ ):
+ output_attentions = output_attentions if output_attentions is not None else self.config.output_attentions
+ output_hidden_states = (
+ output_hidden_states if output_hidden_states is not None else self.config.output_hidden_states
+ )
+ return_dict = return_dict if return_dict is not None else self.config.use_return_dict
+
+ if self.config.is_decoder:
+ use_cache = use_cache if use_cache is not None else self.config.use_cache
+ else:
+ use_cache = False
+
+ if input_ids is not None and inputs_embeds is not None:
+ raise ValueError("You cannot specify both input_ids and inputs_embeds at the same time")
+ elif input_ids is not None:
+ input_shape = input_ids.size()
+ elif inputs_embeds is not None:
+ input_shape = inputs_embeds.size()[:-1]
+ else:
+ raise ValueError("You have to specify either input_ids or inputs_embeds")
+
+ batch_size, seq_length = input_shape
+ device = input_ids.device if input_ids is not None else inputs_embeds.device
+
+ # past_key_values_length
+ past_key_values_length = past_key_values[0][0].shape[2] if past_key_values is not None else 0
+
+ if attention_mask is None:
+ attention_mask = torch.ones(((batch_size, seq_length + past_key_values_length)), device=device)
+
+ if token_type_ids is None:
+ if hasattr(self.embeddings, "token_type_ids"):
+ buffered_token_type_ids = self.embeddings.token_type_ids[:, :seq_length]
+ buffered_token_type_ids_expanded = buffered_token_type_ids.expand(batch_size, seq_length)
+ token_type_ids = buffered_token_type_ids_expanded
+ else:
+ token_type_ids = torch.zeros(input_shape, dtype=torch.long, device=device)
+
+ # We can provide a self-attention mask of dimensions [batch_size, from_seq_length, to_seq_length]
+ # ourselves in which case we just need to make it broadcastable to all heads.
+ extended_attention_mask: torch.Tensor = self.get_extended_attention_mask(attention_mask, input_shape, device)
+
+ # If a 2D or 3D attention mask is provided for the cross-attention
+ # we need to make broadcastable to [batch_size, num_heads, seq_length, seq_length]
+ if self.config.is_decoder and encoder_hidden_states is not None:
+ encoder_batch_size, encoder_sequence_length, _ = encoder_hidden_states.size()
+ encoder_hidden_shape = (encoder_batch_size, encoder_sequence_length)
+ if encoder_attention_mask is None:
+ encoder_attention_mask = torch.ones(encoder_hidden_shape, device=device)
+ encoder_extended_attention_mask = self.invert_attention_mask(encoder_attention_mask)
+ else:
+ encoder_extended_attention_mask = None
+
+ # Prepare head mask if needed
+ # 1.0 in head_mask indicate we keep the head
+ # attention_probs has shape bsz x n_heads x N x N
+ # input head_mask has shape [num_heads] or [num_hidden_layers x num_heads]
+ # and head_mask is converted to shape [num_hidden_layers x batch x num_heads x seq_length x seq_length]
+ head_mask = self.get_head_mask(head_mask, self.config.num_hidden_layers)
+
+ embedding_output = self.embeddings(
+ input_ids=input_ids,
+ position_ids=position_ids,
+ token_type_ids=token_type_ids,
+ inputs_embeds=inputs_embeds,
+ past_key_values_length=0,
+ )
+ encoder_outputs = self.encoder(
+ embedding_output,
+ attention_mask=extended_attention_mask,
+ head_mask=head_mask,
+ encoder_hidden_states=encoder_hidden_states,
+ encoder_attention_mask=encoder_extended_attention_mask,
+ past_key_values=past_key_values,
+ use_cache=use_cache,
+ output_attentions=output_attentions,
+ output_hidden_states=output_hidden_states,
+ return_dict=return_dict,
+ )
+ sequence_output = encoder_outputs[0]
+ pooled_output = self.pooler(sequence_output) if self.pooler is not None else None
+
+ if not return_dict:
+ return (sequence_output, pooled_output) + encoder_outputs[1:]
+
+ return BaseModelOutputWithPoolingAndCrossAttentions(
+ last_hidden_state=sequence_output,
+ pooler_output=pooled_output,
+ past_key_values=encoder_outputs.past_key_values,
+ hidden_states=encoder_outputs.hidden_states,
+ attentions=encoder_outputs.attentions,
+ cross_attentions=encoder_outputs.cross_attentions,
+ )
+
+class PrefixColBERT(BertPreTrainedModel):
+ def __init__(self, config, query_maxlen, doc_maxlen, mask_punctuation, dim=128, similarity_metric='cosine'):
+
+ super(PrefixColBERT, self).__init__(config)
+ self.config = config
+ self.query_maxlen = query_maxlen
+ self.doc_maxlen = doc_maxlen
+ self.similarity_metric = similarity_metric
+ self.dim = dim
+
+ self.mask_punctuation = mask_punctuation
+ self.skiplist = {}
+
+ if self.mask_punctuation:
+ self.tokenizer = BertTokenizerFast.from_pretrained('bert-base-uncased')
+ self.skiplist = {w: True
+ for symbol in string.punctuation
+ for w in [symbol, self.tokenizer.encode(symbol, add_special_tokens=False)[0]]}
+
+ self.bert = BertModel(config)
+ self.dropout = torch.nn.Dropout(config.hidden_dropout_prob)
+ self.linear = nn.Linear(config.hidden_size, dim, bias=False)
+
+ self.init_weights()
+
+ for param in self.bert.parameters():
+ param.requires_grad = False
+
+ self.pre_seq_len = config.pre_seq_len
+ self.n_layer = config.num_hidden_layers
+ self.n_head = config.num_attention_heads
+ self.n_embd = config.hidden_size // config.num_attention_heads
+
+ self.prefix_tokens = torch.arange(self.pre_seq_len).long()
+ self.prefix_encoder = PrefixEncoder(config)
+ # self.prefix_encoder = torch.nn.Embedding(self.pre_seq_len, config.hidden_size)
+
+ bert_param = 0
+ for name, param in self.bert.named_parameters():
+ bert_param += param.numel()
+ all_param = 0
+ for name, param in self.named_parameters():
+ all_param += param.numel()
+ total_param = all_param - bert_param
+ print(f"total param is {all_param} => {total_param}")
+
+ def get_prompt(self, batch_size):
+ prefix_tokens = self.prefix_tokens.unsqueeze(0).expand(batch_size, -1).to(DEVICE)
+ past_key_values = self.prefix_encoder(prefix_tokens)
+ past_key_values = past_key_values.view(
+ batch_size,
+ self.pre_seq_len,
+ self.n_layer * 2,
+ self.n_head,
+ self.n_embd
+ )
+ past_key_values = self.dropout(past_key_values)
+ past_key_values = past_key_values.permute([2, 0, 3, 1, 4]).split(2)
+ return past_key_values
+
+ def forward(self, Q, D):
+ return self.score(self.query(*Q), self.doc(*D))
+
+ def query(self, input_ids, attention_mask):
+ input_ids, attention_mask = input_ids.to(DEVICE), attention_mask.to(DEVICE)
+ batch_size = input_ids.shape[0]
+ past_key_values = self.get_prompt(batch_size=batch_size)
+ prefix_attention_mask = torch.ones(batch_size, self.pre_seq_len).to(DEVICE)
+ attention_mask = torch.cat((prefix_attention_mask, attention_mask), dim=1)
+
+ Q = self.bert(input_ids, attention_mask=attention_mask, past_key_values=past_key_values)[0]
+ Q = self.linear(Q)
+
+ return torch.nn.functional.normalize(Q, p=2, dim=2)
+
+ def doc(self, input_ids, attention_mask, keep_dims=True):
+ input_ids, attention_mask = input_ids.to(DEVICE), attention_mask.to(DEVICE)
+ batch_size = input_ids.shape[0]
+ past_key_values = self.get_prompt(batch_size=batch_size)
+ prefix_attention_mask = torch.ones(batch_size, self.pre_seq_len).to(DEVICE)
+ attention_mask = torch.cat((prefix_attention_mask, attention_mask), dim=1)
+
+ D = self.bert(input_ids, attention_mask=attention_mask, past_key_values=past_key_values)[0]
+ D = self.linear(D)
+
+ mask = torch.tensor(self.mask(input_ids), device=DEVICE).unsqueeze(2).float()
+ D = D * mask
+
+ D = torch.nn.functional.normalize(D, p=2, dim=2)
+
+ if not keep_dims:
+ D, mask = D.cpu().to(dtype=torch.float16), mask.cpu().bool().squeeze(-1)
+ D = [d[mask[idx]] for idx, d in enumerate(D)]
+
+ return D
+
+ def score(self, Q, D):
+ if self.similarity_metric == 'cosine':
+ return (Q @ D.permute(0, 2, 1)).max(2).values.sum(1)
+
+ assert self.similarity_metric == 'l2'
+ return (-1.0 * ((Q.unsqueeze(2) - D.unsqueeze(1))**2).sum(-1)).max(-1).values.sum(-1)
+
+ def mask(self, input_ids):
+ mask = [[(x not in self.skiplist) and (x != 0) for x in d] for d in input_ids.cpu().tolist()]
+ return mask
diff --git a/PT-Retrieval/colbert/colbert/modeling/tokenization/__init__.py b/PT-Retrieval/colbert/colbert/modeling/tokenization/__init__.py
new file mode 100644
index 0000000..bd590eb
--- /dev/null
+++ b/PT-Retrieval/colbert/colbert/modeling/tokenization/__init__.py
@@ -0,0 +1,3 @@
+from colbert.modeling.tokenization.query_tokenization import *
+from colbert.modeling.tokenization.doc_tokenization import *
+from colbert.modeling.tokenization.utils import tensorize_triples
diff --git a/PT-Retrieval/colbert/colbert/modeling/tokenization/doc_tokenization.py b/PT-Retrieval/colbert/colbert/modeling/tokenization/doc_tokenization.py
new file mode 100644
index 0000000..8d2c96f
--- /dev/null
+++ b/PT-Retrieval/colbert/colbert/modeling/tokenization/doc_tokenization.py
@@ -0,0 +1,63 @@
+import torch
+
+from transformers import BertTokenizerFast
+from colbert.modeling.tokenization.utils import _split_into_batches, _sort_by_length
+
+
+class DocTokenizer():
+ def __init__(self, doc_maxlen):
+ self.tok = BertTokenizerFast.from_pretrained('bert-base-uncased')
+ self.doc_maxlen = doc_maxlen
+
+ self.D_marker_token, self.D_marker_token_id = '[D]', self.tok.convert_tokens_to_ids('[unused1]')
+ self.cls_token, self.cls_token_id = self.tok.cls_token, self.tok.cls_token_id
+ self.sep_token, self.sep_token_id = self.tok.sep_token, self.tok.sep_token_id
+
+ assert self.D_marker_token_id == 2
+
+ def tokenize(self, batch_text, add_special_tokens=False):
+ assert type(batch_text) in [list, tuple], (type(batch_text))
+
+ tokens = [self.tok.tokenize(x, add_special_tokens=False) for x in batch_text]
+
+ if not add_special_tokens:
+ return tokens
+
+ prefix, suffix = [self.cls_token, self.D_marker_token], [self.sep_token]
+ tokens = [prefix + lst + suffix for lst in tokens]
+
+ return tokens
+
+ def encode(self, batch_text, add_special_tokens=False):
+ assert type(batch_text) in [list, tuple], (type(batch_text))
+
+ ids = self.tok(batch_text, add_special_tokens=False)['input_ids']
+
+ if not add_special_tokens:
+ return ids
+
+ prefix, suffix = [self.cls_token_id, self.D_marker_token_id], [self.sep_token_id]
+ ids = [prefix + lst + suffix for lst in ids]
+
+ return ids
+
+ def tensorize(self, batch_text, bsize=None):
+ assert type(batch_text) in [list, tuple], (type(batch_text))
+
+ # add placehold for the [D] marker
+ batch_text = ['. ' + x for x in batch_text]
+
+ obj = self.tok(batch_text, padding='longest', truncation='longest_first',
+ return_tensors='pt', max_length=self.doc_maxlen)
+
+ ids, mask = obj['input_ids'], obj['attention_mask']
+
+ # postprocess for the [D] marker
+ ids[:, 1] = self.D_marker_token_id
+
+ if bsize:
+ ids, mask, reverse_indices = _sort_by_length(ids, mask, bsize)
+ batches = _split_into_batches(ids, mask, bsize)
+ return batches, reverse_indices
+
+ return ids, mask
diff --git a/PT-Retrieval/colbert/colbert/modeling/tokenization/query_tokenization.py b/PT-Retrieval/colbert/colbert/modeling/tokenization/query_tokenization.py
new file mode 100644
index 0000000..ece4339
--- /dev/null
+++ b/PT-Retrieval/colbert/colbert/modeling/tokenization/query_tokenization.py
@@ -0,0 +1,64 @@
+import torch
+
+from transformers import BertTokenizerFast
+from colbert.modeling.tokenization.utils import _split_into_batches
+
+
+class QueryTokenizer():
+ def __init__(self, query_maxlen):
+ self.tok = BertTokenizerFast.from_pretrained('bert-base-uncased')
+ self.query_maxlen = query_maxlen
+
+ self.Q_marker_token, self.Q_marker_token_id = '[Q]', self.tok.convert_tokens_to_ids('[unused0]')
+ self.cls_token, self.cls_token_id = self.tok.cls_token, self.tok.cls_token_id
+ self.sep_token, self.sep_token_id = self.tok.sep_token, self.tok.sep_token_id
+ self.mask_token, self.mask_token_id = self.tok.mask_token, self.tok.mask_token_id
+
+ assert self.Q_marker_token_id == 1 and self.mask_token_id == 103
+
+ def tokenize(self, batch_text, add_special_tokens=False):
+ assert type(batch_text) in [list, tuple], (type(batch_text))
+
+ tokens = [self.tok.tokenize(x, add_special_tokens=False) for x in batch_text]
+
+ if not add_special_tokens:
+ return tokens
+
+ prefix, suffix = [self.cls_token, self.Q_marker_token], [self.sep_token]
+ tokens = [prefix + lst + suffix + [self.mask_token] * (self.query_maxlen - (len(lst)+3)) for lst in tokens]
+
+ return tokens
+
+ def encode(self, batch_text, add_special_tokens=False):
+ assert type(batch_text) in [list, tuple], (type(batch_text))
+
+ ids = self.tok(batch_text, add_special_tokens=False)['input_ids']
+
+ if not add_special_tokens:
+ return ids
+
+ prefix, suffix = [self.cls_token_id, self.Q_marker_token_id], [self.sep_token_id]
+ ids = [prefix + lst + suffix + [self.mask_token_id] * (self.query_maxlen - (len(lst)+3)) for lst in ids]
+
+ return ids
+
+ def tensorize(self, batch_text, bsize=None):
+ assert type(batch_text) in [list, tuple], (type(batch_text))
+
+ # add placehold for the [Q] marker
+ batch_text = ['. ' + x for x in batch_text]
+
+ obj = self.tok(batch_text, padding='max_length', truncation=True,
+ return_tensors='pt', max_length=self.query_maxlen)
+
+ ids, mask = obj['input_ids'], obj['attention_mask']
+
+ # postprocess for the [Q] marker and the [MASK] augmentation
+ ids[:, 1] = self.Q_marker_token_id
+ ids[ids == 0] = self.mask_token_id
+
+ if bsize:
+ batches = _split_into_batches(ids, mask, bsize)
+ return batches
+
+ return ids, mask
diff --git a/PT-Retrieval/colbert/colbert/modeling/tokenization/utils.py b/PT-Retrieval/colbert/colbert/modeling/tokenization/utils.py
new file mode 100644
index 0000000..5a9e665
--- /dev/null
+++ b/PT-Retrieval/colbert/colbert/modeling/tokenization/utils.py
@@ -0,0 +1,51 @@
+import torch
+
+
+def tensorize_triples(query_tokenizer, doc_tokenizer, queries, positives, negatives, bsize):
+ assert len(queries) == len(positives) == len(negatives)
+ assert bsize is None or len(queries) % bsize == 0
+
+ N = len(queries)
+ Q_ids, Q_mask = query_tokenizer.tensorize(queries)
+ D_ids, D_mask = doc_tokenizer.tensorize(positives + negatives)
+ D_ids, D_mask = D_ids.view(2, N, -1), D_mask.view(2, N, -1)
+
+ # Compute max among {length of i^th positive, length of i^th negative} for i \in N
+ maxlens = D_mask.sum(-1).max(0).values
+
+ # Sort by maxlens
+ indices = maxlens.sort().indices
+ Q_ids, Q_mask = Q_ids[indices], Q_mask[indices]
+ D_ids, D_mask = D_ids[:, indices], D_mask[:, indices]
+
+ (positive_ids, negative_ids), (positive_mask, negative_mask) = D_ids, D_mask
+
+ query_batches = _split_into_batches(Q_ids, Q_mask, bsize)
+ positive_batches = _split_into_batches(positive_ids, positive_mask, bsize)
+ negative_batches = _split_into_batches(negative_ids, negative_mask, bsize)
+
+ batches = []
+ for (q_ids, q_mask), (p_ids, p_mask), (n_ids, n_mask) in zip(query_batches, positive_batches, negative_batches):
+ Q = (torch.cat((q_ids, q_ids)), torch.cat((q_mask, q_mask)))
+ D = (torch.cat((p_ids, n_ids)), torch.cat((p_mask, n_mask)))
+ batches.append((Q, D))
+
+ return batches
+
+
+def _sort_by_length(ids, mask, bsize):
+ if ids.size(0) <= bsize:
+ return ids, mask, torch.arange(ids.size(0))
+
+ indices = mask.sum(-1).sort().indices
+ reverse_indices = indices.sort().indices
+
+ return ids[indices], mask[indices], reverse_indices
+
+
+def _split_into_batches(ids, mask, bsize):
+ batches = []
+ for offset in range(0, ids.size(0), bsize):
+ batches.append((ids[offset:offset+bsize], mask[offset:offset+bsize]))
+
+ return batches
diff --git a/PT-Retrieval/colbert/colbert/parameters.py b/PT-Retrieval/colbert/colbert/parameters.py
new file mode 100644
index 0000000..60ae0a7
--- /dev/null
+++ b/PT-Retrieval/colbert/colbert/parameters.py
@@ -0,0 +1,9 @@
+import torch
+
+DEVICE = torch.device("cuda")
+
+SAVED_CHECKPOINTS = [32*1000, 100*1000, 150*1000, 200*1000, 300*1000, 400*1000]
+SAVED_CHECKPOINTS += [10*1000, 20*1000, 30*1000, 40*1000, 50*1000, 60*1000, 70*1000, 80*1000, 90*1000]
+SAVED_CHECKPOINTS += [25*1000, 50*1000, 75*1000]
+
+SAVED_CHECKPOINTS = set(SAVED_CHECKPOINTS)
diff --git a/PT-Retrieval/colbert/colbert/ranking/__init__.py b/PT-Retrieval/colbert/colbert/ranking/__init__.py
new file mode 100644
index 0000000..e69de29
diff --git a/PT-Retrieval/colbert/colbert/ranking/batch_reranking.py b/PT-Retrieval/colbert/colbert/ranking/batch_reranking.py
new file mode 100644
index 0000000..1987829
--- /dev/null
+++ b/PT-Retrieval/colbert/colbert/ranking/batch_reranking.py
@@ -0,0 +1,131 @@
+import os
+import time
+import torch
+import queue
+import threading
+
+from collections import defaultdict
+
+from colbert.utils.runs import Run
+from colbert.modeling.inference import ModelInference
+from colbert.evaluation.ranking_logger import RankingLogger
+
+from colbert.utils.utils import print_message, flatten, zipstar
+from colbert.indexing.loaders import get_parts
+from colbert.ranking.index_part import IndexPart
+
+MAX_DEPTH_LOGGED = 1000 # TODO: Use args.depth
+
+
+def prepare_ranges(index_path, dim, step, part_range):
+ print_message("#> Launching a separate thread to load index parts asynchronously.")
+ parts, _, _ = get_parts(index_path)
+
+ positions = [(offset, offset + step) for offset in range(0, len(parts), step)]
+
+ if part_range is not None:
+ positions = positions[part_range.start: part_range.stop]
+
+ loaded_parts = queue.Queue(maxsize=2)
+
+ def _loader_thread(index_path, dim, positions):
+ for offset, endpos in positions:
+ index = IndexPart(index_path, dim=dim, part_range=range(offset, endpos), verbose=True)
+ loaded_parts.put(index, block=True)
+
+ thread = threading.Thread(target=_loader_thread, args=(index_path, dim, positions,))
+ thread.start()
+
+ return positions, loaded_parts, thread
+
+
+def score_by_range(positions, loaded_parts, all_query_embeddings, all_query_rankings, all_pids):
+ print_message("#> Sorting by PID..")
+ all_query_indexes, all_pids = zipstar(all_pids)
+ sorting_pids = torch.tensor(all_pids).sort()
+ all_query_indexes, all_pids = torch.tensor(all_query_indexes)[sorting_pids.indices], sorting_pids.values
+
+ range_start, range_end = 0, 0
+
+ for offset, endpos in positions:
+ print_message(f"#> Fetching parts {offset}--{endpos} from queue..")
+ index = loaded_parts.get()
+
+ print_message(f"#> Filtering PIDs to the range {index.pids_range}..")
+ range_start = range_start + (all_pids[range_start:] < index.pids_range.start).sum()
+ range_end = range_end + (all_pids[range_end:] < index.pids_range.stop).sum()
+
+ pids = all_pids[range_start:range_end]
+ query_indexes = all_query_indexes[range_start:range_end]
+
+ print_message(f"#> Got {len(pids)} query--passage pairs in this range.")
+
+ if len(pids) == 0:
+ continue
+
+ print_message(f"#> Ranking in batches the pairs #{range_start} through #{range_end}...")
+ scores = index.batch_rank(all_query_embeddings, query_indexes, pids, sorted_pids=True)
+
+ for query_index, pid, score in zip(query_indexes.tolist(), pids.tolist(), scores):
+ all_query_rankings[0][query_index].append(pid)
+ all_query_rankings[1][query_index].append(score)
+
+
+def batch_rerank(args):
+ positions, loaded_parts, thread = prepare_ranges(args.index_path, args.dim, args.step, args.part_range)
+
+ inference = ModelInference(args.colbert, amp=args.amp)
+ queries, topK_pids = args.queries, args.topK_pids
+
+ with torch.no_grad():
+ queries_in_order = list(queries.values())
+
+ print_message(f"#> Encoding all {len(queries_in_order)} queries in batches...")
+
+ all_query_embeddings = inference.queryFromText(queries_in_order, bsize=512, to_cpu=True)
+ all_query_embeddings = all_query_embeddings.to(dtype=torch.float16).permute(0, 2, 1).contiguous()
+
+ for qid in queries:
+ """
+ Since topK_pids is a defaultdict, make sure each qid *has* actual PID information (even if empty).
+ """
+ assert qid in topK_pids, qid
+
+ all_pids = flatten([[(query_index, pid) for pid in topK_pids[qid]] for query_index, qid in enumerate(queries)])
+ all_query_rankings = [defaultdict(list), defaultdict(list)]
+
+ print_message(f"#> Will process {len(all_pids)} query--document pairs in total.")
+
+ with torch.no_grad():
+ score_by_range(positions, loaded_parts, all_query_embeddings, all_query_rankings, all_pids)
+
+ ranking_logger = RankingLogger(Run.path, qrels=None, log_scores=args.log_scores)
+
+ with ranking_logger.context('ranking.tsv', also_save_annotations=False) as rlogger:
+ with torch.no_grad():
+ for query_index, qid in enumerate(queries):
+ if query_index % 1000 == 0:
+ print_message("#> Logging query #{} (qid {}) now...".format(query_index, qid))
+
+ pids = all_query_rankings[0][query_index]
+ scores = all_query_rankings[1][query_index]
+
+ K = min(MAX_DEPTH_LOGGED, len(scores))
+
+ if K == 0:
+ continue
+
+ scores_topk = torch.tensor(scores).topk(K, largest=True, sorted=True)
+
+ pids, scores = torch.tensor(pids)[scores_topk.indices].tolist(), scores_topk.values.tolist()
+
+ ranking = [(score, pid, None) for pid, score in zip(pids, scores)]
+ assert len(ranking) <= MAX_DEPTH_LOGGED, (len(ranking), MAX_DEPTH_LOGGED)
+
+ rlogger.log(qid, ranking, is_ranked=True, print_positions=[1, 2] if query_index % 100 == 0 else [])
+
+ print('\n\n')
+ print(ranking_logger.filename)
+ print_message('#> Done.\n')
+
+ thread.join()
diff --git a/PT-Retrieval/colbert/colbert/ranking/batch_retrieval.py b/PT-Retrieval/colbert/colbert/ranking/batch_retrieval.py
new file mode 100644
index 0000000..b288f73
--- /dev/null
+++ b/PT-Retrieval/colbert/colbert/ranking/batch_retrieval.py
@@ -0,0 +1,50 @@
+import os
+import time
+import faiss
+import random
+import torch
+
+from colbert.utils.runs import Run
+from multiprocessing import Pool
+from colbert.modeling.inference import ModelInference
+from colbert.evaluation.ranking_logger import RankingLogger
+
+from colbert.utils.utils import print_message, batch
+from colbert.ranking.faiss_index import FaissIndex
+
+
+def batch_retrieve(args):
+ assert args.retrieve_only, "TODO: Combine batch (multi-query) retrieval with batch re-ranking"
+
+ faiss_index = FaissIndex(args.index_path, args.faiss_index_path, args.nprobe, args.part_range)
+ inference = ModelInference(args.colbert, amp=args.amp)
+
+ ranking_logger = RankingLogger(Run.path, qrels=None)
+
+ with ranking_logger.context('unordered.tsv', also_save_annotations=False) as rlogger:
+ queries = args.queries
+ qids_in_order = list(queries.keys())
+
+ for qoffset, qbatch in batch(qids_in_order, 100_000, provide_offset=True):
+ qbatch_text = [queries[qid] for qid in qbatch]
+
+ print_message(f"#> Embedding {len(qbatch_text)} queries in parallel...")
+ Q = inference.queryFromText(qbatch_text, bsize=512)
+
+ print_message("#> Starting batch retrieval...")
+ all_pids = faiss_index.retrieve(args.faiss_depth, Q, verbose=True)
+
+ # Log the PIDs with rank -1 for all
+ for query_idx, (qid, ranking) in enumerate(zip(qbatch, all_pids)):
+ query_idx = qoffset + query_idx
+
+ if query_idx % 1000 == 0:
+ print_message(f"#> Logging query #{query_idx} (qid {qid}) now...")
+
+ ranking = [(None, pid, None) for pid in ranking]
+ rlogger.log(qid, ranking, is_ranked=False)
+
+ print('\n\n')
+ print(ranking_logger.filename)
+ print("#> Done.")
+ print('\n\n')
diff --git a/PT-Retrieval/colbert/colbert/ranking/faiss_index.py b/PT-Retrieval/colbert/colbert/ranking/faiss_index.py
new file mode 100644
index 0000000..a43b667
--- /dev/null
+++ b/PT-Retrieval/colbert/colbert/ranking/faiss_index.py
@@ -0,0 +1,122 @@
+import os
+import time
+import faiss
+import random
+import torch
+
+from multiprocessing import Pool
+from colbert.modeling.inference import ModelInference
+
+from colbert.utils.utils import print_message, flatten, batch
+from colbert.indexing.loaders import load_doclens
+
+
+class FaissIndex():
+ def __init__(self, index_path, faiss_index_path, nprobe, part_range=None):
+ print_message("#> Loading the FAISS index from", faiss_index_path, "..")
+
+ faiss_part_range = os.path.basename(faiss_index_path).split('.')[-2].split('-')
+
+ if len(faiss_part_range) == 2:
+ faiss_part_range = range(*map(int, faiss_part_range))
+ assert part_range[0] in faiss_part_range, (part_range, faiss_part_range)
+ assert part_range[-1] in faiss_part_range, (part_range, faiss_part_range)
+ else:
+ faiss_part_range = None
+
+ self.part_range = part_range
+ self.faiss_part_range = faiss_part_range
+
+ self.faiss_index = faiss.read_index(faiss_index_path)
+ self.faiss_index.nprobe = nprobe
+
+ print_message("#> Building the emb2pid mapping..")
+ all_doclens = load_doclens(index_path, flatten=False)
+
+ pid_offset = 0
+ if faiss_part_range is not None:
+ print(f"#> Restricting all_doclens to the range {faiss_part_range}.")
+ pid_offset = len(flatten(all_doclens[:faiss_part_range.start]))
+ all_doclens = all_doclens[faiss_part_range.start:faiss_part_range.stop]
+
+ self.relative_range = None
+ if self.part_range is not None:
+ start = self.faiss_part_range.start if self.faiss_part_range is not None else 0
+ a = len(flatten(all_doclens[:self.part_range.start - start]))
+ b = len(flatten(all_doclens[:self.part_range.stop - start]))
+ self.relative_range = range(a, b)
+ print(f"self.relative_range = {self.relative_range}")
+
+ all_doclens = flatten(all_doclens)
+
+ total_num_embeddings = sum(all_doclens)
+ self.emb2pid = torch.zeros(total_num_embeddings, dtype=torch.int)
+
+ offset_doclens = 0
+ for pid, dlength in enumerate(all_doclens):
+ self.emb2pid[offset_doclens: offset_doclens + dlength] = pid_offset + pid
+ offset_doclens += dlength
+
+ print_message("len(self.emb2pid) =", len(self.emb2pid))
+
+ self.parallel_pool = Pool(16)
+
+ def retrieve(self, faiss_depth, Q, verbose=False):
+ embedding_ids = self.queries_to_embedding_ids(faiss_depth, Q, verbose=verbose)
+ pids = self.embedding_ids_to_pids(embedding_ids, verbose=verbose)
+
+ if self.relative_range is not None:
+ pids = [[pid for pid in pids_ if pid in self.relative_range] for pids_ in pids]
+
+ return pids
+
+ def queries_to_embedding_ids(self, faiss_depth, Q, verbose=True):
+ # Flatten into a matrix for the faiss search.
+ num_queries, embeddings_per_query, dim = Q.size()
+ Q_faiss = Q.view(num_queries * embeddings_per_query, dim).cpu().contiguous()
+
+ # Search in large batches with faiss.
+ print_message("#> Search in batches with faiss. \t\t",
+ f"Q.size() = {Q.size()}, Q_faiss.size() = {Q_faiss.size()}",
+ condition=verbose)
+
+ embeddings_ids = []
+ faiss_bsize = embeddings_per_query * 5000
+ for offset in range(0, Q_faiss.size(0), faiss_bsize):
+ endpos = min(offset + faiss_bsize, Q_faiss.size(0))
+
+ print_message("#> Searching from {} to {}...".format(offset, endpos), condition=verbose)
+
+ some_Q_faiss = Q_faiss[offset:endpos].float().numpy()
+ _, some_embedding_ids = self.faiss_index.search(some_Q_faiss, faiss_depth)
+ embeddings_ids.append(torch.from_numpy(some_embedding_ids))
+
+ embedding_ids = torch.cat(embeddings_ids)
+
+ # Reshape to (number of queries, non-unique embedding IDs per query)
+ embedding_ids = embedding_ids.view(num_queries, embeddings_per_query * embedding_ids.size(1))
+
+ return embedding_ids
+
+ def embedding_ids_to_pids(self, embedding_ids, verbose=True):
+ # Find unique PIDs per query.
+ print_message("#> Lookup the PIDs..", condition=verbose)
+ all_pids = self.emb2pid[embedding_ids]
+
+ print_message(f"#> Converting to a list [shape = {all_pids.size()}]..", condition=verbose)
+ all_pids = all_pids.tolist()
+
+ print_message("#> Removing duplicates (in parallel if large enough)..", condition=verbose)
+
+ if len(all_pids) > 5000:
+ all_pids = list(self.parallel_pool.map(uniq, all_pids))
+ else:
+ all_pids = list(map(uniq, all_pids))
+
+ print_message("#> Done with embedding_ids_to_pids().", condition=verbose)
+
+ return all_pids
+
+
+def uniq(l):
+ return list(set(l))
diff --git a/PT-Retrieval/colbert/colbert/ranking/index_part.py b/PT-Retrieval/colbert/colbert/ranking/index_part.py
new file mode 100644
index 0000000..c10707a
--- /dev/null
+++ b/PT-Retrieval/colbert/colbert/ranking/index_part.py
@@ -0,0 +1,82 @@
+import os
+import torch
+import ujson
+
+from math import ceil
+from itertools import accumulate
+from colbert.utils.utils import print_message, dotdict, flatten
+
+from colbert.indexing.loaders import get_parts, load_doclens
+from colbert.indexing.index_manager import load_index_part
+from colbert.ranking.index_ranker import IndexRanker
+
+
+class IndexPart():
+ def __init__(self, directory, dim=128, part_range=None, verbose=True):
+ first_part, last_part = (0, None) if part_range is None else (part_range.start, part_range.stop)
+
+ # Load parts metadata
+ all_parts, all_parts_paths, _ = get_parts(directory)
+ self.parts = all_parts[first_part:last_part]
+ self.parts_paths = all_parts_paths[first_part:last_part]
+
+ # Load doclens metadata
+ all_doclens = load_doclens(directory, flatten=False)
+
+ self.doc_offset = sum([len(part_doclens) for part_doclens in all_doclens[:first_part]])
+ self.doc_endpos = sum([len(part_doclens) for part_doclens in all_doclens[:last_part]])
+ self.pids_range = range(self.doc_offset, self.doc_endpos)
+
+ self.parts_doclens = all_doclens[first_part:last_part]
+ self.doclens = flatten(self.parts_doclens)
+ self.num_embeddings = sum(self.doclens)
+
+ self.tensor = self._load_parts(dim, verbose)
+ self.ranker = IndexRanker(self.tensor, self.doclens)
+
+ def _load_parts(self, dim, verbose):
+ tensor = torch.zeros(self.num_embeddings + 512, dim, dtype=torch.float16)
+
+ if verbose:
+ print_message("tensor.size() = ", tensor.size())
+
+ offset = 0
+ for idx, filename in enumerate(self.parts_paths):
+ print_message("|> Loading", filename, "...", condition=verbose)
+
+ endpos = offset + sum(self.parts_doclens[idx])
+ part = load_index_part(filename, verbose=verbose)
+
+ tensor[offset:endpos] = part
+ offset = endpos
+
+ return tensor
+
+ def pid_in_range(self, pid):
+ return pid in self.pids_range
+
+ def rank(self, Q, pids):
+ """
+ Rank a single batch of Q x pids (e.g., 1k--10k pairs).
+ """
+
+ assert Q.size(0) in [1, len(pids)], (Q.size(0), len(pids))
+ assert all(pid in self.pids_range for pid in pids), self.pids_range
+
+ pids_ = [pid - self.doc_offset for pid in pids]
+ scores = self.ranker.rank(Q, pids_)
+
+ return scores
+
+ def batch_rank(self, all_query_embeddings, query_indexes, pids, sorted_pids):
+ """
+ Rank a large, fairly dense set of query--passage pairs (e.g., 1M+ pairs).
+ Higher overhead, much faster for large batches.
+ """
+
+ assert ((pids >= self.pids_range.start) & (pids < self.pids_range.stop)).sum() == pids.size(0)
+
+ pids_ = pids - self.doc_offset
+ scores = self.ranker.batch_rank(all_query_embeddings, query_indexes, pids_, sorted_pids)
+
+ return scores
diff --git a/PT-Retrieval/colbert/colbert/ranking/index_ranker.py b/PT-Retrieval/colbert/colbert/ranking/index_ranker.py
new file mode 100644
index 0000000..3b11aad
--- /dev/null
+++ b/PT-Retrieval/colbert/colbert/ranking/index_ranker.py
@@ -0,0 +1,164 @@
+import os
+import math
+import torch
+import ujson
+import traceback
+
+from itertools import accumulate
+from colbert.parameters import DEVICE
+from colbert.utils.utils import print_message, dotdict, flatten
+
+BSIZE = 1 << 14
+
+
+class IndexRanker():
+ def __init__(self, tensor, doclens):
+ self.tensor = tensor
+ self.doclens = doclens
+
+ self.maxsim_dtype = torch.float32
+ self.doclens_pfxsum = [0] + list(accumulate(self.doclens))
+
+ self.doclens = torch.tensor(self.doclens)
+ self.doclens_pfxsum = torch.tensor(self.doclens_pfxsum)
+
+ self.dim = self.tensor.size(-1)
+
+ self.strides = [torch_percentile(self.doclens, p) for p in [90]]
+ self.strides.append(self.doclens.max().item())
+ self.strides = sorted(list(set(self.strides)))
+
+ print_message(f"#> Using strides {self.strides}..")
+
+ self.views = self._create_views(self.tensor)
+ self.buffers = self._create_buffers(BSIZE, self.tensor.dtype, {'cpu', 'cuda:0'})
+
+ def _create_views(self, tensor):
+ views = []
+
+ for stride in self.strides:
+ outdim = tensor.size(0) - stride + 1
+ view = torch.as_strided(tensor, (outdim, stride, self.dim), (self.dim, self.dim, 1))
+ views.append(view)
+
+ return views
+
+ def _create_buffers(self, max_bsize, dtype, devices):
+ buffers = {}
+
+ for device in devices:
+ buffers[device] = [torch.zeros(max_bsize, stride, self.dim, dtype=dtype,
+ device=device, pin_memory=(device == 'cpu'))
+ for stride in self.strides]
+
+ return buffers
+
+ def rank(self, Q, pids, views=None, shift=0):
+ assert len(pids) > 0
+ assert Q.size(0) in [1, len(pids)]
+
+ Q = Q.contiguous().to(DEVICE).to(dtype=self.maxsim_dtype)
+
+ views = self.views if views is None else views
+ VIEWS_DEVICE = views[0].device
+
+ D_buffers = self.buffers[str(VIEWS_DEVICE)]
+
+ raw_pids = pids if type(pids) is list else pids.tolist()
+ pids = torch.tensor(pids) if type(pids) is list else pids
+
+ doclens, offsets = self.doclens[pids], self.doclens_pfxsum[pids]
+
+ assignments = (doclens.unsqueeze(1) > torch.tensor(self.strides).unsqueeze(0) + 1e-6).sum(-1)
+
+ one_to_n = torch.arange(len(raw_pids))
+ output_pids, output_scores, output_permutation = [], [], []
+
+ for group_idx, stride in enumerate(self.strides):
+ locator = (assignments == group_idx)
+
+ if locator.sum() < 1e-5:
+ continue
+
+ group_pids, group_doclens, group_offsets = pids[locator], doclens[locator], offsets[locator]
+ group_Q = Q if Q.size(0) == 1 else Q[locator]
+
+ group_offsets = group_offsets.to(VIEWS_DEVICE) - shift
+ group_offsets_uniq, group_offsets_expand = torch.unique_consecutive(group_offsets, return_inverse=True)
+
+ D_size = group_offsets_uniq.size(0)
+ D = torch.index_select(views[group_idx], 0, group_offsets_uniq, out=D_buffers[group_idx][:D_size])
+ D = D.to(DEVICE)
+ D = D[group_offsets_expand.to(DEVICE)].to(dtype=self.maxsim_dtype)
+
+ mask = torch.arange(stride, device=DEVICE) + 1
+ mask = mask.unsqueeze(0) <= group_doclens.to(DEVICE).unsqueeze(-1)
+
+ scores = (D @ group_Q) * mask.unsqueeze(-1)
+ scores = scores.max(1).values.sum(-1).cpu()
+
+ output_pids.append(group_pids)
+ output_scores.append(scores)
+ output_permutation.append(one_to_n[locator])
+
+ output_permutation = torch.cat(output_permutation).sort().indices
+ output_pids = torch.cat(output_pids)[output_permutation].tolist()
+ output_scores = torch.cat(output_scores)[output_permutation].tolist()
+
+ assert len(raw_pids) == len(output_pids)
+ assert len(raw_pids) == len(output_scores)
+ assert raw_pids == output_pids
+
+ return output_scores
+
+ def batch_rank(self, all_query_embeddings, all_query_indexes, all_pids, sorted_pids):
+ assert sorted_pids is True
+
+ ######
+
+ scores = []
+ range_start, range_end = 0, 0
+
+ for pid_offset in range(0, len(self.doclens), 50_000):
+ pid_endpos = min(pid_offset + 50_000, len(self.doclens))
+
+ range_start = range_start + (all_pids[range_start:] < pid_offset).sum()
+ range_end = range_end + (all_pids[range_end:] < pid_endpos).sum()
+
+ pids = all_pids[range_start:range_end]
+ query_indexes = all_query_indexes[range_start:range_end]
+
+ print_message(f"###--> Got {len(pids)} query--passage pairs in this sub-range {(pid_offset, pid_endpos)}.")
+
+ if len(pids) == 0:
+ continue
+
+ print_message(f"###--> Ranking in batches the pairs #{range_start} through #{range_end} in this sub-range.")
+
+ tensor_offset = self.doclens_pfxsum[pid_offset].item()
+ tensor_endpos = self.doclens_pfxsum[pid_endpos].item() + 512
+
+ collection = self.tensor[tensor_offset:tensor_endpos].to(DEVICE)
+ views = self._create_views(collection)
+
+ print_message(f"#> Ranking in batches of {BSIZE} query--passage pairs...")
+
+ for batch_idx, offset in enumerate(range(0, len(pids), BSIZE)):
+ if batch_idx % 100 == 0:
+ print_message("#> Processing batch #{}..".format(batch_idx))
+
+ endpos = offset + BSIZE
+ batch_query_index, batch_pids = query_indexes[offset:endpos], pids[offset:endpos]
+
+ Q = all_query_embeddings[batch_query_index]
+
+ scores.extend(self.rank(Q, batch_pids, views, shift=tensor_offset))
+
+ return scores
+
+
+def torch_percentile(tensor, p):
+ assert p in range(1, 100+1)
+ assert tensor.dim() == 1
+
+ return tensor.kthvalue(int(p * tensor.size(0) / 100.0)).values.item()
diff --git a/PT-Retrieval/colbert/colbert/ranking/rankers.py b/PT-Retrieval/colbert/colbert/ranking/rankers.py
new file mode 100644
index 0000000..320dc13
--- /dev/null
+++ b/PT-Retrieval/colbert/colbert/ranking/rankers.py
@@ -0,0 +1,43 @@
+import torch
+
+from functools import partial
+
+from colbert.ranking.index_part import IndexPart
+from colbert.ranking.faiss_index import FaissIndex
+from colbert.utils.utils import flatten, zipstar
+
+
+class Ranker():
+ def __init__(self, args, inference, faiss_depth=1024):
+ self.inference = inference
+ self.faiss_depth = faiss_depth
+
+ if faiss_depth is not None:
+ self.faiss_index = FaissIndex(args.index_path, args.faiss_index_path, args.nprobe, part_range=args.part_range)
+ self.retrieve = partial(self.faiss_index.retrieve, self.faiss_depth)
+
+ self.index = IndexPart(args.index_path, dim=inference.colbert.dim, part_range=args.part_range, verbose=True)
+
+ def encode(self, queries):
+ assert type(queries) in [list, tuple], type(queries)
+
+ Q = self.inference.queryFromText(queries, bsize=512 if len(queries) > 512 else None)
+
+ return Q
+
+ def rank(self, Q, pids=None):
+ pids = self.retrieve(Q, verbose=False)[0] if pids is None else pids
+
+ assert type(pids) in [list, tuple], type(pids)
+ assert Q.size(0) == 1, (len(pids), Q.size())
+ assert all(type(pid) is int for pid in pids)
+
+ scores = []
+ if len(pids) > 0:
+ Q = Q.permute(0, 2, 1)
+ scores = self.index.rank(Q, pids)
+
+ scores_sorter = torch.tensor(scores).sort(descending=True)
+ pids, scores = torch.tensor(pids)[scores_sorter.indices].tolist(), scores_sorter.values.tolist()
+
+ return pids, scores
diff --git a/PT-Retrieval/colbert/colbert/ranking/reranking.py b/PT-Retrieval/colbert/colbert/ranking/reranking.py
new file mode 100644
index 0000000..b6f0dff
--- /dev/null
+++ b/PT-Retrieval/colbert/colbert/ranking/reranking.py
@@ -0,0 +1,61 @@
+import os
+import time
+import faiss
+import random
+import torch
+
+from colbert.utils.runs import Run
+from multiprocessing import Pool
+from colbert.modeling.inference import ModelInference
+from colbert.evaluation.ranking_logger import RankingLogger
+
+from colbert.utils.utils import print_message, batch
+from colbert.ranking.rankers import Ranker
+
+
+def rerank(args):
+ inference = ModelInference(args.colbert, amp=args.amp)
+ ranker = Ranker(args, inference, faiss_depth=None)
+
+ ranking_logger = RankingLogger(Run.path, qrels=None)
+ milliseconds = 0
+
+ with ranking_logger.context('ranking.tsv', also_save_annotations=False) as rlogger:
+ queries = args.queries
+ qids_in_order = list(queries.keys())
+
+ for qoffset, qbatch in batch(qids_in_order, 100, provide_offset=True):
+ qbatch_text = [queries[qid] for qid in qbatch]
+ qbatch_pids = [args.topK_pids[qid] for qid in qbatch]
+
+ rankings = []
+
+ for query_idx, (q, pids) in enumerate(zip(qbatch_text, qbatch_pids)):
+ torch.cuda.synchronize('cuda:0')
+ s = time.time()
+
+ Q = ranker.encode([q])
+ pids, scores = ranker.rank(Q, pids=pids)
+
+ torch.cuda.synchronize()
+ milliseconds += (time.time() - s) * 1000.0
+
+ if len(pids):
+ print(qoffset+query_idx, q, len(scores), len(pids), scores[0], pids[0],
+ milliseconds / (qoffset+query_idx+1), 'ms')
+
+ rankings.append(zip(pids, scores))
+
+ for query_idx, (qid, ranking) in enumerate(zip(qbatch, rankings)):
+ query_idx = qoffset + query_idx
+
+ if query_idx % 100 == 0:
+ print_message(f"#> Logging query #{query_idx} (qid {qid}) now...")
+
+ ranking = [(score, pid, None) for pid, score in ranking]
+ rlogger.log(qid, ranking, is_ranked=True)
+
+ print('\n\n')
+ print(ranking_logger.filename)
+ print("#> Done.")
+ print('\n\n')
diff --git a/PT-Retrieval/colbert/colbert/ranking/retrieval.py b/PT-Retrieval/colbert/colbert/ranking/retrieval.py
new file mode 100644
index 0000000..de88358
--- /dev/null
+++ b/PT-Retrieval/colbert/colbert/ranking/retrieval.py
@@ -0,0 +1,66 @@
+import os
+import time
+import faiss
+import random
+import torch
+import itertools
+import shutil
+
+from colbert.utils.runs import Run
+from multiprocessing import Pool
+from colbert.modeling.inference import ModelInference
+from colbert.evaluation.ranking_logger import RankingLogger
+
+from colbert.utils.utils import print_message, batch
+from colbert.ranking.rankers import Ranker
+
+
+def retrieve(args):
+ inference = ModelInference(args.colbert, amp=args.amp)
+ ranker = Ranker(args, inference, faiss_depth=args.faiss_depth)
+
+ ranking_logger = RankingLogger(Run.path, qrels=None)
+ milliseconds = 0
+
+ with ranking_logger.context('ranking.tsv', also_save_annotations=False) as rlogger:
+ queries = args.queries
+ qids_in_order = list(queries.keys())
+
+ for qoffset, qbatch in batch(qids_in_order, 100, provide_offset=True):
+ qbatch_text = [queries[qid] for qid in qbatch]
+
+ rankings = []
+
+ for query_idx, q in enumerate(qbatch_text):
+ torch.cuda.synchronize('cuda:0')
+ s = time.time()
+
+ Q = ranker.encode([q])
+ pids, scores = ranker.rank(Q)
+
+ torch.cuda.synchronize()
+ milliseconds += (time.time() - s) * 1000.0
+
+ if len(pids):
+ print(qoffset+query_idx, q, len(scores), len(pids), scores[0], pids[0],
+ milliseconds / (qoffset+query_idx+1), 'ms')
+
+ rankings.append(zip(pids, scores))
+
+ for query_idx, (qid, ranking) in enumerate(zip(qbatch, rankings)):
+ query_idx = qoffset + query_idx
+
+ if query_idx % 100 == 0:
+ print_message(f"#> Logging query #{query_idx} (qid {qid}) now...")
+
+ ranking = [(score, pid, None) for pid, score in itertools.islice(ranking, args.depth)]
+ rlogger.log(qid, ranking, is_ranked=True)
+
+ print('\n\n')
+ print(ranking_logger.filename)
+ if args.ranking_dir:
+ os.makedirs(args.ranking_dir, exist_ok=True)
+ shutil.copyfile(ranking_logger.filename, os.path.join(args.ranking_dir, "ranking.tsv"))
+ print("#> Copied to {}".format(os.path.join(args.ranking_dir, "ranking.tsv")))
+ print("#> Done.")
+ print('\n\n')
diff --git a/PT-Retrieval/colbert/colbert/rerank.py b/PT-Retrieval/colbert/colbert/rerank.py
new file mode 100644
index 0000000..852f6f3
--- /dev/null
+++ b/PT-Retrieval/colbert/colbert/rerank.py
@@ -0,0 +1,50 @@
+import os
+import random
+
+from colbert.utils.parser import Arguments
+from colbert.utils.runs import Run
+
+from colbert.evaluation.loaders import load_colbert, load_qrels, load_queries, load_topK_pids
+from colbert.ranking.reranking import rerank
+from colbert.ranking.batch_reranking import batch_rerank
+
+
+def main():
+ random.seed(12345)
+
+ parser = Arguments(description='Re-ranking over a ColBERT index')
+
+ parser.add_model_parameters()
+ parser.add_model_inference_parameters()
+ parser.add_reranking_input()
+ parser.add_index_use_input()
+
+ parser.add_argument('--step', dest='step', default=1, type=int)
+ parser.add_argument('--part-range', dest='part_range', default=None, type=str)
+ parser.add_argument('--log-scores', dest='log_scores', default=False, action='store_true')
+ parser.add_argument('--batch', dest='batch', default=False, action='store_true')
+ parser.add_argument('--depth', dest='depth', default=1000, type=int)
+
+ args = parser.parse()
+
+ if args.part_range:
+ part_offset, part_endpos = map(int, args.part_range.split('..'))
+ args.part_range = range(part_offset, part_endpos)
+
+ with Run.context():
+ args.colbert, args.checkpoint = load_colbert(args)
+
+ args.queries = load_queries(args.queries)
+ args.qrels = load_qrels(args.qrels)
+ args.topK_pids, args.qrels = load_topK_pids(args.topK, qrels=args.qrels)
+
+ args.index_path = os.path.join(args.index_root, args.index_name)
+
+ if args.batch:
+ batch_rerank(args)
+ else:
+ rerank(args)
+
+
+if __name__ == "__main__":
+ main()
diff --git a/PT-Retrieval/colbert/colbert/retrieve.py b/PT-Retrieval/colbert/colbert/retrieve.py
new file mode 100644
index 0000000..9af6e87
--- /dev/null
+++ b/PT-Retrieval/colbert/colbert/retrieve.py
@@ -0,0 +1,57 @@
+import os
+import random
+
+from colbert.utils.parser import Arguments
+from colbert.utils.runs import Run
+
+from colbert.evaluation.loaders import load_colbert, load_qrels, load_queries
+from colbert.indexing.faiss import get_faiss_index_name
+from colbert.ranking.retrieval import retrieve
+from colbert.ranking.batch_retrieval import batch_retrieve
+
+
+def main():
+ random.seed(12345)
+
+ parser = Arguments(description='End-to-end retrieval and ranking with ColBERT.')
+
+ parser.add_model_parameters()
+ parser.add_model_inference_parameters()
+ parser.add_ranking_input()
+ parser.add_retrieval_input()
+
+ parser.add_argument('--faiss_name', dest='faiss_name', default=None, type=str)
+ parser.add_argument('--faiss_depth', dest='faiss_depth', default=1024, type=int)
+ parser.add_argument('--part-range', dest='part_range', default=None, type=str)
+ parser.add_argument('--batch', dest='batch', default=False, action='store_true')
+ parser.add_argument('--depth', dest='depth', default=1000, type=int)
+ parser.add_argument('--ranking_dir', dest='ranking_dir', default=None, type=str)
+
+ args = parser.parse()
+
+ args.depth = args.depth if args.depth > 0 else None
+
+ if args.part_range:
+ part_offset, part_endpos = map(int, args.part_range.split('..'))
+ args.part_range = range(part_offset, part_endpos)
+
+ with Run.context():
+ args.colbert, args.checkpoint = load_colbert(args)
+ args.qrels = load_qrels(args.qrels)
+ args.queries = load_queries(args.queries)
+
+ args.index_path = os.path.join(args.index_root, args.index_name)
+
+ if args.faiss_name is not None:
+ args.faiss_index_path = os.path.join(args.index_path, args.faiss_name)
+ else:
+ args.faiss_index_path = os.path.join(args.index_path, get_faiss_index_name(args))
+
+ if args.batch:
+ batch_retrieve(args)
+ else:
+ retrieve(args)
+
+
+if __name__ == "__main__":
+ main()
diff --git a/PT-Retrieval/colbert/colbert/test.py b/PT-Retrieval/colbert/colbert/test.py
new file mode 100644
index 0000000..5eb870a
--- /dev/null
+++ b/PT-Retrieval/colbert/colbert/test.py
@@ -0,0 +1,49 @@
+import os
+import random
+
+from colbert.utils.parser import Arguments
+from colbert.utils.runs import Run
+
+from colbert.evaluation.loaders import load_colbert, load_topK, load_qrels
+from colbert.evaluation.loaders import load_queries, load_topK_pids, load_collection
+from colbert.evaluation.ranking import evaluate
+from colbert.evaluation.metrics import evaluate_recall
+
+
+def main():
+ random.seed(12345)
+
+ parser = Arguments(description='Exhaustive (slow, not index-based) evaluation of re-ranking with ColBERT.')
+
+ parser.add_model_parameters()
+ parser.add_model_inference_parameters()
+ parser.add_reranking_input()
+
+ parser.add_argument('--depth', dest='depth', required=False, default=None, type=int)
+
+ args = parser.parse()
+
+ with Run.context():
+ args.colbert, args.checkpoint = load_colbert(args)
+ args.qrels = load_qrels(args.qrels)
+
+ if args.collection or args.queries:
+ assert args.collection and args.queries
+
+ args.queries = load_queries(args.queries)
+ args.collection = load_collection(args.collection)
+ args.topK_pids, args.qrels = load_topK_pids(args.topK, args.qrels)
+
+ else:
+ args.queries, args.topK_docs, args.topK_pids = load_topK(args.topK)
+
+ assert (not args.shortcircuit) or args.qrels, \
+ "Short-circuiting (i.e., applying minimal computation to queries with no positives in the re-ranked set) " \
+ "can only be applied if qrels is provided."
+
+ evaluate_recall(args.qrels, args.queries, args.topK_pids)
+ evaluate(args)
+
+
+if __name__ == "__main__":
+ main()
diff --git a/PT-Retrieval/colbert/colbert/train.py b/PT-Retrieval/colbert/colbert/train.py
new file mode 100644
index 0000000..d6cd236
--- /dev/null
+++ b/PT-Retrieval/colbert/colbert/train.py
@@ -0,0 +1,34 @@
+import os
+import random
+import torch
+import copy
+
+import colbert.utils.distributed as distributed
+
+from colbert.utils.parser import Arguments
+from colbert.utils.runs import Run
+from colbert.training.training import train
+
+
+def main():
+ parser = Arguments(description='Training ColBERT with triples.')
+
+ parser.add_model_parameters()
+ parser.add_model_training_parameters()
+ parser.add_training_input()
+
+ args = parser.parse()
+
+ assert args.bsize % args.accumsteps == 0, ((args.bsize, args.accumsteps),
+ "The batch size must be divisible by the number of gradient accumulation steps.")
+ assert args.query_maxlen <= 512
+ assert args.doc_maxlen <= 512
+
+ args.lazy = args.collection is not None
+
+ with Run.context(consider_failed_if_interrupted=False):
+ train(args)
+
+
+if __name__ == "__main__":
+ main()
diff --git a/PT-Retrieval/colbert/colbert/training/__init__.py b/PT-Retrieval/colbert/colbert/training/__init__.py
new file mode 100644
index 0000000..e69de29
diff --git a/PT-Retrieval/colbert/colbert/training/eager_batcher.py b/PT-Retrieval/colbert/colbert/training/eager_batcher.py
new file mode 100644
index 0000000..2edec7a
--- /dev/null
+++ b/PT-Retrieval/colbert/colbert/training/eager_batcher.py
@@ -0,0 +1,62 @@
+import os
+import ujson
+
+from functools import partial
+from colbert.utils.utils import print_message
+from colbert.modeling.tokenization import QueryTokenizer, DocTokenizer, tensorize_triples
+
+from colbert.utils.runs import Run
+
+
+class EagerBatcher():
+ def __init__(self, args, rank=0, nranks=1):
+ self.rank, self.nranks = rank, nranks
+ self.bsize, self.accumsteps = args.bsize, args.accumsteps
+
+ self.query_tokenizer = QueryTokenizer(args.query_maxlen)
+ self.doc_tokenizer = DocTokenizer(args.doc_maxlen)
+ self.tensorize_triples = partial(tensorize_triples, self.query_tokenizer, self.doc_tokenizer)
+
+ self.triples_path = args.triples
+ self._reset_triples()
+
+ def _reset_triples(self):
+ self.reader = open(self.triples_path, mode='r', encoding="utf-8")
+ self.position = 0
+
+ def __iter__(self):
+ return self
+
+ def __next__(self):
+ queries, positives, negatives = [], [], []
+
+ for line_idx, line in zip(range(self.bsize * self.nranks), self.reader):
+ if (self.position + line_idx) % self.nranks != self.rank:
+ continue
+
+ query, pos, neg = line.strip().split('\t')
+
+ queries.append(query)
+ positives.append(pos)
+ negatives.append(neg)
+
+ self.position += line_idx + 1
+
+ if len(queries) < self.bsize:
+ raise StopIteration
+
+ return self.collate(queries, positives, negatives)
+
+ def collate(self, queries, positives, negatives):
+ assert len(queries) == len(positives) == len(negatives) == self.bsize
+
+ return self.tensorize_triples(queries, positives, negatives, self.bsize // self.accumsteps)
+
+ def skip_to_batch(self, batch_idx, intended_batch_size):
+ self._reset_triples()
+
+ Run.warn(f'Skipping to batch #{batch_idx} (with intended_batch_size = {intended_batch_size}) for training.')
+
+ _ = [self.reader.readline() for _ in range(batch_idx * intended_batch_size)]
+
+ return None
diff --git a/PT-Retrieval/colbert/colbert/training/lazy_batcher.py b/PT-Retrieval/colbert/colbert/training/lazy_batcher.py
new file mode 100644
index 0000000..6b0fed7
--- /dev/null
+++ b/PT-Retrieval/colbert/colbert/training/lazy_batcher.py
@@ -0,0 +1,103 @@
+import os
+import ujson
+
+from functools import partial
+from colbert.utils.utils import print_message
+from colbert.modeling.tokenization import QueryTokenizer, DocTokenizer, tensorize_triples
+
+from colbert.utils.runs import Run
+
+
+class LazyBatcher():
+ def __init__(self, args, rank=0, nranks=1):
+ self.bsize, self.accumsteps = args.bsize, args.accumsteps
+
+ self.query_tokenizer = QueryTokenizer(args.query_maxlen)
+ self.doc_tokenizer = DocTokenizer(args.doc_maxlen)
+ self.tensorize_triples = partial(tensorize_triples, self.query_tokenizer, self.doc_tokenizer)
+ self.position = 0
+
+ self.triples = self._load_triples(args.triples, rank, nranks)
+ self.queries = self._load_queries(args.queries)
+ self.collection = self._load_collection(args.collection)
+
+ def _load_triples(self, path, rank, nranks):
+ """
+ NOTE: For distributed sampling, this isn't equivalent to perfectly uniform sampling.
+ In particular, each subset is perfectly represented in every batch! However, since we never
+ repeat passes over the data, we never repeat any particular triple, and the split across
+ nodes is random (since the underlying file is pre-shuffled), there's no concern here.
+ """
+ print_message("#> Loading triples...")
+
+ triples = []
+
+ with open(path) as f:
+ for line_idx, line in enumerate(f):
+ if line_idx % nranks == rank:
+ qid, pos, neg = ujson.loads(line)
+ triples.append((qid, pos, neg))
+
+ return triples
+
+ def _load_queries(self, path):
+ print_message("#> Loading queries...")
+
+ queries = {}
+
+ with open(path) as f:
+ for line in f:
+ qid, query = line.strip().split('\t')
+ qid = int(qid)
+ queries[qid] = query
+
+ return queries
+
+ def _load_collection(self, path):
+ print_message("#> Loading collection...")
+
+ collection = []
+
+ with open(path) as f:
+ for line_idx, line in enumerate(f):
+ pid, passage, title, *_ = line.strip().split('\t')
+ assert pid == 'id' or int(pid) == line_idx
+
+ passage = title + ' | ' + passage
+ collection.append(passage)
+
+ return collection
+
+ def __iter__(self):
+ return self
+
+ def __len__(self):
+ return len(self.triples)
+
+ def __next__(self):
+ offset, endpos = self.position, min(self.position + self.bsize, len(self.triples))
+ self.position = endpos
+
+ if offset + self.bsize > len(self.triples):
+ raise StopIteration
+
+ queries, positives, negatives = [], [], []
+
+ for position in range(offset, endpos):
+ query, pos, neg = self.triples[position]
+ query, pos, neg = self.queries[query], self.collection[pos], self.collection[neg]
+
+ queries.append(query)
+ positives.append(pos)
+ negatives.append(neg)
+
+ return self.collate(queries, positives, negatives)
+
+ def collate(self, queries, positives, negatives):
+ assert len(queries) == len(positives) == len(negatives) == self.bsize
+
+ return self.tensorize_triples(queries, positives, negatives, self.bsize // self.accumsteps)
+
+ def skip_to_batch(self, batch_idx, intended_batch_size):
+ Run.warn(f'Skipping to batch #{batch_idx} (with intended_batch_size = {intended_batch_size}) for training.')
+ self.position = intended_batch_size * batch_idx
diff --git a/PT-Retrieval/colbert/colbert/training/training.py b/PT-Retrieval/colbert/colbert/training/training.py
new file mode 100644
index 0000000..2be721e
--- /dev/null
+++ b/PT-Retrieval/colbert/colbert/training/training.py
@@ -0,0 +1,138 @@
+import os
+import random
+import time
+import torch
+import torch.nn as nn
+import numpy as np
+
+from transformers import AdamW, BertConfig
+from colbert.utils.runs import Run
+from colbert.utils.amp import MixedPrecisionManager
+
+from colbert.training.lazy_batcher import LazyBatcher
+from colbert.training.eager_batcher import EagerBatcher
+from colbert.parameters import DEVICE
+
+from colbert.modeling.colbert import ColBERT
+from colbert.modeling.prefix import PrefixColBERT
+from colbert.utils.utils import print_message
+from colbert.training.utils import print_progress, manage_checkpoints
+
+
+def train(args):
+ random.seed(12345)
+ np.random.seed(12345)
+ torch.manual_seed(12345)
+ if args.distributed:
+ torch.cuda.manual_seed_all(12345)
+
+ if args.distributed:
+ assert args.bsize % args.nranks == 0, (args.bsize, args.nranks)
+ assert args.accumsteps == 1
+ args.bsize = args.bsize // args.nranks
+
+ print("Using args.bsize =", args.bsize, "(per process) and args.accumsteps =", args.accumsteps)
+
+ if args.lazy:
+ reader = LazyBatcher(args, (0 if args.rank == -1 else args.rank), args.nranks)
+ else:
+ reader = EagerBatcher(args, (0 if args.rank == -1 else args.rank), args.nranks)
+
+ if args.rank not in [-1, 0]:
+ torch.distributed.barrier()
+
+ if args.prefix:
+ config = BertConfig.from_pretrained('bert-base-uncased', cache_dir=".cache")
+ config.pre_seq_len = args.pre_seq_len
+ config.prefix_hidden_size = args.prefix_hidden_size
+ config.prefix_mlp = args.prefix_mlp
+ colbert = PrefixColBERT.from_pretrained('bert-base-uncased', config=config,
+ query_maxlen=args.query_maxlen,
+ doc_maxlen=args.doc_maxlen,
+ dim=args.dim,
+ similarity_metric=args.similarity,
+ mask_punctuation=args.mask_punctuation)
+ else:
+ config = BertConfig.from_pretrained('bert-base-uncased', cache_dir=".cache")
+ colbert = ColBERT.from_pretrained('bert-base-uncased',
+ config=config,
+ query_maxlen=args.query_maxlen,
+ doc_maxlen=args.doc_maxlen,
+ dim=args.dim,
+ similarity_metric=args.similarity,
+ mask_punctuation=args.mask_punctuation)
+
+ if args.checkpoint is not None:
+ assert args.resume_optimizer is False, "TODO: This would mean reload optimizer too."
+ print_message(f"#> Starting from checkpoint {args.checkpoint} -- but NOT the optimizer!")
+
+ checkpoint = torch.load(args.checkpoint, map_location='cpu')
+
+ try:
+ colbert.load_state_dict(checkpoint['model_state_dict'])
+ except:
+ print_message("[WARNING] Loading checkpoint with strict=False")
+ colbert.load_state_dict(checkpoint['model_state_dict'], strict=False)
+
+ if args.rank == 0:
+ torch.distributed.barrier()
+
+ colbert = colbert.to(DEVICE)
+ colbert.train()
+
+ if args.distributed:
+ colbert = torch.nn.parallel.DistributedDataParallel(colbert, device_ids=[args.rank],
+ output_device=args.rank,
+ find_unused_parameters=True)
+
+ optimizer = AdamW(filter(lambda p: p.requires_grad, colbert.parameters()), lr=args.lr, eps=1e-8)
+ optimizer.zero_grad()
+
+ amp = MixedPrecisionManager(args.amp)
+ criterion = nn.CrossEntropyLoss()
+ labels = torch.zeros(args.bsize, dtype=torch.long, device=DEVICE)
+
+ start_time = time.time()
+ train_loss = 0.0
+
+ start_batch_idx = 0
+
+ if args.resume:
+ assert args.checkpoint is not None
+ start_batch_idx = checkpoint['batch']
+
+ reader.skip_to_batch(start_batch_idx, checkpoint['arguments']['bsize'])
+
+ for batch_idx, BatchSteps in zip(range(start_batch_idx, args.maxsteps), reader):
+ this_batch_loss = 0.0
+
+ for queries, passages in BatchSteps:
+ with amp.context():
+ scores = colbert(queries, passages).view(2, -1).permute(1, 0)
+ loss = criterion(scores, labels[:scores.size(0)])
+ loss = loss / args.accumsteps
+
+ if args.rank < 1:
+ print_progress(scores)
+
+ amp.backward(loss)
+
+ train_loss += loss.item()
+ this_batch_loss += loss.item()
+
+ amp.step(colbert, optimizer)
+
+ if args.rank < 1:
+ avg_loss = train_loss / (batch_idx+1)
+
+ num_examples_seen = (batch_idx - start_batch_idx) * args.bsize * args.nranks
+ elapsed = float(time.time() - start_time)
+
+ log_to_mlflow = (batch_idx % 20 == 0)
+ Run.log_metric('train/avg_loss', avg_loss, step=batch_idx, log_to_mlflow=log_to_mlflow)
+ Run.log_metric('train/batch_loss', this_batch_loss, step=batch_idx, log_to_mlflow=log_to_mlflow)
+ Run.log_metric('train/examples', num_examples_seen, step=batch_idx, log_to_mlflow=log_to_mlflow)
+ Run.log_metric('train/throughput', num_examples_seen / elapsed, step=batch_idx, log_to_mlflow=log_to_mlflow)
+
+ print_message(batch_idx, avg_loss)
+ manage_checkpoints(args, colbert, optimizer, batch_idx+1)
diff --git a/PT-Retrieval/colbert/colbert/training/utils.py b/PT-Retrieval/colbert/colbert/training/utils.py
new file mode 100644
index 0000000..5ef002c
--- /dev/null
+++ b/PT-Retrieval/colbert/colbert/training/utils.py
@@ -0,0 +1,28 @@
+import os
+import torch
+
+from colbert.utils.runs import Run
+from colbert.utils.utils import print_message, save_checkpoint
+from colbert.parameters import SAVED_CHECKPOINTS
+
+
+def print_progress(scores):
+ positive_avg, negative_avg = round(scores[:, 0].mean().item(), 2), round(scores[:, 1].mean().item(), 2)
+ print("#>>> ", positive_avg, negative_avg, '\t\t|\t\t', positive_avg - negative_avg)
+
+
+def manage_checkpoints(args, colbert, optimizer, batch_idx):
+ arguments = args.input_arguments.__dict__
+
+ path = os.path.join(Run.path, 'checkpoints')
+
+ if not os.path.exists(path):
+ os.mkdir(path)
+
+ if batch_idx % 2000 == 0:
+ name = os.path.join(path, "colbert.dnn")
+ save_checkpoint(name, 0, batch_idx, colbert, optimizer, arguments)
+
+ if batch_idx in SAVED_CHECKPOINTS or batch_idx % 10000 == 0 or batch_idx==30:
+ name = os.path.join(path, "colbert-{}.dnn".format(batch_idx))
+ save_checkpoint(name, 0, batch_idx, colbert, optimizer, arguments, args.prefix)
diff --git a/PT-Retrieval/colbert/colbert/utils/__init__.py b/PT-Retrieval/colbert/colbert/utils/__init__.py
new file mode 100644
index 0000000..e69de29
diff --git a/PT-Retrieval/colbert/colbert/utils/amp.py b/PT-Retrieval/colbert/colbert/utils/amp.py
new file mode 100644
index 0000000..3971e91
--- /dev/null
+++ b/PT-Retrieval/colbert/colbert/utils/amp.py
@@ -0,0 +1,39 @@
+import torch
+
+from contextlib import contextmanager
+from colbert.utils.utils import NullContextManager
+from packaging import version
+
+v = version.parse
+PyTorch_over_1_6 = v(torch.__version__) >= v('1.6')
+
+class MixedPrecisionManager():
+ def __init__(self, activated):
+ assert (not activated) or PyTorch_over_1_6, "Cannot use AMP for PyTorch version < 1.6"
+
+ self.activated = activated
+
+ if self.activated:
+ self.scaler = torch.cuda.amp.GradScaler()
+
+ def context(self):
+ return torch.cuda.amp.autocast() if self.activated else NullContextManager()
+
+ def backward(self, loss):
+ if self.activated:
+ self.scaler.scale(loss).backward()
+ else:
+ loss.backward()
+
+ def step(self, colbert, optimizer):
+ if self.activated:
+ self.scaler.unscale_(optimizer)
+ torch.nn.utils.clip_grad_norm_(colbert.parameters(), 2.0)
+
+ self.scaler.step(optimizer)
+ self.scaler.update()
+ optimizer.zero_grad()
+ else:
+ torch.nn.utils.clip_grad_norm_(colbert.parameters(), 2.0)
+ optimizer.step()
+ optimizer.zero_grad()
diff --git a/PT-Retrieval/colbert/colbert/utils/distributed.py b/PT-Retrieval/colbert/colbert/utils/distributed.py
new file mode 100644
index 0000000..64b3392
--- /dev/null
+++ b/PT-Retrieval/colbert/colbert/utils/distributed.py
@@ -0,0 +1,25 @@
+import os
+import random
+import torch
+import numpy as np
+
+
+def init(rank):
+ nranks = 'WORLD_SIZE' in os.environ and int(os.environ['WORLD_SIZE'])
+ nranks = max(1, nranks)
+ is_distributed = nranks > 1
+
+ if rank == 0:
+ print('nranks =', nranks, '\t num_gpus =', torch.cuda.device_count())
+
+ if is_distributed:
+ num_gpus = torch.cuda.device_count()
+ torch.cuda.set_device(rank % num_gpus)
+ torch.distributed.init_process_group(backend='nccl', init_method='env://')
+
+ return nranks, is_distributed
+
+
+def barrier(rank):
+ if rank >= 0:
+ torch.distributed.barrier()
diff --git a/PT-Retrieval/colbert/colbert/utils/logging.py b/PT-Retrieval/colbert/colbert/utils/logging.py
new file mode 100644
index 0000000..d11e96e
--- /dev/null
+++ b/PT-Retrieval/colbert/colbert/utils/logging.py
@@ -0,0 +1,99 @@
+import os
+import sys
+import ujson
+import mlflow
+import traceback
+
+from torch.utils.tensorboard import SummaryWriter
+from colbert.utils.utils import print_message, create_directory
+
+
+class Logger():
+ def __init__(self, rank, run):
+ self.rank = rank
+ self.is_main = self.rank in [-1, 0]
+ self.run = run
+ self.logs_path = os.path.join(self.run.path, "logs/")
+
+ if self.is_main:
+ self._init_mlflow()
+ self.initialized_tensorboard = False
+ create_directory(self.logs_path)
+
+ def _init_mlflow(self):
+ mlflow.set_tracking_uri('file://' + os.path.join(self.run.experiments_root, "logs/mlruns/"))
+ mlflow.set_experiment('/'.join([self.run.experiment, self.run.script]))
+
+ mlflow.set_tag('experiment', self.run.experiment)
+ mlflow.set_tag('name', self.run.name)
+ mlflow.set_tag('path', self.run.path)
+
+ def _init_tensorboard(self):
+ root = os.path.join(self.run.experiments_root, "logs/tensorboard/")
+ logdir = '__'.join([self.run.experiment, self.run.script, self.run.name])
+ logdir = os.path.join(root, logdir)
+
+ self.writer = SummaryWriter(log_dir=logdir)
+ self.initialized_tensorboard = True
+
+ def _log_exception(self, etype, value, tb):
+ if not self.is_main:
+ return
+
+ output_path = os.path.join(self.logs_path, 'exception.txt')
+ trace = ''.join(traceback.format_exception(etype, value, tb)) + '\n'
+ print_message(trace, '\n\n')
+
+ self.log_new_artifact(output_path, trace)
+
+ def _log_all_artifacts(self):
+ if not self.is_main:
+ return
+
+ mlflow.log_artifacts(self.logs_path)
+
+ def _log_args(self, args):
+ if not self.is_main:
+ return
+
+ for key in vars(args):
+ value = getattr(args, key)
+ if type(value) in [int, float, str, bool]:
+ mlflow.log_param(key, value)
+
+ with open(os.path.join(self.logs_path, 'args.json'), 'w') as output_metadata:
+ ujson.dump(args.input_arguments.__dict__, output_metadata, indent=4)
+ output_metadata.write('\n')
+
+ with open(os.path.join(self.logs_path, 'args.txt'), 'w') as output_metadata:
+ output_metadata.write(' '.join(sys.argv) + '\n')
+
+ def log_metric(self, name, value, step, log_to_mlflow=True):
+ if not self.is_main:
+ return
+
+ if not self.initialized_tensorboard:
+ self._init_tensorboard()
+
+ if log_to_mlflow:
+ mlflow.log_metric(name, value, step=step)
+ self.writer.add_scalar(name, value, step)
+
+ def log_new_artifact(self, path, content):
+ with open(path, 'w') as f:
+ f.write(content)
+
+ mlflow.log_artifact(path)
+
+ def warn(self, *args):
+ msg = print_message('[WARNING]', '\t', *args)
+
+ with open(os.path.join(self.logs_path, 'warnings.txt'), 'a') as output_metadata:
+ output_metadata.write(msg + '\n\n\n')
+
+ def info_all(self, *args):
+ print_message('[' + str(self.rank) + ']', '\t', *args)
+
+ def info(self, *args):
+ if self.is_main:
+ print_message(*args)
diff --git a/PT-Retrieval/colbert/colbert/utils/parser.py b/PT-Retrieval/colbert/colbert/utils/parser.py
new file mode 100644
index 0000000..af830a9
--- /dev/null
+++ b/PT-Retrieval/colbert/colbert/utils/parser.py
@@ -0,0 +1,120 @@
+import os
+import copy
+import faiss
+
+from argparse import ArgumentParser
+
+import colbert.utils.distributed as distributed
+from colbert.utils.runs import Run
+from colbert.utils.utils import print_message, timestamp, create_directory
+
+
+class Arguments():
+ def __init__(self, description):
+ self.parser = ArgumentParser(description=description)
+ self.checks = []
+
+ self.add_argument('--root', dest='root', default='experiments')
+ self.add_argument('--experiment', dest='experiment', default='dirty')
+ self.add_argument('--run', dest='run', default=Run.name)
+
+ self.add_argument('--local_rank', dest='rank', default=-1, type=int)
+
+ self.add_argument("--prefix", action="store_true", help="use p-tuning v2")
+ self.add_argument("--pre_seq_len", type=int, default=100, help="the length of prefix used in p-tuning v2")
+ self.add_argument("--prefix_hidden_size", type=int, default=512)
+ self.add_argument("--prefix_mlp", action="store_true")
+ self.add_argument("--scheduler", action="store_true")
+
+ def add_model_parameters(self):
+ # Core Arguments
+ self.add_argument('--similarity', dest='similarity', default='cosine', choices=['cosine', 'l2'])
+ self.add_argument('--dim', dest='dim', default=128, type=int)
+ self.add_argument('--query_maxlen', dest='query_maxlen', default=32, type=int)
+ self.add_argument('--doc_maxlen', dest='doc_maxlen', default=180, type=int)
+
+ # Filtering-related Arguments
+ self.add_argument('--mask-punctuation', dest='mask_punctuation', default=False, action='store_true')
+
+ def add_model_training_parameters(self):
+ # NOTE: Providing a checkpoint is one thing, --resume is another, --resume_optimizer is yet another.
+ self.add_argument('--resume', dest='resume', default=False, action='store_true')
+ self.add_argument('--resume_optimizer', dest='resume_optimizer', default=False, action='store_true')
+ self.add_argument('--checkpoint', dest='checkpoint', default=None, required=False)
+
+ self.add_argument('--lr', dest='lr', default=3e-06, type=float)
+ self.add_argument('--maxsteps', dest='maxsteps', default=400000, type=int)
+ self.add_argument('--bsize', dest='bsize', default=32, type=int)
+ self.add_argument('--accum', dest='accumsteps', default=2, type=int)
+ self.add_argument('--amp', dest='amp', default=False, action='store_true')
+
+ def add_model_inference_parameters(self):
+ self.add_argument('--checkpoint', dest='checkpoint', required=True)
+ self.add_argument('--bsize', dest='bsize', default=128, type=int)
+ self.add_argument('--amp', dest='amp', default=False, action='store_true')
+
+ def add_training_input(self):
+ self.add_argument('--triples', dest='triples', required=True)
+ self.add_argument('--queries', dest='queries', default=None)
+ self.add_argument('--collection', dest='collection', default=None)
+
+ def check_training_input(args):
+ assert (args.collection is None) == (args.queries is None), \
+ "For training, both (or neither) --collection and --queries must be supplied." \
+ "If neither is supplied, the --triples file must contain texts (not PIDs)."
+
+ self.checks.append(check_training_input)
+
+ def add_ranking_input(self):
+ self.add_argument('--queries', dest='queries', default=None)
+ self.add_argument('--collection', dest='collection', default=None)
+ self.add_argument('--qrels', dest='qrels', default=None)
+
+ def add_reranking_input(self):
+ self.add_ranking_input()
+ self.add_argument('--topk', dest='topK', required=True)
+ self.add_argument('--shortcircuit', dest='shortcircuit', default=False, action='store_true')
+
+ def add_indexing_input(self):
+ self.add_argument('--collection', dest='collection', required=True)
+ self.add_argument('--index_root', dest='index_root', required=True)
+ self.add_argument('--index_name', dest='index_name', required=True)
+
+ def add_index_use_input(self):
+ self.add_argument('--index_root', dest='index_root', required=True)
+ self.add_argument('--index_name', dest='index_name', required=True)
+ self.add_argument('--partitions', dest='partitions', default=None, type=int)
+
+ def add_retrieval_input(self):
+ self.add_index_use_input()
+ self.add_argument('--nprobe', dest='nprobe', default=10, type=int)
+ self.add_argument('--retrieve_only', dest='retrieve_only', default=False, action='store_true')
+
+ def add_argument(self, *args, **kw_args):
+ return self.parser.add_argument(*args, **kw_args)
+
+ def check_arguments(self, args):
+ for check in self.checks:
+ check(args)
+
+ def parse(self):
+ args = self.parser.parse_args()
+ self.check_arguments(args)
+
+ args.input_arguments = copy.deepcopy(args)
+
+ args.nranks, args.distributed = distributed.init(args.rank)
+
+ args.nthreads = int(max(os.cpu_count(), faiss.omp_get_max_threads()) * 0.8)
+ args.nthreads = max(1, args.nthreads // args.nranks)
+
+ if args.nranks > 1:
+ print_message(f"#> Restricting number of threads for FAISS to {args.nthreads} per process",
+ condition=(args.rank == 0))
+ faiss.omp_set_num_threads(args.nthreads)
+
+ Run.init(args.rank, args.root, args.experiment, args.run)
+ Run._log_args(args)
+ Run.info(args.input_arguments.__dict__, '\n')
+
+ return args
diff --git a/PT-Retrieval/colbert/colbert/utils/runs.py b/PT-Retrieval/colbert/colbert/utils/runs.py
new file mode 100644
index 0000000..283b706
--- /dev/null
+++ b/PT-Retrieval/colbert/colbert/utils/runs.py
@@ -0,0 +1,107 @@
+import os
+import sys
+import time
+import __main__
+import traceback
+import mlflow
+
+import colbert.utils.distributed as distributed
+
+from contextlib import contextmanager
+from colbert.utils.logging import Logger
+from colbert.utils.utils import timestamp, create_directory, print_message
+
+
+class _RunManager():
+ def __init__(self):
+ self.experiments_root = None
+ self.experiment = None
+ self.path = None
+ self.script = self._get_script_name()
+ self.name = self._generate_default_run_name()
+ self.original_name = self.name
+ self.exit_status = 'FINISHED'
+
+ self._logger = None
+ self.start_time = time.time()
+
+ def init(self, rank, root, experiment, name):
+ experiment = experiment.replace("cqadupstack/", "cqadupstack.")
+ name = name.replace("cqadupstack/", "cqadupstack.")
+
+ assert '/' not in experiment, experiment
+ assert '/' not in name, name
+
+ self.experiments_root = os.path.abspath(root)
+ self.experiment = experiment
+ self.name = name
+ self.path = os.path.join(self.experiments_root, self.experiment, self.script, self.name)
+
+ if rank < 1:
+ if os.path.exists(self.path):
+ print('\n\n')
+ print_message("It seems that ", self.path, " already exists.")
+ print_message("Do you want to overwrite it? \t yes/no \n")
+
+ # TODO: This should timeout and exit (i.e., fail) given no response for 60 seconds.
+
+ response = input()
+ if response.strip() != 'yes':
+ assert not os.path.exists(self.path), self.path
+ else:
+ create_directory(self.path)
+
+ distributed.barrier(rank)
+
+ self._logger = Logger(rank, self)
+ self._log_args = self._logger._log_args
+ self.warn = self._logger.warn
+ self.info = self._logger.info
+ self.info_all = self._logger.info_all
+ self.log_metric = self._logger.log_metric
+ self.log_new_artifact = self._logger.log_new_artifact
+
+ def _generate_default_run_name(self):
+ return timestamp()
+
+ def _get_script_name(self):
+ return os.path.basename(__main__.__file__) if '__file__' in dir(__main__) else 'none'
+
+ @contextmanager
+ def context(self, consider_failed_if_interrupted=True):
+ try:
+ yield
+
+ except KeyboardInterrupt as ex:
+ print('\n\nInterrupted\n\n')
+ self._logger._log_exception(ex.__class__, ex, ex.__traceback__)
+ self._logger._log_all_artifacts()
+
+ if consider_failed_if_interrupted:
+ self.exit_status = 'KILLED' # mlflow.entities.RunStatus.KILLED
+
+ sys.exit(128 + 2)
+
+ except Exception as ex:
+ self._logger._log_exception(ex.__class__, ex, ex.__traceback__)
+ self._logger._log_all_artifacts()
+
+ self.exit_status = 'FAILED' # mlflow.entities.RunStatus.FAILED
+
+ raise ex
+
+ finally:
+ total_seconds = str(time.time() - self.start_time) + '\n'
+ original_name = str(self.original_name)
+ name = str(self.name)
+
+ self.log_new_artifact(os.path.join(self._logger.logs_path, 'elapsed.txt'), total_seconds)
+ self.log_new_artifact(os.path.join(self._logger.logs_path, 'name.original.txt'), original_name)
+ self.log_new_artifact(os.path.join(self._logger.logs_path, 'name.txt'), name)
+
+ self._logger._log_all_artifacts()
+
+ mlflow.end_run(status=self.exit_status)
+
+
+Run = _RunManager()
diff --git a/PT-Retrieval/colbert/colbert/utils/utils.py b/PT-Retrieval/colbert/colbert/utils/utils.py
new file mode 100644
index 0000000..a3423d7
--- /dev/null
+++ b/PT-Retrieval/colbert/colbert/utils/utils.py
@@ -0,0 +1,279 @@
+import os
+import tqdm
+import torch
+import datetime
+import itertools
+
+from multiprocessing import Pool
+from collections import OrderedDict, defaultdict
+
+
+def print_message(*s, condition=True):
+ s = ' '.join([str(x) for x in s])
+ msg = "[{}] {}".format(datetime.datetime.now().strftime("%b %d, %H:%M:%S"), s)
+
+ if condition:
+ print(msg, flush=True)
+
+ return msg
+
+
+def timestamp():
+ format_str = "%Y-%m-%d_%H.%M.%S"
+ result = datetime.datetime.now().strftime(format_str)
+ return result
+
+
+def file_tqdm(file):
+ print(f"#> Reading {file.name}")
+
+ with tqdm.tqdm(total=os.path.getsize(file.name) / 1024.0 / 1024.0, unit="MiB") as pbar:
+ for line in file:
+ yield line
+ pbar.update(len(line) / 1024.0 / 1024.0)
+
+ pbar.close()
+
+
+def save_checkpoint(path, epoch_idx, mb_idx, model, optimizer, arguments=None, prefix=False):
+ print(f"#> Saving a checkpoint to {path} ..")
+
+ if hasattr(model, 'module'):
+ model = model.module # extract model from a distributed/data-parallel wrapper
+
+ if prefix:
+ model_dict = {key: value for (key, value) in model.state_dict().items() if "prefix_encoder" in key or "linear" in key}
+ else:
+ model_dict = model.state_dict()
+
+ checkpoint = {}
+ checkpoint['epoch'] = epoch_idx
+ checkpoint['batch'] = mb_idx
+ checkpoint['model_state_dict'] = model_dict
+ checkpoint['optimizer_state_dict'] = optimizer.state_dict()
+ checkpoint['arguments'] = arguments
+
+ torch.save(checkpoint, path)
+
+
+def load_checkpoint(path, model, optimizer=None, do_print=True):
+ if do_print:
+ print_message("#> Loading checkpoint", path, "..")
+
+ if path.startswith("http:") or path.startswith("https:"):
+ checkpoint = torch.hub.load_state_dict_from_url(path, map_location='cpu')
+ else:
+ checkpoint = torch.load(path, map_location='cpu')
+
+ state_dict = checkpoint['model_state_dict']
+ new_state_dict = OrderedDict()
+ for k, v in state_dict.items():
+ name = k
+ if k[:7] == 'module.':
+ name = k[7:]
+ new_state_dict[name] = v
+
+
+ checkpoint['model_state_dict'] = new_state_dict
+ # checkpoint['model_state_dict'] = {key: value for (key, value) in new_state_dict.items() if "prefix_encoder" in key or "linear" in key}
+ # for k, v in checkpoint['model_state_dict'].items():
+ # print(k, v.shape)
+ try:
+ model.load_state_dict(checkpoint['model_state_dict'])
+ except:
+ print_message("[WARNING] Loading checkpoint with strict=False")
+ model.load_state_dict(checkpoint['model_state_dict'], strict=False)
+
+ if optimizer:
+ optimizer.load_state_dict(checkpoint['optimizer_state_dict'])
+
+ if do_print:
+ print_message("#> checkpoint['epoch'] =", checkpoint['epoch'])
+ print_message("#> checkpoint['batch'] =", checkpoint['batch'])
+
+ return checkpoint
+
+
+def create_directory(path):
+ if os.path.exists(path):
+ print('\n')
+ print_message("#> Note: Output directory", path, 'already exists\n\n')
+ else:
+ print('\n')
+ print_message("#> Creating directory", path, '\n\n')
+ os.makedirs(path)
+
+# def batch(file, bsize):
+# while True:
+# L = [ujson.loads(file.readline()) for _ in range(bsize)]
+# yield L
+# return
+
+
+def f7(seq):
+ """
+ Source: https://stackoverflow.com/a/480227/1493011
+ """
+
+ seen = set()
+ return [x for x in seq if not (x in seen or seen.add(x))]
+
+
+def batch(group, bsize, provide_offset=False):
+ offset = 0
+ while offset < len(group):
+ L = group[offset: offset + bsize]
+ yield ((offset, L) if provide_offset else L)
+ offset += len(L)
+ return
+
+
+class dotdict(dict):
+ """
+ dot.notation access to dictionary attributes
+ Credit: derek73 @ https://stackoverflow.com/questions/2352181
+ """
+ __getattr__ = dict.__getitem__
+ __setattr__ = dict.__setitem__
+ __delattr__ = dict.__delitem__
+
+
+def flatten(L):
+ return [x for y in L for x in y]
+
+
+def zipstar(L, lazy=False):
+ """
+ A much faster A, B, C = zip(*[(a, b, c), (a, b, c), ...])
+ May return lists or tuples.
+ """
+
+ if len(L) == 0:
+ return L
+
+ width = len(L[0])
+
+ if width < 100:
+ return [[elem[idx] for elem in L] for idx in range(width)]
+
+ L = zip(*L)
+
+ return L if lazy else list(L)
+
+
+def zip_first(L1, L2):
+ length = len(L1) if type(L1) in [tuple, list] else None
+
+ L3 = list(zip(L1, L2))
+
+ assert length in [None, len(L3)], "zip_first() failure: length differs!"
+
+ return L3
+
+
+def int_or_float(val):
+ if '.' in val:
+ return float(val)
+
+ return int(val)
+
+def load_ranking(path, types=None, lazy=False):
+ print_message(f"#> Loading the ranked lists from {path} ..")
+
+ try:
+ lists = torch.load(path)
+ lists = zipstar([l.tolist() for l in tqdm.tqdm(lists)], lazy=lazy)
+ except:
+ if types is None:
+ types = itertools.cycle([int_or_float])
+
+ with open(path) as f:
+ lists = [[typ(x) for typ, x in zip_first(types, line.strip().split('\t'))]
+ for line in file_tqdm(f)]
+
+ return lists
+
+
+def save_ranking(ranking, path):
+ lists = zipstar(ranking)
+ lists = [torch.tensor(l) for l in lists]
+
+ torch.save(lists, path)
+
+ return lists
+
+
+def groupby_first_item(lst):
+ groups = defaultdict(list)
+
+ for first, *rest in lst:
+ rest = rest[0] if len(rest) == 1 else rest
+ groups[first].append(rest)
+
+ return groups
+
+
+def process_grouped_by_first_item(lst):
+ """
+ Requires items in list to already be grouped by first item.
+ """
+
+ groups = defaultdict(list)
+
+ started = False
+ last_group = None
+
+ for first, *rest in lst:
+ rest = rest[0] if len(rest) == 1 else rest
+
+ if started and first != last_group:
+ yield (last_group, groups[last_group])
+ assert first not in groups, f"{first} seen earlier --- violates precondition."
+
+ groups[first].append(rest)
+
+ last_group = first
+ started = True
+
+ return groups
+
+
+def grouper(iterable, n, fillvalue=None):
+ """
+ Collect data into fixed-length chunks or blocks
+ Example: grouper('ABCDEFG', 3, 'x') --> ABC DEF Gxx"
+ Source: https://docs.python.org/3/library/itertools.html#itertools-recipes
+ """
+
+ args = [iter(iterable)] * n
+ return itertools.zip_longest(*args, fillvalue=fillvalue)
+
+
+# see https://stackoverflow.com/a/45187287
+class NullContextManager(object):
+ def __init__(self, dummy_resource=None):
+ self.dummy_resource = dummy_resource
+ def __enter__(self):
+ return self.dummy_resource
+ def __exit__(self, *args):
+ pass
+
+
+def load_batch_backgrounds(args, qids):
+ if args.qid2backgrounds is None:
+ return None
+
+ qbackgrounds = []
+
+ for qid in qids:
+ back = args.qid2backgrounds[qid]
+
+ if len(back) and type(back[0]) == int:
+ x = [args.collection[pid] for pid in back]
+ else:
+ x = [args.collectionX.get(pid, '') for pid in back]
+
+ x = ' [SEP] '.join(x)
+ qbackgrounds.append(x)
+
+ return qbackgrounds
diff --git a/PT-Retrieval/colbert/scripts/config.sh b/PT-Retrieval/colbert/scripts/config.sh
new file mode 100644
index 0000000..29cb039
--- /dev/null
+++ b/PT-Retrieval/colbert/scripts/config.sh
@@ -0,0 +1,36 @@
+#!/bin/bash
+
+export dataset=$1
+export psl=64
+export step=36
+export SIMILARITY=cosine
+export checkpoint=ptv2.colbert.msmarco.cosine.1e-3.64
+
+export DATA_DIR=../beir_eval/datasets/$dataset/
+
+export CHECKPOINT=./checkpoints/$checkpoint/colbert-${step}0000.dnn
+
+# Path where preprocessed collection and queries are present
+export COLLECTION="../beir_eval/preprocessed_data/${dataset}/collection.tsv"
+export QUERIES="../beir_eval/preprocessed_data/${dataset}/queries.tsv"
+
+# Path to store the faiss index and run output
+export INDEX_ROOT="output/index"
+export OUTPUT_DIR="output/output"
+export EXPERIMENT=$checkpoint.$step.$dataset
+# Path to store the rankings file
+export RANKING_DIR="output/rankings/${checkpoint}/${step}/${dataset}"
+
+# Num of partitions in for IVPQ Faiss index (You must decide yourself)
+export NUM_PARTITIONS=96
+
+# Some Index Name to store the faiss index
+export INDEX_NAME=index.$checkpoint.$step.$dataset
+
+if [ "$dataset" = "msmarco" ]; then
+ SPLIT="dev"
+else
+ SPLIT="test"
+fi
+
+echo $SPLIT
\ No newline at end of file
diff --git a/PT-Retrieval/colbert/scripts/evalute_on_beir.sh b/PT-Retrieval/colbert/scripts/evalute_on_beir.sh
new file mode 100644
index 0000000..22a58e8
--- /dev/null
+++ b/PT-Retrieval/colbert/scripts/evalute_on_beir.sh
@@ -0,0 +1,78 @@
+source scripts/config.sh
+
+MASTER_PORT=$(shuf -n 1 -i 10000-65535)
+
+################################################################
+# 0. BEIR Data Formatting: Format BEIR data useful for ColBERT #
+################################################################
+
+python3 -m colbert.data_prep \
+ --dataset $dataset \
+ --split $SPLIT \
+ --collection $COLLECTION \
+ --queries $QUERIES \
+ --data_dir $DATA_DIR
+
+############################################################################
+# 1. Indexing: Encode document (token) embeddings using ColBERT checkpoint #
+############################################################################
+
+python3 -m torch.distributed.launch --master_port=$MASTER_PORT \
+ --nproc_per_node=2 -m colbert.index \
+ --root $OUTPUT_DIR \
+ --doc_maxlen 300 \
+ --mask-punctuation \
+ --bsize 128 \
+ --amp \
+ --checkpoint $CHECKPOINT \
+ --index_root $INDEX_ROOT \
+ --index_name $INDEX_NAME \
+ --collection $COLLECTION \
+ --experiment $EXPERIMENT \
+ --prefix --pre_seq_len $psl --similarity $SIMILARITY
+
+###########################################################################################
+# 2. Faiss Indexing (End-to-End Retrieval): Store document (token) embeddings using Faiss #
+###########################################################################################
+
+python3 -m colbert.index_faiss \
+ --index_root $INDEX_ROOT \
+ --index_name $INDEX_NAME \
+ --partitions $NUM_PARTITIONS \
+ --sample 0.3 \
+ --root $OUTPUT_DIR \
+ --experiment $EXPERIMENT
+
+####################################################################################
+# 3. Retrieval: retrieve relevant documents of queries from faiss index checkpoint #
+####################################################################################
+
+python3 -m colbert.retrieve \
+ --amp \
+ --doc_maxlen 300 \
+ --mask-punctuation \
+ --bsize 256 \
+ --queries $QUERIES \
+ --nprobe 32 \
+ --partitions $NUM_PARTITIONS \
+ --faiss_depth 100 \
+ --depth 100 \
+ --index_root $INDEX_ROOT \
+ --index_name $INDEX_NAME \
+ --checkpoint $CHECKPOINT \
+ --root $OUTPUT_DIR \
+ --experiment $EXPERIMENT \
+ --ranking_dir $RANKING_DIR \
+ --prefix --pre_seq_len $psl --similarity $SIMILARITY
+
+
+######################################################################
+# 4. BEIR Evaluation: Evaluate Rankings with BEIR Evaluation Metrics #
+######################################################################
+
+python3 -m colbert.beir_eval \
+ --dataset ${dataset} \
+ --split $SPLIT \
+ --collection $COLLECTION \
+ --rankings "${RANKING_DIR}/ranking.tsv" \
+ --data_dir $DATA_DIR \
\ No newline at end of file
diff --git a/PT-Retrieval/colbert/scripts/filter.sh b/PT-Retrieval/colbert/scripts/filter.sh
new file mode 100644
index 0000000..3a056a2
--- /dev/null
+++ b/PT-Retrieval/colbert/scripts/filter.sh
@@ -0,0 +1,47 @@
+export dataset=$1
+export psl=64
+export step=36
+export SIMILARITY=cosine
+export checkpoint=ptv2.colbert.msmarco.cosine.1e-3.64.full
+
+export DATA_DIR=../beir_eval/datasets/$dataset/
+
+export CHECKPOINT=./checkpoints/$checkpoint/colbert-${step}0000.dnn
+
+# Path where preprocessed collection and queries are present
+export COLLECTION="../beir_eval/preprocessed_data/${dataset}/collection.tsv"
+export QUERIES="../beir_eval/preprocessed_data/${dataset}/queries.tsv"
+
+# Path to store the faiss index and run output
+export INDEX_ROOT="output/index"
+export OUTPUT_DIR="output/output"
+export EXPERIMENT=$checkpoint.$step.$dataset
+# Path to store the rankings file
+export RANKING_DIR="output/rankings/${checkpoint}/${step}/${dataset}"
+
+# Num of partitions in for IVPQ Faiss index (You must decide yourself)
+export NUM_PARTITIONS=96
+
+# Some Index Name to store the faiss index
+export INDEX_NAME=index.$checkpoint.$step.$dataset
+
+MASTER_PORT=$(shuf -n 1 -i 10000-65535)
+
+python3 -m colbert.filter_model \
+ --amp \
+ --doc_maxlen 300 \
+ --mask-punctuation \
+ --bsize 256 \
+ --queries $QUERIES \
+ --nprobe 32 \
+ --partitions $NUM_PARTITIONS \
+ --faiss_depth 100 \
+ --depth 100 \
+ --index_root $INDEX_ROOT \
+ --index_name $INDEX_NAME \
+ --checkpoint $CHECKPOINT \
+ --root $OUTPUT_DIR \
+ --experiment $EXPERIMENT \
+ --ranking_dir $RANKING_DIR \
+ --prefix --pre_seq_len $psl --similarity $SIMILARITY
+
diff --git a/PT-Retrieval/colbert/scripts/preprocess.sh b/PT-Retrieval/colbert/scripts/preprocess.sh
new file mode 100644
index 0000000..dcca2c4
--- /dev/null
+++ b/PT-Retrieval/colbert/scripts/preprocess.sh
@@ -0,0 +1,8 @@
+source scripts/config.sh
+
+python3 -m colbert.data_prep \
+ --dataset $dataset \
+ --split "test" \
+ --collection $COLLECTION \
+ --queries $QUERIES \
+ --data_dir $DATA_DIR
\ No newline at end of file
diff --git a/PT-Retrieval/colbert/scripts/run_train_colbert_ptv2.sh b/PT-Retrieval/colbert/scripts/run_train_colbert_ptv2.sh
new file mode 100644
index 0000000..b857af8
--- /dev/null
+++ b/PT-Retrieval/colbert/scripts/run_train_colbert_ptv2.sh
@@ -0,0 +1,20 @@
+export CUDA_VISIBLE_DEVICES=0,1,2,3
+export PYTHONPATH=.
+
+lr=1e-3
+psl=180
+
+MASTER_PORT=$(shuf -n 1 -i 10000-65535)
+
+python3 -m torch.distributed.launch --nproc_per_node=4 --master_port=$MASTER_PORT colbert/train.py \
+ --amp \
+ --doc_maxlen 300 \
+ --mask-punctuation \
+ --lr $lr \
+ --bsize 32 --accum 1 \
+ --triples ../data/msmarco/triples.train.small.tsv \
+ --root ./ \
+ --experiment checkpoints \
+ --similarity cosine \
+ --run ptv2.colbert.msmarco.cosine.$lr.$psl \
+ --prefix --pre_seq_len $psl
\ No newline at end of file
diff --git a/PT-Retrieval/colbert/utility/evaluate/annotate_EM.py b/PT-Retrieval/colbert/utility/evaluate/annotate_EM.py
new file mode 100644
index 0000000..ecd8704
--- /dev/null
+++ b/PT-Retrieval/colbert/utility/evaluate/annotate_EM.py
@@ -0,0 +1,81 @@
+import os
+import sys
+import git
+import tqdm
+import ujson
+import random
+
+from argparse import ArgumentParser
+from multiprocessing import Pool
+
+from colbert.utils.utils import print_message, load_ranking, groupby_first_item
+from utility.utils.qa_loaders import load_qas_, load_collection_
+from utility.utils.save_metadata import format_metadata, get_metadata
+from utility.evaluate.annotate_EM_helpers import *
+
+
+# TODO: Tokenize passages in advance, especially if the ranked list is long! This requires changes to the has_answer input, slightly.
+
+def main(args):
+ qas = load_qas_(args.qas)
+ collection = load_collection_(args.collection, retain_titles=True)
+ rankings = load_ranking(args.ranking)
+ parallel_pool = Pool(30)
+
+ print_message('#> Tokenize the answers in the Q&As in parallel...')
+ qas = list(parallel_pool.map(tokenize_all_answers, qas))
+
+ qid2answers = {qid: tok_answers for qid, _, tok_answers in qas}
+ assert len(qas) == len(qid2answers), (len(qas), len(qid2answers))
+
+ print_message('#> Lookup passages from PIDs...')
+ expanded_rankings = [(qid, pid, rank, collection[pid], qid2answers[qid])
+ for qid, pid, rank, *_ in rankings]
+
+ print_message('#> Assign labels in parallel...')
+ labeled_rankings = list(parallel_pool.map(assign_label_to_passage, enumerate(expanded_rankings)))
+
+ # Dump output.
+ print_message("#> Dumping output to", args.output, "...")
+ qid2rankings = groupby_first_item(labeled_rankings)
+
+ num_judged_queries, num_ranked_queries = check_sizes(qid2answers, qid2rankings)
+
+ # Evaluation metrics and depths.
+ success, counts = compute_and_write_labels(args.output, qid2answers, qid2rankings)
+
+ # Dump metrics.
+ with open(args.output_metrics, 'w') as f:
+ d = {'num_ranked_queries': num_ranked_queries, 'num_judged_queries': num_judged_queries}
+
+ extra = '__WARNING' if num_judged_queries != num_ranked_queries else ''
+ d[f'success{extra}'] = {k: v / num_judged_queries for k, v in success.items()}
+ d[f'counts{extra}'] = {k: v / num_judged_queries for k, v in counts.items()}
+ d['arguments'] = get_metadata(args)
+
+ f.write(format_metadata(d) + '\n')
+
+ print('\n\n')
+ print(args.output)
+ print(args.output_metrics)
+ print("#> Done\n")
+
+
+if __name__ == "__main__":
+ random.seed(12345)
+
+ parser = ArgumentParser(description='.')
+
+ # Input / Output Arguments
+ parser.add_argument('--qas', dest='qas', required=True, type=str)
+ parser.add_argument('--collection', dest='collection', required=True, type=str)
+ parser.add_argument('--ranking', dest='ranking', required=True, type=str)
+
+ args = parser.parse_args()
+
+ args.output = f'{args.ranking}.annotated'
+ args.output_metrics = f'{args.ranking}.annotated.metrics'
+
+ assert not os.path.exists(args.output), args.output
+
+ main(args)
diff --git a/PT-Retrieval/colbert/utility/evaluate/annotate_EM_helpers.py b/PT-Retrieval/colbert/utility/evaluate/annotate_EM_helpers.py
new file mode 100644
index 0000000..b0d357a
--- /dev/null
+++ b/PT-Retrieval/colbert/utility/evaluate/annotate_EM_helpers.py
@@ -0,0 +1,74 @@
+from colbert.utils.utils import print_message
+from utility.utils.dpr import DPR_normalize, has_answer
+
+
+def tokenize_all_answers(args):
+ qid, question, answers = args
+ return qid, question, [DPR_normalize(ans) for ans in answers]
+
+
+def assign_label_to_passage(args):
+ idx, (qid, pid, rank, passage, tokenized_answers) = args
+
+ if idx % (1*1000*1000) == 0:
+ print(idx)
+
+ return qid, pid, rank, has_answer(tokenized_answers, passage)
+
+
+def check_sizes(qid2answers, qid2rankings):
+ num_judged_queries = len(qid2answers)
+ num_ranked_queries = len(qid2rankings)
+
+ print_message('num_judged_queries =', num_judged_queries)
+ print_message('num_ranked_queries =', num_ranked_queries)
+
+ if num_judged_queries != num_ranked_queries:
+ assert num_ranked_queries <= num_judged_queries
+
+ print('\n\n')
+ print_message('[WARNING] num_judged_queries != num_ranked_queries')
+ print('\n\n')
+
+ return num_judged_queries, num_ranked_queries
+
+
+def compute_and_write_labels(output_path, qid2answers, qid2rankings):
+ cutoffs = [1, 5, 10, 20, 30, 50, 100, 1000, 'all']
+ success = {cutoff: 0.0 for cutoff in cutoffs}
+ counts = {cutoff: 0.0 for cutoff in cutoffs}
+
+ with open(output_path, 'w') as f:
+ for qid in qid2answers:
+ if qid not in qid2rankings:
+ continue
+
+ prev_rank = 0 # ranks should start at one (i.e., and not zero)
+ labels = []
+
+ for pid, rank, label in qid2rankings[qid]:
+ assert rank == prev_rank+1, (qid, pid, (prev_rank, rank))
+ prev_rank = rank
+
+ labels.append(label)
+ line = '\t'.join(map(str, [qid, pid, rank, int(label)])) + '\n'
+ f.write(line)
+
+ for cutoff in cutoffs:
+ if cutoff != 'all':
+ success[cutoff] += sum(labels[:cutoff]) > 0
+ counts[cutoff] += sum(labels[:cutoff])
+ else:
+ success[cutoff] += sum(labels) > 0
+ counts[cutoff] += sum(labels)
+
+ return success, counts
+
+
+# def dump_metrics(f, nqueries, cutoffs, success, counts):
+# for cutoff in cutoffs:
+# success_log = "#> P@{} = {}".format(cutoff, success[cutoff] / nqueries)
+# counts_log = "#> D@{} = {}".format(cutoff, counts[cutoff] / nqueries)
+# print('\n'.join([success_log, counts_log]) + '\n')
+
+# f.write('\n'.join([success_log, counts_log]) + '\n\n')
diff --git a/PT-Retrieval/colbert/utility/evaluate/msmarco_passages.py b/PT-Retrieval/colbert/utility/evaluate/msmarco_passages.py
new file mode 100644
index 0000000..770e4cf
--- /dev/null
+++ b/PT-Retrieval/colbert/utility/evaluate/msmarco_passages.py
@@ -0,0 +1,126 @@
+"""
+ Evaluate MS MARCO Passages ranking.
+"""
+
+import os
+import math
+import tqdm
+import ujson
+import random
+
+from argparse import ArgumentParser
+from collections import defaultdict
+from colbert.utils.utils import print_message, file_tqdm
+
+
+def main(args):
+ qid2positives = defaultdict(list)
+ qid2ranking = defaultdict(list)
+ qid2mrr = {}
+ qid2recall = {depth: {} for depth in [50, 200, 1000]}
+
+ with open(args.qrels) as f:
+ print_message(f"#> Loading QRELs from {args.qrels} ..")
+ for line in file_tqdm(f):
+ qid, _, pid, label = map(int, line.strip().split())
+ assert label == 1
+
+ qid2positives[qid].append(pid)
+
+ with open(args.ranking) as f:
+ print_message(f"#> Loading ranked lists from {args.ranking} ..")
+ for line in file_tqdm(f):
+ qid, pid, rank, *score = line.strip().split('\t')
+ qid, pid, rank = int(qid), int(pid), int(rank)
+
+ if len(score) > 0:
+ assert len(score) == 1
+ score = float(score[0])
+ else:
+ score = None
+
+ qid2ranking[qid].append((rank, pid, score))
+
+ assert set.issubset(set(qid2ranking.keys()), set(qid2positives.keys()))
+
+ num_judged_queries = len(qid2positives)
+ num_ranked_queries = len(qid2ranking)
+
+ if num_judged_queries != num_ranked_queries:
+ print()
+ print_message("#> [WARNING] num_judged_queries != num_ranked_queries")
+ print_message(f"#> {num_judged_queries} != {num_ranked_queries}")
+ print()
+
+ print_message(f"#> Computing MRR@10 for {num_judged_queries} queries.")
+
+ for qid in tqdm.tqdm(qid2positives):
+ ranking = qid2ranking[qid]
+ positives = qid2positives[qid]
+
+ for rank, (_, pid, _) in enumerate(ranking):
+ rank = rank + 1 # 1-indexed
+
+ if pid in positives:
+ if rank <= 10:
+ qid2mrr[qid] = 1.0 / rank
+ break
+
+ for rank, (_, pid, _) in enumerate(ranking):
+ rank = rank + 1 # 1-indexed
+
+ if pid in positives:
+ for depth in qid2recall:
+ if rank <= depth:
+ qid2recall[depth][qid] = qid2recall[depth].get(qid, 0) + 1.0 / len(positives)
+
+ assert len(qid2mrr) <= num_ranked_queries, (len(qid2mrr), num_ranked_queries)
+
+ print()
+ mrr_10_sum = sum(qid2mrr.values())
+ print_message(f"#> MRR@10 = {mrr_10_sum / num_judged_queries}")
+ print_message(f"#> MRR@10 (only for ranked queries) = {mrr_10_sum / num_ranked_queries}")
+ print()
+
+ for depth in qid2recall:
+ assert len(qid2recall[depth]) <= num_ranked_queries, (len(qid2recall[depth]), num_ranked_queries)
+
+ print()
+ metric_sum = sum(qid2recall[depth].values())
+ print_message(f"#> Recall@{depth} = {metric_sum / num_judged_queries}")
+ print_message(f"#> Recall@{depth} (only for ranked queries) = {metric_sum / num_ranked_queries}")
+ print()
+
+ if args.annotate:
+ print_message(f"#> Writing annotations to {args.output} ..")
+
+ with open(args.output, 'w') as f:
+ for qid in tqdm.tqdm(qid2positives):
+ ranking = qid2ranking[qid]
+ positives = qid2positives[qid]
+
+ for rank, (_, pid, score) in enumerate(ranking):
+ rank = rank + 1 # 1-indexed
+ label = int(pid in positives)
+
+ line = [qid, pid, rank, score, label]
+ line = [x for x in line if x is not None]
+ line = '\t'.join(map(str, line)) + '\n'
+ f.write(line)
+
+
+if __name__ == "__main__":
+ parser = ArgumentParser(description="msmarco_passages.")
+
+ # Input Arguments.
+ parser.add_argument('--qrels', dest='qrels', required=True, type=str)
+ parser.add_argument('--ranking', dest='ranking', required=True, type=str)
+ parser.add_argument('--annotate', dest='annotate', default=False, action='store_true')
+
+ args = parser.parse_args()
+
+ if args.annotate:
+ args.output = f'{args.ranking}.annotated'
+ assert not os.path.exists(args.output), args.output
+
+ main(args)
diff --git a/PT-Retrieval/colbert/utility/preprocess/docs2passages.py b/PT-Retrieval/colbert/utility/preprocess/docs2passages.py
new file mode 100644
index 0000000..0db8e93
--- /dev/null
+++ b/PT-Retrieval/colbert/utility/preprocess/docs2passages.py
@@ -0,0 +1,149 @@
+"""
+ Divide a document collection into N-word/token passage spans (with wrap-around for last passage).
+"""
+
+import os
+import math
+import ujson
+import random
+
+from multiprocessing import Pool
+from argparse import ArgumentParser
+from colbert.utils.utils import print_message
+
+Format1 = 'docid,text' # MS MARCO Passages
+Format2 = 'docid,text,title' # DPR Wikipedia
+Format3 = 'docid,url,title,text' # MS MARCO Documents
+
+
+def process_page(inp):
+ """
+ Wraps around if we split: make sure last passage isn't too short.
+ This is meant to be similar to the DPR preprocessing.
+ """
+
+ (nwords, overlap, tokenizer), (title_idx, docid, title, url, content) = inp
+
+ if tokenizer is None:
+ words = content.split()
+ else:
+ words = tokenizer.tokenize(content)
+
+ words_ = (words + words) if len(words) > nwords else words
+ passages = [words_[offset:offset + nwords] for offset in range(0, len(words) - overlap, nwords - overlap)]
+
+ assert all(len(psg) in [len(words), nwords] for psg in passages), (list(map(len, passages)), len(words))
+
+ if tokenizer is None:
+ passages = [' '.join(psg) for psg in passages]
+ else:
+ passages = [' '.join(psg).replace(' ##', '') for psg in passages]
+
+ if title_idx % 100000 == 0:
+ print("#> ", title_idx, '\t\t\t', title)
+
+ for p in passages:
+ print("$$$ ", '\t\t', p)
+ print()
+
+ print()
+ print()
+ print()
+
+ return (docid, title, url, passages)
+
+
+def main(args):
+ random.seed(12345)
+ print_message("#> Starting...")
+
+ letter = 'w' if not args.use_wordpiece else 't'
+ output_path = f'{args.input}.{letter}{args.nwords}_{args.overlap}'
+ assert not os.path.exists(output_path)
+
+ RawCollection = []
+ Collection = []
+
+ NumIllFormattedLines = 0
+
+ with open(args.input) as f:
+ for line_idx, line in enumerate(f):
+ if line_idx % (100*1000) == 0:
+ print(line_idx, end=' ')
+
+ title, url = None, None
+
+ try:
+ line = line.strip().split('\t')
+
+ if args.format == Format1:
+ docid, doc = line
+ elif args.format == Format2:
+ docid, doc, title = line
+ elif args.format == Format3:
+ docid, url, title, doc = line
+
+ RawCollection.append((line_idx, docid, title, url, doc))
+ except:
+ NumIllFormattedLines += 1
+
+ if NumIllFormattedLines % 1000 == 0:
+ print(f'\n[{line_idx}] NumIllFormattedLines = {NumIllFormattedLines}\n')
+
+ print()
+ print_message("# of documents is", len(RawCollection), '\n')
+
+ p = Pool(args.nthreads)
+
+ print_message("#> Starting parallel processing...")
+
+ tokenizer = None
+ if args.use_wordpiece:
+ from transformers import BertTokenizerFast
+ tokenizer = BertTokenizerFast.from_pretrained('bert-base-uncased')
+
+ process_page_params = [(args.nwords, args.overlap, tokenizer)] * len(RawCollection)
+ Collection = p.map(process_page, zip(process_page_params, RawCollection))
+
+ print_message(f"#> Writing to {output_path} ...")
+ with open(output_path, 'w') as f:
+ line_idx = 1
+
+ if args.format == Format1:
+ f.write('\t'.join(['id', 'text']) + '\n')
+ elif args.format == Format2:
+ f.write('\t'.join(['id', 'text', 'title']) + '\n')
+ elif args.format == Format3:
+ f.write('\t'.join(['id', 'text', 'title', 'docid']) + '\n')
+
+ for docid, title, url, passages in Collection:
+ for passage in passages:
+ if args.format == Format1:
+ f.write('\t'.join([str(line_idx), passage]) + '\n')
+ elif args.format == Format2:
+ f.write('\t'.join([str(line_idx), passage, title]) + '\n')
+ elif args.format == Format3:
+ f.write('\t'.join([str(line_idx), passage, title, docid]) + '\n')
+
+ line_idx += 1
+
+
+if __name__ == "__main__":
+ parser = ArgumentParser(description="docs2passages.")
+
+ # Input Arguments.
+ parser.add_argument('--input', dest='input', required=True)
+ parser.add_argument('--format', dest='format', required=True, choices=[Format1, Format2, Format3])
+
+ # Output Arguments.
+ parser.add_argument('--use-wordpiece', dest='use_wordpiece', default=False, action='store_true')
+ parser.add_argument('--nwords', dest='nwords', default=100, type=int)
+ parser.add_argument('--overlap', dest='overlap', default=0, type=int)
+
+ # Other Arguments.
+ parser.add_argument('--nthreads', dest='nthreads', default=28, type=int)
+
+ args = parser.parse_args()
+ assert args.nwords in range(50, 500)
+
+ main(args)
diff --git a/PT-Retrieval/colbert/utility/preprocess/queries_split.py b/PT-Retrieval/colbert/utility/preprocess/queries_split.py
new file mode 100644
index 0000000..09c866a
--- /dev/null
+++ b/PT-Retrieval/colbert/utility/preprocess/queries_split.py
@@ -0,0 +1,81 @@
+"""
+ Divide a query set into two.
+"""
+
+import os
+import math
+import ujson
+import random
+
+from argparse import ArgumentParser
+from collections import OrderedDict
+from colbert.utils.utils import print_message
+
+
+def main(args):
+ random.seed(12345)
+
+ """
+ Load the queries
+ """
+ Queries = OrderedDict()
+
+ print_message(f"#> Loading queries from {args.input}..")
+ with open(args.input) as f:
+ for line in f:
+ qid, query = line.strip().split('\t')
+
+ assert qid not in Queries
+ Queries[qid] = query
+
+ """
+ Apply the splitting
+ """
+ size_a = len(Queries) - args.holdout
+ size_b = args.holdout
+ size_a, size_b = max(size_a, size_b), min(size_a, size_b)
+
+ assert size_a > 0 and size_b > 0, (len(Queries), size_a, size_b)
+
+ print_message(f"#> Deterministically splitting the queries into ({size_a}, {size_b})-sized splits.")
+
+ keys = list(Queries.keys())
+ sample_b_indices = sorted(list(random.sample(range(len(keys)), size_b)))
+ sample_a_indices = sorted(list(set.difference(set(list(range(len(keys)))), set(sample_b_indices))))
+
+ assert len(sample_a_indices) == size_a
+ assert len(sample_b_indices) == size_b
+
+ sample_a = [keys[idx] for idx in sample_a_indices]
+ sample_b = [keys[idx] for idx in sample_b_indices]
+
+ """
+ Write the output
+ """
+
+ output_path_a = f'{args.input}.a'
+ output_path_b = f'{args.input}.b'
+
+ assert not os.path.exists(output_path_a), output_path_a
+ assert not os.path.exists(output_path_b), output_path_b
+
+ print_message(f"#> Writing the splits out to {output_path_a} and {output_path_b} ...")
+
+ for output_path, sample in [(output_path_a, sample_a), (output_path_b, sample_b)]:
+ with open(output_path, 'w') as f:
+ for qid in sample:
+ query = Queries[qid]
+ line = '\t'.join([qid, query]) + '\n'
+ f.write(line)
+
+
+if __name__ == "__main__":
+ parser = ArgumentParser(description="queries_split.")
+
+ # Input Arguments.
+ parser.add_argument('--input', dest='input', required=True)
+ parser.add_argument('--holdout', dest='holdout', required=True, type=int)
+
+ args = parser.parse_args()
+
+ main(args)
diff --git a/PT-Retrieval/colbert/utility/preprocess/wikipedia_to_tsv.py b/PT-Retrieval/colbert/utility/preprocess/wikipedia_to_tsv.py
new file mode 100644
index 0000000..34cec87
--- /dev/null
+++ b/PT-Retrieval/colbert/utility/preprocess/wikipedia_to_tsv.py
@@ -0,0 +1,63 @@
+import os
+import ujson
+
+from argparse import ArgumentParser
+from colbert.utils.utils import print_message
+
+
+def main(args):
+ input_path = args.input
+ output_path = args.output
+
+ assert not os.path.exists(output_path), output_path
+
+ RawCollection = []
+
+ walk = [(dirpath, filenames) for dirpath, _, filenames in os.walk(input_path)]
+ walk = sorted(walk)
+
+ for dirpath, filenames in walk:
+ print_message(f"#> Visiting {dirpath}")
+
+ for filename in sorted(filenames):
+ assert 'wiki_' in filename, (dirpath, filename)
+ filename = os.path.join(dirpath, filename)
+
+ print_message(f"#> Opening {filename} --- so far collected {len(RawCollection)} pages/passages")
+ with open(filename) as f:
+ for line in f:
+ RawCollection.append(ujson.loads(line))
+
+ with open(output_path, 'w') as f:
+ #line = '\t'.join(map(str, ['id', 'text', 'title'])) + '\n'
+ #f.write(line)
+
+ PID = 1
+
+ for doc in RawCollection:
+ title, text = doc['title'], doc['text']
+
+ # Join sentences and clean text
+ text = ' '.join(text.split())
+
+ if args.keep_empty_pages or len(text) > 0:
+ line = '\t'.join(map(str, [PID, text, title])) + '\n'
+ f.write(line)
+
+ PID += 1
+
+ print_message("#> All done.")
+
+
+if __name__ == "__main__":
+ parser = ArgumentParser(description="docs2passages.")
+
+ # Input Arguments.
+ parser.add_argument('--input', dest='input', required=True)
+ parser.add_argument('--output', dest='output', required=True)
+
+ parser.add_argument('--keep-empty-pages', dest='keep_empty_pages', default=False, action='store_true')
+
+ args = parser.parse_args()
+
+ main(args)
diff --git a/PT-Retrieval/colbert/utility/rankings/dev_subsample.py b/PT-Retrieval/colbert/utility/rankings/dev_subsample.py
new file mode 100644
index 0000000..a928fe8
--- /dev/null
+++ b/PT-Retrieval/colbert/utility/rankings/dev_subsample.py
@@ -0,0 +1,47 @@
+import os
+import ujson
+import random
+
+from argparse import ArgumentParser
+
+from colbert.utils.utils import print_message, create_directory, load_ranking, groupby_first_item
+from utility.utils.qa_loaders import load_qas_
+
+
+def main(args):
+ print_message("#> Loading all..")
+ qas = load_qas_(args.qas)
+ rankings = load_ranking(args.ranking)
+ qid2rankings = groupby_first_item(rankings)
+
+ print_message("#> Subsampling all..")
+ qas_sample = random.sample(qas, args.sample)
+
+ with open(args.output, 'w') as f:
+ for qid, *_ in qas_sample:
+ for items in qid2rankings[qid]:
+ items = [qid] + items
+ line = '\t'.join(map(str, items)) + '\n'
+ f.write(line)
+
+ print('\n\n')
+ print(args.output)
+ print("#> Done.")
+
+
+if __name__ == "__main__":
+ random.seed(12345)
+
+ parser = ArgumentParser(description='Subsample the dev set.')
+ parser.add_argument('--qas', dest='qas', required=True, type=str)
+ parser.add_argument('--ranking', dest='ranking', required=True)
+ parser.add_argument('--output', dest='output', required=True)
+
+ parser.add_argument('--sample', dest='sample', default=1500, type=int)
+
+ args = parser.parse_args()
+
+ assert not os.path.exists(args.output), args.output
+ create_directory(os.path.dirname(args.output))
+
+ main(args)
diff --git a/PT-Retrieval/colbert/utility/rankings/merge.py b/PT-Retrieval/colbert/utility/rankings/merge.py
new file mode 100644
index 0000000..44b555a
--- /dev/null
+++ b/PT-Retrieval/colbert/utility/rankings/merge.py
@@ -0,0 +1,57 @@
+"""
+ Divide two or more ranking files, by score.
+"""
+
+import os
+import tqdm
+
+from argparse import ArgumentParser
+from collections import defaultdict
+from colbert.utils.utils import print_message, file_tqdm
+
+
+def main(args):
+ Rankings = defaultdict(list)
+
+ for path in args.input:
+ print_message(f"#> Loading the rankings in {path} ..")
+
+ with open(path) as f:
+ for line in file_tqdm(f):
+ qid, pid, rank, score = line.strip().split('\t')
+ qid, pid, rank = map(int, [qid, pid, rank])
+ score = float(score)
+
+ Rankings[qid].append((score, rank, pid))
+
+ with open(args.output, 'w') as f:
+ print_message(f"#> Writing the output rankings to {args.output} ..")
+
+ for qid in tqdm.tqdm(Rankings):
+ ranking = sorted(Rankings[qid], reverse=True)
+
+ for rank, (score, original_rank, pid) in enumerate(ranking):
+ rank = rank + 1 # 1-indexed
+
+ if (args.depth > 0) and (rank > args.depth):
+ break
+
+ line = [qid, pid, rank, score]
+ line = '\t'.join(map(str, line)) + '\n'
+ f.write(line)
+
+
+if __name__ == "__main__":
+ parser = ArgumentParser(description="merge_rankings.")
+
+ # Input Arguments.
+ parser.add_argument('--input', dest='input', required=True, nargs='+')
+ parser.add_argument('--output', dest='output', required=True, type=str)
+
+ parser.add_argument('--depth', dest='depth', required=True, type=int)
+
+ args = parser.parse_args()
+
+ assert not os.path.exists(args.output), args.output
+
+ main(args)
diff --git a/PT-Retrieval/colbert/utility/rankings/split_by_offset.py b/PT-Retrieval/colbert/utility/rankings/split_by_offset.py
new file mode 100644
index 0000000..9d2e8cf
--- /dev/null
+++ b/PT-Retrieval/colbert/utility/rankings/split_by_offset.py
@@ -0,0 +1,44 @@
+"""
+Split the ranked lists after retrieval with a merged query set.
+"""
+
+import os
+import random
+
+from argparse import ArgumentParser
+
+
+def main(args):
+ output_paths = ['{}.{}'.format(args.ranking, split) for split in args.names]
+ assert all(not os.path.exists(path) for path in output_paths), output_paths
+
+ output_files = [open(path, 'w') for path in output_paths]
+
+ with open(args.ranking) as f:
+ for line in f:
+ qid, pid, rank, *other = line.strip().split('\t')
+ qid = int(qid)
+ split_output_path = output_files[qid // args.gap - 1]
+ qid = qid % args.gap
+
+ split_output_path.write('\t'.join([str(x) for x in [qid, pid, rank, *other]]) + '\n')
+
+ print(f.name)
+
+ _ = [f.close() for f in output_files]
+
+ print("#> Done!")
+
+
+if __name__ == "__main__":
+ random.seed(12345)
+
+ parser = ArgumentParser(description='Subsample the dev set.')
+ parser.add_argument('--ranking', dest='ranking', required=True)
+
+ parser.add_argument('--names', dest='names', required=False, default=['train', 'dev', 'test'], type=str, nargs='+') # order matters!
+ parser.add_argument('--gap', dest='gap', required=False, default=1_000_000_000, type=int) # larger than any individual query set
+
+ args = parser.parse_args()
+
+ main(args)
diff --git a/PT-Retrieval/colbert/utility/rankings/split_by_queries.py b/PT-Retrieval/colbert/utility/rankings/split_by_queries.py
new file mode 100644
index 0000000..f052a7c
--- /dev/null
+++ b/PT-Retrieval/colbert/utility/rankings/split_by_queries.py
@@ -0,0 +1,67 @@
+import os
+import sys
+import tqdm
+import ujson
+import random
+
+from argparse import ArgumentParser
+from collections import OrderedDict
+from colbert.utils.utils import print_message, file_tqdm
+
+
+def main(args):
+ qid_to_file_idx = {}
+
+ for qrels_idx, qrels in enumerate(args.all_queries):
+ with open(qrels) as f:
+ for line in f:
+ qid, *_ = line.strip().split('\t')
+ qid = int(qid)
+
+ assert qid_to_file_idx.get(qid, qrels_idx) == qrels_idx, (qid, qrels_idx)
+ qid_to_file_idx[qid] = qrels_idx
+
+ all_outputs_paths = [f'{args.ranking}.{idx}' for idx in range(len(args.all_queries))]
+
+ assert all(not os.path.exists(path) for path in all_outputs_paths)
+
+ all_outputs = [open(path, 'w') for path in all_outputs_paths]
+
+ with open(args.ranking) as f:
+ print_message(f"#> Loading ranked lists from {f.name} ..")
+
+ last_file_idx = -1
+
+ for line in file_tqdm(f):
+ qid, *_ = line.strip().split('\t')
+
+ file_idx = qid_to_file_idx[int(qid)]
+
+ if file_idx != last_file_idx:
+ print_message(f"#> Switched to file #{file_idx} at {all_outputs[file_idx].name}")
+
+ last_file_idx = file_idx
+
+ all_outputs[file_idx].write(line)
+
+ print()
+
+ for f in all_outputs:
+ print(f.name)
+ f.close()
+
+ print("#> Done!")
+
+
+if __name__ == "__main__":
+ random.seed(12345)
+
+ parser = ArgumentParser(description='.')
+
+ # Input Arguments
+ parser.add_argument('--ranking', dest='ranking', required=True, type=str)
+ parser.add_argument('--all-queries', dest='all_queries', required=True, type=str, nargs='+')
+
+ args = parser.parse_args()
+
+ main(args)
diff --git a/PT-Retrieval/colbert/utility/rankings/tune.py b/PT-Retrieval/colbert/utility/rankings/tune.py
new file mode 100644
index 0000000..035d1c0
--- /dev/null
+++ b/PT-Retrieval/colbert/utility/rankings/tune.py
@@ -0,0 +1,66 @@
+import os
+import ujson
+import random
+
+from argparse import ArgumentParser
+from colbert.utils.utils import print_message, create_directory
+from utility.utils.save_metadata import save_metadata
+
+
+def main(args):
+ AllMetrics = {}
+ Scores = {}
+
+ for path in args.paths:
+ with open(path) as f:
+ metric = ujson.load(f)
+ AllMetrics[path] = metric
+
+ for k in args.metric:
+ metric = metric[k]
+
+ assert type(metric) is float
+ Scores[path] = metric
+
+ MaxKey = max(Scores, key=Scores.get)
+
+ MaxCKPT = int(MaxKey.split('/')[-2].split('.')[-1])
+ MaxARGS = os.path.join(os.path.dirname(MaxKey), 'logs', 'args.json')
+
+ with open(MaxARGS) as f:
+ logs = ujson.load(f)
+ MaxCHECKPOINT = logs['checkpoint']
+
+ assert MaxCHECKPOINT.endswith(f'colbert-{MaxCKPT}.dnn'), (MaxCHECKPOINT, MaxCKPT)
+
+ with open(args.output, 'w') as f:
+ f.write(MaxCHECKPOINT)
+
+ args.Scores = Scores
+ args.AllMetrics = AllMetrics
+
+ save_metadata(f'{args.output}.meta', args)
+
+ print('\n\n', args, '\n\n')
+ print(args.output)
+ print_message("#> Done.")
+
+
+if __name__ == "__main__":
+ random.seed(12345)
+
+ parser = ArgumentParser(description='.')
+
+ # Input / Output Arguments
+ parser.add_argument('--metric', dest='metric', required=True, type=str) # e.g., success.20
+ parser.add_argument('--paths', dest='paths', required=True, type=str, nargs='+')
+ parser.add_argument('--output', dest='output', required=True, type=str)
+
+ args = parser.parse_args()
+
+ args.metric = args.metric.split('.')
+
+ assert not os.path.exists(args.output), args.output
+ create_directory(os.path.dirname(args.output))
+
+ main(args)
diff --git a/PT-Retrieval/colbert/utility/supervision/self_training.py b/PT-Retrieval/colbert/utility/supervision/self_training.py
new file mode 100644
index 0000000..814e4d3
--- /dev/null
+++ b/PT-Retrieval/colbert/utility/supervision/self_training.py
@@ -0,0 +1,123 @@
+import os
+import sys
+import git
+import tqdm
+import ujson
+import random
+
+from argparse import ArgumentParser
+from colbert.utils.utils import print_message, load_ranking, groupby_first_item
+
+
+MAX_NUM_TRIPLES = 40_000_000
+
+
+def sample_negatives(negatives, num_sampled, biased=False):
+ num_sampled = min(len(negatives), num_sampled)
+
+ if biased:
+ assert num_sampled % 2 == 0
+ num_sampled_top100 = num_sampled // 2
+ num_sampled_rest = num_sampled - num_sampled_top100
+
+ return random.sample(negatives[:100], num_sampled_top100) + random.sample(negatives[100:], num_sampled_rest)
+
+ return random.sample(negatives, num_sampled)
+
+
+def sample_for_query(qid, ranking, npositives, depth_positive, depth_negative, cutoff_negative):
+ """
+ Requires that the ranks are sorted per qid.
+ """
+ assert npositives <= depth_positive < cutoff_negative < depth_negative
+
+ positives, negatives, triples = [], [], []
+
+ for pid, rank, *_ in ranking:
+ assert rank >= 1, f"ranks should start at 1 \t\t got rank = {rank}"
+
+ if rank > depth_negative:
+ break
+
+ if rank <= depth_positive:
+ positives.append(pid)
+ elif rank > cutoff_negative:
+ negatives.append(pid)
+
+ num_sampled = 100
+
+ for neg in sample_negatives(negatives, num_sampled):
+ positives_ = random.sample(positives, npositives)
+ positives_ = positives_[0] if npositives == 1 else positives_
+ triples.append((qid, positives_, neg))
+
+ return triples
+
+
+def main(args):
+ rankings = load_ranking(args.ranking, types=[int, int, int, float, int])
+
+ print_message("#> Group by QID")
+ qid2rankings = groupby_first_item(tqdm.tqdm(rankings))
+
+ Triples = []
+ NonEmptyQIDs = 0
+
+ for processing_idx, qid in enumerate(qid2rankings):
+ l = sample_for_query(qid, qid2rankings[qid], args.positives, args.depth_positive, args.depth_negative, args.cutoff_negative)
+ NonEmptyQIDs += (len(l) > 0)
+ Triples.extend(l)
+
+ if processing_idx % (10_000) == 0:
+ print_message(f"#> Done with {processing_idx+1} questions!\t\t "
+ f"{str(len(Triples) / 1000)}k triples for {NonEmptyQIDs} unqiue QIDs.")
+
+ print_message(f"#> Sub-sample the triples (if > {MAX_NUM_TRIPLES})..")
+ print_message(f"#> len(Triples) = {len(Triples)}")
+
+ if len(Triples) > MAX_NUM_TRIPLES:
+ Triples = random.sample(Triples, MAX_NUM_TRIPLES)
+
+ ### Prepare the triples ###
+ print_message("#> Shuffling the triples...")
+ random.shuffle(Triples)
+
+ print_message("#> Writing {}M examples to file.".format(len(Triples) / 1000.0 / 1000.0))
+
+ with open(args.output, 'w') as f:
+ for example in Triples:
+ ujson.dump(example, f)
+ f.write('\n')
+
+ with open(f'{args.output}.meta', 'w') as f:
+ args.cmd = ' '.join(sys.argv)
+ args.git_hash = git.Repo(search_parent_directories=True).head.object.hexsha
+ ujson.dump(args.__dict__, f, indent=4)
+ f.write('\n')
+
+ print('\n\n', args, '\n\n')
+ print(args.output)
+ print_message("#> Done.")
+
+
+if __name__ == "__main__":
+ random.seed(12345)
+
+ parser = ArgumentParser(description='Create training triples from ranked list.')
+
+ # Input / Output Arguments
+ parser.add_argument('--ranking', dest='ranking', required=True, type=str)
+ parser.add_argument('--output', dest='output', required=True, type=str)
+
+ # Weak Supervision Arguments.
+ parser.add_argument('--positives', dest='positives', required=True, type=int)
+ parser.add_argument('--depth+', dest='depth_positive', required=True, type=int)
+
+ parser.add_argument('--depth-', dest='depth_negative', required=True, type=int)
+ parser.add_argument('--cutoff-', dest='cutoff_negative', required=True, type=int)
+
+ args = parser.parse_args()
+
+ assert not os.path.exists(args.output), args.output
+
+ main(args)
diff --git a/PT-Retrieval/colbert/utility/supervision/triples.py b/PT-Retrieval/colbert/utility/supervision/triples.py
new file mode 100644
index 0000000..b70c2c0
--- /dev/null
+++ b/PT-Retrieval/colbert/utility/supervision/triples.py
@@ -0,0 +1,150 @@
+"""
+ Example: --positives 5,50 1,1000 ~~> best-5 (in top-50) + best-1 (in top-1000)
+"""
+
+import os
+import sys
+import git
+import tqdm
+import ujson
+import random
+
+from argparse import ArgumentParser
+from colbert.utils.utils import print_message, load_ranking, groupby_first_item, create_directory
+from utility.utils.save_metadata import save_metadata
+
+
+MAX_NUM_TRIPLES = 40_000_000
+
+
+def sample_negatives(negatives, num_sampled, biased=None):
+ assert biased in [None, 100, 200], "NOTE: We bias 50% from the top-200 negatives, if there are twice or more."
+
+ num_sampled = min(len(negatives), num_sampled)
+
+ if biased and num_sampled < len(negatives):
+ assert num_sampled % 2 == 0, num_sampled
+
+ num_sampled_top100 = num_sampled // 2
+ num_sampled_rest = num_sampled - num_sampled_top100
+
+ oversampled, undersampled = negatives[:biased], negatives[biased:]
+
+ if len(oversampled) < len(undersampled):
+ return random.sample(oversampled, num_sampled_top100) + random.sample(undersampled, num_sampled_rest)
+
+ return random.sample(negatives, num_sampled)
+
+
+def sample_for_query(qid, ranking, args_positives, depth, permissive, biased):
+ """
+ Requires that the ranks are sorted per qid.
+ """
+
+ positives, negatives, triples = [], [], []
+
+ for pid, rank, *_, label in ranking:
+ assert rank >= 1, f"ranks should start at 1 \t\t got rank = {rank}"
+ assert label in [0, 1]
+
+ if rank > depth:
+ break
+
+ if label:
+ take_this_positive = any(rank <= maxDepth and len(positives) < maxBest for maxBest, maxDepth in args_positives)
+
+ if take_this_positive:
+ positives.append((pid, 0))
+ elif permissive:
+ positives.append((pid, rank)) # utilize with a few negatives, starting at (next) rank
+
+ else:
+ negatives.append(pid)
+
+ for pos, neg_start in positives:
+ num_sampled = 100 if neg_start == 0 else 5
+ negatives_ = negatives[neg_start:]
+
+ biased_ = biased if neg_start == 0 else None
+ for neg in sample_negatives(negatives_, num_sampled, biased=biased_):
+ triples.append((qid, pos, neg))
+
+ return triples
+
+
+def main(args):
+ try:
+ rankings = load_ranking(args.ranking, types=[int, int, int, float, int])
+ except:
+ rankings = load_ranking(args.ranking, types=[int, int, int, int])
+
+ print_message("#> Group by QID")
+ qid2rankings = groupby_first_item(tqdm.tqdm(rankings))
+
+ Triples = []
+ NonEmptyQIDs = 0
+
+ for processing_idx, qid in enumerate(qid2rankings):
+ l = sample_for_query(qid, qid2rankings[qid], args.positives, args.depth, args.permissive, args.biased)
+ NonEmptyQIDs += (len(l) > 0)
+ Triples.extend(l)
+
+ if processing_idx % (10_000) == 0:
+ print_message(f"#> Done with {processing_idx+1} questions!\t\t "
+ f"{str(len(Triples) / 1000)}k triples for {NonEmptyQIDs} unqiue QIDs.")
+
+ print_message(f"#> Sub-sample the triples (if > {MAX_NUM_TRIPLES})..")
+ print_message(f"#> len(Triples) = {len(Triples)}")
+
+ if len(Triples) > MAX_NUM_TRIPLES:
+ Triples = random.sample(Triples, MAX_NUM_TRIPLES)
+
+ ### Prepare the triples ###
+ print_message("#> Shuffling the triples...")
+ random.shuffle(Triples)
+
+ print_message("#> Writing {}M examples to file.".format(len(Triples) / 1000.0 / 1000.0))
+
+ with open(args.output, 'w') as f:
+ for example in Triples:
+ ujson.dump(example, f)
+ f.write('\n')
+
+ save_metadata(f'{args.output}.meta', args)
+
+ print('\n\n', args, '\n\n')
+ print(args.output)
+ print_message("#> Done.")
+
+
+if __name__ == "__main__":
+ parser = ArgumentParser(description='Create training triples from ranked list.')
+
+ # Input / Output Arguments
+ parser.add_argument('--ranking', dest='ranking', required=True, type=str)
+ parser.add_argument('--output', dest='output', required=True, type=str)
+
+ # Weak Supervision Arguments.
+ parser.add_argument('--positives', dest='positives', required=True, nargs='+')
+ parser.add_argument('--depth', dest='depth', required=True, type=int) # for negatives
+
+ parser.add_argument('--permissive', dest='permissive', default=False, action='store_true')
+ # parser.add_argument('--biased', dest='biased', default=False, action='store_true')
+ parser.add_argument('--biased', dest='biased', default=None, type=int)
+ parser.add_argument('--seed', dest='seed', required=False, default=12345, type=int)
+
+ args = parser.parse_args()
+ random.seed(args.seed)
+
+ assert not os.path.exists(args.output), args.output
+
+ args.positives = [list(map(int, configuration.split(','))) for configuration in args.positives]
+
+ assert all(len(x) == 2 for x in args.positives)
+ assert all(maxBest <= maxDepth for maxBest, maxDepth in args.positives), args.positives
+
+ create_directory(os.path.dirname(args.output))
+
+ assert args.biased in [None, 100, 200]
+
+ main(args)
diff --git a/PT-Retrieval/colbert/utility/utils/dpr.py b/PT-Retrieval/colbert/utility/utils/dpr.py
new file mode 100644
index 0000000..f268d85
--- /dev/null
+++ b/PT-Retrieval/colbert/utility/utils/dpr.py
@@ -0,0 +1,237 @@
+"""
+ Source: DPR Implementation from Facebook Research
+ https://github.com/facebookresearch/DPR/tree/master/dpr
+"""
+
+import string
+import spacy
+import regex
+import unicodedata
+
+
+class Tokens(object):
+ """A class to represent a list of tokenized text."""
+ TEXT = 0
+ TEXT_WS = 1
+ SPAN = 2
+ POS = 3
+ LEMMA = 4
+ NER = 5
+
+ def __init__(self, data, annotators, opts=None):
+ self.data = data
+ self.annotators = annotators
+ self.opts = opts or {}
+
+ def __len__(self):
+ """The number of tokens."""
+ return len(self.data)
+
+ def slice(self, i=None, j=None):
+ """Return a view of the list of tokens from [i, j)."""
+ new_tokens = copy.copy(self)
+ new_tokens.data = self.data[i: j]
+ return new_tokens
+
+ def untokenize(self):
+ """Returns the original text (with whitespace reinserted)."""
+ return ''.join([t[self.TEXT_WS] for t in self.data]).strip()
+
+ def words(self, uncased=False):
+ """Returns a list of the text of each token
+
+ Args:
+ uncased: lower cases text
+ """
+ if uncased:
+ return [t[self.TEXT].lower() for t in self.data]
+ else:
+ return [t[self.TEXT] for t in self.data]
+
+ def offsets(self):
+ """Returns a list of [start, end) character offsets of each token."""
+ return [t[self.SPAN] for t in self.data]
+
+ def pos(self):
+ """Returns a list of part-of-speech tags of each token.
+ Returns None if this annotation was not included.
+ """
+ if 'pos' not in self.annotators:
+ return None
+ return [t[self.POS] for t in self.data]
+
+ def lemmas(self):
+ """Returns a list of the lemmatized text of each token.
+ Returns None if this annotation was not included.
+ """
+ if 'lemma' not in self.annotators:
+ return None
+ return [t[self.LEMMA] for t in self.data]
+
+ def entities(self):
+ """Returns a list of named-entity-recognition tags of each token.
+ Returns None if this annotation was not included.
+ """
+ if 'ner' not in self.annotators:
+ return None
+ return [t[self.NER] for t in self.data]
+
+ def ngrams(self, n=1, uncased=False, filter_fn=None, as_strings=True):
+ """Returns a list of all ngrams from length 1 to n.
+
+ Args:
+ n: upper limit of ngram length
+ uncased: lower cases text
+ filter_fn: user function that takes in an ngram list and returns
+ True or False to keep or not keep the ngram
+ as_string: return the ngram as a string vs list
+ """
+
+ def _skip(gram):
+ if not filter_fn:
+ return False
+ return filter_fn(gram)
+
+ words = self.words(uncased)
+ ngrams = [(s, e + 1)
+ for s in range(len(words))
+ for e in range(s, min(s + n, len(words)))
+ if not _skip(words[s:e + 1])]
+
+ # Concatenate into strings
+ if as_strings:
+ ngrams = ['{}'.format(' '.join(words[s:e])) for (s, e) in ngrams]
+
+ return ngrams
+
+ def entity_groups(self):
+ """Group consecutive entity tokens with the same NER tag."""
+ entities = self.entities()
+ if not entities:
+ return None
+ non_ent = self.opts.get('non_ent', 'O')
+ groups = []
+ idx = 0
+ while idx < len(entities):
+ ner_tag = entities[idx]
+ # Check for entity tag
+ if ner_tag != non_ent:
+ # Chomp the sequence
+ start = idx
+ while (idx < len(entities) and entities[idx] == ner_tag):
+ idx += 1
+ groups.append((self.slice(start, idx).untokenize(), ner_tag))
+ else:
+ idx += 1
+ return groups
+
+
+class Tokenizer(object):
+ """Base tokenizer class.
+ Tokenizers implement tokenize, which should return a Tokens class.
+ """
+
+ def tokenize(self, text):
+ raise NotImplementedError
+
+ def shutdown(self):
+ pass
+
+ def __del__(self):
+ self.shutdown()
+
+
+class SimpleTokenizer(Tokenizer):
+ ALPHA_NUM = r'[\p{L}\p{N}\p{M}]+'
+ NON_WS = r'[^\p{Z}\p{C}]'
+
+ def __init__(self, **kwargs):
+ """
+ Args:
+ annotators: None or empty set (only tokenizes).
+ """
+ self._regexp = regex.compile(
+ '(%s)|(%s)' % (self.ALPHA_NUM, self.NON_WS),
+ flags=regex.IGNORECASE + regex.UNICODE + regex.MULTILINE
+ )
+ if len(kwargs.get('annotators', {})) > 0:
+ logger.warning('%s only tokenizes! Skipping annotators: %s' %
+ (type(self).__name__, kwargs.get('annotators')))
+ self.annotators = set()
+
+ def tokenize(self, text):
+ data = []
+ matches = [m for m in self._regexp.finditer(text)]
+ for i in range(len(matches)):
+ # Get text
+ token = matches[i].group()
+
+ # Get whitespace
+ span = matches[i].span()
+ start_ws = span[0]
+ if i + 1 < len(matches):
+ end_ws = matches[i + 1].span()[0]
+ else:
+ end_ws = span[1]
+
+ # Format data
+ data.append((
+ token,
+ text[start_ws: end_ws],
+ span,
+ ))
+ return Tokens(data, self.annotators)
+
+
+def has_answer(tokenized_answers, text):
+ text = DPR_normalize(text)
+
+ for single_answer in tokenized_answers:
+ for i in range(0, len(text) - len(single_answer) + 1):
+ if single_answer == text[i: i + len(single_answer)]:
+ return True
+
+ return False
+
+
+def locate_answers(tokenized_answers, text):
+ """
+ Returns each occurrence of an answer as (offset, endpos) in terms of *characters*.
+ """
+ tokenized_text = DPR_tokenize(text)
+ occurrences = []
+
+ text_words, text_word_positions = tokenized_text.words(uncased=True), tokenized_text.offsets()
+ answers_words = [ans.words(uncased=True) for ans in tokenized_answers]
+
+ for single_answer in answers_words:
+ for i in range(0, len(text_words) - len(single_answer) + 1):
+ if single_answer == text_words[i: i + len(single_answer)]:
+ (offset, _), (_, endpos) = text_word_positions[i], text_word_positions[i+len(single_answer)-1]
+ occurrences.append((offset, endpos))
+
+ return occurrences
+
+
+STokenizer = SimpleTokenizer()
+
+
+def DPR_tokenize(text):
+ return STokenizer.tokenize(unicodedata.normalize('NFD', text))
+
+
+def DPR_normalize(text):
+ return DPR_tokenize(text).words(uncased=True)
+
+
+# Source: https://github.com/shmsw25/qa-hard-em/blob/master/prepro_util.py
+def strip_accents(text):
+ """Strips accents from a piece of text."""
+ text = unicodedata.normalize("NFD", text)
+ output = []
+ for char in text:
+ cat = unicodedata.category(char)
+ if cat == "Mn":
+ continue
+ output.append(char)
+ return "".join(output)
diff --git a/PT-Retrieval/colbert/utility/utils/qa_loaders.py b/PT-Retrieval/colbert/utility/utils/qa_loaders.py
new file mode 100644
index 0000000..06565a5
--- /dev/null
+++ b/PT-Retrieval/colbert/utility/utils/qa_loaders.py
@@ -0,0 +1,33 @@
+import os
+import ujson
+
+from collections import defaultdict
+from colbert.utils.utils import print_message, file_tqdm
+
+
+def load_collection_(path, retain_titles):
+ with open(path) as f:
+ collection = []
+
+ for line in file_tqdm(f):
+ _, passage, title = line.strip().split('\t')
+
+ if retain_titles:
+ passage = title + ' | ' + passage
+
+ collection.append(passage)
+
+ return collection
+
+
+def load_qas_(path):
+ print_message("#> Loading the reference QAs from", path)
+
+ triples = []
+
+ with open(path) as f:
+ for line in f:
+ qa = ujson.loads(line)
+ triples.append((qa['qid'], qa['question'], qa['answers']))
+
+ return triples
diff --git a/PT-Retrieval/colbert/utility/utils/save_metadata.py b/PT-Retrieval/colbert/utility/utils/save_metadata.py
new file mode 100644
index 0000000..0103f18
--- /dev/null
+++ b/PT-Retrieval/colbert/utility/utils/save_metadata.py
@@ -0,0 +1,41 @@
+import os
+import sys
+import git
+import time
+import copy
+import ujson
+import socket
+
+
+def get_metadata(args):
+ args = copy.deepcopy(args)
+
+ args.hostname = socket.gethostname()
+ args.git_branch = git.Repo(search_parent_directories=True).active_branch.name
+ args.git_hash = git.Repo(search_parent_directories=True).head.object.hexsha
+ args.git_commit_datetime = str(git.Repo(search_parent_directories=True).head.object.committed_datetime)
+ args.current_datetime = time.strftime('%b %d, %Y ; %l:%M%p %Z (%z)')
+ args.cmd = ' '.join(sys.argv)
+
+ try:
+ args.input_arguments = copy.deepcopy(args.input_arguments.__dict__)
+ except:
+ args.input_arguments = None
+
+ return dict(args.__dict__)
+
+
+def format_metadata(metadata):
+ assert type(metadata) == dict
+
+ return ujson.dumps(metadata, indent=4)
+
+
+def save_metadata(path, args):
+ assert not os.path.exists(path), path
+
+ with open(path, 'w') as output_metadata:
+ data = get_metadata(args)
+ output_metadata.write(format_metadata(data) + '\n')
+
+ return data
diff --git a/PT-Retrieval/convert_openqa_data.py b/PT-Retrieval/convert_openqa_data.py
new file mode 100644
index 0000000..cb439e8
--- /dev/null
+++ b/PT-Retrieval/convert_openqa_data.py
@@ -0,0 +1,61 @@
+import json
+import os
+import sys
+
+# dataset = sys.argv[1]
+
+key = {
+ "webq": "psg_id",
+ "nq": "passage_id",
+ "trivia": "psg_id",
+ "curatedtrec": "psg_id"
+}
+
+def convert(dataset):
+ corpus = []
+ queries = []
+ qrels = []
+
+ data = json.load(open(f"data/retriever/{dataset}-dev.json", 'r'))
+
+ for i, q in enumerate(data):
+ qid = f"question-{i}"
+ query = {
+ "_id": qid,
+ "text": q["question"]
+ }
+ for p in q["positive_ctxs"] + q["negative_ctxs"] + q["hard_negative_ctxs"]:
+ psg = {
+ "_id": p[key[dataset]],
+ "title": p["title"],
+ "text": p["text"]
+ }
+ corpus.append(psg)
+
+ for p in q["positive_ctxs"]:
+ pid = p[key[dataset]]
+ qrels.append(f"{qid}\t{pid}\t{1}")
+ queries.append(query)
+
+
+ dataset = f"openqa-{dataset}"
+
+ data_dir = f"beir_eval/datasets/{dataset}"
+
+ if not os.path.exists(data_dir):
+ os.mkdir(data_dir)
+ os.mkdir(data_dir+"/qrels")
+
+ with open(os.path.join(data_dir, "corpus.jsonl"), 'w') as f:
+ for psg in corpus:
+ f.write(json.dumps(psg) + '\n')
+
+ with open(os.path.join(data_dir, "queries.jsonl"), 'w') as f:
+ for query in queries:
+ f.write(json.dumps(query) + '\n')
+
+ with open(os.path.join(data_dir, "qrels", "test.tsv"), 'w') as f:
+ f.write("query-id\tcorpus-id\tscore\n")
+ for rel in qrels:
+ f.write(rel + '\n')
+
diff --git a/PT-Retrieval/dense_retriever.py b/PT-Retrieval/dense_retriever.py
new file mode 100755
index 0000000..550c762
--- /dev/null
+++ b/PT-Retrieval/dense_retriever.py
@@ -0,0 +1,301 @@
+#!/usr/bin/env python3
+# Copyright (c) Facebook, Inc. and its affiliates.
+# All rights reserved.
+#
+# This source code is licensed under the license found in the
+# LICENSE file in the root directory of this source tree.
+
+"""
+ Command line tool to get dense results and validate them
+"""
+import argparse
+import os
+import csv
+import glob
+import json
+import gzip
+import logging
+import pickle
+import time
+from typing import List, Tuple, Dict, Iterator
+
+import numpy as np
+import torch
+from torch import Tensor as T
+from torch import nn
+
+from dpr.data.qa_validation import calculate_matches
+from dpr.models import init_encoder_components
+from dpr.options import add_encoder_params, setup_args_gpu, print_args, set_encoder_params_from_state, \
+ add_tokenizer_params, add_cuda_params, add_tuning_params
+from dpr.utils.data_utils import Tensorizer
+from dpr.utils.model_utils import setup_for_distributed_mode, get_model_obj, load_states_from_checkpoint
+from dpr.indexer.faiss_indexers import DenseIndexer, DenseHNSWFlatIndexer, DenseFlatIndexer
+csv.field_size_limit(10000000)
+
+logger = logging.getLogger()
+logger.setLevel(logging.INFO)
+if (logger.hasHandlers()):
+ logger.handlers.clear()
+console = logging.StreamHandler()
+logger.addHandler(console)
+
+
+class DenseRetriever(object):
+ """
+ Does passage retrieving over the provided index and question encoder
+ """
+ def __init__(self, question_encoder: nn.Module, batch_size: int, tensorizer: Tensorizer, index: DenseIndexer):
+ self.question_encoder = question_encoder
+ self.batch_size = batch_size
+ self.tensorizer = tensorizer
+ self.index = index
+
+ def generate_question_vectors(self, questions: List[str]) -> T:
+ n = len(questions)
+ bsz = self.batch_size
+ query_vectors = []
+
+ self.question_encoder.eval()
+
+ with torch.no_grad():
+ for j, batch_start in enumerate(range(0, n, bsz)):
+
+ batch_token_tensors = [self.tensorizer.text_to_tensor(q) for q in
+ questions[batch_start:batch_start + bsz]]
+
+ q_ids_batch = torch.stack(batch_token_tensors, dim=0).cuda()
+ q_seg_batch = torch.zeros_like(q_ids_batch).cuda()
+ q_attn_mask = self.tensorizer.get_attn_mask(q_ids_batch)
+ _, out, _ = self.question_encoder(q_ids_batch, q_seg_batch, q_attn_mask)
+
+ query_vectors.extend(out.cpu().split(1, dim=0))
+
+ if len(query_vectors) % 100 == 0:
+ logger.info('Encoded queries %d', len(query_vectors))
+
+ query_tensor = torch.cat(query_vectors, dim=0)
+
+ logger.info('Total encoded queries tensor %s', query_tensor.size())
+
+ assert query_tensor.size(0) == len(questions)
+ return query_tensor
+
+ def get_top_docs(self, query_vectors: np.array, top_docs: int = 100) -> List[Tuple[List[object], List[float]]]:
+ """
+ Does the retrieval of the best matching passages given the query vectors batch
+ :param query_vectors:
+ :param top_docs:
+ :return:
+ """
+ time0 = time.time()
+ results = self.index.search_knn(query_vectors, top_docs)
+ logger.info('index search time: %f sec.', time.time() - time0)
+ return results
+
+
+def parse_qa_csv_file(location) -> Iterator[Tuple[str, List[str]]]:
+ with open(location) as ifile:
+ reader = csv.reader(ifile, delimiter='\t')
+ for row in reader:
+ question = row[0]
+ answers = eval(row[1])
+ yield question, answers
+
+
+def validate(passages: Dict[object, Tuple[str, str]], answers: List[List[str]],
+ result_ctx_ids: List[Tuple[List[object], List[float]]],
+ workers_num: int, match_type: str, index: int = 0) -> List[List[bool]]:
+ match_stats = calculate_matches(passages, answers, result_ctx_ids, workers_num, match_type, index)
+ top_k_hits = match_stats.top_k_hits
+
+ logger.info('Validation results: top k documents hits %s', top_k_hits)
+ top_k_hits = [v / len(result_ctx_ids) for v in top_k_hits]
+ logger.info('Validation results: top k documents hits accuracy %s', top_k_hits)
+
+ return match_stats.questions_doc_hits, top_k_hits
+
+
+def load_passages(ctx_file: str) -> Dict[object, Tuple[str, str]]:
+ docs = {}
+ logger.info('Reading data from: %s', ctx_file)
+ if ctx_file.endswith(".gz"):
+ with gzip.open(ctx_file, 'rt') as tsvfile:
+ reader = csv.reader(tsvfile, delimiter='\t', )
+ # file format: doc_id, doc_text, title
+ for row in reader:
+ if row[0] != 'id':
+ docs[row[0]] = (row[1], row[2])
+ else:
+ with open(ctx_file) as tsvfile:
+ reader = csv.reader(tsvfile, delimiter='\t', )
+ # file format: doc_id, doc_text, title
+ for row in reader:
+ if row[0] != 'id':
+ docs[row[0]] = (row[1], row[2])
+ return docs
+
+
+def save_results(passages: Dict[object, Tuple[str, str]], questions: List[str], answers: List[List[str]],
+ top_passages_and_scores: List[Tuple[List[object], List[float]]], per_question_hits: List[List[bool]],
+ out_file: str
+ ):
+ # join passages text with the result ids, their questions and assigning has|no answer labels
+ merged_data = []
+ assert len(per_question_hits) == len(questions) == len(answers)
+ for i, q in enumerate(questions):
+ q_answers = answers[i]
+ results_and_scores = top_passages_and_scores[i]
+ hits = per_question_hits[i]
+ docs = [passages[doc_id] for doc_id in results_and_scores[0]]
+ scores = [str(score) for score in results_and_scores[1]]
+ ctxs_num = len(hits)
+
+ merged_data.append({
+ 'question': q,
+ 'answers': q_answers,
+ 'ctxs': [
+ {
+ 'id': results_and_scores[0][c],
+ 'title': docs[c][1],
+ 'text': docs[c][0],
+ 'score': scores[c],
+ 'has_answer': hits[c],
+ } for c in range(ctxs_num)
+ ]
+ })
+
+ with open(out_file, "w") as writer:
+ for data in merged_data:
+ writer.write(json.dumps(data) + "\n")
+ logger.info('Saved results * scores to %s', out_file)
+
+
+def iterate_encoded_files(vector_files: list) -> Iterator[Tuple[object, np.array]]:
+ for i, file in enumerate(vector_files):
+ logger.info('Reading file %s', file)
+ with open(file, "rb") as reader:
+ doc_vectors = pickle.load(reader)
+ for doc in doc_vectors:
+ db_id, doc_vector = doc
+ yield db_id, doc_vector
+
+
+def main(args):
+ total_time = time.time()
+ if not args.adapter:
+ saved_state = load_states_from_checkpoint(args.model_file)
+ set_encoder_params_from_state(saved_state.encoder_params, args)
+
+ tensorizer, encoder, _ = init_encoder_components("dpr", args, inference_only=True)
+
+ encoder = encoder.question_model
+
+ encoder, _ = setup_for_distributed_mode(encoder, None, args.device, args.n_gpu,
+ args.local_rank,
+ args.fp16)
+ encoder.eval()
+
+ # load weights from the model file
+ model_to_load = get_model_obj(encoder)
+ logger.info('Loading saved model state ...')
+ if args.adapter:
+ adapter_name = model_to_load.load_adapter(args.model_file+".q")
+ model_to_load.set_active_adapters(adapter_name)
+ else:
+ encoder_name = "question_model."
+ prefix_len = len(encoder_name)
+ if args.prefix or args.prompt:
+ encoder_name += "prefix_encoder."
+ question_encoder_state = {key[prefix_len:]: value for (key, value) in saved_state.model_dict.items() if
+ key.startswith(encoder_name)}
+ model_to_load.load_state_dict(question_encoder_state, strict=not args.prefix and not args.prompt)
+ vector_size = model_to_load.get_out_size()
+ logger.info('Encoder vector_size=%d', vector_size)
+
+ if args.hnsw_index:
+ index = DenseHNSWFlatIndexer(vector_size, args.index_buffer)
+ else:
+ index = DenseFlatIndexer(vector_size, args.index_buffer)
+
+ retriever = DenseRetriever(encoder, args.batch_size, tensorizer, index)
+
+
+ # index all passages
+ ctx_files_pattern = args.encoded_ctx_file
+ input_paths = glob.glob(ctx_files_pattern)
+ print(len(input_paths))
+ index_path = "_".join(input_paths[0].split("_")[:-1])
+ if args.save_or_load_index and (os.path.exists(index_path) or os.path.exists(index_path + ".index.dpr")):
+ retriever.index.deserialize_from(index_path)
+ else:
+ logger.info('Reading all passages data from files: %s', input_paths)
+ retriever.index.index_data(input_paths)
+ if args.save_or_load_index:
+ retriever.index.serialize(index_path)
+ # get questions & answers
+ questions = []
+ question_answers = []
+
+ for ds_item in parse_qa_csv_file(args.qa_file):
+ question, answers = ds_item
+ questions.append(question)
+ question_answers.append(answers)
+
+ sample_time = time.time()
+ questions_tensor = retriever.generate_question_vectors(questions)
+
+ # get top k results
+ top_ids_and_scores = retriever.get_top_docs(questions_tensor.numpy(), args.n_docs)
+
+ logger.info('sample time: %f sec.', time.time() - sample_time)
+ all_passages = load_passages(args.ctx_file)
+
+ logger.info('total time: %f sec.', time.time() - total_time)
+ if len(all_passages) == 0:
+ raise RuntimeError('No passages data found. Please specify ctx_file param properly.')
+
+ check_index = 1 if args.oagqa else 0
+ questions_doc_hits, top_k_hits = validate(all_passages, question_answers, top_ids_and_scores, args.validation_workers,
+ args.match, check_index)
+
+ if args.out_file:
+ save_results(all_passages, questions, question_answers, top_ids_and_scores, questions_doc_hits, args.out_file)
+
+ return top_k_hits
+
+if __name__ == '__main__':
+ parser = argparse.ArgumentParser()
+
+ add_encoder_params(parser)
+ add_tokenizer_params(parser)
+ add_cuda_params(parser)
+ add_tuning_params(parser)
+
+ parser.add_argument('--qa_file', required=True, type=str, default=None,
+ help="Question and answers file of the format: question \\t ['answer1','answer2', ...]")
+ parser.add_argument('--ctx_file', required=True, type=str, default=None,
+ help="All passages file in the tsv format: id \\t passage_text \\t title")
+ parser.add_argument('--encoded_ctx_file', type=str, default=None,
+ help='Glob path to encoded passages (from generate_dense_embeddings tool)')
+ parser.add_argument('--out_file', type=str, default=None,
+ help='output .json file path to write results to ')
+ parser.add_argument('--match', type=str, default='string', choices=['regex', 'string'],
+ help="Answer matching logic type")
+ parser.add_argument('--n-docs', type=int, default=200, help="Amount of top docs to return")
+ parser.add_argument('--validation_workers', type=int, default=16,
+ help="Number of parallel processes to validate results")
+ parser.add_argument('--batch_size', type=int, default=32, help="Batch size for question encoder forward pass")
+ parser.add_argument('--index_buffer', type=int, default=50000,
+ help="Temporal memory data buffer size (in samples) for indexer")
+ parser.add_argument("--hnsw_index", action='store_true', help='If enabled, use inference time efficient HNSW index')
+ parser.add_argument("--save_or_load_index", action='store_true', help='If enabled, save index')
+ parser.add_argument("--oagqa", action='store_true')
+
+ args = parser.parse_args()
+
+ assert args.model_file, 'Please specify --model_file checkpoint to init model weights'
+
+ setup_args_gpu(args)
+ print_args(args)
+ main(args)
diff --git a/PT-Retrieval/download_data.py b/PT-Retrieval/download_data.py
new file mode 100755
index 0000000..e7d5c4a
--- /dev/null
+++ b/PT-Retrieval/download_data.py
@@ -0,0 +1,495 @@
+#!/usr/bin/env python3
+# Copyright (c) Facebook, Inc. and its affiliates.
+# All rights reserved.
+#
+# This source code is licensed under the license found in the
+# LICENSE file in the root directory of this source tree.
+
+"""
+ Command line tool to download various preprocessed data sources & checkpoints for DPR
+"""
+
+import gzip
+import os
+import pathlib
+
+import argparse
+import wget
+
+NQ_LICENSE_FILES = [
+ 'https://dl.fbaipublicfiles.com/dpr/nq_license/LICENSE',
+ 'https://dl.fbaipublicfiles.com/dpr/nq_license/README',
+]
+
+RESOURCES_MAP = {
+ 'data.wikipedia_split.psgs_w100': {
+ 's3_url': 'https://dl.fbaipublicfiles.com/dpr/wikipedia_split/psgs_w100.tsv.gz',
+ 'original_ext': '.tsv',
+ 'compressed': True,
+ 'desc': 'Entire wikipedia passages set obtain by splitting all pages into 100-word segments (no overlap)'
+ },
+
+ 'compressed-data.wikipedia_split.psgs_w100': {
+ 's3_url': 'https://dl.fbaipublicfiles.com/dpr/wikipedia_split/psgs_w100.tsv.gz',
+ 'original_ext': '.tsv.gz',
+ 'compressed': False,
+ 'desc': 'Entire wikipedia passages set obtain by splitting all pages into 100-word segments (no overlap)'
+ },
+
+ 'data.retriever.nq-dev': {
+ 's3_url': 'https://dl.fbaipublicfiles.com/dpr/data/retriever/biencoder-nq-dev.json.gz',
+ 'original_ext': '.json',
+ 'compressed': True,
+ 'desc': 'NQ dev subset with passages pools for the Retriever train time validation',
+ 'license_files': NQ_LICENSE_FILES,
+ },
+
+ 'data.retriever.nq-train': {
+ 's3_url': 'https://dl.fbaipublicfiles.com/dpr/data/retriever/biencoder-nq-train.json.gz',
+ 'original_ext': '.json',
+ 'compressed': True,
+ 'desc': 'NQ train subset with passages pools for the Retriever training',
+ 'license_files': NQ_LICENSE_FILES,
+ },
+
+ 'data.retriever.trivia-dev': {
+ 's3_url': 'https://dl.fbaipublicfiles.com/dpr/data/retriever/biencoder-trivia-dev.json.gz',
+ 'original_ext': '.json',
+ 'compressed': True,
+ 'desc': 'TriviaQA dev subset with passages pools for the Retriever train time validation'
+ },
+
+ 'data.retriever.trivia-train': {
+ 's3_url': 'https://dl.fbaipublicfiles.com/dpr/data/retriever/biencoder-trivia-train.json.gz',
+ 'original_ext': '.json',
+ 'compressed': True,
+ 'desc': 'TriviaQA train subset with passages pools for the Retriever training'
+ },
+
+ 'data.retriever.squad1-train': {
+ 's3_url': 'https://dl.fbaipublicfiles.com/dpr/data/retriever/biencoder-squad1-train.json.gz',
+ 'original_ext': '.json',
+ 'compressed': True,
+ 'desc': 'SQUAD 1.1 train subset with passages pools for the Retriever training'
+ },
+
+ 'data.retriever.squad1-dev': {
+ 's3_url': 'https://dl.fbaipublicfiles.com/dpr/data/retriever/biencoder-squad1-dev.json.gz',
+ 'original_ext': '.json',
+ 'compressed': True,
+ 'desc': 'SQUAD 1.1 dev subset with passages pools for the Retriever train time validation'
+ },
+ "data.retriever.qas.squad1-test": {
+ "s3_url": "https://dl.fbaipublicfiles.com/dpr/data/retriever/squad1-test.qa.csv",
+ "original_ext": ".csv",
+ "compressed": False,
+ "desc": "Trivia test subset for Retriever validation and IR results generation",
+ },
+ 'data.retriever.qas.nq-dev': {
+ 's3_url': 'https://dl.fbaipublicfiles.com/dpr/data/retriever/nq-dev.qa.csv',
+ 'original_ext': '.csv',
+ 'compressed': False,
+ 'desc': 'NQ dev subset for Retriever validation and IR results generation',
+ 'license_files': NQ_LICENSE_FILES,
+ },
+
+ 'data.retriever.qas.nq-test': {
+ 's3_url': 'https://dl.fbaipublicfiles.com/dpr/data/retriever/nq-test.qa.csv',
+ 'original_ext': '.csv',
+ 'compressed': False,
+ 'desc': 'NQ test subset for Retriever validation and IR results generation',
+ 'license_files': NQ_LICENSE_FILES,
+ },
+
+ 'data.retriever.qas.nq-train': {
+ 's3_url': 'https://dl.fbaipublicfiles.com/dpr/data/retriever/nq-train.qa.csv',
+ 'original_ext': '.csv',
+ 'compressed': False,
+ 'desc': 'NQ train subset for Retriever validation and IR results generation',
+ 'license_files': NQ_LICENSE_FILES,
+ },
+
+ #
+ 'data.retriever.qas.trivia-dev': {
+ 's3_url': 'https://dl.fbaipublicfiles.com/dpr/data/retriever/trivia-dev.qa.csv.gz',
+ 'original_ext': '.csv',
+ 'compressed': True,
+ 'desc': 'Trivia dev subset for Retriever validation and IR results generation'
+ },
+
+ 'data.retriever.qas.trivia-test': {
+ 's3_url': 'https://dl.fbaipublicfiles.com/dpr/data/retriever/trivia-test.qa.csv.gz',
+ 'original_ext': '.csv',
+ 'compressed': True,
+ 'desc': 'Trivia test subset for Retriever validation and IR results generation'
+ },
+
+ 'data.retriever.qas.trivia-train': {
+ 's3_url': 'https://dl.fbaipublicfiles.com/dpr/data/retriever/trivia-train.qa.csv.gz',
+ 'original_ext': '.csv',
+ 'compressed': True,
+ 'desc': 'Trivia train subset for Retriever validation and IR results generation'
+ },
+
+ 'data.gold_passages_info.nq_train': {
+ 's3_url': 'https://dl.fbaipublicfiles.com/dpr/data/nq_gold_info/nq-train_gold_info.json.gz',
+ 'original_ext': '.json',
+ 'compressed': True,
+ 'desc': 'Original NQ (our train subset) gold positive passages and alternative question tokenization',
+ 'license_files': NQ_LICENSE_FILES,
+ },
+
+ 'data.gold_passages_info.nq_dev': {
+ 's3_url': 'https://dl.fbaipublicfiles.com/dpr/data/nq_gold_info/nq-dev_gold_info.json.gz',
+ 'original_ext': '.json',
+ 'compressed': True,
+ 'desc': 'Original NQ (our dev subset) gold positive passages and alternative question tokenization',
+ 'license_files': NQ_LICENSE_FILES,
+ },
+
+ 'data.gold_passages_info.nq_test': {
+ 's3_url': 'https://dl.fbaipublicfiles.com/dpr/data/nq_gold_info/nq-test_gold_info.json.gz',
+ 'original_ext': '.json',
+ 'compressed': True,
+ 'desc': 'Original NQ (our test, original dev subset) gold positive passages and alternative question '
+ 'tokenization',
+ 'license_files': NQ_LICENSE_FILES,
+ },
+
+ 'pretrained.fairseq.roberta-base.dict': {
+ 's3_url': 'https://dl.fbaipublicfiles.com/dpr/pretrained/fairseq/roberta/dict.txt',
+ 'original_ext': '.txt',
+ 'compressed': False,
+ 'desc': 'Dictionary for pretrained fairseq roberta model'
+ },
+
+ 'pretrained.fairseq.roberta-base.model': {
+ 's3_url': 'https://dl.fbaipublicfiles.com/dpr/pretrained/fairseq/roberta/model.pt',
+ 'original_ext': '.pt',
+ 'compressed': False,
+ 'desc': 'Weights for pretrained fairseq roberta base model'
+ },
+
+ 'pretrained.pytext.bert-base.model': {
+ 's3_url': 'https://dl.fbaipublicfiles.com/dpr/pretrained/pytext/bert/bert-base-uncased.pt',
+ 'original_ext': '.pt',
+ 'compressed': False,
+ 'desc': 'Weights for pretrained pytext bert base model'
+ },
+
+ 'data.retriever_results.nq.single.wikipedia_passages': {
+ 's3_url': ['https://dl.fbaipublicfiles.com/dpr/data/wiki_encoded/single/nq/wiki_passages_{}'.format(i) for i in
+ range(50)],
+ 'original_ext': '.pkl',
+ 'compressed': False,
+ 'desc': 'Encoded wikipedia files using a biencoder checkpoint('
+ 'checkpoint.retriever.single.nq.bert-base-encoder) trained on NQ dataset '
+ },
+
+ 'data.retriever_results.nq.single.test': {
+ 's3_url': 'https://dl.fbaipublicfiles.com/dpr/data/retriever_results/single/nq-test.json.gz',
+ 'original_ext': '.json',
+ 'compressed': True,
+ 'desc': 'Retrieval results of NQ test dataset for the encoder trained on NQ',
+ 'license_files': NQ_LICENSE_FILES,
+ },
+ 'data.retriever_results.nq.single.dev': {
+ 's3_url': 'https://dl.fbaipublicfiles.com/dpr/data/retriever_results/single/nq-dev.json.gz',
+ 'original_ext': '.json',
+ 'compressed': True,
+ 'desc': 'Retrieval results of NQ dev dataset for the encoder trained on NQ',
+ 'license_files': NQ_LICENSE_FILES,
+ },
+ 'data.retriever_results.nq.single.train': {
+ 's3_url': 'https://dl.fbaipublicfiles.com/dpr/data/retriever_results/single/nq-train.json.gz',
+ 'original_ext': '.json',
+ 'compressed': True,
+ 'desc': 'Retrieval results of NQ train dataset for the encoder trained on NQ',
+ 'license_files': NQ_LICENSE_FILES,
+ },
+
+ 'checkpoint.retriever.single.nq.bert-base-encoder': {
+ 's3_url': 'https://dl.fbaipublicfiles.com/dpr/checkpoint/retriever/single/nq/hf_bert_base.cp',
+ 'original_ext': '.cp',
+ 'compressed': False,
+ 'desc': 'Biencoder weights trained on NQ data and HF bert-base-uncased model'
+ },
+
+ 'checkpoint.retriever.multiset.bert-base-encoder': {
+ 's3_url': 'https://dl.fbaipublicfiles.com/dpr/checkpoint/retriver/multiset/hf_bert_base.cp',
+ 'original_ext': '.cp',
+ 'compressed': False,
+ 'desc': 'Biencoder weights trained on multi set data and HF bert-base-uncased model'
+ },
+
+ 'data.reader.nq.single.train': {
+ 's3_url': ['https://dl.fbaipublicfiles.com/dpr/data/reader/nq/single/train.{}.pkl'.format(i) for i in range(8)],
+ 'original_ext': '.pkl',
+ 'compressed': False,
+ 'desc': 'Reader model NQ train dataset input data preprocessed from retriever results (also trained on NQ)',
+ 'license_files': NQ_LICENSE_FILES,
+ },
+
+ 'data.reader.nq.single.dev': {
+ 's3_url': 'https://dl.fbaipublicfiles.com/dpr/data/reader/nq/single/dev.0.pkl',
+ 'original_ext': '.pkl',
+ 'compressed': False,
+ 'desc': 'Reader model NQ dev dataset input data preprocessed from retriever results (also trained on NQ)',
+ 'license_files': NQ_LICENSE_FILES,
+ },
+
+ 'data.reader.nq.single.test': {
+ 's3_url': 'https://dl.fbaipublicfiles.com/dpr/data/reader/nq/single/test.0.pkl',
+ 'original_ext': '.pkl',
+ 'compressed': False,
+ 'desc': 'Reader model NQ test dataset input data preprocessed from retriever results (also trained on NQ)',
+ 'license_files': NQ_LICENSE_FILES,
+ },
+
+ 'data.reader.trivia.multi-hybrid.train': {
+ 's3_url': ['https://dl.fbaipublicfiles.com/dpr/data/reader/trivia/multi-hybrid/train.{}.pkl'.format(i) for i in
+ range(8)],
+ 'original_ext': '.pkl',
+ 'compressed': False,
+ 'desc': 'Reader model Trivia train dataset input data preprocessed from hybrid retriever results '
+ '(where dense part is trained on multiset)'
+ },
+
+ 'data.reader.trivia.multi-hybrid.dev': {
+ 's3_url': 'https://dl.fbaipublicfiles.com/dpr/data/reader/trivia/multi-hybrid/dev.0.pkl',
+ 'original_ext': '.pkl',
+ 'compressed': False,
+ 'desc': 'Reader model Trivia dev dataset input data preprocessed from hybrid retriever results '
+ '(where dense part is trained on multiset)'
+ },
+
+ 'data.reader.trivia.multi-hybrid.test': {
+ 's3_url': 'https://dl.fbaipublicfiles.com/dpr/data/reader/trivia/multi-hybrid/test.0.pkl',
+ 'original_ext': '.pkl',
+ 'compressed': False,
+ 'desc': 'Reader model Trivia test dataset input data preprocessed from hybrid retriever results '
+ '(where dense part is trained on multiset)'
+ },
+
+ 'checkpoint.reader.nq-single.hf-bert-base': {
+ 's3_url': 'https://dl.fbaipublicfiles.com/dpr/checkpoint/reader/nq-single/hf_bert_base.cp',
+ 'original_ext': '.cp',
+ 'compressed': False,
+ 'desc': 'Reader weights trained on NQ-single retriever results and HF bert-base-uncased model'
+ },
+
+ 'checkpoint.reader.nq-trivia-hybrid.hf-bert-base': {
+ 's3_url': 'https://dl.fbaipublicfiles.com/dpr/checkpoint/reader/nq-trivia-hybrid/hf_bert_base.cp',
+ 'original_ext': '.cp',
+ 'compressed': False,
+ 'desc': 'Reader weights trained on Trivia multi hybrid retriever results and HF bert-base-uncased model'
+ },
+
+ # extra checkpoints for EfficientQA competition
+ 'checkpoint.reader.nq-single-subset.hf-bert-base': {
+ 's3_url': 'https://dl.fbaipublicfiles.com/dpr/checkpoint/reader/nq-single-seen_only/hf_bert_base.cp',
+ 'original_ext': '.cp',
+ 'compressed': False,
+ 'desc': 'Reader weights trained on NQ-single retriever results and HF bert-base-uncased model, when only Wikipedia pages seen during training are considered'
+ },
+ 'checkpoint.reader.nq-tfidf.hf-bert-base': {
+ 's3_url': 'https://dl.fbaipublicfiles.com/dpr/checkpoint/reader/nq-drqa/hf_bert_base.cp',
+ 'original_ext': '.cp',
+ 'compressed': False,
+ 'desc': 'Reader weights trained on TFIDF results and HF bert-base-uncased model'
+ },
+ 'checkpoint.reader.nq-tfidf-subset.hf-bert-base': {
+ 's3_url': 'https://dl.fbaipublicfiles.com/dpr/checkpoint/reader/nq-drqa-seen_only/hf_bert_base.cp',
+ 'original_ext': '.cp',
+ 'compressed': False,
+ 'desc': 'Reader weights trained on TFIDF results and HF bert-base-uncased model, when only Wikipedia pages seen during training are considered'
+ },
+
+ # retrieval indexes
+ 'indexes.single.nq.full.index': {
+ 's3_url': 'https://dl.fbaipublicfiles.com/dpr/checkpoint/indexes/single/nq/full.index.dpr',
+ 'original_ext': '.dpr',
+ 'compressed': False,
+ 'desc': 'DPR index on NQ-single retriever'
+ },
+ 'indexes.single.nq.full.index_meta': {
+ 's3_url': 'https://dl.fbaipublicfiles.com/dpr/checkpoint/indexes/single/nq/full.index_meta.dpr',
+ 'original_ext': '.dpr',
+ 'compressed': False,
+ 'desc': 'DPR index on NQ-single retriever (metadata)'
+ },
+ 'indexes.single.nq.subset.index': {
+ 's3_url': 'https://dl.fbaipublicfiles.com/dpr/checkpoint/indexes/single/nq/seen_only.index.dpr',
+ 'original_ext': '.dpr',
+ 'compressed': False,
+ 'desc': 'DPR index on NQ-single retriever when only Wikipedia pages seen during training are considered'
+ },
+ 'indexes.single.nq.subset.index_meta': {
+ 's3_url': 'https://dl.fbaipublicfiles.com/dpr/checkpoint/indexes/single/nq/seen_only.index_meta.dpr',
+ 'original_ext': '.dpr',
+ 'compressed': False,
+ 'desc': 'DPR index on NQ-single retriever when only Wikipedia pages seen during training are considered (metadata)'
+ },
+ 'indexes.tfidf.nq.full': {
+ 's3_url': 'https://dl.fbaipublicfiles.com/dpr/checkpoint/indexes/drqa/nq/full-tfidf.npz',
+ 'original_ext': '.npz',
+ 'compressed': False,
+ 'desc': 'TFIDF index'
+ },
+ 'indexes.tfidf.nq.subset': {
+ 's3_url': 'https://dl.fbaipublicfiles.com/dpr/checkpoint/indexes/drqa/nq/seen_only-tfidf.npz',
+ 'original_ext': '.npz',
+ 'compressed': False,
+ 'desc': 'TFIDF index when only Wikipedia pages seen during training are considered'
+ },
+ "data.retriever.webq-train": {
+ "s3_url": "https://dl.fbaipublicfiles.com/dpr/data/retriever/biencoder-webquestions-train.json.gz",
+ "original_ext": ".json",
+ "compressed": True,
+ "desc": "WebQuestions dev subset with passages pools for the Retriever train time validation",
+ },
+ "data.retriever.webq-dev": {
+ "s3_url": "https://dl.fbaipublicfiles.com/dpr/data/retriever/biencoder-webquestions-dev.json.gz",
+ "original_ext": ".json",
+ "compressed": True,
+ "desc": "WebQuestions dev subset with passages pools for the Retriever train time validation",
+ },
+ "data.retriever.qas.webq-test": {
+ "s3_url": "https://dl.fbaipublicfiles.com/dpr/data/retriever/webquestions-test.qa.csv",
+ "original_ext": ".csv",
+ "compressed": False,
+ "desc": "WebQuestions test subset for Retriever validation and IR results generation",
+ },
+ "data.retriever.curatedtrec-train": {
+ "s3_url": "https://dl.fbaipublicfiles.com/dpr/data/retriever/biencoder-curatedtrec-train.json.gz",
+ "original_ext": ".json",
+ "compressed": True,
+ "desc": "CuratedTrec dev subset with passages pools for the Retriever train time validation",
+ },
+ "data.retriever.curatedtrec-dev": {
+ "s3_url": "https://dl.fbaipublicfiles.com/dpr/data/retriever/biencoder-curatedtrec-dev.json.gz",
+ "original_ext": ".json",
+ "compressed": True,
+ "desc": "CuratedTrec dev subset with passages pools for the Retriever train time validation",
+ },
+ "data.retriever.qas.curatedtrec-test": {
+ "s3_url": "https://dl.fbaipublicfiles.com/dpr/data/retriever/curatedtrec-test.qa.csv",
+ "original_ext": ".csv",
+ "compressed": False,
+ "desc": "CuratedTrec test subset for Retriever validation and IR results generation",
+ },
+ "data.retriever.qas.trivia-test": {
+ "s3_url": "https://dl.fbaipublicfiles.com/dpr/data/retriever/trivia-test.qa.csv.gz",
+ "original_ext": ".csv",
+ "compressed": True,
+ "desc": "Trivia test subset for Retriever validation and IR results generation",
+ },
+}
+
+
+def unpack(gzip_file: str, out_file: str):
+ print('Uncompressing ', gzip_file)
+ input = gzip.GzipFile(gzip_file, 'rb')
+ s = input.read()
+ input.close()
+ output = open(out_file, 'wb')
+ output.write(s)
+ output.close()
+ print('Saved to ', out_file)
+
+
+def download_resource(s3_url: str, original_ext: str, compressed: bool, resource_key: str, out_dir: str) -> str:
+ print('Loading from ', s3_url)
+
+ # create local dir
+ path_names = resource_key.split('.')
+
+ root_dir = out_dir if out_dir else './'
+ save_root = os.path.join(root_dir, *path_names[:-1]) # last segment is for file name
+
+ pathlib.Path(save_root).mkdir(parents=True, exist_ok=True)
+
+ local_file = os.path.join(save_root, path_names[-1] + ('.tmp' if compressed else original_ext))
+
+ if os.path.exists(local_file):
+ print('File already exist ', local_file)
+ return save_root
+
+ wget.download(s3_url, out=local_file)
+
+ print('Saved to ', local_file)
+
+ if compressed:
+ uncompressed_file = os.path.join(save_root, path_names[-1] + original_ext)
+ unpack(local_file, uncompressed_file)
+ os.remove(local_file)
+ return save_root
+
+
+def download_file(s3_url: str, out_dir: str, file_name: str):
+ print('Loading from ', s3_url)
+ local_file = os.path.join(out_dir, file_name)
+
+ if os.path.exists(local_file):
+ print('File already exist ', local_file)
+ return
+
+ wget.download(s3_url, out=local_file)
+ print('Saved to ', local_file)
+
+
+def download(resource_key: str, out_dir: str = None):
+ if resource_key not in RESOURCES_MAP:
+ # match by prefix
+ resources = [k for k in RESOURCES_MAP.keys() if k.startswith(resource_key)]
+ if resources:
+ for key in resources:
+ download(key, out_dir)
+ else:
+ print('no resources found for specified key')
+ return
+ download_info = RESOURCES_MAP[resource_key]
+
+ s3_url = download_info['s3_url']
+
+ save_root_dir = None
+ if isinstance(s3_url, list):
+ for i, url in enumerate(s3_url):
+ save_root_dir = download_resource(url,
+ download_info['original_ext'],
+ download_info['compressed'],
+ '{}_{}'.format(resource_key, i),
+ out_dir)
+ else:
+ save_root_dir = download_resource(s3_url,
+ download_info['original_ext'],
+ download_info['compressed'],
+ resource_key,
+ out_dir)
+
+ license_files = download_info.get('license_files', None)
+ if not license_files:
+ return
+
+ download_file(license_files[0], save_root_dir, 'LICENSE')
+ download_file(license_files[1], save_root_dir, 'README')
+
+
+def main():
+ parser = argparse.ArgumentParser()
+
+ parser.add_argument("--output_dir", default="./", type=str,
+ help="The output directory to download file")
+ parser.add_argument("--resource", type=str,
+ help="Resource name. See RESOURCES_MAP for all possible values")
+ args = parser.parse_args()
+ if args.resource:
+ download(args.resource, args.output_dir)
+ else:
+ print('Please specify resource value. Possible options are:')
+ for k, v in RESOURCES_MAP.items():
+ print('Resource key={} description: {}'.format(k, v['desc']))
+
+
+if __name__ == '__main__':
+ main()
diff --git a/PT-Retrieval/dpr/__init__.py b/PT-Retrieval/dpr/__init__.py
new file mode 100755
index 0000000..e69de29
diff --git a/PT-Retrieval/dpr/data/__init__.py b/PT-Retrieval/dpr/data/__init__.py
new file mode 100755
index 0000000..e69de29
diff --git a/PT-Retrieval/dpr/data/qa_validation.py b/PT-Retrieval/dpr/data/qa_validation.py
new file mode 100755
index 0000000..3e936a0
--- /dev/null
+++ b/PT-Retrieval/dpr/data/qa_validation.py
@@ -0,0 +1,163 @@
+#!/usr/bin/env python3
+# Copyright (c) Facebook, Inc. and its affiliates.
+# All rights reserved.
+#
+# This source code is licensed under the license found in the
+# LICENSE file in the root directory of this source tree.
+
+"""
+ Set of utilities for Q&A results validation tasks - Retriver passage validation and Reader predicted answer validation
+"""
+
+import collections
+import logging
+import string
+import unicodedata
+from functools import partial
+from multiprocessing import Pool as ProcessPool
+from typing import Tuple, List, Dict
+
+import regex as re
+
+from dpr.utils.tokenizers import SimpleTokenizer
+
+logger = logging.getLogger(__name__)
+
+QAMatchStats = collections.namedtuple('QAMatchStats', ['top_k_hits', 'questions_doc_hits'])
+
+
+def calculate_matches(all_docs: Dict[object, Tuple[str, str]], answers: List[List[str]],
+ closest_docs: List[Tuple[List[object], List[float]]], workers_num: int,
+ match_type: str, index: int = 0) -> QAMatchStats:
+ """
+ Evaluates answers presence in the set of documents. This function is supposed to be used with a large collection of
+ documents and results. It internally forks multiple sub-processes for evaluation and then merges results
+ :param all_docs: dictionary of the entire documents database. doc_id -> (doc_text, title)
+ :param answers: list of answers's list. One list per question
+ :param closest_docs: document ids of the top results along with their scores
+ :param workers_num: amount of parallel threads to process data
+ :param match_type: type of answer matching. Refer to has_answer code for available options
+ :return: matching information tuple.
+ top_k_hits - a list where the index is the amount of top documents retrieved and the value is the total amount of
+ valid matches across an entire dataset.
+ questions_doc_hits - more detailed info with answer matches for every question and every retrieved document
+ """
+ global dpr_all_documents
+ dpr_all_documents = all_docs
+
+ tok_opts = {}
+ tokenizer = SimpleTokenizer(**tok_opts)
+
+ processes = ProcessPool(
+ processes=workers_num,
+ )
+
+ logger.info('Matching answers in top docs...')
+
+ get_score_partial = partial(check_answer, match_type=match_type, tokenizer=tokenizer, index=index)
+
+ questions_answers_docs = zip(answers, closest_docs)
+
+ scores = processes.map(get_score_partial, questions_answers_docs)
+
+ logger.info('Per question validation results len=%d', len(scores))
+
+ n_docs = len(closest_docs[0][0])
+ top_k_hits = [0] * n_docs
+ for question_hits in scores:
+ best_hit = next((i for i, x in enumerate(question_hits) if x), None)
+ if best_hit is not None:
+ top_k_hits[best_hit:] = [v + 1 for v in top_k_hits[best_hit:]]
+
+ return QAMatchStats(top_k_hits, scores)
+
+
+def check_answer(questions_answers_docs, tokenizer, match_type, index=0) -> List[bool]:
+ """Search through all the top docs to see if they have any of the answers."""
+ answers, (doc_ids, doc_scores) = questions_answers_docs
+
+ global dpr_all_documents
+ hits = []
+
+ for i, doc_id in enumerate(doc_ids):
+ doc = dpr_all_documents[doc_id]
+ # modified
+ text = doc[index] # 0 for abstract, 1 for title
+
+ answer_found = False
+ if text is None: # cannot find the document for some reason
+ logger.warning("no doc in db")
+ hits.append(False)
+ continue
+
+ if has_answer(answers, text, tokenizer, match_type):
+ answer_found = True
+ hits.append(answer_found)
+ return hits
+
+
+def has_answer(answers, text, tokenizer, match_type) -> bool:
+ """Check if a document contains an answer string.
+ If `match_type` is string, token matching is done between the text and answer.
+ If `match_type` is regex, we search the whole text with the regex.
+ """
+ text = _normalize(text)
+
+ if match_type == 'string':
+ # Answer is a list of possible strings
+ text = tokenizer.tokenize(text).words(uncased=True)
+
+ for single_answer in answers:
+ single_answer = _normalize(single_answer)
+ single_answer = tokenizer.tokenize(single_answer)
+ single_answer = single_answer.words(uncased=True)
+
+ for i in range(0, len(text) - len(single_answer) + 1):
+ if single_answer == text[i: i + len(single_answer)]:
+ return True
+
+ elif match_type == 'regex':
+ # Answer is a regex
+ for single_answer in answers:
+ single_answer = _normalize(single_answer)
+ if regex_match(text, single_answer):
+ return True
+ return False
+
+
+def regex_match(text, pattern):
+ """Test if a regex pattern is contained within a text."""
+ try:
+ pattern = re.compile(
+ pattern,
+ flags=re.IGNORECASE + re.UNICODE + re.MULTILINE,
+ )
+ except BaseException:
+ return False
+ return pattern.search(text) is not None
+
+
+# function for the reader model answer validation
+def exact_match_score(prediction, ground_truth):
+ return _normalize_answer(prediction) == _normalize_answer(ground_truth)
+
+
+def _normalize_answer(s):
+ def remove_articles(text):
+ return re.sub(r'\b(a|an|the)\b', ' ', text)
+
+ def white_space_fix(text):
+ return ' '.join(text.split())
+
+ def remove_punc(text):
+ exclude = set(string.punctuation)
+ return ''.join(ch for ch in text if ch not in exclude)
+
+ def lower(text):
+ return text.lower()
+
+ return white_space_fix(remove_articles(remove_punc(lower(s))))
+
+
+def _normalize(text):
+ return unicodedata.normalize('NFD', text)
diff --git a/PT-Retrieval/dpr/data/qasper_reader.py b/PT-Retrieval/dpr/data/qasper_reader.py
new file mode 100755
index 0000000..ee9afd5
--- /dev/null
+++ b/PT-Retrieval/dpr/data/qasper_reader.py
@@ -0,0 +1,238 @@
+import argparse
+import collections
+import glob
+import json
+import logging
+import os
+from enum import Enum
+from collections import defaultdict
+from typing import List, Dict, Tuple, Any
+
+import numpy as np
+import torch
+from torch import Tensor as T
+
+from transformers import AutoTokenizer
+
+logger = logging.getLogger()
+
+ReaderBatch = collections.namedtuple('ReaderBatch', ['input_ids', 'attention_mask'])
+
+class AnswerType(Enum):
+ EXTRACTIVE = 1
+ ABSTRACTIVE = 2
+ BOOLEAN = 3
+ NONE = 4
+
+class QasperReader():
+ def __init__(self,
+ tokenizer: AutoTokenizer,
+ context: str = "full_text",
+ max_query_length: int = 128,
+ max_document_length: int = 16384,
+ paragraph_separator: bool = False,
+ is_train: bool = True):
+
+ self._context = context
+ self._tokenizer = tokenizer
+ self.max_query_length = max_query_length
+ self.max_document_length = max_document_length
+ self._paragraph_separator = paragraph_separator
+ self.is_train = is_train
+
+ def parser_data(self, path: str):
+ with open(path, 'r', encoding="utf-8") as f:
+ logger.info('Reading file %s' % path)
+ data = json.load(f)
+
+ ret = []
+ for article_id, article in data.items():
+ article['article_id'] = article_id
+ d = self._article_to_dict(article)
+ ret.extend(d)
+ logger.info('Aggregated data size: {}'.format(len(ret)))
+ return ret
+
+ def _article_to_dict(self, article: Dict[str, Any]):
+ paragraphs = self._get_paragraphs_from_article(article)
+ tokenized_context = None
+ paragraph_start_indices = None
+
+ if not self._context == 'question_and_evidence':
+ tokenized_context, paragraph_start_indices = self._tokenize_paragraphs(paragraphs)
+
+ data_dicts = []
+ for question_answer in article['qas']:
+
+ all_answers = []
+ all_evidence = []
+ all_evidence_masks = []
+ for answer_annotation in question_answer['answers']:
+ answer, evidence, answer_type = self._extract_answer_and_evidence(answer_annotation['answer'])
+
+ all_answers.append({'text': answer, 'type': answer_type})
+ all_evidence.append(evidence)
+ all_evidence_masks.append(self._get_evidence_mask(evidence, paragraphs))
+
+ tokenized_question = self._tokenizer.encode(question_answer['question'])
+
+ metadata = {
+ 'question': question_answer['question'],
+ 'all_answers': all_answers
+ }
+ if self.is_train:
+ for answer, evidence_mask in zip(all_answers, all_evidence_masks):
+ data_dicts.append({
+ 'tokenized_question': tokenized_question,
+ 'tokenized_context': tokenized_context,
+ 'paragraph_start_indices': paragraph_start_indices,
+ 'tokenized_answer': self._tokenizer.encode(answer['text']),
+ 'evidence_mask': None, #torch.tensor(evidence_mask),
+ 'metadata': metadata
+ })
+ else:
+ data_dicts.append({
+ 'tokenized_question': tokenized_question,
+ 'tokenized_context': tokenized_context,
+ 'paragraph_start_indices': paragraph_start_indices,
+ 'tokenized_answer': self._tokenizer.encode(all_answers[0]['text']),
+ 'evidence_mask': None, #torch.tensor(evidence_mask),
+ 'metadata': metadata
+ })
+
+ return data_dicts
+
+ @staticmethod
+ def _get_evidence_mask(evidence: List[str], paragraphs: List[str]) -> List[int]:
+ """
+ Takes a list of evidence snippets, and the list of all the paragraphs from the
+ paper, and returns a list of indices of the paragraphs that contain the evidence.
+ """
+ evidence_mask = []
+ for paragraph in paragraphs:
+ for evidence_str in evidence:
+ if evidence_str in paragraph:
+ evidence_mask.append(1)
+ break
+ else:
+ evidence_mask.append(0)
+ return evidence_mask
+
+ def _extract_answer_and_evidence(self, answer: List[Dict]) -> Tuple[str, List[str]]:
+ evidence_spans = [x.replace("\n", " ").strip() for x in answer["evidence"]]
+ evidence_spans = [x for x in evidence_spans if x != ""]
+
+ answer_string = None
+ answer_type = None
+
+ if answer.get("unanswerable", False):
+ answer_string = "Unanswerable"
+ answer_type = AnswerType.NONE
+
+ elif answer.get("yes_no") is not None:
+ answer_string = "Yes" if answer["yes_no"] else "No"
+ answer_type = AnswerType.BOOLEAN
+
+ elif answer.get("extractive_spans", []):
+
+ answer_string = ", ".join(answer["extractive_spans"])
+ answer_type = AnswerType.EXTRACTIVE
+
+ else:
+ answer_string = answer.get("free_form_answer", "")
+ answer_type = AnswerType.ABSTRACTIVE
+
+ return answer_string, evidence_spans, answer_type
+
+ def _tokenize_paragraphs(self, paragraphs: List[str]) -> Tuple[List[int], List[int]]:
+ tokenized_context = []
+ paragraph_start_indices = []
+ for paragraph in paragraphs:
+ tokenized_paragraph = self._tokenizer.encode(paragraph)
+ paragraph_start_indices.append(len(tokenized_context))
+ # if self._paragraph_separator:
+ # tokenized_context.append(self._tokenizer.sep_token_id)
+ tokenized_context.extend(tokenized_paragraph)
+ if self._paragraph_separator:
+ tokenized_context.append(self._tokenizer.sep_token_id)
+ if self._paragraph_separator:
+ # We added the separator after every paragraph, so we remove it after the last one.
+ tokenized_context = tokenized_context[:-1]
+ return tokenized_context, paragraph_start_indices
+
+ def _get_paragraphs_from_article(self, article: Dict) -> List[str]:
+ # if self._context == "question_only":
+ # return []
+ # if self._context == "question_and_abstract":
+ # return [article["abstract"]]
+ full_text = article["full_text"]
+ paragraphs = []
+ for section_info in full_text:
+ if section_info["section_name"] is not None:
+ paragraphs.append(section_info["section_name"])
+ for paragraph in section_info["paragraphs"]:
+ paragraph_text = paragraph.replace("\n", " ").strip()
+ if paragraph_text:
+ paragraphs.append(paragraph_text)
+ # if self._context == "question_and_introduction":
+ # # Assuming the first section is the introduction and stopping here.
+ # break
+ return paragraphs
+
+ # def _get_sections_paragraphs_from_article(self, article: Dict) -> List[List[str]]:
+ # full_text = article["full_text"]
+ # sections = []
+ # for section_info in full_text:
+
+
+
+
+ # def _create_question_answer_tensors(self,
+ # question: str,
+ # paragraphs: List[str],
+ # tokenized_context: List[int],
+ # paragraph_start_indices: List[int] = None,
+ # evidence_mask: List[int] = None,
+ # answer: str = None,
+ # evidence: List[str] = None):
+
+ # tokenized_question = self._tokenizer.encode(question)
+ # if len(tokenized_question) > self.max_query_length:
+ # tokenized_question = tokenized_question[:self.max_document_length]
+
+ # allow_context_length = self.max_document_length - len(tokenized_question) - 1 - 1
+
+ # if len(tokenized_context) > allow_context_length:
+ # tokenized_context = tokenized_context[:allow_context_length]
+ # paragraph_start_indices = [index for index in paragraph_start_indices
+ # if index <= allow_context_length]
+ # if evidence_mask is not None:
+ # num_paragraphs = len(paragraph_start_indices)
+ # evidence_mask = evidence_mask[:num_paragraphs]
+
+ # return {
+ # 'question': tokenized_question,
+ # 'context': tokenized_context,
+ # 'paragraph_start_indices': paragraph_start_indices,
+ # 'evidence_mask': evidence_mask,
+ # 'answer': answer,
+ # 'evidence': evidence
+ # }
+
+ # question_and_context = ([self._tokenizer.cls_token_id]
+ # + tokenized_question
+ # + [self._tokenizer.sep_token_id]
+ # + tokenized_context)
+
+ # start_of_context = 1 + len(tokenized_question)
+ # paragraph_indices_list = [x + start_of_context for x in paragraph_start_indices]
+ # mask_indices = set(list(range(start_of_context)) + paragraph_indices_list)
+ # global_attention_mask = [
+ # True if i in mask_indices else False for i in range(len(question_and_context))
+ # ]
+ # print('question:', tokenized_question)
+ # print('context', tokenized_context)
+ # print('cls', self._tokenizer.cls_token_id, 'sep', self._tokenizer.sep_token_id)
+ # print('input_id', question_and_context)
+ # print(len(tokenized_question), len(tokenized_context), len(question_and_context), len(global_attention_mask))
+ # return torch.tensor(question_and_context), torch.tensor(global_attention_mask)
diff --git a/PT-Retrieval/dpr/data/reader_data.py b/PT-Retrieval/dpr/data/reader_data.py
new file mode 100755
index 0000000..6cebda5
--- /dev/null
+++ b/PT-Retrieval/dpr/data/reader_data.py
@@ -0,0 +1,424 @@
+#!/usr/bin/env python3
+# Copyright (c) Facebook, Inc. and its affiliates.
+# All rights reserved.
+#
+# This source code is licensed under the license found in the
+# LICENSE file in the root directory of this source tree.
+
+"""
+ Set of utilities for the Reader model related data processing tasks
+"""
+
+import collections
+import json
+import logging
+import math
+import multiprocessing
+import pickle
+from functools import partial
+from typing import Tuple, List, Dict, Iterable, Optional
+
+import torch
+from torch import Tensor as T
+from tqdm import tqdm
+
+from dpr.utils.data_utils import Tensorizer
+
+logger = logging.getLogger()
+
+
+class ReaderPassage(object):
+ """
+ Container to collect and cache all Q&A passages related attributes before generating the reader input
+ """
+
+ def __init__(self, id=None, text: str = None, title: str = None, score=None,
+ has_answer: bool = None):
+ self.id = id
+ # string passage representations
+ self.passage_text = text
+ self.title = title
+ self.score = score
+ self.has_answer = has_answer
+ self.passage_token_ids = None
+ # offset of the actual passage (i.e. not a question or may be title) in the sequence_ids
+ self.passage_offset = None
+ self.answers_spans = None
+ # passage token ids
+ self.sequence_ids = None
+
+ def on_serialize(self):
+ # store only final sequence_ids and the ctx offset
+ self.sequence_ids = self.sequence_ids.numpy()
+ self.passage_text = None
+ self.title = None
+ self.passage_token_ids = None
+
+ def on_deserialize(self):
+ self.sequence_ids = torch.tensor(self.sequence_ids)
+
+
+class ReaderSample(object):
+ """
+ Container to collect all Q&A passages data per singe question
+ """
+
+ def __init__(self, question: str, answers: List, positive_passages: List[ReaderPassage] = [],
+ negative_passages: List[ReaderPassage] = [],
+ passages: List[ReaderPassage] = [],
+ ):
+ self.question = question
+ self.answers = answers
+ self.positive_passages = positive_passages
+ self.negative_passages = negative_passages
+ self.passages = passages
+
+ def on_serialize(self):
+ for passage in self.passages + self.positive_passages + self.negative_passages:
+ passage.on_serialize()
+
+ def on_deserialize(self):
+ for passage in self.passages + self.positive_passages + self.negative_passages:
+ passage.on_deserialize()
+
+
+SpanPrediction = collections.namedtuple('SpanPrediction',
+ ['prediction_text', 'span_score', 'relevance_score', 'passage_index',
+ 'passage_token_ids'])
+
+# configuration for reader model passage selection
+ReaderPreprocessingCfg = collections.namedtuple('ReaderPreprocessingCfg',
+ ['use_tailing_sep', 'skip_no_positves', 'include_gold_passage',
+ 'gold_page_only_positives', 'max_positives', 'max_negatives',
+ 'min_negatives', 'max_retriever_passages'])
+
+DEFAULT_PREPROCESSING_CFG_TRAIN = ReaderPreprocessingCfg(use_tailing_sep=False, skip_no_positves=True,
+ include_gold_passage=False, gold_page_only_positives=True,
+ max_positives=20, max_negatives=50, min_negatives=150,
+ max_retriever_passages=200)
+
+DEFAULT_EVAL_PASSAGES = 100
+
+
+def preprocess_retriever_data(samples: List[Dict], gold_info_file: Optional[str], tensorizer: Tensorizer,
+ cfg: ReaderPreprocessingCfg = DEFAULT_PREPROCESSING_CFG_TRAIN,
+ is_train_set: bool = True,
+ ) -> Iterable[ReaderSample]:
+ """
+ Converts retriever results into reader training data.
+ :param samples: samples from the retriever's json file results
+ :param gold_info_file: optional path for the 'gold passages & questions' file. Required to get best results for NQ
+ :param tensorizer: Tensorizer object for text to model input tensors conversions
+ :param cfg: ReaderPreprocessingCfg object with positive and negative passage selection parameters
+ :param is_train_set: if the data should be processed as a train set
+ :return: iterable of ReaderSample objects which can be consumed by the reader model
+ """
+ sep_tensor = tensorizer.get_pair_separator_ids() # separator can be a multi token
+
+ gold_passage_map, canonical_questions = _get_gold_ctx_dict(gold_info_file) if gold_info_file else ({}, {})
+
+ no_positive_passages = 0
+ positives_from_gold = 0
+
+ def create_reader_sample_ids(sample: ReaderPassage, question: str):
+ question_and_title = tensorizer.text_to_tensor(sample.title, title=question, add_special_tokens=True)
+ if sample.passage_token_ids is None:
+ sample.passage_token_ids = tensorizer.text_to_tensor(sample.passage_text, add_special_tokens=False)
+
+ all_concatenated, shift = _concat_pair(question_and_title, sample.passage_token_ids,
+ tailing_sep=sep_tensor if cfg.use_tailing_sep else None)
+
+ sample.sequence_ids = all_concatenated
+ sample.passage_offset = shift
+ assert shift > 1
+ if sample.has_answer and is_train_set:
+ sample.answers_spans = [(span[0] + shift, span[1] + shift) for span in sample.answers_spans]
+ return sample
+
+ for sample in samples:
+ question = sample['question']
+
+ if question in canonical_questions:
+ question = canonical_questions[question]
+
+ positive_passages, negative_passages = _select_reader_passages(sample, question,
+ tensorizer,
+ gold_passage_map,
+ cfg.gold_page_only_positives,
+ cfg.max_positives, cfg.max_negatives,
+ cfg.min_negatives,
+ cfg.max_retriever_passages,
+ cfg.include_gold_passage,
+ is_train_set,
+ )
+ # create concatenated sequence ids for each passage and adjust answer spans
+ positive_passages = [create_reader_sample_ids(s, question) for s in positive_passages]
+ negative_passages = [create_reader_sample_ids(s, question) for s in negative_passages]
+
+ if is_train_set and len(positive_passages) == 0:
+ no_positive_passages += 1
+ if cfg.skip_no_positves:
+ continue
+
+ if next(iter(ctx for ctx in positive_passages if ctx.score == -1), None):
+ positives_from_gold += 1
+
+ if is_train_set:
+ yield ReaderSample(question, sample['answers'], positive_passages=positive_passages,
+ negative_passages=negative_passages)
+ else:
+ yield ReaderSample(question, sample['answers'], passages=negative_passages)
+
+ logger.info('no positive passages samples: %d', no_positive_passages)
+ logger.info('positive passages from gold samples: %d', positives_from_gold)
+
+
+def convert_retriever_results(is_train_set: bool, input_file: str, out_file_prefix: str,
+ gold_passages_file: str,
+ tensorizer: Tensorizer,
+ num_workers: int = 8) -> List[str]:
+ """
+ Converts the file with dense retriever(or any compatible file format) results into the reader input data and
+ serializes them into a set of files.
+ Conversion splits the input data into multiple chunks and processes them in parallel. Each chunk results are stored
+ in a separate file with name out_file_prefix.{number}.pkl
+ :param is_train_set: if the data should be processed for a train set (i.e. with answer span detection)
+ :param input_file: path to a json file with data to convert
+ :param out_file_prefix: output path prefix.
+ :param gold_passages_file: optional path for the 'gold passages & questions' file. Required to get best results for NQ
+ :param tensorizer: Tensorizer object for text to model input tensors conversions
+ :param num_workers: the number of parallel processes for conversion
+ :return: names of files with serialized results
+ """
+ with open(input_file, 'r', encoding="utf-8") as f:
+ samples = json.loads("["+",".join(f.readlines())+"]")
+ logger.info("Loaded %d questions + retrieval results from %s", len(samples), input_file)
+ workers = multiprocessing.Pool(num_workers)
+ ds_size = len(samples)
+ step = max(math.ceil(ds_size / num_workers), 1)
+ chunks = [samples[i:i + step] for i in range(0, ds_size, step)]
+ chunks = [(i, chunks[i]) for i in range(len(chunks))]
+
+ logger.info("Split data into %d chunks", len(chunks))
+
+ processed = 0
+ _parse_batch = partial(_preprocess_reader_samples_chunk, out_file_prefix=out_file_prefix,
+ gold_passages_file=gold_passages_file, tensorizer=tensorizer,
+ is_train_set=is_train_set)
+ serialized_files = []
+ for file_name in workers.map(_parse_batch, chunks):
+ processed += 1
+ serialized_files.append(file_name)
+ logger.info('Chunks processed %d', processed)
+ logger.info('Data saved to %s', file_name)
+ logger.info('Preprocessed data stored in %s', serialized_files)
+ return serialized_files
+
+
+def get_best_spans(tensorizer: Tensorizer, start_logits: List, end_logits: List, ctx_ids: List, max_answer_length: int,
+ passage_idx: int, relevance_score: float, top_spans: int = 1) -> List[SpanPrediction]:
+ """
+ Finds the best answer span for the extractive Q&A model
+ """
+ scores = []
+ for (i, s) in enumerate(start_logits):
+ for (j, e) in enumerate(end_logits[i:i + max_answer_length]):
+ scores.append(((i, i + j), s + e))
+
+ scores = sorted(scores, key=lambda x: x[1], reverse=True)
+
+ chosen_span_intervals = []
+ best_spans = []
+
+ for (start_index, end_index), score in scores:
+ assert start_index <= end_index
+ length = end_index - start_index + 1
+ assert length <= max_answer_length
+
+ if any([start_index <= prev_start_index <= prev_end_index <= end_index or
+ prev_start_index <= start_index <= end_index <= prev_end_index
+ for (prev_start_index, prev_end_index) in chosen_span_intervals]):
+ continue
+
+ # extend bpe subtokens to full tokens
+ start_index, end_index = _extend_span_to_full_words(tensorizer, ctx_ids,
+ (start_index, end_index))
+
+ predicted_answer = tensorizer.to_string(ctx_ids[start_index:end_index + 1])
+ best_spans.append(SpanPrediction(predicted_answer, score, relevance_score, passage_idx, ctx_ids))
+ chosen_span_intervals.append((start_index, end_index))
+
+ if len(chosen_span_intervals) == top_spans:
+ break
+ return best_spans
+
+
+def _select_reader_passages(sample: Dict,
+ question: str,
+ tensorizer: Tensorizer, gold_passage_map: Dict[str, ReaderPassage],
+ gold_page_only_positives: bool,
+ max_positives: int,
+ max1_negatives: int,
+ max2_negatives: int,
+ max_retriever_passages: int,
+ include_gold_passage: bool,
+ is_train_set: bool
+ ) -> Tuple[List[ReaderPassage], List[ReaderPassage]]:
+ answers = sample['answers']
+
+ ctxs = [ReaderPassage(**ctx) for ctx in sample['ctxs']][0:max_retriever_passages]
+ answers_token_ids = [tensorizer.text_to_tensor(a, add_special_tokens=False) for a in answers]
+
+ if is_train_set:
+ positive_samples = list(filter(lambda ctx: ctx.has_answer, ctxs))
+ negative_samples = list(filter(lambda ctx: not ctx.has_answer, ctxs))
+ else:
+ positive_samples = []
+ negative_samples = ctxs
+
+ positive_ctxs_from_gold_page = list(
+ filter(lambda ctx: _is_from_gold_wiki_page(gold_passage_map, ctx.title, question),
+ positive_samples)) if gold_page_only_positives else []
+
+ def find_answer_spans(ctx: ReaderPassage):
+ if ctx.has_answer:
+ if ctx.passage_token_ids is None:
+ ctx.passage_token_ids = tensorizer.text_to_tensor(ctx.passage_text, add_special_tokens=False)
+
+ answer_spans = [_find_answer_positions(ctx.passage_token_ids, answers_token_ids[i]) for i in
+ range(len(answers))]
+
+ # flatten spans list
+ answer_spans = [item for sublist in answer_spans for item in sublist]
+ answers_spans = list(filter(None, answer_spans))
+ ctx.answers_spans = answers_spans
+
+ if not answers_spans:
+ logger.warning('No answer found in passage id=%s text=%s, answers=%s, question=%s', ctx.id,
+ ctx.passage_text,
+ answers, question)
+
+ ctx.has_answer = bool(answers_spans)
+
+ return ctx
+
+ # check if any of the selected ctx+ has answer spans
+ selected_positive_ctxs = list(
+ filter(lambda ctx: ctx.has_answer, [find_answer_spans(ctx) for ctx in positive_ctxs_from_gold_page]))
+
+ if not selected_positive_ctxs: # fallback to positive ctx not from gold pages
+ selected_positive_ctxs = list(
+ filter(lambda ctx: ctx.has_answer, [find_answer_spans(ctx) for ctx in positive_samples])
+ )[0:max_positives]
+
+ # optionally include gold passage itself if it is still not in the positives list
+ if include_gold_passage and question in gold_passage_map:
+ gold_passage = gold_passage_map[question]
+ included_gold_passage = next(iter(ctx for ctx in selected_positive_ctxs if ctx.id == gold_passage.id), None)
+ if not included_gold_passage:
+ gold_passage = find_answer_spans(gold_passage)
+ if not gold_passage.has_answer:
+ logger.warning('No answer found in gold passage %s', gold_passage)
+ else:
+ selected_positive_ctxs.append(gold_passage)
+
+ max_negatives = min(max(10 * len(selected_positive_ctxs), max1_negatives),
+ max2_negatives) if is_train_set else DEFAULT_EVAL_PASSAGES
+ negative_samples = negative_samples[0:max_negatives]
+ return selected_positive_ctxs, negative_samples
+
+
+def _find_answer_positions(ctx_ids: T, answer: T) -> List[Tuple[int, int]]:
+ c_len = ctx_ids.size(0)
+ a_len = answer.size(0)
+ answer_occurences = []
+ for i in range(0, c_len - a_len + 1):
+ if (answer == ctx_ids[i: i + a_len]).all():
+ answer_occurences.append((i, i + a_len - 1))
+ return answer_occurences
+
+
+def _concat_pair(t1: T, t2: T, middle_sep: T = None, tailing_sep: T = None):
+ middle = ([middle_sep] if middle_sep else [])
+ r = [t1] + middle + [t2] + ([tailing_sep] if tailing_sep else [])
+ return torch.cat(r, dim=0), t1.size(0) + len(middle)
+
+
+def _get_gold_ctx_dict(file: str) -> Tuple[Dict[str, ReaderPassage], Dict[str, str]]:
+ gold_passage_infos = {} # question|question_tokens -> ReaderPassage (with title and gold ctx)
+
+ # original NQ dataset has 2 forms of same question - original, and tokenized.
+ # Tokenized form is not fully consisted with the original question if tokenized by some encoder tokenizers
+ # Specifically, this is the case for the BERT tokenizer.
+ # Depending of which form was used for retriever training and results generation, it may be useful to convert
+ # all questions to the canonical original representation.
+ original_questions = {} # question from tokens -> original question (NQ only)
+
+ with open(file, 'r', encoding="utf-8") as f:
+ logger.info('Reading file %s' % file)
+ data = json.load(f)['data']
+
+ for sample in data:
+ question = sample['question']
+ question_from_tokens = sample['question_tokens'] if 'question_tokens' in sample else question
+ original_questions[question_from_tokens] = question
+ title = sample['title'].lower()
+ context = sample['context'] # Note: This one is cased
+ rp = ReaderPassage(sample['example_id'], text=context, title=title)
+ if question in gold_passage_infos:
+ logger.info('Duplicate question %s', question)
+ rp_exist = gold_passage_infos[question]
+ logger.info('Duplicate question gold info: title new =%s | old title=%s', title, rp_exist.title)
+ logger.info('Duplicate question gold info: new ctx =%s ', context)
+ logger.info('Duplicate question gold info: old ctx =%s ', rp_exist.passage_text)
+
+ gold_passage_infos[question] = rp
+ gold_passage_infos[question_from_tokens] = rp
+ return gold_passage_infos, original_questions
+
+
+def _is_from_gold_wiki_page(gold_passage_map: Dict[str, ReaderPassage], passage_title: str, question: str):
+ gold_info = gold_passage_map.get(question, None)
+ if gold_info:
+ return passage_title.lower() == gold_info.title.lower()
+ return False
+
+
+def _extend_span_to_full_words(tensorizer: Tensorizer, tokens: List[int], span: Tuple[int, int]) -> Tuple[int, int]:
+ start_index, end_index = span
+ max_len = len(tokens)
+ while start_index > 0 and tensorizer.is_sub_word_id(tokens[start_index]):
+ start_index -= 1
+
+ while end_index < max_len - 1 and tensorizer.is_sub_word_id(tokens[end_index + 1]):
+ end_index += 1
+
+ return start_index, end_index
+
+
+def _preprocess_reader_samples_chunk(samples: List, out_file_prefix: str, gold_passages_file: str,
+ tensorizer: Tensorizer,
+ is_train_set: bool) -> str:
+ chunk_id, samples = samples
+ logger.info('Start batch %d', len(samples))
+ iterator = preprocess_retriever_data(
+ samples,
+ gold_passages_file,
+ tensorizer,
+ is_train_set=is_train_set,
+ )
+
+ results = []
+
+ iterator = tqdm(iterator)
+ for i, r in enumerate(iterator):
+ r.on_serialize()
+ if (len(r.positive_passages) > 0):
+ logger.info("%d Q: %s POS: %s", i, r.question, r.positive_passages[0].title)
+ results.append(r)
+
+ out_file = out_file_prefix + '.' + str(chunk_id) + '.pkl'
+ with open(out_file, mode='wb') as f:
+ logger.info('Serialize %d results to %s', len(results), out_file)
+ pickle.dump(results, f)
+ return out_file
diff --git a/PT-Retrieval/dpr/indexer/faiss_indexers.py b/PT-Retrieval/dpr/indexer/faiss_indexers.py
new file mode 100755
index 0000000..5b89539
--- /dev/null
+++ b/PT-Retrieval/dpr/indexer/faiss_indexers.py
@@ -0,0 +1,191 @@
+#!/usr/bin/env python3
+# Copyright (c) Facebook, Inc. and its affiliates.
+# All rights reserved.
+#
+# This source code is licensed under the license found in the
+# LICENSE file in the root directory of this source tree.
+
+"""
+ FAISS-based index components for dense retriver
+"""
+
+import os
+import time
+import logging
+import pickle
+from typing import List, Tuple, Iterator
+
+import faiss
+import numpy as np
+
+logger = logging.getLogger()
+
+
+class DenseIndexer(object):
+
+ def __init__(self, buffer_size: int = 50000):
+ self.buffer_size = buffer_size
+ self.index_id_to_db_id = []
+ self.index = None
+
+ def index_data(self, vector_files: List[str]):
+ start_time = time.time()
+ buffer = []
+ for i, item in enumerate(iterate_encoded_files(vector_files)):
+ db_id, doc_vector = item
+ buffer.append((db_id, doc_vector))
+ if 0 < self.buffer_size == len(buffer):
+ # indexing in batches is beneficial for many faiss index types
+ self._index_batch(buffer)
+ logger.info('data indexed %d, used_time: %f sec.',
+ len(self.index_id_to_db_id), time.time() - start_time)
+ buffer = []
+ self._index_batch(buffer)
+
+ indexed_cnt = len(self.index_id_to_db_id)
+ logger.info('Total data indexed %d', indexed_cnt)
+ logger.info('Data indexing completed.')
+
+ def _index_batch(self, data: List[Tuple[object, np.array]]):
+ raise NotImplementedError
+
+ def search_knn(self, query_vectors: np.array, top_docs: int) -> List[Tuple[List[object], List[float]]]:
+ raise NotImplementedError
+
+ def serialize(self, file: str):
+ logger.info('Serializing index to %s', file)
+
+ if os.path.isdir(file):
+ index_file = os.path.join(file, "index.dpr")
+ meta_file = os.path.join(file, "index_meta.dpr")
+ else:
+ index_file = file + '.index.dpr'
+ meta_file = file + '.index_meta.dpr'
+
+ faiss.write_index(self.index, index_file)
+ with open(meta_file, mode='wb') as f:
+ pickle.dump(self.index_id_to_db_id, f)
+
+ def deserialize_from(self, file: str):
+ logger.info('Loading index from %s', file)
+
+ if os.path.isdir(file):
+ index_file = os.path.join(file, "index.dpr")
+ meta_file = os.path.join(file, "index_meta.dpr")
+ else:
+ index_file = file + '.index.dpr'
+ meta_file = file + '.index_meta.dpr'
+
+ self.index = faiss.read_index(index_file)
+ logger.info('Loaded index of type %s and size %d', type(self.index), self.index.ntotal)
+
+ with open(meta_file, "rb") as reader:
+ self.index_id_to_db_id = pickle.load(reader)
+ assert len(
+ self.index_id_to_db_id) == self.index.ntotal, 'Deserialized index_id_to_db_id should match faiss index size'
+
+ def _update_id_mapping(self, db_ids: List):
+ self.index_id_to_db_id.extend(db_ids)
+
+
+class DenseFlatIndexer(DenseIndexer):
+
+ def __init__(self, vector_sz: int, buffer_size: int = 50000):
+ super(DenseFlatIndexer, self).__init__(buffer_size=buffer_size)
+ self.index = faiss.IndexFlatIP(vector_sz)
+
+ def _index_batch(self, data: List[Tuple[object, np.array]]):
+ db_ids = [t[0] for t in data]
+ vectors = [np.reshape(t[1], (1, -1)) for t in data]
+ vectors = np.concatenate(vectors, axis=0)
+ self._update_id_mapping(db_ids)
+ self.index.add(vectors)
+
+ def search_knn(self, query_vectors: np.array, top_docs: int) -> List[Tuple[List[object], List[float]]]:
+ scores, indexes = self.index.search(query_vectors, top_docs)
+ # convert to external ids
+ db_ids = [[self.index_id_to_db_id[i] for i in query_top_idxs] for query_top_idxs in indexes]
+ result = [(db_ids[i], scores[i]) for i in range(len(db_ids))]
+ return result
+
+
+class DenseHNSWFlatIndexer(DenseIndexer):
+ """
+ Efficient index for retrieval. Note: default settings are for hugh accuracy but also high RAM usage
+ """
+
+ def __init__(self, vector_sz: int, buffer_size: int = 50000, store_n: int = 512
+ , ef_search: int = 128, ef_construction: int = 200):
+ super(DenseHNSWFlatIndexer, self).__init__(buffer_size=buffer_size)
+
+ # IndexHNSWFlat supports L2 similarity only
+ # so we have to apply DOT -> L2 similairy space conversion with the help of an extra dimension
+ index = faiss.IndexHNSWFlat(vector_sz + 1, store_n)
+ index.hnsw.efSearch = ef_search
+ index.hnsw.efConstruction = ef_construction
+ self.index = index
+ self.phi = None
+
+ def index_data(self, vector_files: List[str]):
+ self._set_phi(vector_files)
+
+ super(DenseHNSWFlatIndexer, self).index_data(vector_files)
+
+ def _set_phi(self, vector_files: List[str]):
+ """
+ Calculates the max norm from the whole data and assign it to self.phi: necessary to transform IP -> L2 space
+ :param vector_files: file names to get passages vectors from
+ :return:
+ """
+ phi = 0
+ for i, item in enumerate(iterate_encoded_files(vector_files)):
+ id, doc_vector = item
+ norms = (doc_vector ** 2).sum()
+ phi = max(phi, norms)
+ logger.info('HNSWF DotProduct -> L2 space phi={}'.format(phi))
+ self.phi = phi
+
+ def _index_batch(self, data: List[Tuple[object, np.array]]):
+ # max norm is required before putting all vectors in the index to convert inner product similarity to L2
+ if self.phi is None:
+ raise RuntimeError('Max norm needs to be calculated from all data at once,'
+ 'results will be unpredictable otherwise.'
+ 'Run `_set_phi()` before calling this method.')
+
+ db_ids = [t[0] for t in data]
+ vectors = [np.reshape(t[1], (1, -1)) for t in data]
+
+ norms = [(doc_vector ** 2).sum() for doc_vector in vectors]
+ aux_dims = [np.sqrt(self.phi - norm) for norm in norms]
+ hnsw_vectors = [np.hstack((doc_vector, aux_dims[i].reshape(-1, 1))) for i, doc_vector in
+ enumerate(vectors)]
+ hnsw_vectors = np.concatenate(hnsw_vectors, axis=0)
+
+ self._update_id_mapping(db_ids)
+ self.index.add(hnsw_vectors)
+
+ def search_knn(self, query_vectors: np.array, top_docs: int) -> List[Tuple[List[object], List[float]]]:
+
+ aux_dim = np.zeros(len(query_vectors), dtype='float32')
+ query_nhsw_vectors = np.hstack((query_vectors, aux_dim.reshape(-1, 1)))
+ logger.info('query_hnsw_vectors %s', query_nhsw_vectors.shape)
+ scores, indexes = self.index.search(query_nhsw_vectors, top_docs)
+ # convert to external ids
+ db_ids = [[self.index_id_to_db_id[i] for i in query_top_idxs] for query_top_idxs in indexes]
+ result = [(db_ids[i], scores[i]) for i in range(len(db_ids))]
+ return result
+
+ def deserialize_from(self, file: str):
+ super(DenseHNSWFlatIndexer, self).deserialize_from(file)
+ # to trigger warning on subsequent indexing
+ self.phi = None
+
+
+def iterate_encoded_files(vector_files: list) -> Iterator[Tuple[object, np.array]]:
+ for i, file in enumerate(vector_files):
+ logger.info('Reading file %s', file)
+ with open(file, "rb") as reader:
+ doc_vectors = pickle.load(reader)
+ for doc in doc_vectors:
+ db_id, doc_vector = doc
+ yield db_id, doc_vector
\ No newline at end of file
diff --git a/PT-Retrieval/dpr/models/__init__.py b/PT-Retrieval/dpr/models/__init__.py
new file mode 100755
index 0000000..26d135b
--- /dev/null
+++ b/PT-Retrieval/dpr/models/__init__.py
@@ -0,0 +1,43 @@
+#!/usr/bin/env python3
+# Copyright (c) Facebook, Inc. and its affiliates.
+# All rights reserved.
+#
+# This source code is licensed under the license found in the
+# LICENSE file in the root directory of this source tree.
+
+from .hf_models import get_bert_biencoder_components, get_bert_reader_components, get_bert_tensorizer, get_roberta_tensorizer
+
+"""
+ 'Router'-like set of methods for component initialization with lazy imports
+"""
+
+ENCODER_INITIALIZERS = {
+ "dpr": get_bert_biencoder_components,
+}
+
+READER_INITIALIZERS = {
+ 'bert': get_bert_reader_components,
+}
+
+TENSORIZER_INITIALIZERS = {
+ 'bert': get_bert_tensorizer,
+ 'roberta': get_roberta_tensorizer,
+}
+
+def init_encoder_components(encoder_type: str, args, **kwargs):
+ if encoder_type in ENCODER_INITIALIZERS:
+ return ENCODER_INITIALIZERS[encoder_type](args, **kwargs)
+ else:
+ raise RuntimeError('unsupported encoder type: {}'.format(encoder_type))
+
+def init_reader_components(reader_type: str, args, **kwargs):
+ if reader_type in READER_INITIALIZERS:
+ return READER_INITIALIZERS[reader_type](args)
+ else:
+ raise RuntimeError('unsupported reader type: {}'.format(reader_type))
+
+def init_tensorizer(tensorizer_type: str, args, **kwargs):
+ if tensorizer_type in TENSORIZER_INITIALIZERS:
+ return TENSORIZER_INITIALIZERS[tensorizer_type](args)
+ else:
+ raise RuntimeError('unsupported tensorizer type: {}'.format(tensorizer_type))
diff --git a/PT-Retrieval/dpr/models/biencoder.py b/PT-Retrieval/dpr/models/biencoder.py
new file mode 100755
index 0000000..bacf822
--- /dev/null
+++ b/PT-Retrieval/dpr/models/biencoder.py
@@ -0,0 +1,198 @@
+#!/usr/bin/env python3
+# Copyright (c) Facebook, Inc. and its affiliates.
+# All rights reserved.
+#
+# This source code is licensed under the license found in the
+# LICENSE file in the root directory of this source tree.
+
+"""
+BiEncoder component + loss function for 'all-in-batch' training
+"""
+
+import collections
+import logging
+import random
+from typing import Tuple, List
+
+import numpy as np
+import torch
+import torch.nn.functional as F
+from torch import Tensor as T
+from torch import nn
+
+from dpr.utils.data_utils import Tensorizer
+from dpr.utils.data_utils import normalize_question
+
+logger = logging.getLogger(__name__)
+
+BiEncoderBatch = collections.namedtuple('BiENcoderInput',
+ ['question_ids', 'question_segments', 'context_ids', 'ctx_segments',
+ 'is_positive', 'hard_negatives'])
+
+
+def dot_product_scores(q_vectors: T, ctx_vectors: T) -> T:
+ """
+ calculates q->ctx scores for every row in ctx_vector
+ :param q_vector:
+ :param ctx_vector:
+ :return:
+ """
+ # q_vector: n1 x D, ctx_vectors: n2 x D, result n1 x n2
+ r = torch.matmul(q_vectors, torch.transpose(ctx_vectors, 0, 1))
+ return r
+
+
+def cosine_scores(q_vector: T, ctx_vectors: T):
+ # q_vector: n1 x D, ctx_vectors: n2 x D, result n1 x n2
+ return F.cosine_similarity(q_vector, ctx_vectors, dim=1)
+
+
+class BiEncoder(nn.Module):
+ """ Bi-Encoder model component. Encapsulates query/question and context/passage encoders.
+ """
+
+ def __init__(self, question_model: nn.Module, ctx_model: nn.Module, fix_q_encoder: bool = False,
+ fix_ctx_encoder: bool = False):
+ super(BiEncoder, self).__init__()
+ self.question_model = question_model
+ self.ctx_model = ctx_model
+ self.fix_q_encoder = fix_q_encoder
+ self.fix_ctx_encoder = fix_ctx_encoder
+
+ @staticmethod
+ def get_representation(sub_model: nn.Module, ids: T, segments: T, attn_mask: T, fix_encoder: bool = False) -> Tuple[
+ T, T, T]:
+ sequence_output = None
+ pooled_output = None
+ hidden_states = None
+ if ids is not None:
+ if fix_encoder:
+ with torch.no_grad():
+ sequence_output, pooled_output, hidden_states = sub_model(ids, segments, attn_mask)
+
+ if sub_model.training:
+ sequence_output.requires_grad_(requires_grad=True)
+ pooled_output.requires_grad_(requires_grad=True)
+ else:
+ sequence_output, pooled_output, hidden_states = sub_model(ids, segments, attn_mask)
+
+ return sequence_output, pooled_output, hidden_states
+
+ def forward(self, question_ids: T, question_segments: T, question_attn_mask: T, context_ids: T, ctx_segments: T,
+ ctx_attn_mask: T) -> Tuple[T, T]:
+
+ _q_seq, q_pooled_out, _q_hidden = self.get_representation(self.question_model, question_ids, question_segments,
+ question_attn_mask, self.fix_q_encoder)
+ _ctx_seq, ctx_pooled_out, _ctx_hidden = self.get_representation(self.ctx_model, context_ids, ctx_segments,
+ ctx_attn_mask, self.fix_ctx_encoder)
+
+ return q_pooled_out, ctx_pooled_out
+
+ @classmethod
+ def create_biencoder_input(cls,
+ samples: List,
+ tensorizer: Tensorizer,
+ ctx_field: str,
+ insert_title: bool,
+ num_hard_negatives: int = 0,
+ num_other_negatives: int = 0,
+ shuffle: bool = True,
+ shuffle_positives: bool = False,
+ ) -> BiEncoderBatch:
+ """
+ Creates a batch of the biencoder training tuple.
+ :param samples: list of data items (from json) to create the batch for
+ :param tensorizer: components to create model input tensors from a text sequence
+ :param insert_title: enables title insertion at the beginning of the context sequences
+ :param num_hard_negatives: amount of hard negatives per question (taken from samples' pools)
+ :param num_other_negatives: amount of other negatives per question (taken from samples' pools)
+ :param shuffle: shuffles negative passages pools
+ :param shuffle_positives: shuffles positive passages pools
+ :return: BiEncoderBatch tuple
+ """
+ question_tensors = []
+ ctx_tensors = []
+ positive_ctx_indices = []
+ hard_neg_ctx_indices = []
+
+ for sample in samples:
+ # ctx+ & [ctx-] composition
+ # as of now, take the first(gold) ctx+ only
+ if shuffle and shuffle_positives:
+ positive_ctxs = sample['positive_ctxs']
+ positive_ctx = positive_ctxs[np.random.choice(len(positive_ctxs))]
+ else:
+ positive_ctx = sample['positive_ctxs'][0]
+
+ neg_ctxs = sample['negative_ctxs']
+ hard_neg_ctxs = sample['hard_negative_ctxs']
+ question = normalize_question(sample['question'])
+
+ if shuffle:
+ random.shuffle(neg_ctxs)
+ random.shuffle(hard_neg_ctxs)
+
+ neg_ctxs = neg_ctxs[0:num_other_negatives]
+ hard_neg_ctxs = hard_neg_ctxs[0:num_hard_negatives]
+
+ all_ctxs = [positive_ctx] + neg_ctxs + hard_neg_ctxs
+ hard_negatives_start_idx = 1
+ hard_negatives_end_idx = 1 + len(hard_neg_ctxs)
+
+ current_ctxs_len = len(ctx_tensors)
+
+ sample_ctxs_tensors = [tensorizer.text_to_tensor(ctx[ctx_field], title=ctx['title'] if insert_title else None)
+ for
+ ctx in all_ctxs]
+
+ ctx_tensors.extend(sample_ctxs_tensors)
+ positive_ctx_indices.append(current_ctxs_len)
+ hard_neg_ctx_indices.append(
+ [i for i in
+ range(current_ctxs_len + hard_negatives_start_idx, current_ctxs_len + hard_negatives_end_idx)])
+
+ question_tensors.append(tensorizer.text_to_tensor(question))
+
+ ctxs_tensor = torch.cat([ctx.view(1, -1) for ctx in ctx_tensors], dim=0)
+ questions_tensor = torch.cat([q.view(1, -1) for q in question_tensors], dim=0)
+
+ ctx_segments = torch.zeros_like(ctxs_tensor)
+ question_segments = torch.zeros_like(questions_tensor)
+
+ return BiEncoderBatch(questions_tensor, question_segments, ctxs_tensor, ctx_segments, positive_ctx_indices,
+ hard_neg_ctx_indices)
+
+
+class BiEncoderNllLoss(object):
+
+ def calc(self, q_vectors: T, ctx_vectors: T, positive_idx_per_question: list,
+ hard_negative_idx_per_question: list = None) -> Tuple[T, int]:
+ """
+ Computes nll loss for the given lists of question and ctx vectors.
+ Note that although hard_negative_idx_per_question in not currently in use, one can use it for the
+ loss modifications. For example - weighted NLL with different factors for hard vs regular negatives.
+ :return: a tuple of loss value and amount of correct predictions per batch
+ """
+ scores = self.get_scores(q_vectors, ctx_vectors)
+
+ if len(q_vectors.size()) > 1:
+ q_num = q_vectors.size(0)
+ scores = scores.view(q_num, -1)
+
+ softmax_scores = F.log_softmax(scores, dim=1)
+
+ loss = F.nll_loss(softmax_scores, torch.tensor(positive_idx_per_question).to(softmax_scores.device),
+ reduction='mean')
+
+ max_score, max_idxs = torch.max(softmax_scores, 1)
+ correct_predictions_count = (max_idxs == torch.tensor(positive_idx_per_question).to(max_idxs.device)).sum()
+ return loss, correct_predictions_count
+
+ @staticmethod
+ def get_scores(q_vector: T, ctx_vectors: T) -> T:
+ f = BiEncoderNllLoss.get_similarity_function()
+ return f(q_vector, ctx_vectors)
+
+ @staticmethod
+ def get_similarity_function():
+ return dot_product_scores
diff --git a/PT-Retrieval/dpr/models/hf_led.py b/PT-Retrieval/dpr/models/hf_led.py
new file mode 100755
index 0000000..fd17907
--- /dev/null
+++ b/PT-Retrieval/dpr/models/hf_led.py
@@ -0,0 +1,80 @@
+import logging
+from typing import Tuple
+
+import torch
+from torch import Tensor as T
+from torch import nn
+
+from transformers import AdamW, BertConfig, BertModel, BertTokenizer, AutoConfig, AutoModelForSeq2SeqLM, AutoTokenizer
+from dpr.models.hf_models import get_optimizer, get_bert_tensorizer
+from dpr.utils.data_utils import Tensorizer
+from .ledreader import LedReader
+
+logger = logging.getLogger(__name__)
+
+def get_led_reader_components(args, inference_only: bool = False, **kwargs):
+ dropout = args.dropout if hasattr(args, 'dropout') else 0.0
+ # encoder = HFSeq2SeqLM.init_encoder(args.pretrained_model_cfg,
+ # projection_dim=args.projection_dim, dropout=dropout)
+ print("led")
+ cfg = AutoConfig.from_pretrained(args.pretrained_model_cfg)
+ if dropout != 0:
+ cfg.attention_dropout = dropout
+ cfg.gradient_checkpointing = False
+ cfg.use_cache=False
+ cfg.attention_window = [args.attention_window] * len(cfg.attention_window)
+ encoder = AutoModelForSeq2SeqLM.from_pretrained(args.pretrained_model_cfg, config=cfg)
+
+ hidden_size = encoder.config.hidden_size
+ reader = LedReader(encoder, hidden_size)
+ # for param in reader.parameters():
+ # print(param.data.shape)
+ optimizer = get_optimizer(reader,
+ learning_rate=args.learning_rate,
+ adam_eps=args.adam_eps, weight_decay=args.weight_decay,
+ ) if not inference_only else None
+
+ # tensorizer = get_bert_tensorizer(args, AutoTokenizer.from_pretrained(args.pretrained_model_cfg))
+ tokenizer = AutoTokenizer.from_pretrained(args.pretrained_model_cfg, add_special_tokens=False)
+ return tokenizer, reader, optimizer
+
+
+class HFSeq2SeqLM(AutoModelForSeq2SeqLM):
+
+ def __init__(self, config, project_dim: int = 0):
+ AutoModelForSeq2SeqLM.__init__(self, config)
+ assert config.hidden_size > 0, 'Encoder hidden_size can\'t be zero'
+ self.encode_proj = nn.Linear(config.hidden_size, project_dim) if project_dim != 0 else None
+ self.init_weights()
+
+ @classmethod
+ def init_encoder(cls, cfg_name: str, projection_dim: int = 0, dropout: float = 0.1, **kwargs) -> BertModel:
+ cfg = AutoConfig.from_pretrained(cfg_name)
+ if dropout != 0:
+ cfg.attention_dropout = dropout
+ cfg.gradient_checkpointing = False
+ cfg.attention_window = [1024] * len(cfg.attention_window)
+ # config.attention_window = [attention_window_size] * len(config.attention_window)
+ # config.gradient_checkpointing = gradient_checkpointing
+ return cls.from_pretrained(cfg_name, config=cfg, **kwargs)
+
+ def forward(self, input_ids: T, token_type_ids: T, attention_mask: T) -> Tuple[T, ...]:
+ if self.config.output_hidden_states:
+ sequence_output, pooled_output, hidden_states = super().forward(input_ids=input_ids,
+ token_type_ids=token_type_ids,
+ attention_mask=attention_mask)
+ else:
+ hidden_states = None
+ sequence_output, pooled_output = super().forward(input_ids=input_ids, token_type_ids=token_type_ids,
+ attention_mask=attention_mask)
+
+ pooled_output = sequence_output[:, 0, :]
+ if self.encode_proj:
+ pooled_output = self.encode_proj(pooled_output)
+ return sequence_output, pooled_output, hidden_states
+
+ def get_out_size(self):
+ if self.encode_proj:
+ return self.encode_proj.out_features
+ return self.config.hidden_size
+
diff --git a/PT-Retrieval/dpr/models/hf_models.py b/PT-Retrieval/dpr/models/hf_models.py
new file mode 100755
index 0000000..82cb485
--- /dev/null
+++ b/PT-Retrieval/dpr/models/hf_models.py
@@ -0,0 +1,390 @@
+#!/usr/bin/env python3
+# Copyright (c) Facebook, Inc. and its affiliates.
+# All rights reserved.
+#
+# This source code is licensed under the license found in the
+# LICENSE file in the root directory of this source tree.
+
+"""
+Encoder model wrappers based on HuggingFace code
+"""
+
+import logging
+from typing import Tuple
+
+import torch
+from torch import Tensor as T
+from torch import nn
+from transformers import AdamW, AutoConfig, BertConfig, BertModel, BertTokenizer, RobertaTokenizer
+from .prefix import BertPrefixModel, BertPromptModel
+
+from dpr.utils.data_utils import Tensorizer
+from .biencoder import BiEncoder
+from .reader import Reader
+
+logger = logging.getLogger(__name__)
+
+def get_bert_biencoder_components(args, inference_only: bool = False, **kwargs):
+ if args.prefix:
+ logger.info("***** P-tuning v2 mode *****")
+ question_encoder = BertPrefixEncoder.init_encoder(args, **kwargs)
+ ctx_encoder = BertPrefixEncoder.init_encoder(args, **kwargs)
+ elif args.bitfit:
+ logger.info("***** Bitfit mode *****")
+ question_encoder = BertEncoder.init_encoder(args, **kwargs)
+ ctx_encoder = BertEncoder.init_encoder(args, **kwargs)
+ elif args.adapter:
+ logger.info("***** Adapter mode *****")
+ question_encoder = BertAdapterEncoder.init_encoder(args, **kwargs)
+ ctx_encoder = BertAdapterEncoder.init_encoder(args, **kwargs)
+ elif args.prompt:
+ logger.info("***** Prompt (Lester at el. & P-tuning) mode *****")
+ question_encoder = BertPromptEncoder.init_encoder(args, **kwargs)
+ ctx_encoder = BertPromptEncoder.init_encoder(args, **kwargs)
+ else:
+ logger.info("***** Finetuning mode *****")
+ question_encoder = BertEncoder.init_encoder(args, **kwargs)
+ ctx_encoder = BertEncoder.init_encoder(args, **kwargs)
+
+
+ biencoder = BiEncoder(question_encoder, ctx_encoder)
+
+ if args.bitfit:
+ _deactivate_relevant_gradients(biencoder,trainable_components=["bias"])
+
+ question_encoder.print_params()
+
+ optimizer = get_optimizer(biencoder,
+ learning_rate=args.learning_rate,
+ adam_eps=args.adam_eps, weight_decay=args.weight_decay,
+ ) if not inference_only else None
+
+ tensorizer = get_bert_tensorizer(args)
+
+ return tensorizer, biencoder, optimizer
+
+def get_bert_reader_components(args, inference_only: bool = False, **kwargs):
+ encoder = BertEncoder.init_encoder(args)
+
+ hidden_size = encoder.config.hidden_size
+ reader = Reader(encoder, hidden_size)
+
+ optimizer = get_optimizer(reader,
+ learning_rate=args.learning_rate,
+ adam_eps=args.adam_eps, weight_decay=args.weight_decay,
+ ) if not inference_only else None
+
+ tensorizer = get_bert_tensorizer(args)
+ return tensorizer, reader, optimizer
+
+def get_bert_tensorizer(args, tokenizer=None):
+ if not tokenizer:
+ tokenizer = get_bert_tokenizer(args.pretrained_model_cfg, do_lower_case=args.do_lower_case)
+ return BertTensorizer(tokenizer, args.sequence_length)
+
+
+def get_roberta_tensorizer(args, tokenizer=None):
+ if not tokenizer:
+ tokenizer = get_roberta_tokenizer(args.pretrained_model_cfg, do_lower_case=args.do_lower_case)
+ return RobertaTensorizer(tokenizer, args.sequence_length)
+
+
+def get_optimizer(model: nn.Module, learning_rate: float = 1e-5, adam_eps: float = 1e-8,
+ weight_decay: float = 0.0, ) -> torch.optim.Optimizer:
+ no_decay = ['bias', 'LayerNorm.weight']
+
+ optimizer_grouped_parameters = [
+ {'params': [p for n, p in model.named_parameters() if not any(nd in n for nd in no_decay)],
+ 'weight_decay': weight_decay},
+ {'params': [p for n, p in model.named_parameters() if any(nd in n for nd in no_decay)], 'weight_decay': 0.0}
+ ]
+ optimizer = AdamW(optimizer_grouped_parameters, lr=learning_rate, eps=adam_eps)
+ return optimizer
+
+def get_bert_tokenizer(pretrained_cfg_name: str, do_lower_case: bool = True):
+ return BertTokenizer.from_pretrained(pretrained_cfg_name, do_lower_case=do_lower_case, cache_dir=".cache")
+
+
+def get_roberta_tokenizer(pretrained_cfg_name: str, do_lower_case: bool = True):
+ # still uses HF code for tokenizer since they are the same
+ return RobertaTokenizer.from_pretrained(pretrained_cfg_name, do_lower_case=do_lower_case, cache_dir=".cache")
+
+class BertEncoder(BertModel):
+
+ def __init__(self, config, project_dim: int = 0):
+ BertModel.__init__(self, config)
+ assert config.hidden_size > 0, 'Encoder hidden_size can\'t be zero'
+ self.encode_proj = nn.Linear(config.hidden_size, project_dim) if project_dim != 0 else None
+ self.init_weights()
+
+ @classmethod
+ def init_encoder(cls, args, **kwargs) -> BertModel:
+ cfg = BertConfig.from_pretrained(args.pretrained_model_cfg, cache_dir=".cache")
+ dropout = args.dropout if hasattr(args, 'dropout') else 0.0
+ if dropout != 0:
+ cfg.attention_probs_dropout_prob = dropout
+ cfg.hidden_dropout_prob = dropout
+ return cls.from_pretrained(args.pretrained_model_cfg, config=cfg, cache_dir=".cache", **kwargs)
+
+ def forward(self, input_ids: T, token_type_ids: T, attention_mask: T) -> Tuple[T, ...]:
+ if self.config.output_hidden_states:
+ sequence_output, pooled_output, hidden_states = super().forward(input_ids=input_ids,
+ token_type_ids=token_type_ids,
+ attention_mask=attention_mask,return_dict=False)
+ else:
+ hidden_states = None
+ sequence_output, pooled_output = super().forward(input_ids=input_ids, token_type_ids=token_type_ids,
+ attention_mask=attention_mask,return_dict=False)
+ pooled_output = sequence_output[:, 0, :]
+ if self.encode_proj:
+ pooled_output = self.encode_proj(pooled_output)
+ return sequence_output, pooled_output, hidden_states
+
+ def get_out_size(self):
+ if self.encode_proj:
+ return self.encode_proj.out_features
+ return self.config.hidden_size
+
+ def print_params(self):
+ all_param = 0
+ trainable_param = 0
+ for _, param in self.named_parameters():
+ all_param += param.numel()
+ if param.requires_grad:
+ trainable_param += param.numel()
+
+ logger.info( " ***************** PARAMETERS ***************** ")
+ logger.info(f" # all param : {all_param}")
+ logger.info(f" # trainable param : {trainable_param}")
+ logger.info( " ***************** PARAMETERS ***************** ")
+
+class BertPrefixEncoder(BertPrefixModel):
+
+ def __init__(self, config, project_dim: int = 0):
+ BertPrefixModel.__init__(self, config)
+ assert config.hidden_size > 0, 'Encoder hidden_size can\'t be zero'
+ self.encode_proj = nn.Linear(config.hidden_size, project_dim) if project_dim != 0 else None
+ self.init_weights()
+
+ @classmethod
+ def init_encoder(cls, args, **kwargs) -> BertPrefixModel:
+
+ cfg = AutoConfig.from_pretrained(args.pretrained_model_cfg, cache_dir=".cache")
+
+ cfg.pre_seq_len = args.pre_seq_len
+ cfg.prefix_mlp = args.prefix_mlp
+ cfg.prefix_hidden_size = args.prefix_hidden_size
+
+ dropout = args.dropout if hasattr(args, 'dropout') else 0.0
+ if dropout != 0:
+ cfg.attention_probs_dropout_prob = dropout
+ cfg.hidden_dropout_prob = dropout
+
+ return cls.from_pretrained(args.pretrained_model_cfg, config=cfg, cache_dir=".cache", **kwargs)
+
+ def forward(self, input_ids: T, token_type_ids: T, attention_mask: T) -> Tuple[T, ...]:
+ if self.config.output_hidden_states:
+ sequence_output, pooled_output, hidden_states = super().forward(input_ids=input_ids,
+ token_type_ids=token_type_ids,
+ attention_mask=attention_mask,return_dict=False)
+ else:
+ hidden_states = None
+ sequence_output, pooled_output = super().forward(input_ids=input_ids, token_type_ids=token_type_ids,
+ attention_mask=attention_mask,return_dict=False)
+ pooled_output = sequence_output[:, 0, :]
+ if self.encode_proj:
+ pooled_output = self.encode_proj(pooled_output)
+ return sequence_output, pooled_output, hidden_states
+
+ def get_out_size(self):
+ if self.encode_proj:
+ return self.encode_proj.out_features
+ return self.config.hidden_size
+
+ def print_params(self):
+ all_param = 0
+ trainable_param = 0
+ for _, param in self.named_parameters():
+ all_param += param.numel()
+ if param.requires_grad:
+ trainable_param += param.numel()
+
+ logger.info( " ***************** PARAMETERS ***************** ")
+ logger.info(f" # all param : {all_param}")
+ logger.info(f" # trainable param : {trainable_param}")
+ logger.info( " ***************** PARAMETERS ***************** ")
+
+class BertAdapterEncoder(BertModel):
+
+ def __init__(self, config, project_dim: int = 0):
+ BertModel.__init__(self, config)
+ assert config.hidden_size > 0, 'Encoder hidden_size can\'t be zero'
+ self.encode_proj = nn.Linear(config.hidden_size, project_dim) if project_dim != 0 else None
+ self.init_weights()
+
+ @classmethod
+ def init_encoder(cls, args, **kwargs):
+ cfg = AutoConfig.from_pretrained(args.pretrained_model_cfg, cache_dir=".cache")
+
+ cfg.pre_seq_len = args.pre_seq_len
+ cfg.prefix_mlp = args.prefix_mlp
+ cfg.prefix_hidden_size = args.prefix_hidden_size
+
+ dropout = args.dropout if hasattr(args, 'dropout') else 0.0
+ if dropout != 0:
+ cfg.attention_probs_dropout_prob = dropout
+ cfg.hidden_dropout_prob = dropout
+
+ model = cls.from_pretrained(args.pretrained_model_cfg, config=cfg, cache_dir=".cache", **kwargs)
+ model.add_adapter("adapter", config=args.adapter_config)
+ model.train_adapter("adapter")
+
+ return model
+
+ def forward(self, input_ids: T, token_type_ids: T, attention_mask: T) -> Tuple[T, ...]:
+ if self.config.output_hidden_states:
+ sequence_output, pooled_output, hidden_states = super().forward(input_ids=input_ids,
+ token_type_ids=token_type_ids,
+ attention_mask=attention_mask,return_dict=False)
+ else:
+ hidden_states = None
+ sequence_output, pooled_output = super().forward(input_ids=input_ids, token_type_ids=token_type_ids,
+ attention_mask=attention_mask,return_dict=False)
+ pooled_output = sequence_output[:, 0, :]
+ if self.encode_proj:
+ pooled_output = self.encode_proj(pooled_output)
+ return sequence_output, pooled_output, hidden_states
+
+ def get_out_size(self):
+ if self.encode_proj:
+ return self.encode_proj.out_features
+ return self.config.hidden_size
+
+ def print_params(self):
+ all_param = 0
+ trainable_param = 0
+ for _, param in self.named_parameters():
+ all_param += param.numel()
+ if param.requires_grad:
+ trainable_param += param.numel()
+
+ logger.info( " ***************** PARAMETERS ***************** ")
+ logger.info(f" # all param : {all_param}")
+ logger.info(f" # trainable param : {trainable_param}")
+ logger.info( " ***************** PARAMETERS ***************** ")
+
+class BertPromptEncoder(BertPromptModel):
+
+ def __init__(self, config, project_dim: int = 0):
+ BertPromptModel.__init__(self, config)
+ assert config.hidden_size > 0, 'Encoder hidden_size can\'t be zero'
+ self.encode_proj = nn.Linear(config.hidden_size, project_dim) if project_dim != 0 else None
+ self.init_weights()
+
+ @classmethod
+ def init_encoder(cls, args, **kwargs) -> BertPromptModel:
+
+ cfg = AutoConfig.from_pretrained(args.pretrained_model_cfg, cache_dir=".cache")
+
+ cfg.pre_seq_len = args.pre_seq_len
+ cfg.output_hidden_states = False
+
+ dropout = args.dropout if hasattr(args, 'dropout') else 0.0
+ if dropout != 0:
+ cfg.attention_probs_dropout_prob = dropout
+ cfg.hidden_dropout_prob = dropout
+
+ return cls.from_pretrained(args.pretrained_model_cfg, config=cfg, cache_dir=".cache", **kwargs)
+
+ def forward(self, input_ids: T, token_type_ids: T, attention_mask: T) -> Tuple[T, ...]:
+ hidden_states = None
+ sequence_output, pooled_output = super().forward(input_ids=input_ids, token_type_ids=token_type_ids,
+ attention_mask=attention_mask,return_dict=False)
+ pooled_output = sequence_output[:, 0, :]
+ if self.encode_proj:
+ pooled_output = self.encode_proj(pooled_output)
+ return sequence_output, pooled_output, hidden_states
+
+ def get_out_size(self):
+ if self.encode_proj:
+ return self.encode_proj.out_features
+ return self.config.hidden_size
+
+ def print_params(self):
+ all_param = 0
+ trainable_param = 0
+ for _, param in self.named_parameters():
+ all_param += param.numel()
+ if param.requires_grad:
+ trainable_param += param.numel()
+
+ logger.info( " ***************** PARAMETERS ***************** ")
+ logger.info(f" # all param : {all_param}")
+ logger.info(f" # trainable param : {trainable_param}")
+ logger.info( " ***************** PARAMETERS ***************** ")
+
+
+class BertTensorizer(Tensorizer):
+ def __init__(self, tokenizer: BertTokenizer, max_length: int, pad_to_max: bool = True):
+ self.tokenizer = tokenizer
+ self.max_length = max_length
+ self.pad_to_max = pad_to_max
+
+ def text_to_tensor(self, text: str, title: str = None, add_special_tokens: bool = True):
+ text = text.strip()
+
+ # tokenizer automatic padding is explicitly disabled since its inconsistent behavior
+ if title:
+ token_ids = self.tokenizer.encode(title, text_pair=text, add_special_tokens=add_special_tokens,
+ max_length=self.max_length,
+ pad_to_max_length=False, truncation='only_second')#, truncation='longest_first')
+ else:
+ token_ids = self.tokenizer.encode(text, add_special_tokens=add_special_tokens, max_length=self.max_length,
+ pad_to_max_length=False, truncation='only_second')#, truncation='longest_first')
+
+ seq_len = self.max_length
+ if self.pad_to_max and len(token_ids) < seq_len:
+ token_ids = token_ids + [self.tokenizer.pad_token_id] * (seq_len - len(token_ids))
+ if len(token_ids) > seq_len:
+ token_ids = token_ids[0:seq_len]
+ token_ids[-1] = self.tokenizer.sep_token_id
+
+ return torch.tensor(token_ids)
+
+ def get_pair_separator_ids(self) -> T:
+ return torch.tensor([self.tokenizer.sep_token_id])
+
+ def get_pad_id(self) -> int:
+ return self.tokenizer.pad_token_type_id
+
+ def get_attn_mask(self, tokens_tensor: T) -> T:
+ return tokens_tensor != self.get_pad_id()
+
+ def is_sub_word_id(self, token_id: int):
+ token = self.tokenizer.convert_ids_to_tokens([token_id])[0]
+ return token.startswith("##") or token.startswith(" ##")
+
+ def to_string(self, token_ids, skip_special_tokens=True):
+ return self.tokenizer.decode(token_ids, skip_special_tokens=True)
+
+ def set_pad_to_max(self, do_pad: bool):
+ self.pad_to_max = do_pad
+
+
+class RobertaTensorizer(BertTensorizer):
+ def __init__(self, tokenizer, max_length: int, pad_to_max: bool = True):
+ super(RobertaTensorizer, self).__init__(tokenizer, max_length, pad_to_max=pad_to_max)
+
+
+def _deactivate_relevant_gradients(model, trainable_components):
+ # turns off the model parameters requires_grad except the trainable bias terms.
+ for param in model.parameters():
+ param.requires_grad = False
+ if trainable_components:
+ trainable_components = trainable_components + ['pooler.dense.bias']
+
+ for name, param in model.named_parameters():
+ for component in trainable_components:
+ if component in name:
+ param.requires_grad = True
+ break
diff --git a/PT-Retrieval/dpr/models/prefix.py b/PT-Retrieval/dpr/models/prefix.py
new file mode 100755
index 0000000..6e8e9ae
--- /dev/null
+++ b/PT-Retrieval/dpr/models/prefix.py
@@ -0,0 +1,307 @@
+import logging
+
+import torch
+from transformers import BertModel, BertPreTrainedModel
+from transformers.modeling_outputs import BaseModelOutputWithPoolingAndCrossAttentions
+
+logger = logging.getLogger(__name__)
+
+class PrefixEncoder(torch.nn.Module):
+ r'''
+ The torch.nn model to encode the prefix
+
+ Input shape: (batch-size, prefix-length)
+
+ Output shape: (batch-size, prefix-length, 2*layers*hidden)
+ '''
+ def __init__(self, config):
+ super().__init__()
+ self.prefix_mlp = config.prefix_mlp
+ logger.info(f"Initializing PrefixEncoder")
+ logger.info(f" > pre_seq_len: {config.pre_seq_len}")
+ logger.info(f" > prefix_mlp: {config.prefix_mlp}")
+ logger.info(f" > prefix_hidden_size: {config.prefix_hidden_size}")
+
+ if self.prefix_mlp:
+ # Use a two-layer MLP to encode the prefix
+ self.embedding = torch.nn.Embedding(config.pre_seq_len, config.hidden_size)
+ self.trans = torch.nn.Sequential(
+ torch.nn.Linear(config.hidden_size, config.prefix_hidden_size),
+ torch.nn.Tanh(),
+ torch.nn.Linear(config.prefix_hidden_size, config.num_hidden_layers * 2 * config.hidden_size)
+ )
+ else:
+ self.embedding = torch.nn.Embedding(config.pre_seq_len, config.num_hidden_layers * 2 * config.hidden_size)
+
+ def forward(self, prefix: torch.Tensor):
+ if self.prefix_mlp:
+ prefix_tokens = self.embedding(prefix)
+ past_key_values = self.trans(prefix_tokens)
+ else:
+ past_key_values = self.embedding(prefix)
+ return past_key_values
+
+
+class BertModelModifed(BertModel):
+ def __init__(self, config, add_pooling_layer=True):
+ super().__init__(config)
+
+ def forward(
+ self,
+ input_ids=None,
+ attention_mask=None,
+ token_type_ids=None,
+ position_ids=None,
+ head_mask=None,
+ inputs_embeds=None,
+ encoder_hidden_states=None,
+ encoder_attention_mask=None,
+ past_key_values=None,
+ use_cache=None,
+ output_attentions=None,
+ output_hidden_states=None,
+ return_dict=None,
+ ):
+ output_attentions = output_attentions if output_attentions is not None else self.config.output_attentions
+ output_hidden_states = (
+ output_hidden_states if output_hidden_states is not None else self.config.output_hidden_states
+ )
+ return_dict = return_dict if return_dict is not None else self.config.use_return_dict
+
+ if self.config.is_decoder:
+ use_cache = use_cache if use_cache is not None else self.config.use_cache
+ else:
+ use_cache = False
+
+ if input_ids is not None and inputs_embeds is not None:
+ raise ValueError("You cannot specify both input_ids and inputs_embeds at the same time")
+ elif input_ids is not None:
+ input_shape = input_ids.size()
+ elif inputs_embeds is not None:
+ input_shape = inputs_embeds.size()[:-1]
+ else:
+ raise ValueError("You have to specify either input_ids or inputs_embeds")
+
+ batch_size, seq_length = input_shape
+ device = input_ids.device if input_ids is not None else inputs_embeds.device
+
+ # past_key_values_length
+ past_key_values_length = past_key_values[0][0].shape[2] if past_key_values is not None else 0
+
+ if attention_mask is None:
+ attention_mask = torch.ones(((batch_size, seq_length + past_key_values_length)), device=device)
+
+ if token_type_ids is None:
+ if hasattr(self.embeddings, "token_type_ids"):
+ buffered_token_type_ids = self.embeddings.token_type_ids[:, :seq_length]
+ buffered_token_type_ids_expanded = buffered_token_type_ids.expand(batch_size, seq_length)
+ token_type_ids = buffered_token_type_ids_expanded
+ else:
+ token_type_ids = torch.zeros(input_shape, dtype=torch.long, device=device)
+
+ # We can provide a self-attention mask of dimensions [batch_size, from_seq_length, to_seq_length]
+ # ourselves in which case we just need to make it broadcastable to all heads.
+ extended_attention_mask: torch.Tensor = self.get_extended_attention_mask(attention_mask, input_shape, device)
+
+ # If a 2D or 3D attention mask is provided for the cross-attention
+ # we need to make broadcastable to [batch_size, num_heads, seq_length, seq_length]
+ if self.config.is_decoder and encoder_hidden_states is not None:
+ encoder_batch_size, encoder_sequence_length, _ = encoder_hidden_states.size()
+ encoder_hidden_shape = (encoder_batch_size, encoder_sequence_length)
+ if encoder_attention_mask is None:
+ encoder_attention_mask = torch.ones(encoder_hidden_shape, device=device)
+ encoder_extended_attention_mask = self.invert_attention_mask(encoder_attention_mask)
+ else:
+ encoder_extended_attention_mask = None
+
+ # Prepare head mask if needed
+ # 1.0 in head_mask indicate we keep the head
+ # attention_probs has shape bsz x n_heads x N x N
+ # input head_mask has shape [num_heads] or [num_hidden_layers x num_heads]
+ # and head_mask is converted to shape [num_hidden_layers x batch x num_heads x seq_length x seq_length]
+ head_mask = self.get_head_mask(head_mask, self.config.num_hidden_layers)
+
+ embedding_output = self.embeddings(
+ input_ids=input_ids,
+ position_ids=position_ids,
+ token_type_ids=token_type_ids,
+ inputs_embeds=inputs_embeds,
+ past_key_values_length=0,
+ )
+ encoder_outputs = self.encoder(
+ embedding_output,
+ attention_mask=extended_attention_mask,
+ head_mask=head_mask,
+ encoder_hidden_states=encoder_hidden_states,
+ encoder_attention_mask=encoder_extended_attention_mask,
+ past_key_values=past_key_values,
+ use_cache=use_cache,
+ output_attentions=output_attentions,
+ output_hidden_states=output_hidden_states,
+ return_dict=return_dict,
+ )
+ sequence_output = encoder_outputs[0]
+ pooled_output = self.pooler(sequence_output) if self.pooler is not None else None
+
+ if not return_dict:
+ return (sequence_output, pooled_output) + encoder_outputs[1:]
+
+ return BaseModelOutputWithPoolingAndCrossAttentions(
+ last_hidden_state=sequence_output,
+ pooler_output=pooled_output,
+ past_key_values=encoder_outputs.past_key_values,
+ hidden_states=encoder_outputs.hidden_states,
+ attentions=encoder_outputs.attentions,
+ cross_attentions=encoder_outputs.cross_attentions,
+ )
+
+class BertPrefixModel(BertPreTrainedModel):
+ def __init__(self, config, add_pooling_layer=True):
+ super().__init__(config)
+ self.num_labels = config.num_labels
+
+ self.bert = BertModelModifed(config, add_pooling_layer=add_pooling_layer)
+ self.dropout = torch.nn.Dropout(config.hidden_dropout_prob)
+
+ for param in self.bert.parameters():
+ param.requires_grad = False
+
+ self.pre_seq_len = config.pre_seq_len
+ self.n_layer = config.num_hidden_layers
+ self.n_head = config.num_attention_heads
+ self.n_embd = config.hidden_size // config.num_attention_heads
+
+ self.prefix_tokens = torch.arange(self.pre_seq_len).long()
+ self.prefix_encoder = PrefixEncoder(config)
+
+ def get_prompt(self, batch_size):
+ prefix_tokens = self.prefix_tokens.unsqueeze(0).expand(batch_size, -1).to(self.bert.device)
+ past_key_values = self.prefix_encoder(prefix_tokens)
+ past_key_values = past_key_values.view(
+ batch_size,
+ self.pre_seq_len,
+ self.n_layer * 2,
+ self.n_head,
+ self.n_embd
+ )
+ past_key_values = self.dropout(past_key_values)
+ past_key_values = past_key_values.permute([2, 0, 3, 1, 4]).split(2)
+ return past_key_values
+
+ def forward(
+ self,
+ input_ids=None,
+ attention_mask=None,
+ token_type_ids=None,
+ position_ids=None,
+ head_mask=None,
+ inputs_embeds=None,
+ labels=None,
+ output_attentions=None,
+ output_hidden_states=None,
+ return_dict=None,
+ ):
+ return_dict = return_dict if return_dict is not None else self.config.use_return_dict
+
+ batch_size = input_ids.shape[0]
+ past_key_values = self.get_prompt(batch_size=batch_size)
+ prefix_attention_mask = torch.ones(batch_size, self.pre_seq_len).to(self.bert.device)
+ attention_mask = torch.cat((prefix_attention_mask, attention_mask), dim=1)
+
+ outputs = self.bert(
+ input_ids,
+ attention_mask=attention_mask,
+ token_type_ids=token_type_ids,
+ position_ids=position_ids,
+ head_mask=head_mask,
+ inputs_embeds=inputs_embeds,
+ output_attentions=output_attentions,
+ output_hidden_states=output_hidden_states,
+ return_dict=return_dict,
+ past_key_values=past_key_values,
+ )
+
+ return outputs
+
+
+class BertPromptModel(BertPreTrainedModel):
+ def __init__(self, config, add_pooling_layer=True):
+ super().__init__(config)
+ self.num_labels = config.num_labels
+
+ self.bert = BertModel(config, add_pooling_layer=add_pooling_layer)
+ self.embeddings = self.bert.embeddings
+ self.dropout = torch.nn.Dropout(config.hidden_dropout_prob)
+
+ for param in self.bert.parameters():
+ param.requires_grad = False
+
+ self.pre_seq_len = config.pre_seq_len
+ self.n_layer = config.num_hidden_layers
+ self.n_head = config.num_attention_heads
+ self.n_embd = config.hidden_size // config.num_attention_heads
+
+ self.prefix_tokens = torch.arange(self.pre_seq_len).long()
+ self.prefix_encoder = torch.nn.Embedding(self.pre_seq_len, config.hidden_size)
+
+ bert_param = 0
+ for _, param in self.bert.named_parameters():
+ bert_param += param.numel()
+ all_param = 0
+ for _, param in self.named_parameters():
+ all_param += param.numel()
+ logger.info( " ***************** PARAMETERS ***************** ")
+ logger.info(f" # all param : {all_param}")
+ logger.info(f" # bert param : {bert_param}")
+ logger.info(f" # trainable param : {all_param-bert_param}")
+ logger.info( " ***************** PARAMETERS ***************** ")
+
+ def get_prompt(self, batch_size):
+ prefix_tokens = self.prefix_tokens.unsqueeze(0).expand(batch_size, -1).to(self.bert.device)
+ prompts = self.prefix_encoder(prefix_tokens)
+ return prompts
+
+ def forward(
+ self,
+ input_ids=None,
+ attention_mask=None,
+ token_type_ids=None,
+ position_ids=None,
+ head_mask=None,
+ inputs_embeds=None,
+ labels=None,
+ output_attentions=None,
+ output_hidden_states=None,
+ return_dict=None,
+ ):
+ return_dict = return_dict if return_dict is not None else self.config.use_return_dict
+
+ batch_size = input_ids.shape[0]
+ raw_embedding = self.embeddings(
+ input_ids=input_ids,
+ position_ids=position_ids,
+ token_type_ids=token_type_ids,
+ )
+ prompts = self.get_prompt(batch_size=batch_size)
+ inputs_embeds = torch.cat((prompts, raw_embedding), dim=1)
+ prefix_attention_mask = torch.ones(batch_size, self.pre_seq_len).to(self.bert.device)
+ attention_mask = torch.cat((prefix_attention_mask, attention_mask), dim=1)
+
+ outputs = self.bert(
+ attention_mask=attention_mask,
+ head_mask=head_mask,
+ inputs_embeds=inputs_embeds,
+ output_attentions=output_attentions,
+ output_hidden_states=output_hidden_states,
+ return_dict=return_dict,
+ )
+
+ sequence_output = outputs[0]
+ sequence_output = sequence_output[:, self.pre_seq_len:, :].contiguous()
+ first_token_tensor = sequence_output[:, 0]
+ pooled_output = self.bert.pooler.dense(first_token_tensor)
+ pooled_output = self.bert.pooler.activation(pooled_output)
+
+ pooled_output = self.dropout(pooled_output)
+
+ return sequence_output, pooled_output
\ No newline at end of file
diff --git a/PT-Retrieval/dpr/models/reader.py b/PT-Retrieval/dpr/models/reader.py
new file mode 100755
index 0000000..7600db5
--- /dev/null
+++ b/PT-Retrieval/dpr/models/reader.py
@@ -0,0 +1,236 @@
+#!/usr/bin/env python3
+# Copyright (c) Facebook, Inc. and its affiliates.
+# All rights reserved.
+#
+# This source code is licensed under the license found in the
+# LICENSE file in the root directory of this source tree.
+
+"""
+The reader model code + its utilities (loss computation and input batch tensor generator)
+"""
+
+import collections
+import logging
+from typing import List
+
+import numpy as np
+import torch
+import torch.nn as nn
+from torch import Tensor as T
+from torch.nn import CrossEntropyLoss
+
+from dpr.data.reader_data import ReaderSample, ReaderPassage
+from dpr.utils.model_utils import init_weights
+
+logger = logging.getLogger()
+
+ReaderBatch = collections.namedtuple('ReaderBatch', ['input_ids', 'start_positions', 'end_positions', 'answers_mask'])
+
+
+class Reader(nn.Module):
+
+ def __init__(self, encoder: nn.Module, hidden_size):
+ super(Reader, self).__init__()
+ self.encoder = encoder
+ self.qa_outputs = nn.Linear(hidden_size, 2)
+ self.qa_classifier = nn.Linear(hidden_size, 1)
+ init_weights([self.qa_outputs, self.qa_classifier])
+
+ def forward(self, input_ids: T, attention_mask: T, start_positions=None, end_positions=None, answer_mask=None):
+ # notations: N - number of questions in a batch, M - number of passages per questions, L - sequence length
+ N, M, L = input_ids.size()
+ start_logits, end_logits, relevance_logits = self._forward(input_ids.view(N * M, L),
+ attention_mask.view(N * M, L))
+ if self.training:
+ return compute_loss(start_positions, end_positions, answer_mask, start_logits, end_logits, relevance_logits,
+ N, M)
+
+ return start_logits.view(N, M, L), end_logits.view(N, M, L), relevance_logits.view(N, M)
+
+ def _forward(self, input_ids, attention_mask):
+ # TODO: provide segment values
+ sequence_output, _pooled_output, _hidden_states = self.encoder(input_ids, None, attention_mask)
+ logits = self.qa_outputs(sequence_output)
+ start_logits, end_logits = logits.split(1, dim=-1)
+ start_logits = start_logits.squeeze(-1)
+ end_logits = end_logits.squeeze(-1)
+ rank_logits = self.qa_classifier(sequence_output[:, 0, :])
+ return start_logits, end_logits, rank_logits
+
+
+def compute_loss(start_positions, end_positions, answer_mask, start_logits, end_logits, relevance_logits, N, M):
+ start_positions = start_positions.view(N * M, -1)
+ end_positions = end_positions.view(N * M, -1)
+ answer_mask = answer_mask.view(N * M, -1)
+
+ start_logits = start_logits.view(N * M, -1)
+ end_logits = end_logits.view(N * M, -1)
+ relevance_logits = relevance_logits.view(N * M)
+
+ answer_mask = answer_mask.type(torch.FloatTensor).cuda()
+
+ ignored_index = start_logits.size(1)
+ start_positions.clamp_(0, ignored_index)
+ end_positions.clamp_(0, ignored_index)
+ loss_fct = CrossEntropyLoss(reduce=False, ignore_index=ignored_index)
+
+ # compute switch loss
+ relevance_logits = relevance_logits.view(N, M)
+ switch_labels = torch.zeros(N, dtype=torch.long).cuda()
+ switch_loss = torch.sum(loss_fct(relevance_logits, switch_labels))
+
+ # compute span loss
+ start_losses = [(loss_fct(start_logits, _start_positions) * _span_mask)
+ for (_start_positions, _span_mask)
+ in zip(torch.unbind(start_positions, dim=1), torch.unbind(answer_mask, dim=1))]
+
+ end_losses = [(loss_fct(end_logits, _end_positions) * _span_mask)
+ for (_end_positions, _span_mask)
+ in zip(torch.unbind(end_positions, dim=1), torch.unbind(answer_mask, dim=1))]
+ loss_tensor = torch.cat([t.unsqueeze(1) for t in start_losses], dim=1) + \
+ torch.cat([t.unsqueeze(1) for t in end_losses], dim=1)
+
+ loss_tensor = loss_tensor.view(N, M, -1).max(dim=1)[0]
+ span_loss = _calc_mml(loss_tensor)
+ return span_loss + switch_loss
+
+
+def create_reader_input(pad_token_id: int,
+ samples: List[ReaderSample],
+ passages_per_question: int,
+ max_length: int,
+ max_n_answers: int,
+ is_train: bool,
+ shuffle: bool,
+ ) -> ReaderBatch:
+ """
+ Creates a reader batch instance out of a list of ReaderSample-s
+ :param pad_token_id: id of the padding token
+ :param samples: list of samples to create the batch for
+ :param passages_per_question: amount of passages for every question in a batch
+ :param max_length: max model input sequence length
+ :param max_n_answers: max num of answers per single question
+ :param is_train: if the samples are for a train set
+ :param shuffle: should passages selection be randomized
+ :return: ReaderBatch instance
+ """
+ input_ids = []
+ start_positions = []
+ end_positions = []
+ answers_masks = []
+ empty_sequence = torch.Tensor().new_full((max_length,), pad_token_id, dtype=torch.long)
+
+ for sample in samples:
+ positive_ctxs = sample.positive_passages
+ negative_ctxs = sample.negative_passages if is_train else sample.passages
+ logger.warning("%d %d",len(positive_ctxs), len(negative_ctxs))
+ sample_tensors = _create_question_passages_tensors(positive_ctxs,
+ negative_ctxs,
+ passages_per_question,
+ empty_sequence,
+ max_n_answers,
+ pad_token_id,
+ is_train,
+ is_random=shuffle)
+ if not sample_tensors:
+ logger.warning('No valid passages combination for question=%s ', sample.question)
+ continue
+ sample_input_ids, starts_tensor, ends_tensor, answer_mask = sample_tensors
+ input_ids.append(sample_input_ids)
+ if is_train:
+ start_positions.append(starts_tensor)
+ end_positions.append(ends_tensor)
+ answers_masks.append(answer_mask)
+ input_ids = torch.cat([ids.unsqueeze(0) for ids in input_ids], dim=0)
+
+ if is_train:
+ start_positions = torch.stack(start_positions, dim=0)
+ end_positions = torch.stack(end_positions, dim=0)
+ answers_masks = torch.stack(answers_masks, dim=0)
+
+ return ReaderBatch(input_ids, start_positions, end_positions, answers_masks)
+
+
+def _calc_mml(loss_tensor):
+ marginal_likelihood = torch.sum(torch.exp(
+ - loss_tensor - 1e10 * (loss_tensor == 0).float()), 1)
+ return -torch.sum(torch.log(marginal_likelihood +
+ torch.ones(loss_tensor.size(0)).cuda() * (marginal_likelihood == 0).float()))
+
+
+def _pad_to_len(seq: T, pad_id: int, max_len: int):
+ s_len = seq.size(0)
+ if s_len > max_len:
+ return seq[0: max_len]
+ return torch.cat([seq, torch.Tensor().new_full((max_len - s_len,), pad_id, dtype=torch.long)], dim=0)
+
+
+def _get_answer_spans(idx, positives: List[ReaderPassage], max_len: int):
+ positive_a_spans = positives[idx].answers_spans
+ return [span for span in positive_a_spans if (span[0] < max_len and span[1] < max_len)]
+
+
+def _get_positive_idx(positives: List[ReaderPassage], max_len: int, is_random: bool):
+ # select just one positive
+ positive_idx = np.random.choice(len(positives)) if is_random else 0
+
+ if not _get_answer_spans(positive_idx, positives, max_len):
+ # question may be too long, find the first positive with at least one valid span
+ positive_idx = next((i for i in range(len(positives)) if _get_answer_spans(i, positives, max_len)),
+ None)
+ return positive_idx
+
+
+def _create_question_passages_tensors(positives: List[ReaderPassage], negatives: List[ReaderPassage], total_size: int,
+ empty_ids: T,
+ max_n_answers: int,
+ pad_token_id: int,
+ is_train: bool,
+ is_random: bool = True):
+ max_len = empty_ids.size(0)
+ if is_train:
+ # select just one positive
+ positive_idx = _get_positive_idx(positives, max_len, is_random)
+ if positive_idx is None:
+ return None
+ logger.warning("POS: %s", positives[positive_idx].title)
+ positive_a_spans = _get_answer_spans(positive_idx, positives, max_len)[0: max_n_answers]
+
+ answer_starts = [span[0] for span in positive_a_spans]
+ answer_ends = [span[1] for span in positive_a_spans]
+
+ assert all(s < max_len for s in answer_starts)
+ assert all(e < max_len for e in answer_ends)
+
+ positive_input_ids = _pad_to_len(positives[positive_idx].sequence_ids, pad_token_id, max_len)
+
+ answer_starts_tensor = torch.zeros((total_size, max_n_answers)).long()
+ answer_starts_tensor[0, 0:len(answer_starts)] = torch.tensor(answer_starts)
+
+ answer_ends_tensor = torch.zeros((total_size, max_n_answers)).long()
+ answer_ends_tensor[0, 0:len(answer_ends)] = torch.tensor(answer_ends)
+
+ answer_mask = torch.zeros((total_size, max_n_answers), dtype=torch.long)
+ answer_mask[0, 0:len(answer_starts)] = torch.tensor([1 for _ in range(len(answer_starts))])
+
+ positives_selected = [positive_input_ids]
+
+ else:
+ positives_selected = []
+ answer_starts_tensor = None
+ answer_ends_tensor = None
+ answer_mask = None
+
+ positives_num = len(positives_selected)
+ negative_idxs = np.random.permutation(range(len(negatives))) if is_random else range(
+ len(negatives) - positives_num)
+
+ negative_idxs = negative_idxs[:total_size - positives_num]
+
+ negatives_selected = [_pad_to_len(negatives[i].sequence_ids, pad_token_id, max_len) for i in negative_idxs]
+
+ while len(negatives_selected) < total_size - positives_num:
+ negatives_selected.append(empty_ids.clone())
+
+ input_ids = torch.stack([t for t in positives_selected + negatives_selected], dim=0)
+ return input_ids, answer_starts_tensor, answer_ends_tensor, answer_mask
diff --git a/PT-Retrieval/dpr/options.py b/PT-Retrieval/dpr/options.py
new file mode 100755
index 0000000..8cd480e
--- /dev/null
+++ b/PT-Retrieval/dpr/options.py
@@ -0,0 +1,189 @@
+#!/usr/bin/env python3
+# Copyright (c) Facebook, Inc. and its affiliates.
+# All rights reserved.
+#
+# This source code is licensed under the license found in the
+# LICENSE file in the root directory of this source tree.
+
+"""
+Command line arguments utils
+"""
+
+import argparse
+import logging
+import os
+import random
+import socket
+
+import numpy as np
+import torch
+
+logger = logging.getLogger()
+
+
+def add_tokenizer_params(parser: argparse.ArgumentParser):
+ parser.add_argument("--do_lower_case", action='store_true',
+ help="Whether to lower case the input text. True for uncased models, False for cased models.")
+
+
+def add_encoder_params(parser: argparse.ArgumentParser):
+ """
+ Common parameters to initialize an encoder-based model
+ """
+ parser.add_argument("--pretrained_model_cfg", default=None, type=str, help="config name for model initialization")
+ parser.add_argument("--encoder_model_type", default=None, type=str,
+ help="model type. One of [hf_bert, pytext_bert, fairseq_roberta]")
+ parser.add_argument('--pretrained_file', type=str, help="Some encoders need to be initialized from a file")
+ parser.add_argument("--model_file", default=None, type=str,
+ help="Saved bi-encoder checkpoint file to initialize the model")
+ parser.add_argument("--projection_dim", default=0, type=int,
+ help="Extra linear layer on top of standard bert/roberta encoder")
+ parser.add_argument("--sequence_length", type=int, default=512, help="Max length of the encoder input sequence")
+
+def add_tuning_params(parser: argparse.ArgumentParser):
+ # p-tuning v2 params
+ parser.add_argument("--prefix", action="store_true")
+ parser.add_argument("--pre_seq_len", type=int, default=8)
+ parser.add_argument("--prefix_hidden_size", type=int, default=512)
+ parser.add_argument("--prefix_mlp", action="store_true")
+
+ parser.add_argument("--bitfit", action="store_true")
+
+ # adapter params
+ parser.add_argument("--adapter", action="store_true")
+ parser.add_argument("--adapter_config", type=str, default="pfeiffer")
+
+ # p-tuning params
+ parser.add_argument("--prompt", action="store_true")
+
+def add_training_params(parser: argparse.ArgumentParser):
+ """
+ Common parameters for training
+ """
+ add_cuda_params(parser)
+ parser.add_argument("--train_file", default=None, type=str, help="File pattern for the train set")
+ parser.add_argument("--dev_file", default=None, type=str, help="")
+ parser.add_argument("--tb_folder", type=str)
+
+ parser.add_argument("--batch_size", default=2, type=int, help="Amount of questions per batch")
+ parser.add_argument("--dev_batch_size", type=int, default=4,
+ help="amount of questions per batch for dev set validation")
+ parser.add_argument('--seed', type=int, default=0, help="random seed for initialization and dataset shuffling")
+
+ parser.add_argument("--adam_eps", default=1e-8, type=float, help="Epsilon for Adam optimizer.")
+ parser.add_argument("--adam_betas", default='(0.9, 0.999)', type=str, help="Betas for Adam optimizer.")
+
+ parser.add_argument("--max_grad_norm", default=1.0, type=float, help="Max gradient norm.")
+ parser.add_argument("--log_batch_step", default=100, type=int, help="")
+ parser.add_argument("--train_rolling_loss_step", default=100, type=int, help="")
+ parser.add_argument("--weight_decay", default=0.0, type=float, help="")
+ parser.add_argument("--momentum", default=0.9, type=float, help="")
+ parser.add_argument("--learning_rate", default=1e-5, type=float, help="The initial learning rate for Adam.")
+
+ parser.add_argument("--warmup_steps", default=100, type=int, help="Linear warmup over warmup_steps.")
+ parser.add_argument("--dropout", default=0.1, type=float, help="")
+
+ parser.add_argument('--gradient_accumulation_steps', type=int, default=1,
+ help="Number of updates steps to accumulate before performing a backward/update pass.")
+ parser.add_argument("--num_train_epochs", default=3.0, type=float,
+ help="Total number of training epochs to perform.")
+ parser.add_argument('--ctx_field', type=str, choices=['title', 'abstract', 'text'], default='text')
+
+
+def add_cuda_params(parser: argparse.ArgumentParser):
+ parser.add_argument("--no_cuda", action='store_true', help="Whether not to use CUDA when available")
+ parser.add_argument("--local_rank", type=int, default=-1, help="local_rank for distributed training on gpus")
+ parser.add_argument('--fp16', action='store_true',
+ help="Whether to use 16-bit float precision instead of 32-bit")
+
+ parser.add_argument('--fp16_opt_level', type=str, default='O1',
+ help="For fp16: Apex AMP optimization level selected in ['O0', 'O1', 'O2', and 'O3']."
+ "See details at https://nvidia.github.io/apex/amp.html")
+
+
+def add_reader_preprocessing_params(parser: argparse.ArgumentParser):
+ parser.add_argument("--gold_passages_src", type=str,
+ help="File with the original dataset passages (json format). Required for train set")
+ parser.add_argument("--gold_passages_src_dev", type=str,
+ help="File with the original dataset passages (json format). Required for dev set")
+ parser.add_argument("--num_workers", type=int, default=16,
+ help="number of parallel processes to binarize reader data")
+
+
+
+
+def get_encoder_checkpoint_params_names():
+ return ['do_lower_case', 'pretrained_model_cfg', 'encoder_model_type',
+ 'pretrained_file',
+ 'projection_dim', 'sequence_length']
+
+
+def get_encoder_params_state(args):
+ """
+ Selects the param values to be saved in a checkpoint, so that a trained model faile can be used for downstream
+ tasks without the need to specify these parameter again
+ :return: Dict of params to memorize in a checkpoint
+ """
+ params_to_save = get_encoder_checkpoint_params_names()
+
+ r = {}
+ for param in params_to_save:
+ r[param] = getattr(args, param)
+ return r
+
+
+def set_encoder_params_from_state(state, args):
+ if not state:
+ return
+ params_to_save = get_encoder_checkpoint_params_names()
+
+ override_params = [(param, state[param]) for param in params_to_save if param in state and state[param]]
+ for param, value in override_params:
+ if hasattr(args, param):
+ logger.warning('Overriding args parameter value from checkpoint state. Param = %s, value = %s', param,
+ value)
+ setattr(args, param, value)
+ return args
+
+
+def set_seed(args):
+ seed = args.seed
+ random.seed(seed)
+ np.random.seed(seed)
+ torch.manual_seed(seed)
+ if args.n_gpu > 0:
+ torch.cuda.manual_seed_all(seed)
+
+
+def setup_args_gpu(args):
+ """
+ Setup arguments CUDA, GPU & distributed training
+ """
+
+ if args.local_rank == -1 or args.no_cuda: # single-node multi-gpu (or cpu) mode
+ device = torch.device("cuda" if torch.cuda.is_available() and not args.no_cuda else "cpu")
+ args.n_gpu = torch.cuda.device_count()
+ else: # distributed mode
+ torch.cuda.set_device(args.local_rank)
+ device = torch.device("cuda", args.local_rank)
+ torch.distributed.init_process_group(backend="nccl")
+ args.n_gpu = 1
+ args.device = device
+ ws = os.environ.get('WORLD_SIZE')
+
+ args.distributed_world_size = int(ws) if ws else 1
+
+ logger.info(
+ 'Initialized host %s as d.rank %d on device=%s, n_gpu=%d, world size=%d', socket.gethostname(),
+ args.local_rank, device,
+ args.n_gpu,
+ args.distributed_world_size)
+ logger.info("16-bits training: %s ", args.fp16)
+
+
+def print_args(args):
+ logger.info(" **************** CONFIGURATION **************** ")
+ for key, val in sorted(vars(args).items()):
+ keystr = "{}".format(key) + (" " * (30 - len(key)))
+ logger.info("%s --> %s", keystr, val)
+ logger.info(" **************** CONFIGURATION **************** ")
diff --git a/PT-Retrieval/dpr/utils/__init__.py b/PT-Retrieval/dpr/utils/__init__.py
new file mode 100755
index 0000000..e69de29
diff --git a/PT-Retrieval/dpr/utils/data_utils.py b/PT-Retrieval/dpr/utils/data_utils.py
new file mode 100755
index 0000000..e498cee
--- /dev/null
+++ b/PT-Retrieval/dpr/utils/data_utils.py
@@ -0,0 +1,168 @@
+#!/usr/bin/env python3
+# Copyright (c) Facebook, Inc. and its affiliates.
+# All rights reserved.
+#
+# This source code is licensed under the license found in the
+# LICENSE file in the root directory of this source tree.
+
+"""
+Utilities for general purpose data processing
+"""
+
+import json
+import logging
+import math
+import pickle
+import random
+from typing import List, Iterator, Callable
+
+from torch import Tensor as T
+
+logger = logging.getLogger()
+
+
+def read_serialized_data_from_files(paths: List[str]) -> List:
+ results = []
+ for i, path in enumerate(paths):
+ with open(path, "rb") as reader:
+ logger.info('Reading file %s', path)
+ data = pickle.load(reader)
+ results.extend(data)
+ logger.info('Aggregated data size: {}'.format(len(results)))
+ logger.info('Total data size: {}'.format(len(results)))
+ return results
+
+
+def read_data_from_json_files(paths: List[str], upsample_rates: List = None) -> List:
+ results = []
+ if upsample_rates is None:
+ upsample_rates = [1] * len(paths)
+
+ assert len(upsample_rates) == len(paths), 'up-sample rates parameter doesn\'t match input files amount'
+
+ for i, path in enumerate(paths):
+ with open(path, 'r', encoding="utf-8") as f:
+ logger.info('Reading file %s' % path)
+ data = json.load(f)
+ upsample_factor = int(upsample_rates[i])
+ data = data * upsample_factor
+ results.extend(data)
+ logger.info('Aggregated data size: {}'.format(len(results)))
+ return results
+
+
+class ShardedDataIterator(object):
+ """
+ General purpose data iterator to be used for Pytorch's DDP mode where every node should handle its own part of
+ the data.
+ Instead of cutting data shards by their min size, it sets the amount of iterations by the maximum shard size.
+ It fills the extra sample by just taking first samples in a shard.
+ It can also optionally enforce identical batch size for all iterations (might be useful for DP mode).
+ """
+ def __init__(self, data: list, shard_id: int = 0, num_shards: int = 1, batch_size: int = 1, shuffle=True,
+ shuffle_seed: int = 0, offset: int = 0,
+ strict_batch_size: bool = False
+ ):
+
+ self.data = data
+ total_size = len(data)
+
+ self.shards_num = max(num_shards, 1)
+ self.shard_id = max(shard_id, 0)
+
+ samples_per_shard = math.ceil(total_size / self.shards_num)
+
+ self.shard_start_idx = self.shard_id * samples_per_shard
+
+ self.shard_end_idx = min(self.shard_start_idx + samples_per_shard, total_size)
+
+ if strict_batch_size:
+ self.max_iterations = math.ceil(samples_per_shard / batch_size)
+ else:
+ self.max_iterations = int(samples_per_shard / batch_size)
+
+ logger.debug(
+ 'samples_per_shard=%d, shard_start_idx=%d, shard_end_idx=%d, max_iterations=%d', samples_per_shard,
+ self.shard_start_idx,
+ self.shard_end_idx,
+ self.max_iterations)
+
+ self.iteration = offset # to track in-shard iteration status
+ self.shuffle = shuffle
+ self.batch_size = batch_size
+ self.shuffle_seed = shuffle_seed
+ self.strict_batch_size = strict_batch_size
+
+ def total_data_len(self) -> int:
+ return len(self.data)
+
+ def iterate_data(self, epoch: int = 0) -> Iterator[List]:
+ if self.shuffle:
+ # to be able to resume, same shuffling should be used when starting from a failed/stopped iteration
+ epoch_rnd = random.Random(self.shuffle_seed + epoch)
+ epoch_rnd.shuffle(self.data)
+
+ # if resuming iteration somewhere in the middle of epoch, one needs to adjust max_iterations
+
+ max_iterations = self.max_iterations - self.iteration
+
+ shard_samples = self.data[self.shard_start_idx:self.shard_end_idx]
+ for i in range(self.iteration * self.batch_size, len(shard_samples), self.batch_size):
+ items = shard_samples[i:i + self.batch_size]
+ if self.strict_batch_size and len(items) < self.batch_size:
+ logger.debug('Extending batch to max size')
+ items.extend(shard_samples[0:self.batch_size - len(items)])
+ self.iteration += 1
+ yield items
+
+ # some shards may done iterating while the others are at the last batch. Just return the first batch
+ while self.iteration < max_iterations:
+ logger.debug('Fulfilling non complete shard='.format(self.shard_id))
+ self.iteration += 1
+ batch = shard_samples[0:self.batch_size]
+ yield batch
+
+ logger.debug('Finished iterating, iteration={}, shard={}'.format(self.iteration, self.shard_id))
+ # reset the iteration status
+ self.iteration = 0
+
+ def get_iteration(self) -> int:
+ return self.iteration
+
+ def apply(self, visitor_func: Callable):
+ for sample in self.data:
+ visitor_func(sample)
+
+
+def normalize_question(question: str) -> str:
+ if question[-1] == '?':
+ question = question[:-1]
+ return question
+
+
+class Tensorizer(object):
+ """
+ Component for all text to model input data conversions and related utility methods
+ """
+
+ # Note: title, if present, is supposed to be put before text (i.e. optional title + document body)
+ def text_to_tensor(self, text: str, title: str = None, add_special_tokens: bool = True):
+ raise NotImplementedError
+
+ def get_pair_separator_ids(self) -> T:
+ raise NotImplementedError
+
+ def get_pad_id(self) -> int:
+ raise NotImplementedError
+
+ def get_attn_mask(self, tokens_tensor: T):
+ raise NotImplementedError
+
+ def is_sub_word_id(self, token_id: int):
+ raise NotImplementedError
+
+ def to_string(self, token_ids, skip_special_tokens=True):
+ raise NotImplementedError
+
+ def set_pad_to_max(self, pad: bool):
+ raise NotImplementedError
diff --git a/PT-Retrieval/dpr/utils/dist_utils.py b/PT-Retrieval/dpr/utils/dist_utils.py
new file mode 100755
index 0000000..3b0bf85
--- /dev/null
+++ b/PT-Retrieval/dpr/utils/dist_utils.py
@@ -0,0 +1,96 @@
+#!/usr/bin/env python3
+# Copyright (c) Facebook, Inc. and its affiliates.
+# All rights reserved.
+#
+# This source code is licensed under the license found in the
+# LICENSE file in the root directory of this source tree.
+
+"""
+Utilities for distributed model training
+"""
+
+import pickle
+
+import torch
+import torch.distributed as dist
+
+
+def get_rank():
+ return dist.get_rank()
+
+
+def get_world_size():
+ return dist.get_world_size()
+
+
+def get_default_group():
+ return dist.group.WORLD
+
+
+def all_reduce(tensor, group=None):
+ if group is None:
+ group = get_default_group()
+ return dist.all_reduce(tensor, group=group)
+
+
+def all_gather_list(data, group=None, max_size=16384):
+ """Gathers arbitrary data from all nodes into a list.
+ Similar to :func:`~torch.distributed.all_gather` but for arbitrary Python
+ data. Note that *data* must be picklable.
+ Args:
+ data (Any): data from the local worker to be gathered on other workers
+ group (optional): group of the collective
+ """
+ SIZE_STORAGE_BYTES = 4 # int32 to encode the payload size
+
+ enc = pickle.dumps(data)
+ enc_size = len(enc)
+
+ if enc_size + SIZE_STORAGE_BYTES > max_size:
+ raise ValueError(
+ 'encoded data exceeds max_size, this can be fixed by increasing buffer size: {}'.format(enc_size))
+
+ rank = get_rank()
+ world_size = get_world_size()
+ buffer_size = max_size * world_size
+
+ if not hasattr(all_gather_list, '_buffer') or \
+ all_gather_list._buffer.numel() < buffer_size:
+ all_gather_list._buffer = torch.cuda.ByteTensor(buffer_size)
+ all_gather_list._cpu_buffer = torch.ByteTensor(max_size).pin_memory()
+
+ buffer = all_gather_list._buffer
+ buffer.zero_()
+ cpu_buffer = all_gather_list._cpu_buffer
+
+ assert enc_size < 256 ** SIZE_STORAGE_BYTES, 'Encoded object size should be less than {} bytes'.format(
+ 256 ** SIZE_STORAGE_BYTES)
+
+ size_bytes = enc_size.to_bytes(SIZE_STORAGE_BYTES, byteorder='big')
+
+ cpu_buffer[0:SIZE_STORAGE_BYTES] = torch.ByteTensor(list(size_bytes))
+ cpu_buffer[SIZE_STORAGE_BYTES: enc_size + SIZE_STORAGE_BYTES] = torch.ByteTensor(list(enc))
+
+ start = rank * max_size
+ size = enc_size + SIZE_STORAGE_BYTES
+ buffer[start: start + size].copy_(cpu_buffer[:size])
+
+ all_reduce(buffer, group=group)
+
+ try:
+ result = []
+ for i in range(world_size):
+ out_buffer = buffer[i * max_size: (i + 1) * max_size]
+ size = int.from_bytes(out_buffer[0:SIZE_STORAGE_BYTES], byteorder='big')
+ if size > 0:
+ result.append(pickle.loads(bytes(out_buffer[SIZE_STORAGE_BYTES: size + SIZE_STORAGE_BYTES].tolist())))
+ return result
+ except pickle.UnpicklingError:
+ raise Exception(
+ 'Unable to unpickle data from other workers. all_gather_list requires all '
+ 'workers to enter the function together, so this error usually indicates '
+ 'that the workers have fallen out of sync somehow. Workers can fall out of '
+ 'sync if one of them runs out of memory, or if there are other conditions '
+ 'in your training script that can cause one worker to finish an epoch '
+ 'while other workers are still iterating over their portions of the data.'
+ )
diff --git a/PT-Retrieval/dpr/utils/model_utils.py b/PT-Retrieval/dpr/utils/model_utils.py
new file mode 100755
index 0000000..6255b11
--- /dev/null
+++ b/PT-Retrieval/dpr/utils/model_utils.py
@@ -0,0 +1,143 @@
+#!/usr/bin/env python3
+# Copyright (c) Facebook, Inc. and its affiliates.
+# All rights reserved.
+#
+# This source code is licensed under the license found in the
+# LICENSE file in the root directory of this source tree.
+
+import collections
+import glob
+import logging
+import os
+from typing import List
+
+import torch
+from torch import nn
+from torch.optim.lr_scheduler import LambdaLR
+from torch.serialization import default_restore_location
+
+logger = logging.getLogger()
+
+CheckpointState = collections.namedtuple("CheckpointState",
+ ['model_dict', 'optimizer_dict', 'scheduler_dict', 'offset', 'epoch',
+ 'encoder_params'])
+
+
+def setup_for_distributed_mode(model: nn.Module, optimizer: torch.optim.Optimizer, device: object, n_gpu: int = 1,
+ local_rank: int = -1,
+ fp16: bool = False,
+ fp16_opt_level: str = "O1") -> (nn.Module, torch.optim.Optimizer):
+ model.to(device)
+ if fp16:
+ logger.info("Using fp16")
+ try:
+ import apex
+ from apex import amp
+ apex.amp.register_half_function(torch, "einsum")
+ except ImportError:
+ raise ImportError("Please install apex from https://www.github.com/nvidia/apex to use fp16 training.")
+
+ model, optimizer = amp.initialize(model, optimizer, opt_level=fp16_opt_level)
+
+ if n_gpu > 1:
+ model = torch.nn.DataParallel(model)
+
+ if local_rank != -1:
+ model = torch.nn.parallel.DistributedDataParallel(model, device_ids=[local_rank],
+ output_device=local_rank,
+ find_unused_parameters=True)
+ return model, optimizer
+
+
+def move_to_cuda(sample):
+ if len(sample) == 0:
+ return {}
+
+ def _move_to_cuda(maybe_tensor):
+ if torch.is_tensor(maybe_tensor):
+ return maybe_tensor.cuda()
+ elif isinstance(maybe_tensor, dict):
+ return {
+ key: _move_to_cuda(value)
+ for key, value in maybe_tensor.items()
+ }
+ elif isinstance(maybe_tensor, list):
+ return [_move_to_cuda(x) for x in maybe_tensor]
+ elif isinstance(maybe_tensor, tuple):
+ return [_move_to_cuda(x) for x in maybe_tensor]
+ else:
+ return maybe_tensor
+
+ return _move_to_cuda(sample)
+
+
+def move_to_device(sample, device):
+ if len(sample) == 0:
+ return {}
+
+ def _move_to_device(maybe_tensor, device):
+ if torch.is_tensor(maybe_tensor):
+ return maybe_tensor.to(device)
+ elif isinstance(maybe_tensor, dict):
+ return {
+ key: _move_to_device(value, device)
+ for key, value in maybe_tensor.items()
+ }
+ elif isinstance(maybe_tensor, list):
+ return [_move_to_device(x, device) for x in maybe_tensor]
+ elif isinstance(maybe_tensor, tuple):
+ return [_move_to_device(x, device) for x in maybe_tensor]
+ else:
+ return maybe_tensor
+
+ return _move_to_device(sample, device)
+
+
+def get_schedule_linear(optimizer, warmup_steps, training_steps, last_epoch=-1):
+ """ Create a schedule with a learning rate that decreases linearly after
+ linearly increasing during a warmup period.
+ """
+
+ def lr_lambda(current_step):
+ if current_step < warmup_steps:
+ return float(current_step) / float(max(1, warmup_steps))
+ return max(
+ 0.0, float(training_steps - current_step) / float(max(1, training_steps - warmup_steps))
+ )
+
+ return LambdaLR(optimizer, lr_lambda, last_epoch)
+
+
+def init_weights(modules: List):
+ for module in modules:
+ if isinstance(module, (nn.Linear, nn.Embedding)):
+ module.weight.data.normal_(mean=0.0, std=0.02)
+ elif isinstance(module, nn.LayerNorm):
+ module.bias.data.zero_()
+ module.weight.data.fill_(1.0)
+ if isinstance(module, nn.Linear) and module.bias is not None:
+ module.bias.data.zero_()
+
+
+def get_model_obj(model: nn.Module):
+ return model.module if hasattr(model, 'module') else model
+
+
+def get_model_file(args, file_prefix) -> str:
+ if args.model_file and os.path.exists(args.model_file):
+ return args.model_file
+
+ out_cp_files = glob.glob(os.path.join(args.output_dir, file_prefix + '*')) if args.output_dir else []
+ logger.info('Checkpoint files %s', out_cp_files)
+ model_file = None
+
+ if len(out_cp_files) > 0:
+ model_file = max(out_cp_files, key=os.path.getctime)
+ return model_file
+
+
+def load_states_from_checkpoint(model_file: str) -> CheckpointState:
+ logger.info('Reading saved model from %s', model_file)
+ state_dict = torch.load(model_file, map_location=lambda s, l: default_restore_location(s, 'cpu'))
+ logger.info('model_state_dict keys %s', state_dict.keys())
+ return CheckpointState(**state_dict)
diff --git a/PT-Retrieval/dpr/utils/tokenizers.py b/PT-Retrieval/dpr/utils/tokenizers.py
new file mode 100755
index 0000000..a5234a5
--- /dev/null
+++ b/PT-Retrieval/dpr/utils/tokenizers.py
@@ -0,0 +1,241 @@
+#!/usr/bin/env python3
+# Copyright (c) Facebook, Inc. and its affiliates.
+# All rights reserved.
+#
+# This source code is licensed under the license found in the
+# LICENSE file in the root directory of this source tree.
+
+
+"""
+Most of the tokenizers code here is copied from DrQA codebase to avoid adding extra dependency
+"""
+
+import copy
+import logging
+
+import regex
+import spacy
+
+logger = logging.getLogger(__name__)
+
+
+class Tokens(object):
+ """A class to represent a list of tokenized text."""
+ TEXT = 0
+ TEXT_WS = 1
+ SPAN = 2
+ POS = 3
+ LEMMA = 4
+ NER = 5
+
+ def __init__(self, data, annotators, opts=None):
+ self.data = data
+ self.annotators = annotators
+ self.opts = opts or {}
+
+ def __len__(self):
+ """The number of tokens."""
+ return len(self.data)
+
+ def slice(self, i=None, j=None):
+ """Return a view of the list of tokens from [i, j)."""
+ new_tokens = copy.copy(self)
+ new_tokens.data = self.data[i: j]
+ return new_tokens
+
+ def untokenize(self):
+ """Returns the original text (with whitespace reinserted)."""
+ return ''.join([t[self.TEXT_WS] for t in self.data]).strip()
+
+ def words(self, uncased=False):
+ """Returns a list of the text of each token
+
+ Args:
+ uncased: lower cases text
+ """
+ if uncased:
+ return [t[self.TEXT].lower() for t in self.data]
+ else:
+ return [t[self.TEXT] for t in self.data]
+
+ def offsets(self):
+ """Returns a list of [start, end) character offsets of each token."""
+ return [t[self.SPAN] for t in self.data]
+
+ def pos(self):
+ """Returns a list of part-of-speech tags of each token.
+ Returns None if this annotation was not included.
+ """
+ if 'pos' not in self.annotators:
+ return None
+ return [t[self.POS] for t in self.data]
+
+ def lemmas(self):
+ """Returns a list of the lemmatized text of each token.
+ Returns None if this annotation was not included.
+ """
+ if 'lemma' not in self.annotators:
+ return None
+ return [t[self.LEMMA] for t in self.data]
+
+ def entities(self):
+ """Returns a list of named-entity-recognition tags of each token.
+ Returns None if this annotation was not included.
+ """
+ if 'ner' not in self.annotators:
+ return None
+ return [t[self.NER] for t in self.data]
+
+ def ngrams(self, n=1, uncased=False, filter_fn=None, as_strings=True):
+ """Returns a list of all ngrams from length 1 to n.
+
+ Args:
+ n: upper limit of ngram length
+ uncased: lower cases text
+ filter_fn: user function that takes in an ngram list and returns
+ True or False to keep or not keep the ngram
+ as_string: return the ngram as a string vs list
+ """
+
+ def _skip(gram):
+ if not filter_fn:
+ return False
+ return filter_fn(gram)
+
+ words = self.words(uncased)
+ ngrams = [(s, e + 1)
+ for s in range(len(words))
+ for e in range(s, min(s + n, len(words)))
+ if not _skip(words[s:e + 1])]
+
+ # Concatenate into strings
+ if as_strings:
+ ngrams = ['{}'.format(' '.join(words[s:e])) for (s, e) in ngrams]
+
+ return ngrams
+
+ def entity_groups(self):
+ """Group consecutive entity tokens with the same NER tag."""
+ entities = self.entities()
+ if not entities:
+ return None
+ non_ent = self.opts.get('non_ent', 'O')
+ groups = []
+ idx = 0
+ while idx < len(entities):
+ ner_tag = entities[idx]
+ # Check for entity tag
+ if ner_tag != non_ent:
+ # Chomp the sequence
+ start = idx
+ while (idx < len(entities) and entities[idx] == ner_tag):
+ idx += 1
+ groups.append((self.slice(start, idx).untokenize(), ner_tag))
+ else:
+ idx += 1
+ return groups
+
+
+class Tokenizer(object):
+ """Base tokenizer class.
+ Tokenizers implement tokenize, which should return a Tokens class.
+ """
+
+ def tokenize(self, text):
+ raise NotImplementedError
+
+ def shutdown(self):
+ pass
+
+ def __del__(self):
+ self.shutdown()
+
+
+class SimpleTokenizer(Tokenizer):
+ ALPHA_NUM = r'[\p{L}\p{N}\p{M}]+'
+ NON_WS = r'[^\p{Z}\p{C}]'
+
+ def __init__(self, **kwargs):
+ """
+ Args:
+ annotators: None or empty set (only tokenizes).
+ """
+ self._regexp = regex.compile(
+ '(%s)|(%s)' % (self.ALPHA_NUM, self.NON_WS),
+ flags=regex.IGNORECASE + regex.UNICODE + regex.MULTILINE
+ )
+ if len(kwargs.get('annotators', {})) > 0:
+ logger.warning('%s only tokenizes! Skipping annotators: %s' %
+ (type(self).__name__, kwargs.get('annotators')))
+ self.annotators = set()
+
+ def tokenize(self, text):
+ data = []
+ matches = [m for m in self._regexp.finditer(text)]
+ for i in range(len(matches)):
+ # Get text
+ token = matches[i].group()
+
+ # Get whitespace
+ span = matches[i].span()
+ start_ws = span[0]
+ if i + 1 < len(matches):
+ end_ws = matches[i + 1].span()[0]
+ else:
+ end_ws = span[1]
+
+ # Format data
+ data.append((
+ token,
+ text[start_ws: end_ws],
+ span,
+ ))
+ return Tokens(data, self.annotators)
+
+
+class SpacyTokenizer(Tokenizer):
+
+ def __init__(self, **kwargs):
+ """
+ Args:
+ annotators: set that can include pos, lemma, and ner.
+ model: spaCy model to use (either path, or keyword like 'en').
+ """
+ model = kwargs.get('model', 'en')
+ self.annotators = copy.deepcopy(kwargs.get('annotators', set()))
+ nlp_kwargs = {'parser': False}
+ if not any([p in self.annotators for p in ['lemma', 'pos', 'ner']]):
+ nlp_kwargs['tagger'] = False
+ if 'ner' not in self.annotators:
+ nlp_kwargs['entity'] = False
+ self.nlp = spacy.load(model, **nlp_kwargs)
+
+ def tokenize(self, text):
+ # We don't treat new lines as tokens.
+ clean_text = text.replace('\n', ' ')
+ tokens = self.nlp.tokenizer(clean_text)
+ if any([p in self.annotators for p in ['lemma', 'pos', 'ner']]):
+ self.nlp.tagger(tokens)
+ if 'ner' in self.annotators:
+ self.nlp.entity(tokens)
+
+ data = []
+ for i in range(len(tokens)):
+ # Get whitespace
+ start_ws = tokens[i].idx
+ if i + 1 < len(tokens):
+ end_ws = tokens[i + 1].idx
+ else:
+ end_ws = tokens[i].idx + len(tokens[i].text)
+
+ data.append((
+ tokens[i].text,
+ text[start_ws: end_ws],
+ (tokens[i].idx, tokens[i].idx + len(tokens[i].text)),
+ tokens[i].tag_,
+ tokens[i].lemma_,
+ tokens[i].ent_type_,
+ ))
+
+ # Set special option for non-entity tag: '' vs 'O' in spaCy
+ return Tokens(data, self.annotators, opts={'non_ent': ''})
diff --git a/PT-Retrieval/eval_scripts/calibration_on_beir.sh b/PT-Retrieval/eval_scripts/calibration_on_beir.sh
new file mode 100644
index 0000000..624aa93
--- /dev/null
+++ b/PT-Retrieval/eval_scripts/calibration_on_beir.sh
@@ -0,0 +1,21 @@
+model_cfg=bert-base-uncased
+filename=ptv2-dpr-multidata-128-40-8e-3-64
+checkpoint=dpr_biencoder.39.1045
+psl=64
+
+dataset=$1
+
+if [ "$dataset" = "msmarco" ]; then
+ split="dev"
+else
+ split="test"
+fi
+
+python3 calibration/calibration_beir.py \
+ --pretrained_model_cfg $model_cfg \
+ --model_file ./checkpoints/$filename/$checkpoint \
+ --batch_size 128 \
+ --sequence_length 512 \
+ --prefix --pre_seq_len $psl \
+ --dataset $dataset --split $split
+
diff --git a/PT-Retrieval/eval_scripts/calibration_on_openqa.sh b/PT-Retrieval/eval_scripts/calibration_on_openqa.sh
new file mode 100755
index 0000000..2b867e8
--- /dev/null
+++ b/PT-Retrieval/eval_scripts/calibration_on_openqa.sh
@@ -0,0 +1,27 @@
+model_cfg=bert-base-uncased
+filename=ptv2-dpr-multidata-128-40-8e-3-64
+checkpoint=dpr_biencoder.39.1045
+psl=64
+
+dataset=$1
+
+echo "Ploy Calibration Curve"
+python3 calibration/calibration_plot_openqa.py \
+ --pretrained_model_cfg $model_cfg \
+ --model_file ./checkpoints/$filename/$checkpoint \
+ --batch_size 128 \
+ --sequence_length 512 \
+ --prefix --pre_seq_len $psl \
+ --dataset $dataset
+
+echo "Calculate ECE"
+python3 calibration/calibration_ece_openqa.py \
+ --pretrained_model_cfg $model_cfg \
+ --model_file checkpoints/$filename/$checkpoint \
+ --ctx_file data/wikipedia_split/psgs_w100.tsv \
+ --encoded_ctx_file "encoded_files/encoded-wiki-$filename-*.pkl" \
+ --n-docs 5 \
+ --batch_size 128 \
+ --sequence_length 256 \
+ --prefix --pre_seq_len $psl \
+ --dataset $dataset
\ No newline at end of file
diff --git a/PT-Retrieval/eval_scripts/evaluate_on_beir.sh b/PT-Retrieval/eval_scripts/evaluate_on_beir.sh
new file mode 100755
index 0000000..90aed1d
--- /dev/null
+++ b/PT-Retrieval/eval_scripts/evaluate_on_beir.sh
@@ -0,0 +1,21 @@
+model_cfg=bert-base-uncased
+filename=ptv2-dpr-multidata-128-40-8e-3-100
+checkpoint=dpr_biencoder.39.1045
+psl=100
+
+dataset=$1
+
+if [ "$dataset" = "msmarco" ]; then
+ split="dev"
+else
+ split="test"
+fi
+
+CUDA_VISIBLE_DEVICES=$2 python3 beir_eval/evaluate_dpr_on_beir.py \
+ --pretrained_model_cfg $model_cfg \
+ --model_file ./checkpoints/$filename/$checkpoint \
+ --batch_size 128 \
+ --sequence_length 512 \
+ --prefix --pre_seq_len $psl \
+ --dataset $dataset --split $split
+
diff --git a/PT-Retrieval/eval_scripts/evaluate_on_oagqa.sh b/PT-Retrieval/eval_scripts/evaluate_on_oagqa.sh
new file mode 100755
index 0000000..d84889e
--- /dev/null
+++ b/PT-Retrieval/eval_scripts/evaluate_on_oagqa.sh
@@ -0,0 +1,29 @@
+model_cfg=bert-base-uncased
+filename=ptv2-dpr-multidata-128-40-8e-3-64
+checkpoint=dpr_biencoder.39.1045
+psl=64
+
+topic=$1
+topk=$2
+
+python3 generate_dense_embeddings.py \
+ --pretrained_model_cfg $model_cfg \
+ --model_file checkpoints/$filename/$checkpoint \
+ --shard_id 0 --num_shards 1 \
+ --ctx_file data/oagqa-topic-v2/$topic-papers-10k.tsv \
+ --out_file encoded_files/encoded-oagqa-$topic-$filename \
+ --batch_size 128 \
+ --sequence_length 256 \
+ --prefix --pre_seq_len $psl
+
+python3 dense_retriever.py \
+ --pretrained_model_cfg $model_cfg \
+ --model_file checkpoints/$filename/$checkpoint \
+ --ctx_file data/oagqa-topic-v2/$topic-papers-10k.tsv \
+ --qa_file data/oagqa-topic-v2/$topic-questions.tsv \
+ --encoded_ctx_file "encoded_files/encoded-oagqa-$topic-$filename-*.pkl" \
+ --n-docs $topk \
+ --batch_size 128 \
+ --sequence_length 256 \
+ --oagqa \
+ --prefix --pre_seq_len $psl
diff --git a/PT-Retrieval/eval_scripts/evaluate_on_openqa.sh b/PT-Retrieval/eval_scripts/evaluate_on_openqa.sh
new file mode 100755
index 0000000..fc858b3
--- /dev/null
+++ b/PT-Retrieval/eval_scripts/evaluate_on_openqa.sh
@@ -0,0 +1,26 @@
+model_cfg=bert-base-uncased
+filename=ptv2-dpr-multidata-128-40-8e-3-64
+checkpoint=dpr_biencoder.39.1045
+psl=64
+
+dataset=$1
+topk=$2
+
+if [ "$dataset" = "curatedtrec" ]; then
+ match="regex"
+else
+ match="string"
+fi
+
+python3 dense_retriever.py \
+ --pretrained_model_cfg $model_cfg \
+ --model_file checkpoints/$filename/$checkpoint \
+ --ctx_file data/wikipedia_split/psgs_w100.tsv \
+ --qa_file data/retriever/qas/$dataset-test.csv \
+ --encoded_ctx_file "encoded_files/encoded-wiki-$filename-*.pkl" \
+ --n-docs $topk \
+ --batch_size 128 \
+ --sequence_length 256 \
+ --prefix --pre_seq_len $psl --match $match
+
+# add "--match regex" for CuratedTREC
\ No newline at end of file
diff --git a/PT-Retrieval/eval_scripts/generate_wiki_embeddings.sh b/PT-Retrieval/eval_scripts/generate_wiki_embeddings.sh
new file mode 100755
index 0000000..640e91a
--- /dev/null
+++ b/PT-Retrieval/eval_scripts/generate_wiki_embeddings.sh
@@ -0,0 +1,26 @@
+model_cfg=bert-base-uncased
+filename=ptv2-dpr-multidata-128-40-8e-3-64
+checkpoint=dpr_biencoder.39.1045
+psl=64
+
+run_func() {
+CUDA_VISIBLE_DEVICES=$1 python3 generate_dense_embeddings.py \
+ --pretrained_model_cfg $model_cfg \
+ --model_file checkpoints/$filename/$checkpoint \
+ --shard_id $2 --num_shards 8 \
+ --ctx_file data/wikipedia_split/psgs_w100.tsv \
+ --out_file encoded_files/encoded-wiki-$filename \
+ --batch_size 128 \
+ --sequence_length 256 \
+ --prefix --pre_seq_len $psl
+}
+
+
+for node in 0 1 2 3 4 5 6 7
+do
+ shard_id=$((node))
+ run_func $node $shard_id &
+done
+
+wait
+echo "All done"
diff --git a/PT-Retrieval/figures/PT-Retrieval.png b/PT-Retrieval/figures/PT-Retrieval.png
new file mode 100644
index 0000000..938f9e2
Binary files /dev/null and b/PT-Retrieval/figures/PT-Retrieval.png differ
diff --git a/PT-Retrieval/generate_dense_embeddings.py b/PT-Retrieval/generate_dense_embeddings.py
new file mode 100755
index 0000000..71cfd5f
--- /dev/null
+++ b/PT-Retrieval/generate_dense_embeddings.py
@@ -0,0 +1,154 @@
+#!/usr/bin/env python3
+# Copyright (c) Facebook, Inc. and its affiliates.
+# All rights reserved.
+#
+# This source code is licensed under the license found in the
+# LICENSE file in the root directory of this source tree.
+
+"""
+ Command line tool that produces embeddings for a large documents base based on the pretrained ctx & question encoders
+ Supposed to be used in a 'sharded' way to speed up the process.
+"""
+import os
+import pathlib
+
+import argparse
+import csv
+import logging
+import pickle
+from typing import List, Tuple
+import codecs
+from tqdm import tqdm
+
+import numpy as np
+import torch
+from torch import nn
+
+from dpr.models import init_encoder_components
+from dpr.options import add_encoder_params, setup_args_gpu, print_args, set_encoder_params_from_state, \
+ add_tokenizer_params, add_cuda_params, add_tuning_params
+from dpr.utils.data_utils import Tensorizer
+from dpr.utils.model_utils import setup_for_distributed_mode, get_model_obj, load_states_from_checkpoint,move_to_device
+
+logger = logging.getLogger()
+logger.setLevel(logging.INFO)
+if (logger.hasHandlers()):
+ logger.handlers.clear()
+console = logging.StreamHandler()
+logger.addHandler(console)
+
+csv.field_size_limit(10000000)
+def gen_ctx_vectors(ctx_rows: List[Tuple[object, str, str]], model: nn.Module, tensorizer: Tensorizer,
+ args, insert_title: bool = True) -> List[Tuple[object, np.array]]:
+ n = len(ctx_rows)
+ bsz = args.batch_size
+ total = 0
+ results = []
+ for j, batch_start in tqdm(enumerate(range(0, n, bsz)), total=round(n/bsz)):
+
+ batch_token_tensors = [tensorizer.text_to_tensor(ctx[1], title=ctx[2] if insert_title else None) for ctx in
+ ctx_rows[batch_start:batch_start + bsz]]
+
+ ctx_ids_batch = move_to_device(torch.stack(batch_token_tensors, dim=0),args.device)
+ ctx_seg_batch = move_to_device(torch.zeros_like(ctx_ids_batch),args.device)
+ ctx_attn_mask = move_to_device(tensorizer.get_attn_mask(ctx_ids_batch),args.device)
+ with torch.no_grad():
+ _, out, _ = model(ctx_ids_batch, ctx_seg_batch, ctx_attn_mask)
+ out = out.cpu()
+
+ ctx_ids = [r[0] for r in ctx_rows[batch_start:batch_start + bsz]]
+
+ assert len(ctx_ids) == out.size(0)
+
+ total += len(ctx_ids)
+
+ results.extend([
+ (ctx_ids[i], out[i].view(-1).numpy())
+ for i in range(out.size(0))
+ ])
+
+ return results
+
+
+def main(args):
+ if not args.adapter:
+ saved_state = load_states_from_checkpoint(args.model_file)
+ set_encoder_params_from_state(saved_state.encoder_params, args)
+ print_args(args)
+
+ tensorizer, encoder, _ = init_encoder_components("dpr", args, inference_only=True)
+
+ encoder = encoder.ctx_model
+
+ encoder, _ = setup_for_distributed_mode(encoder, None, args.device, args.n_gpu,
+ args.local_rank,
+ args.fp16,
+ args.fp16_opt_level)
+ encoder.eval()
+
+ # load weights from the model file
+ model_to_load = get_model_obj(encoder)
+ logger.info('Loading saved model state ...')
+ if args.adapter:
+ adapter_name = model_to_load.load_adapter(args.model_file+".q")
+ model_to_load.set_active_adapters(adapter_name)
+ else:
+ logger.debug('saved model keys =%s', saved_state.model_dict.keys())
+
+ encoder_name = "ctx_model."
+ prefix_len = len(encoder_name)
+ if args.prefix or args.prompt:
+ encoder_name += "prefix_encoder."
+
+ ctx_state = {key[prefix_len:]: value for (key, value) in saved_state.model_dict.items() if
+ key.startswith(encoder_name)}
+ model_to_load.load_state_dict(ctx_state, strict=not args.prefix and not args.prompt)
+
+ logger.info('reading data from file=%s', args.ctx_file)
+
+ rows = []
+ with open(args.ctx_file) as tsvfile:
+ reader = csv.reader(tsvfile, delimiter='\t')
+ # file format: doc_id, doc_text, title
+ rows.extend([(row[0], row[1], row[2]) for row in reader if row[0] != 'id'])
+
+ shard_size = int(len(rows) / args.num_shards)
+ start_idx = args.shard_id * shard_size
+ end_idx = start_idx + shard_size
+
+ logger.info('Producing encodings for passages range: %d to %d (out of total %d)', start_idx, end_idx, len(rows))
+ rows = rows[start_idx:end_idx]
+
+ data = gen_ctx_vectors(rows, encoder, tensorizer, args, True)
+
+ file = args.out_file + '-' + str(args.shard_id) + '.pkl'
+ pathlib.Path(os.path.dirname(file)).mkdir(parents=True, exist_ok=True)
+ logger.info('Writing results to %s' % file)
+ with open(file, mode='wb') as f:
+ pickle.dump(data, f)
+
+ logger.info('Total passages processed %d. Written to %s', len(data), file)
+
+
+if __name__ == '__main__':
+ parser = argparse.ArgumentParser()
+
+ add_encoder_params(parser)
+ add_tokenizer_params(parser)
+ add_cuda_params(parser)
+ add_tuning_params(parser)
+
+ parser.add_argument('--ctx_file', type=str, default=None, help='Path to passages set .tsv file')
+ parser.add_argument('--out_file', required=True, type=str, default=None,
+ help='output file path to write results to')
+ parser.add_argument('--shard_id', type=int, default=0, help="Number(0-based) of data shard to process")
+ parser.add_argument('--num_shards', type=int, default=1, help="Total amount of data shards")
+ parser.add_argument('--batch_size', type=int, default=32, help="Batch size for the passage encoder forward pass")
+ args = parser.parse_args()
+
+ assert args.model_file, 'Please specify --model_file checkpoint to init model weights'
+
+ setup_args_gpu(args)
+
+
+ main(args)
diff --git a/PT-Retrieval/requirements.txt b/PT-Retrieval/requirements.txt
new file mode 100644
index 0000000..bc92d70
--- /dev/null
+++ b/PT-Retrieval/requirements.txt
@@ -0,0 +1,16 @@
+beir==1.0.0
+GitPython==3.1.27
+matplotlib==3.5.2
+mlflow==1.23.0
+numpy==1.22.3
+packaging==21.3
+regex==2021.8.21
+scikit_learn==1.1.1
+scipy==1.8.1
+setuptools==61.2.0
+spacy==3.3.1
+torch==1.12.0
+tqdm==4.64.0
+transformers==4.20.1
+ujson==5.4.0
+wget==3.2
\ No newline at end of file
diff --git a/PT-Retrieval/run_scripts/run_train_dpr_multidata_adapter.sh b/PT-Retrieval/run_scripts/run_train_dpr_multidata_adapter.sh
new file mode 100755
index 0000000..0d182ad
--- /dev/null
+++ b/PT-Retrieval/run_scripts/run_train_dpr_multidata_adapter.sh
@@ -0,0 +1,23 @@
+model_cfg=bert-base-uncased
+bs=128
+epoch=40
+lr=1e-4
+filename=ad-dpr-multidata-$bs-$epoch-$lr
+
+python3 train_dense_encoder.py \
+ --pretrained_model_cfg $model_cfg \
+ --train_file "data/retriever/*-train.json" \
+ --dev_file "data/retriever/*-dev.json" \
+ --output_dir checkpoints/$filename \
+ --seed 12345 \
+ --do_lower_case \
+ --max_grad_norm 2.0 \
+ --sequence_length 256 \
+ --warmup_percentage 0.05 \
+ --val_av_rank_start_epoch 30 \
+ --batch_size $bs \
+ --learning_rate $lr \
+ --num_train_epochs $epoch \
+ --dev_batch_size $bs \
+ --hard_negatives 1 \
+ --adapter
diff --git a/PT-Retrieval/run_scripts/run_train_dpr_multidata_bitfit.sh b/PT-Retrieval/run_scripts/run_train_dpr_multidata_bitfit.sh
new file mode 100755
index 0000000..ccd0f0c
--- /dev/null
+++ b/PT-Retrieval/run_scripts/run_train_dpr_multidata_bitfit.sh
@@ -0,0 +1,23 @@
+model_cfg=bert-base-uncased
+bs=128
+epoch=40
+lr=1e-2
+filename=bf-dpr-multidata-$bs-$epoch-$lr
+
+python3 train_dense_encoder.py \
+ --pretrained_model_cfg $model_cfg \
+ --train_file "data/retriever/*-train.json" \
+ --dev_file "data/retriever/*-dev.json" \
+ --output_dir checkpoints/$filename \
+ --seed 12345 \
+ --do_lower_case \
+ --max_grad_norm 2.0 \
+ --sequence_length 256 \
+ --warmup_percentage 0.05 \
+ --val_av_rank_start_epoch 30 \
+ --batch_size $bs \
+ --learning_rate $lr \
+ --num_train_epochs $epoch \
+ --dev_batch_size $bs \
+ --hard_negatives 1 \
+ --bitfit
diff --git a/PT-Retrieval/run_scripts/run_train_dpr_multidata_finetune.sh b/PT-Retrieval/run_scripts/run_train_dpr_multidata_finetune.sh
new file mode 100755
index 0000000..ef1a5e5
--- /dev/null
+++ b/PT-Retrieval/run_scripts/run_train_dpr_multidata_finetune.sh
@@ -0,0 +1,22 @@
+model_cfg=bert-base-uncased
+bs=128
+epoch=40
+lr=1e-5
+filename=ft-dpr-multidata-$bs-$epoch-$lr
+
+python3 train_dense_encoder.py \
+ --pretrained_model_cfg $model_cfg \
+ --train_file "data/retriever/*-train.json" \
+ --dev_file "data/retriever/*-dev.json" \
+ --output_dir checkpoints/$filename \
+ --seed 12345 \
+ --do_lower_case \
+ --max_grad_norm 2.0 \
+ --sequence_length 256 \
+ --warmup_percentage 0.05 \
+ --val_av_rank_start_epoch 30 \
+ --batch_size $bs \
+ --learning_rate $lr \
+ --num_train_epochs $epoch \
+ --dev_batch_size $bs \
+ --hard_negatives 1
diff --git a/PT-Retrieval/run_scripts/run_train_dpr_multidata_prompt.sh b/PT-Retrieval/run_scripts/run_train_dpr_multidata_prompt.sh
new file mode 100755
index 0000000..eec9728
--- /dev/null
+++ b/PT-Retrieval/run_scripts/run_train_dpr_multidata_prompt.sh
@@ -0,0 +1,24 @@
+model_cfg=bert-base-uncased
+bs=128
+epoch=40
+lr=1e-2
+psl=100
+filename=pt-dpr-multidata-$bs-$epoch-$lr-$psl
+
+python3 train_dense_encoder.py \
+ --pretrained_model_cfg $model_cfg \
+ --train_file "data/retriever/*-train.json" \
+ --dev_file "data/retriever/*-dev.json" \
+ --output_dir checkpoints/$filename \
+ --seed 12345 \
+ --do_lower_case \
+ --max_grad_norm 2.0 \
+ --sequence_length 256 \
+ --warmup_percentage 0.05 \
+ --val_av_rank_start_epoch 30 \
+ --batch_size $bs \
+ --learning_rate $lr \
+ --num_train_epochs $epoch \
+ --dev_batch_size $bs \
+ --hard_negatives 1 \
+ --prompt --pre_seq_len $psl
diff --git a/PT-Retrieval/run_scripts/run_train_dpr_multidata_ptv2.sh b/PT-Retrieval/run_scripts/run_train_dpr_multidata_ptv2.sh
new file mode 100755
index 0000000..8c6f9a8
--- /dev/null
+++ b/PT-Retrieval/run_scripts/run_train_dpr_multidata_ptv2.sh
@@ -0,0 +1,24 @@
+model_cfg=bert-base-uncased
+bs=128
+epoch=40
+lr=1e-2
+psl=64
+filename=ptv2-dpr-multidata-$bs-$epoch-$lr-$psl
+
+python3 train_dense_encoder.py \
+ --pretrained_model_cfg $model_cfg \
+ --train_file "data/retriever/*-train.json" \
+ --dev_file "data/retriever/*-dev.json" \
+ --output_dir checkpoints/$filename \
+ --seed 12345 \
+ --do_lower_case \
+ --max_grad_norm 2.0 \
+ --sequence_length 256 \
+ --warmup_percentage 0.05 \
+ --val_av_rank_start_epoch 30 \
+ --batch_size $bs \
+ --learning_rate $lr \
+ --num_train_epochs $epoch \
+ --dev_batch_size $bs \
+ --hard_negatives 1 \
+ --prefix --pre_seq_len $psl
\ No newline at end of file
diff --git a/PT-Retrieval/train_dense_encoder.py b/PT-Retrieval/train_dense_encoder.py
new file mode 100755
index 0000000..1c4664c
--- /dev/null
+++ b/PT-Retrieval/train_dense_encoder.py
@@ -0,0 +1,613 @@
+#!/usr/bin/env python3
+# Copyright (c) Facebook, Inc. and its affiliates.
+# All rights reserved.
+#
+# This source code is licensed under the license found in the
+# LICENSE file in the root directory of this source tree.
+
+
+"""
+ Pipeline to train DPR Biencoder
+"""
+
+import argparse
+import glob
+import logging
+import math
+import os
+import random
+import time
+
+import torch
+
+from typing import Tuple
+from torch import nn
+from torch import Tensor as T
+
+from dpr.models import init_encoder_components
+from dpr.models.biencoder import BiEncoder, BiEncoderNllLoss, BiEncoderBatch
+from dpr.options import add_encoder_params, add_training_params, setup_args_gpu, set_seed, print_args, \
+ get_encoder_params_state, add_tokenizer_params, set_encoder_params_from_state, add_tuning_params
+from dpr.utils.data_utils import ShardedDataIterator, read_data_from_json_files, Tensorizer
+from dpr.utils.dist_utils import all_gather_list
+from dpr.utils.model_utils import setup_for_distributed_mode, move_to_device, get_schedule_linear, CheckpointState, \
+ get_model_file, get_model_obj, load_states_from_checkpoint
+
+logger = logging.getLogger()
+logger.setLevel(logging.INFO)
+if (logger.hasHandlers()):
+ logger.handlers.clear()
+console = logging.StreamHandler()
+logger.addHandler(console)
+
+
+class BiEncoderTrainer(object):
+ """
+ BiEncoder training pipeline component. Can be used to initiate or resume training and validate the trained model
+ using either binary classification's NLL loss or average rank of the question's gold passages across dataset
+ provided pools of negative passages. For full IR accuracy evaluation, please see generate_dense_embeddings.py
+ and dense_retriever.py CLI tools.
+ """
+
+ def __init__(self, args):
+ self.args = args
+
+ self.prefix = args.prefix
+ self.prompt = args.prompt
+ self.adapter = args.adapter
+
+ self.shard_id = args.local_rank if args.local_rank != -1 else 0
+ self.distributed_factor = args.distributed_world_size or 1
+
+ logger.info("***** Initializing components for training *****")
+
+ # if model file is specified, encoder parameters from saved state should be used for initialization
+ model_file = get_model_file(self.args, self.args.checkpoint_file_name)
+ saved_state = None
+ if model_file and not self.adapter:
+ saved_state = load_states_from_checkpoint(model_file)
+ set_encoder_params_from_state(saved_state.encoder_params, args)
+
+
+ tensorizer, model, optimizer = init_encoder_components("dpr", args)
+
+ model, optimizer = setup_for_distributed_mode(model, optimizer, args.device, args.n_gpu,
+ args.local_rank,
+ args.fp16,
+ args.fp16_opt_level)
+ self.biencoder = model
+ self.optimizer = optimizer
+ self.tensorizer = tensorizer
+ self.start_epoch = 0
+ self.start_batch = 0
+ self.scheduler_state = None
+ self.best_validation_result = None
+ self.best_cp_name = None
+
+ if saved_state:
+ self._load_saved_state(saved_state)
+
+ def get_data_iterator(self, path: str, batch_size: int, shuffle=True,
+ shuffle_seed: int = 0,
+ offset: int = 0, upsample_rates: list = None) -> ShardedDataIterator:
+ data_files = glob.glob(path)
+
+ if len(data_files) > 1:
+ upsample_rates = []
+ for path in data_files:
+ if "trec" in path or "webq" in path:
+ upsample_rates.append(4)
+ else:
+ upsample_rates.append(1)
+ print("upsample ratse =", upsample_rates)
+
+ data = read_data_from_json_files(data_files, upsample_rates)
+
+ # filter those without positive ctx
+ data = [r for r in data if len(r['positive_ctxs']) > 0]
+ logger.info('Total cleaned data size: {}'.format(len(data)))
+
+ return ShardedDataIterator(data, shard_id=self.shard_id,
+ num_shards=self.distributed_factor,
+ batch_size=batch_size, shuffle=shuffle, shuffle_seed=shuffle_seed, offset=offset,
+ strict_batch_size=True, # this is not really necessary, one can probably disable it
+ )
+
+ def run_train(self, ):
+ args = self.args
+ upsample_rates = None
+ if args.train_files_upsample_rates is not None:
+ upsample_rates = eval(args.train_files_upsample_rates)
+
+ train_iterator = self.get_data_iterator(args.train_file, args.batch_size,
+ shuffle=True,
+ shuffle_seed=args.seed, offset=self.start_batch,
+ upsample_rates=upsample_rates)
+
+ logger.info(" Total iterations per epoch=%d", train_iterator.max_iterations)
+ updates_per_epoch = train_iterator.max_iterations // args.gradient_accumulation_steps
+ total_updates = max(updates_per_epoch * (args.num_train_epochs - self.start_epoch - 1), 0) + \
+ (train_iterator.max_iterations - self.start_batch) // args.gradient_accumulation_steps
+ logger.info(" Total updates=%d", total_updates)
+ if args.warmup_percentage is not None:
+ warmup_steps = round(args.warmup_percentage * total_updates)
+ else:
+ warmup_steps = args.warmup_steps
+ logger.info(" Total warmup=%d", warmup_steps)
+ scheduler = get_schedule_linear(self.optimizer, warmup_steps, total_updates)
+
+ if self.scheduler_state:
+ logger.info("Loading scheduler state %s", self.scheduler_state)
+ scheduler.load_state_dict(self.scheduler_state)
+
+ eval_step = math.ceil(updates_per_epoch / args.eval_per_epoch)
+ logger.info(" Eval step = %d", eval_step)
+ logger.info("***** Training *****")
+
+ for epoch in range(self.start_epoch, int(args.num_train_epochs)):
+ logger.info("***** Epoch %d *****", epoch)
+ self._train_epoch(scheduler, epoch, eval_step, train_iterator)
+
+ if args.local_rank in [-1, 0]:
+ logger.info('Training finished. Best validation checkpoint %s', self.best_cp_name)
+
+ def validate_and_save(self, epoch: int, iteration: int, scheduler):
+ args = self.args
+ # for distributed mode, save checkpoint for only one process
+ save_cp = args.local_rank in [-1, 0]
+
+ if epoch == args.val_av_rank_start_epoch:
+ self.best_validation_result = None
+
+ if epoch >= args.val_av_rank_start_epoch:
+ validation_loss = self.validate_average_rank()
+ else:
+ validation_loss = self.validate_nll()
+
+ if save_cp:
+ cp_name = self._save_checkpoint(scheduler, epoch, iteration)
+ logger.info('Saved checkpoint to %s', cp_name)
+
+ if validation_loss < (self.best_validation_result or validation_loss + 1):
+ self.best_validation_result = validation_loss
+ self.best_cp_name = cp_name
+ logger.info('New Best validation checkpoint %s', cp_name)
+
+ def validate_nll(self) -> float:
+ logger.info('NLL validation ...')
+ args = self.args
+ self.biencoder.eval()
+ data_iterator = self.get_data_iterator(args.dev_file, args.dev_batch_size, shuffle=False)
+
+ total_loss = 0.0
+ start_time = time.time()
+ total_correct_predictions = 0
+ num_hard_negatives = args.hard_negatives
+ num_other_negatives = args.other_negatives
+ log_result_step = args.log_batch_step
+ batches = 0
+ for i, samples_batch in enumerate(data_iterator.iterate_data()):
+ biencoder_input = BiEncoder.create_biencoder_input(samples_batch, self.tensorizer, self.args.ctx_field,
+ True,
+ num_hard_negatives, num_other_negatives, shuffle=False)
+
+ loss, correct_cnt = _do_biencoder_fwd_pass(self.biencoder, biencoder_input, self.tensorizer, args)
+ total_loss += loss.item()
+ total_correct_predictions += correct_cnt
+ batches += 1
+ if (i + 1) % log_result_step == 0:
+ logger.info('Eval step: %d , used_time=%f sec., loss=%f ', i, time.time() - start_time, loss.item())
+
+ total_loss = total_loss / batches
+ total_samples = batches * args.dev_batch_size * self.distributed_factor
+ correct_ratio = float(total_correct_predictions / total_samples)
+ logger.info('NLL Validation: loss = %f. correct prediction ratio %d/%d ~ %f', total_loss,
+ total_correct_predictions,
+ total_samples,
+ correct_ratio
+ )
+ return total_loss
+
+ def validate_average_rank(self) -> float:
+ """
+ Validates biencoder model using each question's gold passage's rank across the set of passages from the dataset.
+ It generates vectors for specified amount of negative passages from each question (see --val_av_rank_xxx params)
+ and stores them in RAM as well as question vectors.
+ Then the similarity scores are calculted for the entire
+ num_questions x (num_questions x num_passages_per_question) matrix and sorted per quesrtion.
+ Each question's gold passage rank in that sorted list of scores is averaged across all the questions.
+ :return: averaged rank number
+ """
+ logger.info('Average rank validation ...')
+
+ args = self.args
+ self.biencoder.eval()
+ distributed_factor = self.distributed_factor
+
+ data_iterator = self.get_data_iterator(args.dev_file, args.dev_batch_size, shuffle=False)
+
+ sub_batch_size = args.val_av_rank_bsz
+ sim_score_f = BiEncoderNllLoss.get_similarity_function()
+ q_represenations = []
+ ctx_represenations = []
+ positive_idx_per_question = []
+
+ num_hard_negatives = args.val_av_rank_hard_neg
+ num_other_negatives = args.val_av_rank_other_neg
+
+ log_result_step = args.log_batch_step
+
+ for i, samples_batch in enumerate(data_iterator.iterate_data()):
+ # samples += 1
+ if len(q_represenations) > args.val_av_rank_max_qs / distributed_factor:
+ break
+
+ biencoder_input = BiEncoder.create_biencoder_input(samples_batch, self.tensorizer, self.args.ctx_field,
+ True,
+ num_hard_negatives, num_other_negatives, shuffle=False)
+ total_ctxs = len(ctx_represenations)
+ ctxs_ids = biencoder_input.context_ids
+ ctxs_segments = biencoder_input.ctx_segments
+ bsz = ctxs_ids.size(0)
+
+ # split contexts batch into sub batches since it is supposed to be too large to be processed in one batch
+ for j, batch_start in enumerate(range(0, bsz, sub_batch_size)):
+
+ q_ids, q_segments = (biencoder_input.question_ids, biencoder_input.question_segments) if j == 0 \
+ else (None, None)
+
+ if j == 0 and args.n_gpu > 1 and q_ids.size(0) == 1:
+ # if we are in DP (but not in DDP) mode, all model input tensors should have batch size >1 or 0,
+ # otherwise the other input tensors will be split but only the first split will be called
+ continue
+
+ ctx_ids_batch = ctxs_ids[batch_start:batch_start + sub_batch_size]
+ ctx_seg_batch = ctxs_segments[batch_start:batch_start + sub_batch_size]
+
+ q_attn_mask = self.tensorizer.get_attn_mask(q_ids)
+ ctx_attn_mask = self.tensorizer.get_attn_mask(ctx_ids_batch)
+ with torch.no_grad():
+ q_dense, ctx_dense = self.biencoder(q_ids, q_segments, q_attn_mask, ctx_ids_batch, ctx_seg_batch,
+ ctx_attn_mask)
+
+ if q_dense is not None:
+ q_represenations.extend(q_dense.cpu().split(1, dim=0))
+
+ ctx_represenations.extend(ctx_dense.cpu().split(1, dim=0))
+
+ batch_positive_idxs = biencoder_input.is_positive
+ positive_idx_per_question.extend([total_ctxs + v for v in batch_positive_idxs])
+
+ if (i + 1) % log_result_step == 0:
+ logger.info('Av.rank validation: step %d, computed ctx_vectors %d, q_vectors %d', i,
+ len(ctx_represenations), len(q_represenations))
+
+ ctx_represenations = torch.cat(ctx_represenations, dim=0)
+ q_represenations = torch.cat(q_represenations, dim=0)
+
+ logger.info('Av.rank validation: total q_vectors size=%s', q_represenations.size())
+ logger.info('Av.rank validation: total ctx_vectors size=%s', ctx_represenations.size())
+
+ q_num = q_represenations.size(0)
+ assert q_num == len(positive_idx_per_question)
+
+ scores = sim_score_f(q_represenations, ctx_represenations)
+ values, indices = torch.sort(scores, dim=1, descending=True)
+
+ rank = 0
+ for i, idx in enumerate(positive_idx_per_question):
+ # aggregate the rank of the known gold passage in the sorted results for each question
+ gold_idx = (indices[i] == idx).nonzero()
+ rank += gold_idx.item()
+
+ if distributed_factor > 1:
+ # each node calcuated its own rank, exchange the information between node and calculate the "global" average rank
+ # NOTE: the set of passages is still unique for every node
+ eval_stats = all_gather_list([rank, q_num], max_size=100)
+ for i, item in enumerate(eval_stats):
+ remote_rank, remote_q_num = item
+ if i != args.local_rank:
+ rank += remote_rank
+ q_num += remote_q_num
+
+ av_rank = float(rank / q_num)
+ logger.info('Av.rank validation: average rank %s, total questions=%d', av_rank, q_num)
+ return av_rank
+
+ def _train_epoch(self, scheduler, epoch: int, eval_step: int,
+ train_data_iterator: ShardedDataIterator, ):
+ logger.info('Best validation checkpoint %s: %f', self.best_cp_name, self.best_validation_result if self.best_validation_result else 0)
+
+ args = self.args
+ rolling_train_loss = 0.0
+ epoch_loss = 0
+ epoch_correct_predictions = 0
+
+ log_result_step = args.log_batch_step
+ rolling_loss_step = args.train_rolling_loss_step
+ num_hard_negatives = args.hard_negatives
+ num_other_negatives = args.other_negatives
+ seed = args.seed
+ self.biencoder.train()
+ epoch_batches = train_data_iterator.max_iterations
+ data_iteration = 0
+ start_time = time.time()
+ for i, samples_batch in enumerate(train_data_iterator.iterate_data(epoch=epoch)):
+ # to be able to resume shuffled ctx- pools
+ data_iteration = train_data_iterator.get_iteration()
+ random.seed(seed + epoch + data_iteration)
+ biencoder_batch = BiEncoder.create_biencoder_input(samples_batch, self.tensorizer, self.args.ctx_field,
+ True,
+ num_hard_negatives, num_other_negatives, shuffle=True,
+ shuffle_positives=args.shuffle_positive_ctx
+ )
+
+ loss, correct_cnt = _do_biencoder_fwd_pass(self.biencoder, biencoder_batch, self.tensorizer, args)
+
+ epoch_correct_predictions += correct_cnt
+ epoch_loss += loss.item()
+ rolling_train_loss += loss.item()
+
+ if args.fp16:
+ from apex import amp
+ with amp.scale_loss(loss, self.optimizer) as scaled_loss:
+ scaled_loss.backward()
+ if args.max_grad_norm > 0:
+ torch.nn.utils.clip_grad_norm_(amp.master_params(self.optimizer), args.max_grad_norm)
+ else:
+ loss.backward()
+ if args.max_grad_norm > 0:
+ torch.nn.utils.clip_grad_norm_(self.biencoder.parameters(), args.max_grad_norm)
+
+ if (i + 1) % args.gradient_accumulation_steps == 0:
+ self.optimizer.step()
+ scheduler.step()
+ self.biencoder.zero_grad()
+
+ if i % log_result_step == 0:
+ lr = self.optimizer.param_groups[0]['lr']
+ logger.info(
+ 'Epoch: %d: Step: %d/%d, loss=%f, lr=%f, used_time=%f sec.', epoch, data_iteration, epoch_batches, loss.item(), lr, time.time() - start_time)
+
+ if (i + 1) % rolling_loss_step == 0:
+ logger.info('Train batch %d', data_iteration)
+ latest_rolling_train_av_loss = rolling_train_loss / rolling_loss_step
+ logger.info('Avg. loss per last %d batches: %f', rolling_loss_step, latest_rolling_train_av_loss)
+ rolling_train_loss = 0.0
+
+ # if data_iteration % eval_step == 0:
+ # logger.info('Validation: Epoch: %d Step: %d/%d', epoch, data_iteration, epoch_batches)
+ # self.validate_and_save(epoch, train_data_iterator.get_iteration(), scheduler)
+ # self.biencoder.train()
+
+
+ self.validate_and_save(epoch, data_iteration, scheduler)
+
+ epoch_loss = (epoch_loss / epoch_batches) if epoch_batches > 0 else 0
+ logger.info('Av Loss per epoch=%f', epoch_loss)
+ logger.info('epoch total correct predictions=%d', epoch_correct_predictions)
+
+ def _save_checkpoint(self, scheduler, epoch: int, offset: int) -> str:
+ args = self.args
+ model_to_save = get_model_obj(self.biencoder)
+ cp = os.path.join(args.output_dir,
+ args.checkpoint_file_name + '.' + str(epoch) + ('.' + str(offset) if offset > 0 else ''))
+
+ meta_params = get_encoder_params_state(args)
+
+ if self.prefix or self.prompt:
+ model_dict = {key: value for (key, value) in model_to_save.state_dict().items() if "prefix_encoder" in key}
+ elif self.adapter:
+ model_to_save.question_model.save_adapter(cp+".q", "adapter")
+ model_to_save.ctx_model.save_adapter(cp+".ctx", "adapter")
+ return cp
+ else:
+ model_dict = model_to_save.state_dict()
+
+ state = CheckpointState(model_dict,
+ self.optimizer.state_dict(),
+ scheduler.state_dict(),
+ offset,
+ epoch, meta_params
+ )
+
+ torch.save(state._asdict(), cp)
+ logger.info('Saved checkpoint at %s', cp)
+ return cp
+
+ def _load_saved_state(self, saved_state: CheckpointState):
+ epoch = saved_state.epoch
+ offset = saved_state.offset
+ if offset == 0: # epoch has been completed
+ epoch += 1
+ logger.info('Loading checkpoint @ batch=%s and epoch=%s', offset, epoch)
+
+ self.start_epoch = epoch
+ self.start_batch = offset
+
+ model_to_load = get_model_obj(self.biencoder)
+ logger.info('Loading saved model state ...')
+ if self.adapter:
+ adapter_name = model_to_load.question_model.load_adapter(self.args.model_file+".q")
+ model_to_load.question_model.set_active_adapters(adapter_name)
+ return
+ else:
+ model_to_load.load_state_dict(saved_state.model_dict, strict=not self.prefix and not self.prompt)
+
+ if saved_state.optimizer_dict:
+ logger.info('Loading saved optimizer state ...')
+ self.optimizer.load_state_dict(saved_state.optimizer_dict)
+
+ if saved_state.scheduler_dict:
+ self.scheduler_state = saved_state.scheduler_dict
+
+ def _load_pretrained_state(self, saved_state: CheckpointState):
+
+ model_to_load = get_model_obj(self.biencoder)
+ logger.info('Loading saved model state ...')
+ if self.prefix:
+ model_to_load.load_state_dict(saved_state.model_dict, strict=False)
+ else:
+ model_to_load.load_state_dict(saved_state.model_dict) # set strict=False if you use extra projection
+
+
+
+def _calc_loss(args, loss_function, local_q_vector, local_ctx_vectors, local_positive_idxs,
+ local_hard_negatives_idxs: list = None,
+ ) -> Tuple[T, bool]:
+ """
+ Calculates In-batch negatives schema loss and supports to run it in DDP mode by exchanging the representations
+ across all the nodes.
+ """
+ distributed_world_size = args.distributed_world_size or 1
+ if distributed_world_size > 1:
+ q_vector_to_send = torch.empty_like(local_q_vector).cpu().copy_(local_q_vector).detach_()
+ ctx_vector_to_send = torch.empty_like(local_ctx_vectors).cpu().copy_(local_ctx_vectors).detach_()
+
+ global_question_ctx_vectors = all_gather_list(
+ [q_vector_to_send, ctx_vector_to_send, local_positive_idxs, local_hard_negatives_idxs],
+ max_size=args.global_loss_buf_sz)
+
+ global_q_vector = []
+ global_ctxs_vector = []
+
+ # ctxs_per_question = local_ctx_vectors.size(0)
+ positive_idx_per_question = []
+ hard_negatives_per_question = []
+
+ total_ctxs = 0
+
+ for i, item in enumerate(global_question_ctx_vectors):
+ q_vector, ctx_vectors, positive_idx, hard_negatives_idxs = item
+
+ if i != args.local_rank:
+ global_q_vector.append(q_vector.to(local_q_vector.device))
+ global_ctxs_vector.append(ctx_vectors.to(local_q_vector.device))
+ positive_idx_per_question.extend([v + total_ctxs for v in positive_idx])
+ hard_negatives_per_question.extend([[v + total_ctxs for v in l] for l in hard_negatives_idxs])
+ else:
+ global_q_vector.append(local_q_vector)
+ global_ctxs_vector.append(local_ctx_vectors)
+ positive_idx_per_question.extend([v + total_ctxs for v in local_positive_idxs])
+ hard_negatives_per_question.extend([[v + total_ctxs for v in l] for l in local_hard_negatives_idxs])
+ total_ctxs += ctx_vectors.size(0)
+
+ global_q_vector = torch.cat(global_q_vector, dim=0)
+ global_ctxs_vector = torch.cat(global_ctxs_vector, dim=0)
+
+ else:
+ global_q_vector = local_q_vector
+ global_ctxs_vector = local_ctx_vectors
+ positive_idx_per_question = local_positive_idxs
+ hard_negatives_per_question = local_hard_negatives_idxs
+
+ loss, is_correct = loss_function.calc(global_q_vector, global_ctxs_vector, positive_idx_per_question,
+ hard_negatives_per_question)
+
+ return loss, is_correct
+
+
+def _do_biencoder_fwd_pass(model: nn.Module, input: BiEncoderBatch, tensorizer: Tensorizer, args) -> Tuple[
+ torch.Tensor, int]:
+ input = BiEncoderBatch(**move_to_device(input._asdict(), args.device))
+
+ q_attn_mask = tensorizer.get_attn_mask(input.question_ids)
+ ctx_attn_mask = tensorizer.get_attn_mask(input.context_ids)
+
+ if model.training:
+ model_out = model(input.question_ids, input.question_segments, q_attn_mask, input.context_ids,
+ input.ctx_segments, ctx_attn_mask)
+ else:
+ with torch.no_grad():
+ model_out = model(input.question_ids, input.question_segments, q_attn_mask, input.context_ids,
+ input.ctx_segments, ctx_attn_mask)
+
+ local_q_vector, local_ctx_vectors = model_out
+
+ loss_function = BiEncoderNllLoss()
+
+ loss, is_correct = _calc_loss(args, loss_function, local_q_vector, local_ctx_vectors, input.is_positive,
+ input.hard_negatives)
+
+ is_correct = is_correct.sum().item()
+
+ if args.n_gpu > 1:
+ loss = loss.mean()
+ if args.gradient_accumulation_steps > 1:
+ loss = loss / args.gradient_accumulation_steps
+
+ return loss, is_correct
+
+
+def main():
+ parser = argparse.ArgumentParser()
+
+ add_encoder_params(parser)
+ add_training_params(parser)
+ add_tokenizer_params(parser)
+ add_tuning_params(parser)
+
+ # biencoder specific training features
+ parser.add_argument("--eval_per_epoch", default=1, type=int,
+ help="How many times it evaluates on dev set per epoch and saves a checkpoint")
+
+ parser.add_argument("--global_loss_buf_sz", type=int, default=150000,
+ help='Buffer size for distributed mode representations al gather operation. \
+ Increase this if you see errors like "encoded data exceeds max_size ..."')
+
+ parser.add_argument("--fix_ctx_encoder", action='store_true')
+ parser.add_argument("--shuffle_positive_ctx", action='store_true')
+
+ # input/output src params
+ parser.add_argument("--output_dir", default=None, type=str,
+ help="The output directory where the model checkpoints will be written or resumed from")
+
+ # data handling parameters
+ parser.add_argument("--hard_negatives", default=1, type=int,
+ help="amount of hard negative ctx per question")
+ parser.add_argument("--other_negatives", default=0, type=int,
+ help="amount of 'other' negative ctx per question")
+ parser.add_argument("--train_files_upsample_rates", type=str,
+ help="list of up-sample rates per each train file. Example: [1,2,1]")
+
+ # parameters for Av.rank validation method
+ parser.add_argument("--val_av_rank_start_epoch", type=int, default=10000,
+ help="Av.rank validation: the epoch from which to enable this validation")
+ parser.add_argument("--val_av_rank_hard_neg", type=int, default=30,
+ help="Av.rank validation: how many hard negatives to take from each question pool")
+ parser.add_argument("--val_av_rank_other_neg", type=int, default=30,
+ help="Av.rank validation: how many 'other' negatives to take from each question pool")
+ parser.add_argument("--val_av_rank_bsz", type=int, default=128,
+ help="Av.rank validation: batch size to process passages")
+ parser.add_argument("--val_av_rank_max_qs", type=int, default=10000,
+ help="Av.rank validation: max num of questions")
+ parser.add_argument('--checkpoint_file_name', type=str, default='dpr_biencoder', help="Checkpoints file prefix")
+
+ parser.add_argument("--warmup_percentage", default=None, type=float)
+ args = parser.parse_args()
+
+ if args.gradient_accumulation_steps < 1:
+ raise ValueError("Invalid gradient_accumulation_steps parameter: {}, should be >= 1".format(
+ args.gradient_accumulation_steps))
+
+ if args.output_dir is not None:
+ os.makedirs(args.output_dir, exist_ok=True)
+
+ setup_args_gpu(args)
+ set_seed(args)
+ print_args(args)
+
+ trainer = BiEncoderTrainer(args)
+
+ if args.train_file is not None:
+ trainer.run_train()
+ elif args.model_file and args.dev_file:
+ logger.info("No train files are specified. Run 2 types of validation for specified model file")
+ trainer.validate_nll()
+ trainer.validate_average_rank()
+ else:
+ logger.warning("Neither train_file or (model_file & dev_file) parameters are specified. Nothing to do.")
+
+
+if __name__ == "__main__":
+ main()
diff --git a/README.md b/README.md
index 5777175..81b4cf2 100644
--- a/README.md
+++ b/README.md
@@ -1,2 +1,132 @@
-# UPET
-The repository for paper "Uncertainty-aware Parameter-Efficient Self-training for Semi-supervised Language Understanding"
+# UPET: Uncertainty-aware Parameter-Efficient Tuning for Semi-supervised Language Understanding
+
+
+Head Tuning: Training the model with CLS head, whith or whitout prefix / adapter
+Prompt Tuning: Training the model with prompt and verbalizer (MLM head), whith or whitout prefix / adapter
+
+augment definition:
+e.g.,
+--prefix -> --head-prefix or --prompt-prefix
+--prompt -> --head-ptuning or --prompt-ptuning
+
+### Setup
+We conduct our experiment with Anaconda3. If you have installed Anaconda3, then create the environment for P-tuning v2:
+
+```shell
+conda create -n pt2 python=3.8.5
+conda activate pt2
+```
+
+After we setup basic conda environment, install pytorch related packages via:
+
+```shell
+conda install -n pt2 pytorch==1.7.1 torchvision==0.8.2 torchaudio==0.7.2 cudatoolkit=11.0 -c pytorch
+```
+
+Finally, install other python packages we need:
+
+```shell
+pip install -r requirements.txt
+```
+
+### Data
+For SuperGLUE and SQuAD datasets, we download them from the Huggingface Datasets APIs (embedded in our codes).
+
+For sequence tagging (NER, SRL) datasets, we prepare a non-official packup [here](https://zenodo.org/record/6318701/files/P-tuning-v2_data.tar.gz?download=1).
+After downloading, unzip the packup to the project root.
+Please use at your own risk.
+
+### Training
+Run training scripts in [run_script](run_script) (e.g., RoBERTa for RTE):
+
+You can change the augments and run:
+```shell
+bash run_script/run_rte_roberta.sh
+```
+or
+
+```shell
+export TASK_NAME=superglue
+export DATASET_NAME=rte
+export CUDA_VISIBLE_DEVICES=0
+bs=32
+lr=5e-3
+dropout=0.1
+psl=128
+epoch=100
+python3 run.py \
+ --model_name_or_path /wjn/pre-trained-lm/roberta-large \
+ --task_name $TASK_NAME \
+ --dataset_name $DATASET_NAME \
+ --do_train \
+ --do_eval \
+ --max_seq_length 128 \
+ --per_device_train_batch_size $bs \
+ --learning_rate $lr \
+ --num_train_epochs $epoch \
+ --output_dir checkpoints/$DATASET_NAME-roberta/ \
+ --overwrite_output_dir \
+ --hidden_dropout_prob $dropout \
+ --seed 11 \
+ --save_strategy no \
+ --evaluation_strategy epoch \
+ --prefix
+```
+This script is run for Full-data Full-supervised Pre-fix Tuning.
+
+We provide the following kinds of settings:
+- Full-data v.s. Few-shot: The training data is full / few-shot
+- Full-supervised v.s. Semi-supervised: We use full-supervised / self-training
+- Full-Tuning v.s. Patameter-efficient Tuning: Only tuning the full parameters / Tuning the few parameters
+- One-stage v.s. Two-stage: directly tuning / tuning the few paraemters and then tuning the full
+- Head-Tuning v.s. Prompt-Tuning: Prefix/Adapter + CLS head / Prefix/Adapter + Prompt + Vaberlizer
+
+The specific augments for different scenarios:
+
+
+#### Few-shot Head Tuning
+
+### Implemented Results
+Currently we have released our reimplementation on following tasks and datasets. More implementation will be released soon.
+
+Released results on BERT-large
+
+| | BoolQ | COPA | RTE | WiC | WSC | CoNLL04 | OntoNotes 5.0 | CoNLL12 |
+|--------------|-------|------|------|------|------|---------|---------------|---------|
+| Result | 74.3 | 77.0 | 80.1 | 75.1 | 68.3 | 84.5 | 86.4 | 85.3 |
+| Total Epochs | 100 | 80 | 60 | 80 | 80 | 40 | 30 | 45 |
+| Best Epoch | 58 | 12 | 30 | 56 | 17 | 33 | 24 | 43 |
+
+Released results on RoBERTa-large
+
+| | BoolQ | COPA | RTE | WiC | WSC | CoNLL03 | CoNLL04 | OntoNotes 5.0 | CoNLL12 | CoNLL05 WSJ | CoNLL05 Brown | SQuAD 1.1 | SQuAD 2.0 |
+|--------------|-------|------|------|------|------|---------|---------|---------------|---------|-------------|---------------|-----------|-----------|
+| Results | 84.0 | 92.0 | 86.6 | 73.7 | 64.4 | 91.8 | 88.4 | 90.1 | 84.7 | 89.4 | 83.9 | 88.1/94.2 | 81.3/84.7 |
+| Total Epochs | 100 | 120 | 100 | 50 | 10 | 30 | 80 | 60 | 45 | 15 | - | 30 | 10 |
+| Best Epoch | 86 | 78 | 65 | 31 | 3 | 28 | 45 | 59 | 37 | 13 | - | 24 | 9 |
+
+For other hyper-parameters, please refer to the training scripts.
+If you can not achieve the reported results at the best epoch, there is probably an environmental mismatch and hyper-parameter search is needed.
+
+## Citation
+
+If you find our work useful, please kindly cite our paper:
+
+
+
+
+
+
+---
+
+use_prompt
+sst-2,mr,cr,mnli,snli,qnli,rte,mrpc,qqp,cola,trec
+
+
+
+superglue
+BoolQ CB COPA MultiRC ReCoRD RTE WiC WSC AX-b AX-g
+glue
+CoLA SST-2 MRPC STS-B QQP MNLI-m MNLI-mm QNLI RTE WNLI AX
+
+
diff --git a/arguments.py b/arguments.py
new file mode 100644
index 0000000..21dbe64
--- /dev/null
+++ b/arguments.py
@@ -0,0 +1,328 @@
+from enum import Enum
+import argparse
+import dataclasses
+from dataclasses import dataclass, field
+from typing import Optional
+
+from transformers import HfArgumentParser, TrainingArguments
+
+from tasks.utils import *
+
+
+@dataclass
+class DataTrainingArguments:
+ """
+ Arguments pertaining to what data we are going to input our model for training and eval.
+
+ Using `HfArgumentParser` we can turn this class
+ into argparse arguments to be able to specify them on
+ the command line.training_args
+ """
+ task_name: str = field(
+ metadata={
+ "help": "The name of the task to train on: " + ", ".join(TASKS),
+ "choices": TASKS
+ },
+ )
+ dataset_name: str = field(
+ metadata={
+ "help": "The name of the dataset to use: " + ", ".join(DATASETS),
+ "choices": DATASETS
+ }
+ )
+ # add by wjn
+ num_examples_per_label: Optional[int] = field(
+ default=None,
+ metadata={
+ "help": "Randomly sampling k-shot examples for each label "
+ },
+ )
+ dataset_config_name: Optional[str] = field(
+ default=None, metadata={"help": "The configuration name of the dataset to use (via the datasets library)."}
+ )
+ max_seq_length: int = field(
+ default=128,
+ metadata={
+ "help": "The maximum total input sequence length after tokenization. Sequences longer "
+ "than this will be truncated, sequences shorter will be padded."
+ },
+ )
+ overwrite_cache: bool = field(
+ default=False, metadata={"help": "Overwrite the cached preprocessed datasets or not."}
+ )
+ pad_to_max_length: bool = field(
+ default=True,
+ metadata={
+ "help": "Whether to pad all samples to `max_seq_length`. "
+ "If False, will pad the samples dynamically when batching to the maximum length in the batch."
+ },
+ )
+ max_train_samples: Optional[int] = field(
+ default=None,
+ metadata={
+ "help": "For debugging purposes or quicker training, truncate the number of training examples to this "
+ "value if set."
+ },
+ )
+ max_eval_samples: Optional[int] = field(
+ default=None,
+ metadata={
+ "help": "For debugging purposes or quicker training, truncate the number of evaluation examples to this "
+ "value if set."
+ },
+ )
+ max_predict_samples: Optional[int] = field(
+ default=None,
+ metadata={
+ "help": "For debugging purposes or quicker training, truncate the number of prediction examples to this "
+ "value if set."
+ },
+ )
+ train_file: Optional[str] = field(
+ default=None, metadata={"help": "A csv or a json file containing the training data."}
+ )
+ validation_file: Optional[str] = field(
+ default=None, metadata={"help": "A csv or a json file containing the validation data."}
+ )
+ test_file: Optional[str] = field(
+ default=None,
+ metadata={"help": "A csv or a json file containing the test data."}
+ )
+ template_id: Optional[int] = field(
+ default=0,
+ metadata={
+ "help": "The specific prompt string to use"
+ }
+ )
+
+
+@dataclass
+class ModelArguments:
+ """
+ Arguments pertaining to which model/config/tokenizer we are going to fine-tune from.
+ """
+ model_name_or_path: str = field(
+ metadata={"help": "Path to pretrained model or model identifier from huggingface.co/models"}
+ )
+ config_name: Optional[str] = field(
+ default=None, metadata={"help": "Pretrained config name or path if not the same as model_name"}
+ )
+ tokenizer_name: Optional[str] = field(
+ default=None, metadata={"help": "Pretrained tokenizer name or path if not the same as model_name"}
+ )
+ cache_dir: Optional[str] = field(
+ default=None,
+ metadata={"help": "Where do you want to store the pretrained models downloaded from huggingface.co"},
+ )
+ use_fast_tokenizer: bool = field(
+ default=True,
+ metadata={"help": "Whether to use one of the fast tokenizer (backed by the tokenizers library) or not."},
+ )
+ model_revision: str = field(
+ default="main",
+ metadata={"help": "The specific model version to use (can be a branch name, tag name or commit id)."},
+ )
+ use_auth_token: bool = field(
+ default=False,
+ metadata={
+ "help": "Will use the token generated when running `transformers-cli login` (necessary to use this script "
+ "with private models)."
+ },
+ )
+ use_pe: bool = field(
+ default=False,
+ metadata={
+ "help": "Whether to use parameter-efficient settings. If true, that means fix the parameters of the backbone, and only"
+ "tune the new initialized modules (e.g., adapter, prefix, ptuning, etc.)"
+ }
+ )
+ head_prefix: bool = field(
+ default=False,
+ metadata={
+ "help": "Will use Head-tuning Prefix (P-tuning v2) during training"
+ }
+ )
+ prompt_prefix: bool = field(
+ default=False,
+ metadata={
+ "help": "Will use Prompt-tuning Prefix (P-tuning v2) during training"
+ }
+ )
+ head_only: bool = field(
+ default=False,
+ metadata={
+ "help": "Will use Head Fine-tuning during training (w/o. any new modules)"
+ }
+ )
+ prompt_only: bool = field(
+ default=False,
+ metadata={
+ "help": "Will use Prompt Fine-tuning during training (w/o. any new modules)"
+ }
+ )
+ head_ptuning: bool = field(
+ default=False,
+ metadata={
+ "help": "Will use Head-tuning P-tuning during training (w. parameter-efficient)"
+ }
+ )
+ prompt_ptuning: bool = field(
+ default=False,
+ metadata={
+ "help": "Will use Prompt-tuning P-tuning during training (w. parameter-efficient)"
+ }
+ )
+ head_adapter: bool = field(
+ default=False,
+ metadata={
+ "help": "Will use Head-tuning Adapter tuning during training (w. parameter-efficient)"
+ }
+ )
+ prompt_adapter: bool = field(
+ default=False,
+ metadata={
+ "help": "Will use Prompt-tuning Adapter tuning during training (w. parameter-efficient)"
+ }
+ )
+ adapter_choice: str = field(
+ default="LiST",
+ metadata={"help": "The choice of adapter, list, lora, houlsby."},
+ )
+ adapter_dim: int = field(
+ default=128,
+ metadata={"help": "The hidden size of adapter. default is 128."},
+ )
+ pre_seq_len: int = field(
+ default=4,
+ metadata={
+ "help": "The length of prompt"
+ }
+ )
+ prefix_projection: bool = field(
+ default=False,
+ metadata={
+ "help": "Apply a two-layer MLP head over the prefix embeddings"
+ }
+ )
+ prefix_hidden_size: int = field(
+ default=512,
+ metadata={
+ "help": "The hidden size of the MLP projection head in Prefix Encoder if prefix projection is used"
+ }
+ )
+ hidden_dropout_prob: float = field(
+ default=0.1,
+ metadata={
+ "help": "The dropout probability used in the models"
+ }
+ )
+
+
+@dataclass
+class SemiSupervisedArguments:
+ use_semi: bool = field(
+ default=False, metadata={"help": "If true, the training process will be transformed into self-training framework."}
+ )
+ unlabeled_data_num: int = field(
+ default=-1,
+ metadata={
+ "help": "The total number of unlabeled data. If set -1 means all the training data (expect of few-shot labeled data)"
+ }
+ )
+ unlabeled_data_batch_size: int = field(
+ default=16,
+ metadata={
+ "help": "The number of unlabeled data in one batch."
+ }
+ )
+ pseudo_sample_num_or_ratio: float = field(
+ default=0.1,
+ metadata={
+ "help": "The number / ratio of pseudo-labeled data sampling. For example, if have 1000 unlabeled data, 0.1 / 100 means sampling 100 pseduo-labeled data."
+ }
+ )
+ teacher_training_epoch: int = field(
+ default=10,
+ metadata={
+ "help": "The epoch number of teacher training at the beginning of self-training."
+ }
+ )
+ teacher_tuning_epoch: int = field(
+ default=10,
+ metadata={
+ "help": "The epoch number of teacher tuning in each self-training iteration."
+ }
+ )
+ student_training_epoch: int = field(
+ default=16,
+ metadata={
+ "help": "The epoch number of student training in each self-training iteration."
+ }
+ )
+ student_learning_rate: float = field(
+ default=1e-5,
+ metadata={
+ "help": "The learning rate of student training in each self-training iteration."
+ }
+ )
+ self_training_epoch: int = field(
+ default=30,
+ metadata={
+ "help": "The number of teacher-student iteration ."
+ }
+ )
+ # self-training过程中,unlabeled data会先采样一个子集,因此student模型学习的样本数量只有不到1024个
+ # post_student_train设置后,即允许在self-training后,选择最佳的teacher模型,并在全部的unlabeled data上打标和mc dropout
+ # 此时student模型将会在更多的样本上训练(样本数量不超过20k)
+ post_student_train: bool = field(
+ default=False,
+ metadata={
+ "help": "Whether to train a student model on large pseudo-labeled data after self-training iteration"
+ }
+ )
+ student_pre_seq_len: int = field(
+ default=4,
+ metadata={
+ "help": "The length of prompt"
+ }
+ )
+
+
+
+
+
+@dataclass
+class QuestionAnwseringArguments:
+ n_best_size: int = field(
+ default=20,
+ metadata={"help": "The total number of n-best predictions to generate when looking for an answer."},
+ )
+ max_answer_length: int = field(
+ default=30,
+ metadata={
+ "help": "The maximum length of an answer that can be generated. This is needed because the start "
+ "and end predictions are not conditioned on one another."
+ },
+ )
+ version_2_with_negative: bool = field(
+ default=False, metadata={"help": "If true, some of the examples do not have an answer."}
+ )
+ null_score_diff_threshold: float = field(
+ default=0.0,
+ metadata={
+ "help": "The threshold used to select the null answer: if the best answer has a score that is less than "
+ "the score of the null answer minus this threshold, the null answer is selected for this example. "
+ "Only useful when `version_2_with_negative=True`."
+ },
+ )
+
+
+
+
+def get_args():
+ """Parse all the args."""
+ parser = HfArgumentParser((ModelArguments, DataTrainingArguments, TrainingArguments, SemiSupervisedArguments, QuestionAnwseringArguments))
+
+ args = parser.parse_args_into_dataclasses()
+
+ return args
\ No newline at end of file
diff --git a/metrics/accuracy/README.md b/metrics/accuracy/README.md
new file mode 100644
index 0000000..3c4805d
--- /dev/null
+++ b/metrics/accuracy/README.md
@@ -0,0 +1,97 @@
+# Metric Card for Accuracy
+
+
+## Metric Description
+
+Accuracy is the proportion of correct predictions among the total number of cases processed. It can be computed with:
+Accuracy = (TP + TN) / (TP + TN + FP + FN)
+ Where:
+TP: True positive
+TN: True negative
+FP: False positive
+FN: False negative
+
+
+## How to Use
+
+At minimum, this metric requires predictions and references as inputs.
+
+```python
+>>> accuracy_metric = datasets.load_metric("accuracy")
+>>> results = accuracy_metric.compute(references=[0, 1], predictions=[0, 1])
+>>> print(results)
+{'accuracy': 1.0}
+```
+
+
+### Inputs
+- **predictions** (`list` of `int`): Predicted labels.
+- **references** (`list` of `int`): Ground truth labels.
+- **normalize** (`boolean`): If set to False, returns the number of correctly classified samples. Otherwise, returns the fraction of correctly classified samples. Defaults to True.
+- **sample_weight** (`list` of `float`): Sample weights Defaults to None.
+
+
+### Output Values
+- **accuracy**(`float` or `int`): Accuracy score. Minimum possible value is 0. Maximum possible value is 1.0, or the number of examples input, if `normalize` is set to `True`.. A higher score means higher accuracy.
+
+Output Example(s):
+```python
+{'accuracy': 1.0}
+```
+
+This metric outputs a dictionary, containing the accuracy score.
+
+
+#### Values from Popular Papers
+
+Top-1 or top-5 accuracy is often used to report performance on supervised classification tasks such as image classification (e.g. on [ImageNet](https://paperswithcode.com/sota/image-classification-on-imagenet)) or sentiment analysis (e.g. on [IMDB](https://paperswithcode.com/sota/text-classification-on-imdb)).
+
+
+### Examples
+
+Example 1-A simple example
+```python
+>>> accuracy_metric = datasets.load_metric("accuracy")
+>>> results = accuracy_metric.compute(references=[0, 1, 2, 0, 1, 2], predictions=[0, 1, 1, 2, 1, 0])
+>>> print(results)
+{'accuracy': 0.5}
+```
+
+Example 2-The same as Example 1, except with `normalize` set to `False`.
+```python
+>>> accuracy_metric = datasets.load_metric("accuracy")
+>>> results = accuracy_metric.compute(references=[0, 1, 2, 0, 1, 2], predictions=[0, 1, 1, 2, 1, 0], normalize=False)
+>>> print(results)
+{'accuracy': 3.0}
+```
+
+Example 3-The same as Example 1, except with `sample_weight` set.
+```python
+>>> accuracy_metric = datasets.load_metric("accuracy")
+>>> results = accuracy_metric.compute(references=[0, 1, 2, 0, 1, 2], predictions=[0, 1, 1, 2, 1, 0], sample_weight=[0.5, 2, 0.7, 0.5, 9, 0.4])
+>>> print(results)
+{'accuracy': 0.8778625954198473}
+```
+
+
+## Limitations and Bias
+This metric can be easily misleading, especially in the case of unbalanced classes. For example, a high accuracy might be because a model is doing well, but if the data is unbalanced, it might also be because the model is only accurately labeling the high-frequency class. In such cases, a more detailed analysis of the model's behavior, or the use of a different metric entirely, is necessary to determine how well the model is actually performing.
+
+
+## Citation(s)
+```bibtex
+@article{scikit-learn,
+ title={Scikit-learn: Machine Learning in {P}ython},
+ author={Pedregosa, F. and Varoquaux, G. and Gramfort, A. and Michel, V.
+ and Thirion, B. and Grisel, O. and Blondel, M. and Prettenhofer, P.
+ and Weiss, R. and Dubourg, V. and Vanderplas, J. and Passos, A. and
+ Cournapeau, D. and Brucher, M. and Perrot, M. and Duchesnay, E.},
+ journal={Journal of Machine Learning Research},
+ volume={12},
+ pages={2825--2830},
+ year={2011}
+}
+```
+
+
+## Further References
diff --git a/metrics/accuracy/accuracy.py b/metrics/accuracy/accuracy.py
new file mode 100644
index 0000000..b9b0e33
--- /dev/null
+++ b/metrics/accuracy/accuracy.py
@@ -0,0 +1,105 @@
+# Copyright 2020 The HuggingFace Datasets Authors and the current dataset script contributor.
+#
+# Licensed under the Apache License, Version 2.0 (the "License");
+# you may not use this file except in compliance with the License.
+# You may obtain a copy of the License at
+#
+# http://www.apache.org/licenses/LICENSE-2.0
+#
+# Unless required by applicable law or agreed to in writing, software
+# distributed under the License is distributed on an "AS IS" BASIS,
+# WITHOUT WARRANTIES OR CONDITIONS OF ANY KIND, either express or implied.
+# See the License for the specific language governing permissions and
+# limitations under the License.
+"""Accuracy metric."""
+
+from sklearn.metrics import accuracy_score
+
+import datasets
+
+
+_DESCRIPTION = """
+Accuracy is the proportion of correct predictions among the total number of cases processed. It can be computed with:
+Accuracy = (TP + TN) / (TP + TN + FP + FN)
+ Where:
+TP: True positive
+TN: True negative
+FP: False positive
+FN: False negative
+"""
+
+
+_KWARGS_DESCRIPTION = """
+Args:
+ predictions (`list` of `int`): Predicted labels.
+ references (`list` of `int`): Ground truth labels.
+ normalize (`boolean`): If set to False, returns the number of correctly classified samples. Otherwise, returns the fraction of correctly classified samples. Defaults to True.
+ sample_weight (`list` of `float`): Sample weights Defaults to None.
+
+Returns:
+ accuracy (`float` or `int`): Accuracy score. Minimum possible value is 0. Maximum possible value is 1.0, or the number of examples input, if `normalize` is set to `True`.. A higher score means higher accuracy.
+
+Examples:
+
+ Example 1-A simple example
+ >>> accuracy_metric = datasets.load_metric("accuracy")
+ >>> results = accuracy_metric.compute(references=[0, 1, 2, 0, 1, 2], predictions=[0, 1, 1, 2, 1, 0])
+ >>> print(results)
+ {'accuracy': 0.5}
+
+ Example 2-The same as Example 1, except with `normalize` set to `False`.
+ >>> accuracy_metric = datasets.load_metric("accuracy")
+ >>> results = accuracy_metric.compute(references=[0, 1, 2, 0, 1, 2], predictions=[0, 1, 1, 2, 1, 0], normalize=False)
+ >>> print(results)
+ {'accuracy': 3.0}
+
+ Example 3-The same as Example 1, except with `sample_weight` set.
+ >>> accuracy_metric = datasets.load_metric("accuracy")
+ >>> results = accuracy_metric.compute(references=[0, 1, 2, 0, 1, 2], predictions=[0, 1, 1, 2, 1, 0], sample_weight=[0.5, 2, 0.7, 0.5, 9, 0.4])
+ >>> print(results)
+ {'accuracy': 0.8778625954198473}
+"""
+
+
+_CITATION = """
+@article{scikit-learn,
+ title={Scikit-learn: Machine Learning in {P}ython},
+ author={Pedregosa, F. and Varoquaux, G. and Gramfort, A. and Michel, V.
+ and Thirion, B. and Grisel, O. and Blondel, M. and Prettenhofer, P.
+ and Weiss, R. and Dubourg, V. and Vanderplas, J. and Passos, A. and
+ Cournapeau, D. and Brucher, M. and Perrot, M. and Duchesnay, E.},
+ journal={Journal of Machine Learning Research},
+ volume={12},
+ pages={2825--2830},
+ year={2011}
+}
+"""
+
+
+@datasets.utils.file_utils.add_start_docstrings(_DESCRIPTION, _KWARGS_DESCRIPTION)
+class Accuracy(datasets.Metric):
+ def _info(self):
+ return datasets.MetricInfo(
+ description=_DESCRIPTION,
+ citation=_CITATION,
+ inputs_description=_KWARGS_DESCRIPTION,
+ features=datasets.Features(
+ {
+ "predictions": datasets.Sequence(datasets.Value("int32")),
+ "references": datasets.Sequence(datasets.Value("int32")),
+ }
+ if self.config_name == "multilabel"
+ else {
+ "predictions": datasets.Value("int32"),
+ "references": datasets.Value("int32"),
+ }
+ ),
+ reference_urls=["https://scikit-learn.org/stable/modules/generated/sklearn.metrics.accuracy_score.html"],
+ )
+
+ def _compute(self, predictions, references, normalize=True, sample_weight=None):
+ return {
+ "accuracy": float(
+ accuracy_score(references, predictions, normalize=normalize, sample_weight=sample_weight)
+ )
+ }
diff --git a/metrics/bertscore/README.md b/metrics/bertscore/README.md
new file mode 100644
index 0000000..cf43849
--- /dev/null
+++ b/metrics/bertscore/README.md
@@ -0,0 +1,111 @@
+# Metric Card for BERT Score
+
+## Metric description
+
+BERTScore is an automatic evaluation metric for text generation that computes a similarity score for each token in the candidate sentence with each token in the reference sentence. It leverages the pre-trained contextual embeddings from [BERT](https://huggingface.co/bert-base-uncased) models and matches words in candidate and reference sentences by cosine similarity.
+
+Moreover, BERTScore computes precision, recall, and F1 measure, which can be useful for evaluating different language generation tasks.
+
+## How to use
+
+BERTScore takes 3 mandatory arguments : `predictions` (a list of string of candidate sentences), `references` (a list of strings or list of list of strings of reference sentences) and either `lang` (a string of two letters indicating the language of the sentences, in [ISO 639-1 format](https://en.wikipedia.org/wiki/List_of_ISO_639-1_codes)) or `model_type` (a string specififying which model to use, according to the BERT specification). The default behavior of the metric is to use the suggested model for the target language when one is specified, otherwise to use the `model_type` indicated.
+
+```python
+from datasets import load_metric
+bertscore = load_metric("bertscore")
+predictions = ["hello there", "general kenobi"]
+references = ["hello there", "general kenobi"]
+results = bertscore.compute(predictions=predictions, references=references, lang="en")
+```
+
+BERTScore also accepts multiple optional arguments:
+
+
+`num_layers` (int): The layer of representation to use. The default is the number of layers tuned on WMT16 correlation data, which depends on the `model_type` used.
+
+`verbose` (bool): Turn on intermediate status update. The default value is `False`.
+
+`idf` (bool or dict): Use idf weighting; can also be a precomputed idf_dict.
+
+`device` (str): On which the contextual embedding model will be allocated on. If this argument is `None`, the model lives on `cuda:0` if cuda is available.
+
+`nthreads` (int): Number of threads used for computation. The default value is `4`.
+
+`rescale_with_baseline` (bool): Rescale BERTScore with the pre-computed baseline. The default value is `False`.
+
+`batch_size` (int): BERTScore processing batch size, at least one of `model_type` or `lang`. `lang` needs to be specified when `rescale_with_baseline` is `True`.
+
+`baseline_path` (str): Customized baseline file.
+
+`use_fast_tokenizer` (bool): `use_fast` parameter passed to HF tokenizer. The default value is `False`.
+
+
+## Output values
+
+BERTScore outputs a dictionary with the following values:
+
+`precision`: The [precision](https://huggingface.co/metrics/precision) for each sentence from the `predictions` + `references` lists, which ranges from 0.0 to 1.0.
+
+`recall`: The [recall](https://huggingface.co/metrics/recall) for each sentence from the `predictions` + `references` lists, which ranges from 0.0 to 1.0.
+
+`f1`: The [F1 score](https://huggingface.co/metrics/f1) for each sentence from the `predictions` + `references` lists, which ranges from 0.0 to 1.0.
+
+`hashcode:` The hashcode of the library.
+
+
+### Values from popular papers
+The [original BERTScore paper](https://openreview.net/pdf?id=SkeHuCVFDr) reported average model selection accuracies (Hits@1) on WMT18 hybrid systems for different language pairs, which ranged from 0.004 for `en<->tr` to 0.824 for `en<->de`.
+
+For more recent model performance, see the [metric leaderboard](https://paperswithcode.com/paper/bertscore-evaluating-text-generation-with).
+
+## Examples
+
+Maximal values with the `distilbert-base-uncased` model:
+
+```python
+from datasets import load_metric
+bertscore = load_metric("bertscore")
+predictions = ["hello world", "general kenobi"]
+references = ["hello world", "general kenobi"]
+results = bertscore.compute(predictions=predictions, references=references, model_type="distilbert-base-uncased")
+print(results)
+{'precision': [1.0, 1.0], 'recall': [1.0, 1.0], 'f1': [1.0, 1.0], 'hashcode': 'distilbert-base-uncased_L5_no-idf_version=0.3.10(hug_trans=4.10.3)'}
+```
+
+Partial match with the `bert-base-uncased` model:
+
+```python
+from datasets import load_metric
+bertscore = load_metric("bertscore")
+predictions = ["hello world", "general kenobi"]
+references = ["goodnight moon", "the sun is shining"]
+results = bertscore.compute(predictions=predictions, references=references, model_type="distilbert-base-uncased")
+print(results)
+{'precision': [0.7380737066268921, 0.5584042072296143], 'recall': [0.7380737066268921, 0.5889028906822205], 'f1': [0.7380737066268921, 0.5732481479644775], 'hashcode': 'bert-base-uncased_L5_no-idf_version=0.3.10(hug_trans=4.10.3)'}
+```
+
+## Limitations and bias
+
+The [original BERTScore paper](https://openreview.net/pdf?id=SkeHuCVFDr) showed that BERTScore correlates well with human judgment on sentence-level and system-level evaluation, but this depends on the model and language pair selected.
+
+Furthermore, not all languages are supported by the metric -- see the [BERTScore supported language list](https://github.com/google-research/bert/blob/master/multilingual.md#list-of-languages) for more information.
+
+Finally, calculating the BERTScore metric involves downloading the BERT model that is used to compute the score-- the default model for `en`, `roberta-large`, takes over 1.4GB of storage space and downloading it can take a significant amount of time depending on the speed of your internet connection. If this is an issue, choose a smaller model; for instance `distilbert-base-uncased` is 268MB. A full list of compatible models can be found [here](https://docs.google.com/spreadsheets/d/1RKOVpselB98Nnh_EOC4A2BYn8_201tmPODpNWu4w7xI/edit#gid=0).
+
+
+## Citation
+
+```bibtex
+@inproceedings{bert-score,
+ title={BERTScore: Evaluating Text Generation with BERT},
+ author={Tianyi Zhang* and Varsha Kishore* and Felix Wu* and Kilian Q. Weinberger and Yoav Artzi},
+ booktitle={International Conference on Learning Representations},
+ year={2020},
+ url={https://openreview.net/forum?id=SkeHuCVFDr}
+}
+```
+
+## Further References
+
+- [BERTScore Project README](https://github.com/Tiiiger/bert_score#readme)
+- [BERTScore ICLR 2020 Poster Presentation](https://iclr.cc/virtual_2020/poster_SkeHuCVFDr.html)
diff --git a/metrics/bertscore/bertscore.py b/metrics/bertscore/bertscore.py
new file mode 100644
index 0000000..e6a7afe
--- /dev/null
+++ b/metrics/bertscore/bertscore.py
@@ -0,0 +1,207 @@
+# Copyright 2020 The HuggingFace Datasets Authors.
+#
+# Licensed under the Apache License, Version 2.0 (the "License");
+# you may not use this file except in compliance with the License.
+# You may obtain a copy of the License at
+#
+# http://www.apache.org/licenses/LICENSE-2.0
+#
+# Unless required by applicable law or agreed to in writing, software
+# distributed under the License is distributed on an "AS IS" BASIS,
+# WITHOUT WARRANTIES OR CONDITIONS OF ANY KIND, either express or implied.
+# See the License for the specific language governing permissions and
+# limitations under the License.
+""" BERTScore metric. """
+
+import functools
+from contextlib import contextmanager
+
+import bert_score
+from packaging import version
+
+import datasets
+
+
+@contextmanager
+def filter_logging_context():
+ def filter_log(record):
+ return False if "This IS expected if you are initializing" in record.msg else True
+
+ logger = datasets.utils.logging.get_logger("transformers.modeling_utils")
+ logger.addFilter(filter_log)
+ try:
+ yield
+ finally:
+ logger.removeFilter(filter_log)
+
+
+_CITATION = """\
+@inproceedings{bert-score,
+ title={BERTScore: Evaluating Text Generation with BERT},
+ author={Tianyi Zhang* and Varsha Kishore* and Felix Wu* and Kilian Q. Weinberger and Yoav Artzi},
+ booktitle={International Conference on Learning Representations},
+ year={2020},
+ url={https://openreview.net/forum?id=SkeHuCVFDr}
+}
+"""
+
+_DESCRIPTION = """\
+BERTScore leverages the pre-trained contextual embeddings from BERT and matches words in candidate and reference
+sentences by cosine similarity.
+It has been shown to correlate with human judgment on sentence-level and system-level evaluation.
+Moreover, BERTScore computes precision, recall, and F1 measure, which can be useful for evaluating different language
+generation tasks.
+
+See the project's README at https://github.com/Tiiiger/bert_score#readme for more information.
+"""
+
+_KWARGS_DESCRIPTION = """
+BERTScore Metrics with the hashcode from a source against one or more references.
+
+Args:
+ predictions (list of str): Prediction/candidate sentences.
+ references (list of str or list of list of str): Reference sentences.
+ lang (str): Language of the sentences; required (e.g. 'en').
+ model_type (str): Bert specification, default using the suggested
+ model for the target language; has to specify at least one of
+ `model_type` or `lang`.
+ num_layers (int): The layer of representation to use,
+ default using the number of layers tuned on WMT16 correlation data.
+ verbose (bool): Turn on intermediate status update.
+ idf (bool or dict): Use idf weighting; can also be a precomputed idf_dict.
+ device (str): On which the contextual embedding model will be allocated on.
+ If this argument is None, the model lives on cuda:0 if cuda is available.
+ nthreads (int): Number of threads.
+ batch_size (int): Bert score processing batch size,
+ at least one of `model_type` or `lang`. `lang` needs to be
+ specified when `rescale_with_baseline` is True.
+ rescale_with_baseline (bool): Rescale bertscore with pre-computed baseline.
+ baseline_path (str): Customized baseline file.
+ use_fast_tokenizer (bool): `use_fast` parameter passed to HF tokenizer. New in version 0.3.10.
+
+Returns:
+ precision: Precision.
+ recall: Recall.
+ f1: F1 score.
+ hashcode: Hashcode of the library.
+
+Examples:
+
+ >>> predictions = ["hello there", "general kenobi"]
+ >>> references = ["hello there", "general kenobi"]
+ >>> bertscore = datasets.load_metric("bertscore")
+ >>> results = bertscore.compute(predictions=predictions, references=references, lang="en")
+ >>> print([round(v, 2) for v in results["f1"]])
+ [1.0, 1.0]
+"""
+
+
+@datasets.utils.file_utils.add_start_docstrings(_DESCRIPTION, _KWARGS_DESCRIPTION)
+class BERTScore(datasets.Metric):
+ def _info(self):
+ return datasets.MetricInfo(
+ description=_DESCRIPTION,
+ citation=_CITATION,
+ homepage="https://github.com/Tiiiger/bert_score",
+ inputs_description=_KWARGS_DESCRIPTION,
+ features=datasets.Features(
+ {
+ "predictions": datasets.Value("string", id="sequence"),
+ "references": datasets.Sequence(datasets.Value("string", id="sequence"), id="references"),
+ }
+ ),
+ codebase_urls=["https://github.com/Tiiiger/bert_score"],
+ reference_urls=[
+ "https://github.com/Tiiiger/bert_score",
+ "https://arxiv.org/abs/1904.09675",
+ ],
+ )
+
+ def _compute(
+ self,
+ predictions,
+ references,
+ lang=None,
+ model_type=None,
+ num_layers=None,
+ verbose=False,
+ idf=False,
+ device=None,
+ batch_size=64,
+ nthreads=4,
+ all_layers=False,
+ rescale_with_baseline=False,
+ baseline_path=None,
+ use_fast_tokenizer=False,
+ ):
+ get_hash = bert_score.utils.get_hash
+ scorer = bert_score.BERTScorer
+
+ if version.parse(bert_score.__version__) >= version.parse("0.3.10"):
+ get_hash = functools.partial(get_hash, use_fast_tokenizer=use_fast_tokenizer)
+ scorer = functools.partial(scorer, use_fast_tokenizer=use_fast_tokenizer)
+ elif use_fast_tokenizer:
+ raise ImportWarning(
+ "To use a fast tokenizer, the module `bert-score>=0.3.10` is required, and the current version of `bert-score` doesn't match this condition.\n"
+ 'You can install it with `pip install "bert-score>=0.3.10"`.'
+ )
+
+ if model_type is None:
+ assert lang is not None, "either lang or model_type should be specified"
+ model_type = bert_score.utils.lang2model[lang.lower()]
+
+ if num_layers is None:
+ num_layers = bert_score.utils.model2layers[model_type]
+
+ hashcode = get_hash(
+ model=model_type,
+ num_layers=num_layers,
+ idf=idf,
+ rescale_with_baseline=rescale_with_baseline,
+ use_custom_baseline=baseline_path is not None,
+ )
+
+ with filter_logging_context():
+ if not hasattr(self, "cached_bertscorer") or self.cached_bertscorer.hash != hashcode:
+ self.cached_bertscorer = scorer(
+ model_type=model_type,
+ num_layers=num_layers,
+ batch_size=batch_size,
+ nthreads=nthreads,
+ all_layers=all_layers,
+ idf=idf,
+ device=device,
+ lang=lang,
+ rescale_with_baseline=rescale_with_baseline,
+ baseline_path=baseline_path,
+ )
+
+ (P, R, F) = self.cached_bertscorer.score(
+ cands=predictions,
+ refs=references,
+ verbose=verbose,
+ batch_size=batch_size,
+ )
+ output_dict = {
+ "precision": P.tolist(),
+ "recall": R.tolist(),
+ "f1": F.tolist(),
+ "hashcode": hashcode,
+ }
+ return output_dict
+
+ def add_batch(self, predictions=None, references=None, **kwargs):
+ """Add a batch of predictions and references for the metric's stack."""
+ # References can be strings or lists of strings
+ # Let's change strings to lists of strings with one element
+ if references is not None:
+ references = [[ref] if isinstance(ref, str) else ref for ref in references]
+ super().add_batch(predictions=predictions, references=references, **kwargs)
+
+ def add(self, prediction=None, reference=None, **kwargs):
+ """Add one prediction and reference for the metric's stack."""
+ # References can be strings or lists of strings
+ # Let's change strings to lists of strings with one element
+ if isinstance(reference, str):
+ reference = [reference]
+ super().add(prediction=prediction, reference=reference, **kwargs)
diff --git a/metrics/bleu/README.md b/metrics/bleu/README.md
new file mode 100644
index 0000000..0191a1a
--- /dev/null
+++ b/metrics/bleu/README.md
@@ -0,0 +1,123 @@
+# Metric Card for BLEU
+
+
+## Metric Description
+BLEU (Bilingual Evaluation Understudy) is an algorithm for evaluating the quality of text which has been machine-translated from one natural language to another. Quality is considered to be the correspondence between a machine's output and that of a human: "the closer a machine translation is to a professional human translation, the better it is" – this is the central idea behind BLEU. BLEU was one of the first metrics to claim a high correlation with human judgements of quality, and remains one of the most popular automated and inexpensive metrics.
+
+Scores are calculated for individual translated segments—generally sentences—by comparing them with a set of good quality reference translations. Those scores are then averaged over the whole corpus to reach an estimate of the translation's overall quality. Neither intelligibility nor grammatical correctness are not taken into account.
+
+## Intended Uses
+BLEU and BLEU-derived metrics are most often used for machine translation.
+
+## How to Use
+
+This metric takes as input lists of predicted sentences and reference sentences:
+
+```python
+>>> predictions = [
+... ["hello", "there", "general", "kenobi"],
+... ["foo", "bar", "foobar"]
+... ]
+>>> references = [
+... [["hello", "there", "general", "kenobi"]],
+... [["foo", "bar", "foobar"]]
+... ]
+>>> bleu = datasets.load_metric("bleu")
+>>> results = bleu.compute(predictions=predictions, references=references)
+>>> print(results)
+{'bleu': 1.0, 'precisions': [1.0, 1.0, 1.0, 1.0], 'brevity_penalty': 1.0, 'length_ratio': 1.0, 'translation_length': 7, 'reference_length': 7}
+```
+
+### Inputs
+- **predictions** (`list`): Translations to score. Each translation should be tokenized into a list of tokens.
+- **references** (`list` of `list`s): references for each translation. Each reference should be tokenized into a list of tokens.
+- **max_order** (`int`): Maximum n-gram order to use when computing BLEU score. Defaults to `4`.
+- **smooth** (`boolean`): Whether or not to apply Lin et al. 2004 smoothing. Defaults to `False`.
+
+### Output Values
+- **bleu** (`float`): bleu score
+- **precisions** (`list` of `float`s): geometric mean of n-gram precisions,
+- **brevity_penalty** (`float`): brevity penalty,
+- **length_ratio** (`float`): ratio of lengths,
+- **translation_length** (`int`): translation_length,
+- **reference_length** (`int`): reference_length
+
+Output Example:
+```python
+{'bleu': 1.0, 'precisions': [1.0, 1.0, 1.0, 1.0], 'brevity_penalty': 1.0, 'length_ratio': 1.167, 'translation_length': 7, 'reference_length': 6}
+```
+
+BLEU's output is always a number between 0 and 1. This value indicates how similar the candidate text is to the reference texts, with values closer to 1 representing more similar texts. Few human translations will attain a score of 1, since this would indicate that the candidate is identical to one of the reference translations. For this reason, it is not necessary to attain a score of 1. Because there are more opportunities to match, adding additional reference translations will increase the BLEU score.
+
+#### Values from Popular Papers
+The [original BLEU paper](https://aclanthology.org/P02-1040/) (Papineni et al. 2002) compares BLEU scores of five different models on the same 500-sentence corpus. These scores ranged from 0.0527 to 0.2571.
+
+The [Attention is All you Need paper](https://proceedings.neurips.cc/paper/2017/file/3f5ee243547dee91fbd053c1c4a845aa-Paper.pdf) (Vaswani et al. 2017) got a BLEU score of 0.284 on the WMT 2014 English-to-German translation task, and 0.41 on the WMT 2014 English-to-French translation task.
+
+### Examples
+
+Example where each sample has 1 reference:
+```python
+>>> predictions = [
+... ["hello", "there", "general", "kenobi"],
+... ["foo", "bar", "foobar"]
+... ]
+>>> references = [
+... [["hello", "there", "general", "kenobi"]],
+... [["foo", "bar", "foobar"]]
+... ]
+>>> bleu = datasets.load_metric("bleu")
+>>> results = bleu.compute(predictions=predictions, references=references)
+>>> print(results)
+{'bleu': 1.0, 'precisions': [1.0, 1.0, 1.0, 1.0], 'brevity_penalty': 1.0, 'length_ratio': 1.0, 'translation_length': 7, 'reference_length': 7}
+```
+
+Example where the first sample has 2 references:
+```python
+>>> predictions = [
+... ["hello", "there", "general", "kenobi"],
+... ["foo", "bar", "foobar"]
+... ]
+>>> references = [
+... [["hello", "there", "general", "kenobi"], ["hello", "there", "!"]],
+... [["foo", "bar", "foobar"]]
+... ]
+>>> bleu = datasets.load_metric("bleu")
+>>> results = bleu.compute(predictions=predictions, references=references)
+>>> print(results)
+{'bleu': 1.0, 'precisions': [1.0, 1.0, 1.0, 1.0], 'brevity_penalty': 1.0, 'length_ratio': 1.1666666666666667, 'translation_length': 7, 'reference_length': 6}
+```
+
+## Limitations and Bias
+This metric hase multiple known limitations and biases:
+- BLEU compares overlap in tokens from the predictions and references, instead of comparing meaning. This can lead to discrepencies between BLEU scores and human ratings.
+- BLEU scores are not comparable across different datasets, nor are they comparable across different languages.
+- BLEU scores can vary greatly depending on which parameters are used to generate the scores, especially when different tokenization and normalization techniques are used. It is therefore not possible to compare BLEU scores generated using different parameters, or when these parameters are unknown.
+- Shorter predicted translations achieve higher scores than longer ones, simply due to how the score is calculated. A brevity penalty is introduced to attempt to counteract this.
+
+
+## Citation
+```bibtex
+@INPROCEEDINGS{Papineni02bleu:a,
+ author = {Kishore Papineni and Salim Roukos and Todd Ward and Wei-jing Zhu},
+ title = {BLEU: a Method for Automatic Evaluation of Machine Translation},
+ booktitle = {},
+ year = {2002},
+ pages = {311--318}
+}
+@inproceedings{lin-och-2004-orange,
+ title = "{ORANGE}: a Method for Evaluating Automatic Evaluation Metrics for Machine Translation",
+ author = "Lin, Chin-Yew and
+ Och, Franz Josef",
+ booktitle = "{COLING} 2004: Proceedings of the 20th International Conference on Computational Linguistics",
+ month = "aug 23{--}aug 27",
+ year = "2004",
+ address = "Geneva, Switzerland",
+ publisher = "COLING",
+ url = "https://www.aclweb.org/anthology/C04-1072",
+ pages = "501--507",
+}
+```
+
+## Further References
+- This Hugging Face implementation uses [this Tensorflow implementation](https://github.com/tensorflow/nmt/blob/master/nmt/scripts/bleu.py)
diff --git a/metrics/bleu/bleu.py b/metrics/bleu/bleu.py
new file mode 100644
index 0000000..545cb1e
--- /dev/null
+++ b/metrics/bleu/bleu.py
@@ -0,0 +1,126 @@
+# Copyright 2020 The HuggingFace Datasets Authors.
+#
+# Licensed under the Apache License, Version 2.0 (the "License");
+# you may not use this file except in compliance with the License.
+# You may obtain a copy of the License at
+#
+# http://www.apache.org/licenses/LICENSE-2.0
+#
+# Unless required by applicable law or agreed to in writing, software
+# distributed under the License is distributed on an "AS IS" BASIS,
+# WITHOUT WARRANTIES OR CONDITIONS OF ANY KIND, either express or implied.
+# See the License for the specific language governing permissions and
+# limitations under the License.
+""" BLEU metric. """
+
+import datasets
+
+from .nmt_bleu import compute_bleu # From: https://github.com/tensorflow/nmt/blob/master/nmt/scripts/bleu.py
+
+
+_CITATION = """\
+@INPROCEEDINGS{Papineni02bleu:a,
+ author = {Kishore Papineni and Salim Roukos and Todd Ward and Wei-jing Zhu},
+ title = {BLEU: a Method for Automatic Evaluation of Machine Translation},
+ booktitle = {},
+ year = {2002},
+ pages = {311--318}
+}
+@inproceedings{lin-och-2004-orange,
+ title = "{ORANGE}: a Method for Evaluating Automatic Evaluation Metrics for Machine Translation",
+ author = "Lin, Chin-Yew and
+ Och, Franz Josef",
+ booktitle = "{COLING} 2004: Proceedings of the 20th International Conference on Computational Linguistics",
+ month = "aug 23{--}aug 27",
+ year = "2004",
+ address = "Geneva, Switzerland",
+ publisher = "COLING",
+ url = "https://www.aclweb.org/anthology/C04-1072",
+ pages = "501--507",
+}
+"""
+
+_DESCRIPTION = """\
+BLEU (bilingual evaluation understudy) is an algorithm for evaluating the quality of text which has been machine-translated from one natural language to another.
+Quality is considered to be the correspondence between a machine's output and that of a human: "the closer a machine translation is to a professional human translation,
+the better it is" – this is the central idea behind BLEU. BLEU was one of the first metrics to claim a high correlation with human judgements of quality, and
+remains one of the most popular automated and inexpensive metrics.
+
+Scores are calculated for individual translated segments—generally sentences—by comparing them with a set of good quality reference translations.
+Those scores are then averaged over the whole corpus to reach an estimate of the translation's overall quality. Intelligibility or grammatical correctness
+are not taken into account[citation needed].
+
+BLEU's output is always a number between 0 and 1. This value indicates how similar the candidate text is to the reference texts, with values closer to 1
+representing more similar texts. Few human translations will attain a score of 1, since this would indicate that the candidate is identical to one of the
+reference translations. For this reason, it is not necessary to attain a score of 1. Because there are more opportunities to match, adding additional
+reference translations will increase the BLEU score.
+"""
+
+_KWARGS_DESCRIPTION = """
+Computes BLEU score of translated segments against one or more references.
+Args:
+ predictions: list of translations to score.
+ Each translation should be tokenized into a list of tokens.
+ references: list of lists of references for each translation.
+ Each reference should be tokenized into a list of tokens.
+ max_order: Maximum n-gram order to use when computing BLEU score.
+ smooth: Whether or not to apply Lin et al. 2004 smoothing.
+Returns:
+ 'bleu': bleu score,
+ 'precisions': geometric mean of n-gram precisions,
+ 'brevity_penalty': brevity penalty,
+ 'length_ratio': ratio of lengths,
+ 'translation_length': translation_length,
+ 'reference_length': reference_length
+Examples:
+
+ >>> predictions = [
+ ... ["hello", "there", "general", "kenobi"], # tokenized prediction of the first sample
+ ... ["foo", "bar", "foobar"] # tokenized prediction of the second sample
+ ... ]
+ >>> references = [
+ ... [["hello", "there", "general", "kenobi"], ["hello", "there", "!"]], # tokenized references for the first sample (2 references)
+ ... [["foo", "bar", "foobar"]] # tokenized references for the second sample (1 reference)
+ ... ]
+ >>> bleu = datasets.load_metric("bleu")
+ >>> results = bleu.compute(predictions=predictions, references=references)
+ >>> print(results["bleu"])
+ 1.0
+"""
+
+
+@datasets.utils.file_utils.add_start_docstrings(_DESCRIPTION, _KWARGS_DESCRIPTION)
+class Bleu(datasets.Metric):
+ def _info(self):
+ return datasets.MetricInfo(
+ description=_DESCRIPTION,
+ citation=_CITATION,
+ inputs_description=_KWARGS_DESCRIPTION,
+ features=datasets.Features(
+ {
+ "predictions": datasets.Sequence(datasets.Value("string", id="token"), id="sequence"),
+ "references": datasets.Sequence(
+ datasets.Sequence(datasets.Value("string", id="token"), id="sequence"), id="references"
+ ),
+ }
+ ),
+ codebase_urls=["https://github.com/tensorflow/nmt/blob/master/nmt/scripts/bleu.py"],
+ reference_urls=[
+ "https://en.wikipedia.org/wiki/BLEU",
+ "https://towardsdatascience.com/evaluating-text-output-in-nlp-bleu-at-your-own-risk-e8609665a213",
+ ],
+ )
+
+ def _compute(self, predictions, references, max_order=4, smooth=False):
+ score = compute_bleu(
+ reference_corpus=references, translation_corpus=predictions, max_order=max_order, smooth=smooth
+ )
+ (bleu, precisions, bp, ratio, translation_length, reference_length) = score
+ return {
+ "bleu": bleu,
+ "precisions": precisions,
+ "brevity_penalty": bp,
+ "length_ratio": ratio,
+ "translation_length": translation_length,
+ "reference_length": reference_length,
+ }
diff --git a/metrics/bleurt/bleurt.py b/metrics/bleurt/bleurt.py
new file mode 100644
index 0000000..3a791a5
--- /dev/null
+++ b/metrics/bleurt/bleurt.py
@@ -0,0 +1,124 @@
+# Copyright 2020 The HuggingFace Datasets Authors.
+#
+# Licensed under the Apache License, Version 2.0 (the "License");
+# you may not use this file except in compliance with the License.
+# You may obtain a copy of the License at
+#
+# http://www.apache.org/licenses/LICENSE-2.0
+#
+# Unless required by applicable law or agreed to in writing, software
+# distributed under the License is distributed on an "AS IS" BASIS,
+# WITHOUT WARRANTIES OR CONDITIONS OF ANY KIND, either express or implied.
+# See the License for the specific language governing permissions and
+# limitations under the License.
+""" BLEURT metric. """
+
+import os
+
+from bleurt import score # From: git+https://github.com/google-research/bleurt.git
+
+import datasets
+
+
+logger = datasets.logging.get_logger(__name__)
+
+
+_CITATION = """\
+@inproceedings{bleurt,
+ title={BLEURT: Learning Robust Metrics for Text Generation},
+ author={Thibault Sellam and Dipanjan Das and Ankur P. Parikh},
+ booktitle={ACL},
+ year={2020},
+ url={https://arxiv.org/abs/2004.04696}
+}
+"""
+
+_DESCRIPTION = """\
+BLEURT a learnt evaluation metric for Natural Language Generation. It is built using multiple phases of transfer learning starting from a pretrained BERT model (Devlin et al. 2018)
+and then employing another pre-training phrase using synthetic data. Finally it is trained on WMT human annotations. You may run BLEURT out-of-the-box or fine-tune
+it for your specific application (the latter is expected to perform better).
+
+See the project's README at https://github.com/google-research/bleurt#readme for more information.
+"""
+
+_KWARGS_DESCRIPTION = """
+BLEURT score.
+
+Args:
+ `predictions` (list of str): prediction/candidate sentences
+ `references` (list of str): reference sentences
+ `checkpoint` BLEURT checkpoint. Will default to BLEURT-tiny if None.
+
+Returns:
+ 'scores': List of scores.
+Examples:
+
+ >>> predictions = ["hello there", "general kenobi"]
+ >>> references = ["hello there", "general kenobi"]
+ >>> bleurt = datasets.load_metric("bleurt")
+ >>> results = bleurt.compute(predictions=predictions, references=references)
+ >>> print([round(v, 2) for v in results["scores"]])
+ [1.03, 1.04]
+"""
+
+CHECKPOINT_URLS = {
+ "bleurt-tiny-128": "https://storage.googleapis.com/bleurt-oss/bleurt-tiny-128.zip",
+ "bleurt-tiny-512": "https://storage.googleapis.com/bleurt-oss/bleurt-tiny-512.zip",
+ "bleurt-base-128": "https://storage.googleapis.com/bleurt-oss/bleurt-base-128.zip",
+ "bleurt-base-512": "https://storage.googleapis.com/bleurt-oss/bleurt-base-512.zip",
+ "bleurt-large-128": "https://storage.googleapis.com/bleurt-oss/bleurt-large-128.zip",
+ "bleurt-large-512": "https://storage.googleapis.com/bleurt-oss/bleurt-large-512.zip",
+ "BLEURT-20-D3": "https://storage.googleapis.com/bleurt-oss-21/BLEURT-20-D3.zip",
+ "BLEURT-20-D6": "https://storage.googleapis.com/bleurt-oss-21/BLEURT-20-D6.zip",
+ "BLEURT-20-D12": "https://storage.googleapis.com/bleurt-oss-21/BLEURT-20-D12.zip",
+ "BLEURT-20": "https://storage.googleapis.com/bleurt-oss-21/BLEURT-20.zip",
+}
+
+
+@datasets.utils.file_utils.add_start_docstrings(_DESCRIPTION, _KWARGS_DESCRIPTION)
+class BLEURT(datasets.Metric):
+ def _info(self):
+
+ return datasets.MetricInfo(
+ description=_DESCRIPTION,
+ citation=_CITATION,
+ homepage="https://github.com/google-research/bleurt",
+ inputs_description=_KWARGS_DESCRIPTION,
+ features=datasets.Features(
+ {
+ "predictions": datasets.Value("string", id="sequence"),
+ "references": datasets.Value("string", id="sequence"),
+ }
+ ),
+ codebase_urls=["https://github.com/google-research/bleurt"],
+ reference_urls=["https://github.com/google-research/bleurt", "https://arxiv.org/abs/2004.04696"],
+ )
+
+ def _download_and_prepare(self, dl_manager):
+
+ # check that config name specifies a valid BLEURT model
+ if self.config_name == "default":
+ logger.warning(
+ "Using default BLEURT-Base checkpoint for sequence maximum length 128. "
+ "You can use a bigger model for better results with e.g.: datasets.load_metric('bleurt', 'bleurt-large-512')."
+ )
+ self.config_name = "bleurt-base-128"
+
+ if self.config_name.lower() in CHECKPOINT_URLS:
+ checkpoint_name = self.config_name.lower()
+
+ elif self.config_name.upper() in CHECKPOINT_URLS:
+ checkpoint_name = self.config_name.upper()
+
+ else:
+ raise KeyError(
+ f"{self.config_name} model not found. You should supply the name of a model checkpoint for bleurt in {CHECKPOINT_URLS.keys()}"
+ )
+
+ # download the model checkpoint specified by self.config_name and set up the scorer
+ model_path = dl_manager.download_and_extract(CHECKPOINT_URLS[checkpoint_name])
+ self.scorer = score.BleurtScorer(os.path.join(model_path, checkpoint_name))
+
+ def _compute(self, predictions, references):
+ scores = self.scorer.score(references=references, candidates=predictions)
+ return {"scores": scores}
diff --git a/metrics/cer/README.md b/metrics/cer/README.md
new file mode 100644
index 0000000..f3661a3
--- /dev/null
+++ b/metrics/cer/README.md
@@ -0,0 +1,124 @@
+# Metric Card for CER
+
+## Metric description
+
+Character error rate (CER) is a common metric of the performance of an automatic speech recognition (ASR) system. CER is similar to Word Error Rate (WER), but operates on character instead of word.
+
+Character error rate can be computed as:
+
+`CER = (S + D + I) / N = (S + D + I) / (S + D + C)`
+
+where
+
+`S` is the number of substitutions,
+
+`D` is the number of deletions,
+
+`I` is the number of insertions,
+
+`C` is the number of correct characters,
+
+`N` is the number of characters in the reference (`N=S+D+C`).
+
+
+## How to use
+
+The metric takes two inputs: references (a list of references for each speech input) and predictions (a list of transcriptions to score).
+
+```python
+from datasets import load_metric
+cer = load_metric("cer")
+cer_score = cer.compute(predictions=predictions, references=references)
+```
+## Output values
+
+This metric outputs a float representing the character error rate.
+
+```
+print(cer_score)
+0.34146341463414637
+```
+
+The **lower** the CER value, the **better** the performance of the ASR system, with a CER of 0 being a perfect score.
+
+However, CER's output is not always a number between 0 and 1, in particular when there is a high number of insertions (see [Examples](#Examples) below).
+
+### Values from popular papers
+
+This metric is highly dependent on the content and quality of the dataset, and therefore users can expect very different values for the same model but on different datasets.
+
+Multilingual datasets such as [Common Voice](https://huggingface.co/datasets/common_voice) report different CERs depending on the language, ranging from 0.02-0.03 for languages such as French and Italian, to 0.05-0.07 for English (see [here](https://github.com/speechbrain/speechbrain/tree/develop/recipes/CommonVoice/ASR/CTC) for more values).
+
+## Examples
+
+Perfect match between prediction and reference:
+
+```python
+from datasets import load_metric
+cer = load_metric("cer")
+predictions = ["hello world", "good night moon"]
+references = ["hello world", "good night moon"]
+cer_score = cer.compute(predictions=predictions, references=references)
+print(cer_score)
+0.0
+```
+
+Partial match between prediction and reference:
+
+```python
+from datasets import load_metric
+cer = load_metric("cer")
+predictions = ["this is the prediction", "there is an other sample"]
+references = ["this is the reference", "there is another one"]
+cer_score = cer.compute(predictions=predictions, references=references)
+print(cer_score)
+0.34146341463414637
+```
+
+No match between prediction and reference:
+
+```python
+from datasets import load_metric
+cer = load_metric("cer")
+predictions = ["hello"]
+references = ["gracias"]
+cer_score = cer.compute(predictions=predictions, references=references)
+print(cer_score)
+1.0
+```
+
+CER above 1 due to insertion errors:
+
+```python
+from datasets import load_metric
+cer = load_metric("cer")
+predictions = ["hello world"]
+references = ["hello"]
+cer_score = cer.compute(predictions=predictions, references=references)
+print(cer_score)
+1.2
+```
+
+## Limitations and bias
+
+CER is useful for comparing different models for tasks such as automatic speech recognition (ASR) and optic character recognition (OCR), especially for multilingual datasets where WER is not suitable given the diversity of languages. However, CER provides no details on the nature of translation errors and further work is therefore required to identify the main source(s) of error and to focus any research effort.
+
+Also, in some cases, instead of reporting the raw CER, a normalized CER is reported where the number of mistakes is divided by the sum of the number of edit operations (`I` + `S` + `D`) and `C` (the number of correct characters), which results in CER values that fall within the range of 0–100%.
+
+
+## Citation
+
+
+```bibtex
+@inproceedings{morris2004,
+author = {Morris, Andrew and Maier, Viktoria and Green, Phil},
+year = {2004},
+month = {01},
+pages = {},
+title = {From WER and RIL to MER and WIL: improved evaluation measures for connected speech recognition.}
+}
+```
+
+## Further References
+
+- [Hugging Face Tasks -- Automatic Speech Recognition](https://huggingface.co/tasks/automatic-speech-recognition)
diff --git a/metrics/cer/cer.py b/metrics/cer/cer.py
new file mode 100644
index 0000000..0e3732d
--- /dev/null
+++ b/metrics/cer/cer.py
@@ -0,0 +1,158 @@
+# Copyright 2021 The HuggingFace Datasets Authors.
+#
+# Licensed under the Apache License, Version 2.0 (the "License");
+# you may not use this file except in compliance with the License.
+# You may obtain a copy of the License at
+#
+# http://www.apache.org/licenses/LICENSE-2.0
+#
+# Unless required by applicable law or agreed to in writing, software
+# distributed under the License is distributed on an "AS IS" BASIS,
+# WITHOUT WARRANTIES OR CONDITIONS OF ANY KIND, either express or implied.
+# See the License for the specific language governing permissions and
+# limitations under the License.
+""" Character Error Ratio (CER) metric. """
+
+from typing import List
+
+import jiwer
+import jiwer.transforms as tr
+from packaging import version
+
+import datasets
+from datasets.config import PY_VERSION
+
+
+if PY_VERSION < version.parse("3.8"):
+ import importlib_metadata
+else:
+ import importlib.metadata as importlib_metadata
+
+
+SENTENCE_DELIMITER = ""
+
+
+if version.parse(importlib_metadata.version("jiwer")) < version.parse("2.3.0"):
+
+ class SentencesToListOfCharacters(tr.AbstractTransform):
+ def __init__(self, sentence_delimiter: str = " "):
+ self.sentence_delimiter = sentence_delimiter
+
+ def process_string(self, s: str):
+ return list(s)
+
+ def process_list(self, inp: List[str]):
+ chars = []
+ for sent_idx, sentence in enumerate(inp):
+ chars.extend(self.process_string(sentence))
+ if self.sentence_delimiter is not None and self.sentence_delimiter != "" and sent_idx < len(inp) - 1:
+ chars.append(self.sentence_delimiter)
+ return chars
+
+ cer_transform = tr.Compose(
+ [tr.RemoveMultipleSpaces(), tr.Strip(), SentencesToListOfCharacters(SENTENCE_DELIMITER)]
+ )
+else:
+ cer_transform = tr.Compose(
+ [
+ tr.RemoveMultipleSpaces(),
+ tr.Strip(),
+ tr.ReduceToSingleSentence(SENTENCE_DELIMITER),
+ tr.ReduceToListOfListOfChars(),
+ ]
+ )
+
+
+_CITATION = """\
+@inproceedings{inproceedings,
+ author = {Morris, Andrew and Maier, Viktoria and Green, Phil},
+ year = {2004},
+ month = {01},
+ pages = {},
+ title = {From WER and RIL to MER and WIL: improved evaluation measures for connected speech recognition.}
+}
+"""
+
+_DESCRIPTION = """\
+Character error rate (CER) is a common metric of the performance of an automatic speech recognition system.
+
+CER is similar to Word Error Rate (WER), but operates on character instead of word. Please refer to docs of WER for further information.
+
+Character error rate can be computed as:
+
+CER = (S + D + I) / N = (S + D + I) / (S + D + C)
+
+where
+
+S is the number of substitutions,
+D is the number of deletions,
+I is the number of insertions,
+C is the number of correct characters,
+N is the number of characters in the reference (N=S+D+C).
+
+CER's output is not always a number between 0 and 1, in particular when there is a high number of insertions. This value is often associated to the percentage of characters that were incorrectly predicted. The lower the value, the better the
+performance of the ASR system with a CER of 0 being a perfect score.
+"""
+
+_KWARGS_DESCRIPTION = """
+Computes CER score of transcribed segments against references.
+Args:
+ references: list of references for each speech input.
+ predictions: list of transcribtions to score.
+ concatenate_texts: Whether or not to concatenate sentences before evaluation, set to True for more accurate result.
+Returns:
+ (float): the character error rate
+
+Examples:
+
+ >>> predictions = ["this is the prediction", "there is an other sample"]
+ >>> references = ["this is the reference", "there is another one"]
+ >>> cer = datasets.load_metric("cer")
+ >>> cer_score = cer.compute(predictions=predictions, references=references)
+ >>> print(cer_score)
+ 0.34146341463414637
+"""
+
+
+@datasets.utils.file_utils.add_start_docstrings(_DESCRIPTION, _KWARGS_DESCRIPTION)
+class CER(datasets.Metric):
+ def _info(self):
+ return datasets.MetricInfo(
+ description=_DESCRIPTION,
+ citation=_CITATION,
+ inputs_description=_KWARGS_DESCRIPTION,
+ features=datasets.Features(
+ {
+ "predictions": datasets.Value("string", id="sequence"),
+ "references": datasets.Value("string", id="sequence"),
+ }
+ ),
+ codebase_urls=["https://github.com/jitsi/jiwer/"],
+ reference_urls=[
+ "https://en.wikipedia.org/wiki/Word_error_rate",
+ "https://sites.google.com/site/textdigitisation/qualitymeasures/computingerrorrates",
+ ],
+ )
+
+ def _compute(self, predictions, references, concatenate_texts=False):
+ if concatenate_texts:
+ return jiwer.compute_measures(
+ references,
+ predictions,
+ truth_transform=cer_transform,
+ hypothesis_transform=cer_transform,
+ )["wer"]
+
+ incorrect = 0
+ total = 0
+ for prediction, reference in zip(predictions, references):
+ measures = jiwer.compute_measures(
+ reference,
+ prediction,
+ truth_transform=cer_transform,
+ hypothesis_transform=cer_transform,
+ )
+ incorrect += measures["substitutions"] + measures["deletions"] + measures["insertions"]
+ total += measures["substitutions"] + measures["deletions"] + measures["hits"]
+
+ return incorrect / total
diff --git a/metrics/cer/test_cer.py b/metrics/cer/test_cer.py
new file mode 100644
index 0000000..3344ce3
--- /dev/null
+++ b/metrics/cer/test_cer.py
@@ -0,0 +1,128 @@
+# Copyright 2021 The HuggingFace Datasets Authors.
+#
+# Licensed under the Apache License, Version 2.0 (the "License");
+# you may not use this file except in compliance with the License.
+# You may obtain a copy of the License at
+#
+# http://www.apache.org/licenses/LICENSE-2.0
+#
+# Unless required by applicable law or agreed to in writing, software
+# distributed under the License is distributed on an "AS IS" BASIS,
+# WITHOUT WARRANTIES OR CONDITIONS OF ANY KIND, either express or implied.
+# See the License for the specific language governing permissions and
+# limitations under the License.
+import unittest
+
+from cer import CER
+
+
+cer = CER()
+
+
+class TestCER(unittest.TestCase):
+ def test_cer_case_senstive(self):
+ refs = ["White House"]
+ preds = ["white house"]
+ # S = 2, D = 0, I = 0, N = 11, CER = 2 / 11
+ char_error_rate = cer.compute(predictions=preds, references=refs)
+ self.assertTrue(abs(char_error_rate - 0.1818181818) < 1e-6)
+
+ def test_cer_whitespace(self):
+ refs = ["were wolf"]
+ preds = ["werewolf"]
+ # S = 0, D = 0, I = 1, N = 9, CER = 1 / 9
+ char_error_rate = cer.compute(predictions=preds, references=refs)
+ self.assertTrue(abs(char_error_rate - 0.1111111) < 1e-6)
+
+ refs = ["werewolf"]
+ preds = ["weae wolf"]
+ # S = 1, D = 1, I = 0, N = 8, CER = 0.25
+ char_error_rate = cer.compute(predictions=preds, references=refs)
+ self.assertTrue(abs(char_error_rate - 0.25) < 1e-6)
+
+ # consecutive whitespaces case 1
+ refs = ["were wolf"]
+ preds = ["were wolf"]
+ # S = 0, D = 0, I = 0, N = 9, CER = 0
+ char_error_rate = cer.compute(predictions=preds, references=refs)
+ self.assertTrue(abs(char_error_rate - 0.0) < 1e-6)
+
+ # consecutive whitespaces case 2
+ refs = ["were wolf"]
+ preds = ["were wolf"]
+ # S = 0, D = 0, I = 0, N = 9, CER = 0
+ char_error_rate = cer.compute(predictions=preds, references=refs)
+ self.assertTrue(abs(char_error_rate - 0.0) < 1e-6)
+
+ def test_cer_sub(self):
+ refs = ["werewolf"]
+ preds = ["weaewolf"]
+ # S = 1, D = 0, I = 0, N = 8, CER = 0.125
+ char_error_rate = cer.compute(predictions=preds, references=refs)
+ self.assertTrue(abs(char_error_rate - 0.125) < 1e-6)
+
+ def test_cer_del(self):
+ refs = ["werewolf"]
+ preds = ["wereawolf"]
+ # S = 0, D = 1, I = 0, N = 8, CER = 0.125
+ char_error_rate = cer.compute(predictions=preds, references=refs)
+ self.assertTrue(abs(char_error_rate - 0.125) < 1e-6)
+
+ def test_cer_insert(self):
+ refs = ["werewolf"]
+ preds = ["wereolf"]
+ # S = 0, D = 0, I = 1, N = 8, CER = 0.125
+ char_error_rate = cer.compute(predictions=preds, references=refs)
+ self.assertTrue(abs(char_error_rate - 0.125) < 1e-6)
+
+ def test_cer_equal(self):
+ refs = ["werewolf"]
+ char_error_rate = cer.compute(predictions=refs, references=refs)
+ self.assertEqual(char_error_rate, 0.0)
+
+ def test_cer_list_of_seqs(self):
+ refs = ["werewolf", "I am your father"]
+ char_error_rate = cer.compute(predictions=refs, references=refs)
+ self.assertEqual(char_error_rate, 0.0)
+
+ refs = ["werewolf", "I am your father", "doge"]
+ preds = ["werxwolf", "I am your father", "doge"]
+ # S = 1, D = 0, I = 0, N = 28, CER = 1 / 28
+ char_error_rate = cer.compute(predictions=preds, references=refs)
+ self.assertTrue(abs(char_error_rate - 0.03571428) < 1e-6)
+
+ def test_correlated_sentences(self):
+ refs = ["My hovercraft", "is full of eels"]
+ preds = ["My hovercraft is full", " of eels"]
+ # S = 0, D = 0, I = 2, N = 28, CER = 2 / 28
+ # whitespace at the front of " of eels" will be strip during preporcessing
+ # so need to insert 2 whitespaces
+ char_error_rate = cer.compute(predictions=preds, references=refs, concatenate_texts=True)
+ self.assertTrue(abs(char_error_rate - 0.071428) < 1e-6)
+
+ def test_cer_unicode(self):
+ refs = ["我能吞下玻璃而不伤身体"]
+ preds = [" 能吞虾玻璃而 不霜身体啦"]
+ # S = 3, D = 2, I = 0, N = 11, CER = 5 / 11
+ char_error_rate = cer.compute(predictions=preds, references=refs)
+ self.assertTrue(abs(char_error_rate - 0.4545454545) < 1e-6)
+
+ refs = ["我能吞下玻璃", "而不伤身体"]
+ preds = ["我 能 吞 下 玻 璃", "而不伤身体"]
+ # S = 0, D = 5, I = 0, N = 11, CER = 5 / 11
+ char_error_rate = cer.compute(predictions=preds, references=refs)
+ self.assertTrue(abs(char_error_rate - 0.454545454545) < 1e-6)
+
+ refs = ["我能吞下玻璃而不伤身体"]
+ char_error_rate = cer.compute(predictions=refs, references=refs)
+ self.assertFalse(char_error_rate, 0.0)
+
+ def test_cer_empty(self):
+ refs = [""]
+ preds = ["Hypothesis"]
+ with self.assertRaises(ValueError):
+ cer.compute(predictions=preds, references=refs)
+
+
+if __name__ == "__main__":
+ unittest.main()
diff --git a/metrics/chrf/README.md b/metrics/chrf/README.md
new file mode 100644
index 0000000..a3a602a
--- /dev/null
+++ b/metrics/chrf/README.md
@@ -0,0 +1,133 @@
+# Metric Card for chrF(++)
+
+
+## Metric Description
+ChrF and ChrF++ are two MT evaluation metrics that use the F-score statistic for character n-gram matches. ChrF++ additionally includes word n-grams, which correlate more strongly with direct assessment. We use the implementation that is already present in sacrebleu.
+
+While this metric is included in sacreBLEU, the implementation here is slightly different from sacreBLEU in terms of the required input format. Here, the length of the references and hypotheses lists need to be the same, so you may need to transpose your references compared to sacrebleu's required input format. See https://github.com/huggingface/datasets/issues/3154#issuecomment-950746534
+
+See the [sacreBLEU README.md](https://github.com/mjpost/sacreBLEU#chrf--chrf) for more information.
+
+
+## How to Use
+At minimum, this metric requires a `list` of predictions and a `list` of `list`s of references:
+```python
+>>> prediction = ["The relationship between cats and dogs is not exactly friendly.", "a good bookshop is just a genteel black hole that knows how to read."]
+>>> reference = [["The relationship between dogs and cats is not exactly friendly.", ], ["A good bookshop is just a genteel Black Hole that knows how to read."]]
+>>> chrf = datasets.load_metric("chrf")
+>>> results = chrf.compute(predictions=prediction, references=reference)
+>>> print(results)
+{'score': 84.64214891738334, 'char_order': 6, 'word_order': 0, 'beta': 2}
+```
+
+### Inputs
+- **`predictions`** (`list` of `str`): The predicted sentences.
+- **`references`** (`list` of `list` of `str`): The references. There should be one reference sub-list for each prediction sentence.
+- **`char_order`** (`int`): Character n-gram order. Defaults to `6`.
+- **`word_order`** (`int`): Word n-gram order. If equals to 2, the metric is referred to as chrF++. Defaults to `0`.
+- **`beta`** (`int`): Determine the importance of recall w.r.t precision. Defaults to `2`.
+- **`lowercase`** (`bool`): If `True`, enables case-insensitivity. Defaults to `False`.
+- **`whitespace`** (`bool`): If `True`, include whitespaces when extracting character n-grams. Defaults to `False`.
+- **`eps_smoothing`** (`bool`): If `True`, applies epsilon smoothing similar to reference chrF++.py, NLTK, and Moses implementations. If `False`, takes into account effective match order similar to sacreBLEU < 2.0.0. Defaults to `False`.
+
+
+
+### Output Values
+The output is a dictionary containing the following fields:
+- **`'score'`** (`float`): The chrF (chrF++) score.
+- **`'char_order'`** (`int`): The character n-gram order.
+- **`'word_order'`** (`int`): The word n-gram order. If equals to `2`, the metric is referred to as chrF++.
+- **`'beta'`** (`int`): Determine the importance of recall w.r.t precision.
+
+
+The output is formatted as below:
+```python
+{'score': 61.576379378113785, 'char_order': 6, 'word_order': 0, 'beta': 2}
+```
+
+The chrF(++) score can be any value between `0.0` and `100.0`, inclusive.
+
+#### Values from Popular Papers
+
+
+### Examples
+A simple example of calculating chrF:
+```python
+>>> prediction = ["The relationship between cats and dogs is not exactly friendly.", "a good bookshop is just a genteel black hole that knows how to read."]
+>>> reference = [["The relationship between dogs and cats is not exactly friendly.", ], ["A good bookshop is just a genteel Black Hole that knows how to read."]]
+>>> chrf = datasets.load_metric("chrf")
+>>> results = chrf.compute(predictions=prediction, references=reference)
+>>> print(results)
+{'score': 84.64214891738334, 'char_order': 6, 'word_order': 0, 'beta': 2}
+```
+
+The same example, but with the argument `word_order=2`, to calculate chrF++ instead of chrF:
+```python
+>>> prediction = ["The relationship between cats and dogs is not exactly friendly.", "a good bookshop is just a genteel black hole that knows how to read."]
+>>> reference = [["The relationship between dogs and cats is not exactly friendly.", ], ["A good bookshop is just a genteel Black Hole that knows how to read."]]
+>>> chrf = datasets.load_metric("chrf")
+>>> results = chrf.compute(predictions=prediction,
+... references=reference,
+... word_order=2)
+>>> print(results)
+{'score': 82.87263732906315, 'char_order': 6, 'word_order': 2, 'beta': 2}
+```
+
+The same chrF++ example as above, but with `lowercase=True` to normalize all case:
+```python
+>>> prediction = ["The relationship between cats and dogs is not exactly friendly.", "a good bookshop is just a genteel black hole that knows how to read."]
+>>> reference = [["The relationship between dogs and cats is not exactly friendly.", ], ["A good bookshop is just a genteel Black Hole that knows how to read."]]
+>>> chrf = datasets.load_metric("chrf")
+>>> results = chrf.compute(predictions=prediction,
+... references=reference,
+... word_order=2,
+... lowercase=True)
+>>> print(results)
+{'score': 92.12853119829202, 'char_order': 6, 'word_order': 2, 'beta': 2}
+```
+
+
+## Limitations and Bias
+- According to [Popović 2017](https://www.statmt.org/wmt17/pdf/WMT70.pdf), chrF+ (where `word_order=1`) and chrF++ (where `word_order=2`) produce scores that correlate better with human judgements than chrF (where `word_order=0`) does.
+
+## Citation
+```bibtex
+@inproceedings{popovic-2015-chrf,
+ title = "chr{F}: character n-gram {F}-score for automatic {MT} evaluation",
+ author = "Popovi{\'c}, Maja",
+ booktitle = "Proceedings of the Tenth Workshop on Statistical Machine Translation",
+ month = sep,
+ year = "2015",
+ address = "Lisbon, Portugal",
+ publisher = "Association for Computational Linguistics",
+ url = "https://aclanthology.org/W15-3049",
+ doi = "10.18653/v1/W15-3049",
+ pages = "392--395",
+}
+@inproceedings{popovic-2017-chrf,
+ title = "chr{F}++: words helping character n-grams",
+ author = "Popovi{\'c}, Maja",
+ booktitle = "Proceedings of the Second Conference on Machine Translation",
+ month = sep,
+ year = "2017",
+ address = "Copenhagen, Denmark",
+ publisher = "Association for Computational Linguistics",
+ url = "https://aclanthology.org/W17-4770",
+ doi = "10.18653/v1/W17-4770",
+ pages = "612--618",
+}
+@inproceedings{post-2018-call,
+ title = "A Call for Clarity in Reporting {BLEU} Scores",
+ author = "Post, Matt",
+ booktitle = "Proceedings of the Third Conference on Machine Translation: Research Papers",
+ month = oct,
+ year = "2018",
+ address = "Belgium, Brussels",
+ publisher = "Association for Computational Linguistics",
+ url = "https://www.aclweb.org/anthology/W18-6319",
+ pages = "186--191",
+}
+```
+
+## Further References
+- See the [sacreBLEU README.md](https://github.com/mjpost/sacreBLEU#chrf--chrf) for more information on this implementation.
\ No newline at end of file
diff --git a/metrics/chrf/chrf.py b/metrics/chrf/chrf.py
new file mode 100644
index 0000000..e2047f4
--- /dev/null
+++ b/metrics/chrf/chrf.py
@@ -0,0 +1,174 @@
+# Copyright 2021 The HuggingFace Datasets Authors.
+#
+# Licensed under the Apache License, Version 2.0 (the "License");
+# you may not use this file except in compliance with the License.
+# You may obtain a copy of the License at
+#
+# http://www.apache.org/licenses/LICENSE-2.0
+#
+# Unless required by applicable law or agreed to in writing, software
+# distributed under the License is distributed on an "AS IS" BASIS,
+# WITHOUT WARRANTIES OR CONDITIONS OF ANY KIND, either express or implied.
+# See the License for the specific language governing permissions and
+# limitations under the License.
+""" Chrf(++) metric as available in sacrebleu. """
+import sacrebleu as scb
+from packaging import version
+from sacrebleu import CHRF
+
+import datasets
+
+
+_CITATION = """\
+@inproceedings{popovic-2015-chrf,
+ title = "chr{F}: character n-gram {F}-score for automatic {MT} evaluation",
+ author = "Popovi{\'c}, Maja",
+ booktitle = "Proceedings of the Tenth Workshop on Statistical Machine Translation",
+ month = sep,
+ year = "2015",
+ address = "Lisbon, Portugal",
+ publisher = "Association for Computational Linguistics",
+ url = "https://aclanthology.org/W15-3049",
+ doi = "10.18653/v1/W15-3049",
+ pages = "392--395",
+}
+@inproceedings{popovic-2017-chrf,
+ title = "chr{F}++: words helping character n-grams",
+ author = "Popovi{\'c}, Maja",
+ booktitle = "Proceedings of the Second Conference on Machine Translation",
+ month = sep,
+ year = "2017",
+ address = "Copenhagen, Denmark",
+ publisher = "Association for Computational Linguistics",
+ url = "https://aclanthology.org/W17-4770",
+ doi = "10.18653/v1/W17-4770",
+ pages = "612--618",
+}
+@inproceedings{post-2018-call,
+ title = "A Call for Clarity in Reporting {BLEU} Scores",
+ author = "Post, Matt",
+ booktitle = "Proceedings of the Third Conference on Machine Translation: Research Papers",
+ month = oct,
+ year = "2018",
+ address = "Belgium, Brussels",
+ publisher = "Association for Computational Linguistics",
+ url = "https://www.aclweb.org/anthology/W18-6319",
+ pages = "186--191",
+}
+"""
+
+_DESCRIPTION = """\
+ChrF and ChrF++ are two MT evaluation metrics. They both use the F-score statistic for character n-gram matches,
+and ChrF++ adds word n-grams as well which correlates more strongly with direct assessment. We use the implementation
+that is already present in sacrebleu.
+
+The implementation here is slightly different from sacrebleu in terms of the required input format. The length of
+the references and hypotheses lists need to be the same, so you may need to transpose your references compared to
+sacrebleu's required input format. See https://github.com/huggingface/datasets/issues/3154#issuecomment-950746534
+
+See the README.md file at https://github.com/mjpost/sacreBLEU#chrf--chrf for more information.
+"""
+
+_KWARGS_DESCRIPTION = """
+Produces ChrF(++) scores for hypotheses given reference translations.
+
+Args:
+ predictions (list of str): The predicted sentences.
+ references (list of list of str): The references. There should be one reference sub-list for each prediction sentence.
+ char_order (int): Character n-gram order. Defaults to `6`.
+ word_order (int): Word n-gram order. If equals to `2`, the metric is referred to as chrF++. Defaults to `0`.
+ beta (int): Determine the importance of recall w.r.t precision. Defaults to `2`.
+ lowercase (bool): if `True`, enables case-insensitivity. Defaults to `False`.
+ whitespace (bool): If `True`, include whitespaces when extracting character n-grams.
+ eps_smoothing (bool): If `True`, applies epsilon smoothing similar
+ to reference chrF++.py, NLTK and Moses implementations. If `False`,
+ it takes into account effective match order similar to sacreBLEU < 2.0.0. Defaults to `False`.
+
+Returns:
+ 'score' (float): The chrF (chrF++) score,
+ 'char_order' (int): The character n-gram order,
+ 'word_order' (int): The word n-gram order. If equals to 2, the metric is referred to as chrF++,
+ 'beta' (int): Determine the importance of recall w.r.t precision
+
+Examples:
+ Example 1--a simple example of calculating chrF:
+ >>> prediction = ["The relationship between cats and dogs is not exactly friendly.", "a good bookshop is just a genteel black hole that knows how to read."]
+ >>> reference = [["The relationship between dogs and cats is not exactly friendly."], ["A good bookshop is just a genteel Black Hole that knows how to read."]]
+ >>> chrf = datasets.load_metric("chrf")
+ >>> results = chrf.compute(predictions=prediction, references=reference)
+ >>> print(results)
+ {'score': 84.64214891738334, 'char_order': 6, 'word_order': 0, 'beta': 2}
+
+ Example 2--the same example, but with the argument word_order=2, to calculate chrF++ instead of chrF:
+ >>> prediction = ["The relationship between cats and dogs is not exactly friendly.", "a good bookshop is just a genteel black hole that knows how to read."]
+ >>> reference = [["The relationship between dogs and cats is not exactly friendly."], ["A good bookshop is just a genteel Black Hole that knows how to read."]]
+ >>> chrf = datasets.load_metric("chrf")
+ >>> results = chrf.compute(predictions=prediction,
+ ... references=reference,
+ ... word_order=2)
+ >>> print(results)
+ {'score': 82.87263732906315, 'char_order': 6, 'word_order': 2, 'beta': 2}
+
+ Example 3--the same chrF++ example as above, but with `lowercase=True` to normalize all case:
+ >>> prediction = ["The relationship between cats and dogs is not exactly friendly.", "a good bookshop is just a genteel black hole that knows how to read."]
+ >>> reference = [["The relationship between dogs and cats is not exactly friendly."], ["A good bookshop is just a genteel Black Hole that knows how to read."]]
+ >>> chrf = datasets.load_metric("chrf")
+ >>> results = chrf.compute(predictions=prediction,
+ ... references=reference,
+ ... word_order=2,
+ ... lowercase=True)
+ >>> print(results)
+ {'score': 92.12853119829202, 'char_order': 6, 'word_order': 2, 'beta': 2}
+"""
+
+
+@datasets.utils.file_utils.add_start_docstrings(_DESCRIPTION, _KWARGS_DESCRIPTION)
+class ChrF(datasets.Metric):
+ def _info(self):
+ if version.parse(scb.__version__) < version.parse("1.4.12"):
+ raise ImportWarning(
+ "To use `sacrebleu`, the module `sacrebleu>=1.4.12` is required, and the current version of `sacrebleu` doesn't match this condition.\n"
+ 'You can install it with `pip install "sacrebleu>=1.4.12"`.'
+ )
+ return datasets.MetricInfo(
+ description=_DESCRIPTION,
+ citation=_CITATION,
+ homepage="https://github.com/mjpost/sacreBLEU#chrf--chrf",
+ inputs_description=_KWARGS_DESCRIPTION,
+ features=datasets.Features(
+ {
+ "predictions": datasets.Value("string", id="sequence"),
+ "references": datasets.Sequence(datasets.Value("string", id="sequence"), id="references"),
+ }
+ ),
+ codebase_urls=["https://github.com/mjpost/sacreBLEU#chrf--chrf"],
+ reference_urls=[
+ "https://github.com/m-popovic/chrF",
+ ],
+ )
+
+ def _compute(
+ self,
+ predictions,
+ references,
+ char_order: int = CHRF.CHAR_ORDER,
+ word_order: int = CHRF.WORD_ORDER,
+ beta: int = CHRF.BETA,
+ lowercase: bool = False,
+ whitespace: bool = False,
+ eps_smoothing: bool = False,
+ ):
+ references_per_prediction = len(references[0])
+ if any(len(refs) != references_per_prediction for refs in references):
+ raise ValueError("Sacrebleu requires the same number of references for each prediction")
+ transformed_references = [[refs[i] for refs in references] for i in range(references_per_prediction)]
+
+ sb_chrf = CHRF(char_order, word_order, beta, lowercase, whitespace, eps_smoothing)
+ output = sb_chrf.corpus_score(predictions, transformed_references)
+
+ return {
+ "score": output.score,
+ "char_order": output.char_order,
+ "word_order": output.word_order,
+ "beta": output.beta,
+ }
diff --git a/metrics/code_eval/README.md b/metrics/code_eval/README.md
new file mode 100644
index 0000000..01c7659
--- /dev/null
+++ b/metrics/code_eval/README.md
@@ -0,0 +1,128 @@
+# Metric Card for Code Eval
+
+## Metric description
+
+The CodeEval metric estimates the pass@k metric for code synthesis.
+
+It implements the evaluation harness for the HumanEval problem solving dataset described in the paper ["Evaluating Large Language Models Trained on Code"](https://arxiv.org/abs/2107.03374).
+
+
+## How to use
+
+The Code Eval metric calculates how good are predictions given a set of references. Its arguments are:
+
+`predictions`: a list of candidates to evaluate. Each candidate should be a list of strings with several code candidates to solve the problem.
+
+`references`: a list with a test for each prediction. Each test should evaluate the correctness of a code candidate.
+
+`k`: number of code candidates to consider in the evaluation. The default value is `[1, 10, 100]`.
+
+`num_workers`: the number of workers used to evaluate the candidate programs (The default value is `4`).
+
+`timeout`: The maximum time taken to produce a prediction before it is considered a "timeout". The default value is `3.0` (i.e. 3 seconds).
+
+```python
+from datasets import load_metric
+code_eval = load_metric("code_eval")
+test_cases = ["assert add(2,3)==5"]
+candidates = [["def add(a,b): return a*b", "def add(a, b): return a+b"]]
+pass_at_k, results = code_eval.compute(references=test_cases, predictions=candidates, k=[1, 2])
+```
+
+N.B.
+This metric exists to run untrusted model-generated code. Users are strongly encouraged not to do so outside of a robust security sandbox. Before running this metric and once you've taken the necessary precautions, you will need to set the `HF_ALLOW_CODE_EVAL` environment variable. Use it at your own risk:
+```python
+import os
+os.environ["HF_ALLOW_CODE_EVAL"] = "1"`
+```
+
+## Output values
+
+The Code Eval metric outputs two things:
+
+`pass_at_k`: a dictionary with the pass rates for each k value defined in the arguments.
+
+`results`: a dictionary with granular results of each unit test.
+
+### Values from popular papers
+The [original CODEX paper](https://arxiv.org/pdf/2107.03374.pdf) reported that the CODEX-12B model had a pass@k score of 28.8% at `k=1`, 46.8% at `k=10` and 72.3% at `k=100`. However, since the CODEX model is not open source, it is hard to verify these numbers.
+
+
+
+## Examples
+
+Full match at `k=1`:
+
+```python
+from datasets import load_metric
+code_eval = load_metric("code_eval")
+test_cases = ["assert add(2,3)==5"]
+candidates = [["def add(a, b): return a+b"]]
+pass_at_k, results = code_eval.compute(references=test_cases, predictions=candidates, k=[1])
+print(pass_at_k)
+{'pass@1': 1.0}
+```
+
+No match for k = 1:
+
+```python
+from datasets import load_metric
+code_eval = load_metric("code_eval")
+test_cases = ["assert add(2,3)==5"]
+candidates = [["def add(a,b): return a*b"]]
+pass_at_k, results = code_eval.compute(references=test_cases, predictions=candidates, k=[1])
+print(pass_at_k)
+{'pass@1': 0.0}
+```
+
+Partial match at k=1, full match at k=2:
+
+```python
+from datasets import load_metric
+code_eval = load_metric("code_eval")
+test_cases = ["assert add(2,3)==5"]
+candidates = [["def add(a, b): return a+b", "def add(a,b): return a*b"]]
+pass_at_k, results = code_eval.compute(references=test_cases, predictions=candidates, k=[1, 2])
+print(pass_at_k)
+{'pass@1': 0.5, 'pass@2': 1.0}
+```
+
+## Limitations and bias
+
+As per the warning included in the metric code itself:
+> This program exists to execute untrusted model-generated code. Although it is highly unlikely that model-generated code will do something overtly malicious in response to this test suite, model-generated code may act destructively due to a lack of model capability or alignment. Users are strongly encouraged to sandbox this evaluation suite so that it does not perform destructive actions on their host or network. For more information on how OpenAI sandboxes its code, see the accompanying paper. Once you have read this disclaimer and taken appropriate precautions, uncomment the following line and proceed at your own risk:
+
+More information about the limitations of the code can be found on the [Human Eval Github repository](https://github.com/openai/human-eval).
+
+## Citation
+
+```bibtex
+@misc{chen2021evaluating,
+ title={Evaluating Large Language Models Trained on Code},
+ author={Mark Chen and Jerry Tworek and Heewoo Jun and Qiming Yuan \
+and Henrique Ponde de Oliveira Pinto and Jared Kaplan and Harri Edwards \
+and Yuri Burda and Nicholas Joseph and Greg Brockman and Alex Ray \
+and Raul Puri and Gretchen Krueger and Michael Petrov and Heidy Khlaaf \
+and Girish Sastry and Pamela Mishkin and Brooke Chan and Scott Gray \
+and Nick Ryder and Mikhail Pavlov and Alethea Power and Lukasz Kaiser \
+and Mohammad Bavarian and Clemens Winter and Philippe Tillet \
+and Felipe Petroski Such and Dave Cummings and Matthias Plappert \
+and Fotios Chantzis and Elizabeth Barnes and Ariel Herbert-Voss \
+and William Hebgen Guss and Alex Nichol and Alex Paino and Nikolas Tezak \
+and Jie Tang and Igor Babuschkin and Suchir Balaji and Shantanu Jain \
+and William Saunders and Christopher Hesse and Andrew N. Carr \
+and Jan Leike and Josh Achiam and Vedant Misra and Evan Morikawa \
+and Alec Radford and Matthew Knight and Miles Brundage and Mira Murati \
+and Katie Mayer and Peter Welinder and Bob McGrew and Dario Amodei \
+and Sam McCandlish and Ilya Sutskever and Wojciech Zaremba},
+ year={2021},
+ eprint={2107.03374},
+ archivePrefix={arXiv},
+ primaryClass={cs.LG}
+}
+```
+
+## Further References
+
+- [Human Eval Github repository](https://github.com/openai/human-eval)
+- [OpenAI Codex website](https://openai.com/blog/openai-codex/)
diff --git a/metrics/code_eval/code_eval.py b/metrics/code_eval/code_eval.py
new file mode 100644
index 0000000..444685a
--- /dev/null
+++ b/metrics/code_eval/code_eval.py
@@ -0,0 +1,212 @@
+# Copyright 2020 The HuggingFace Datasets Authors and the current dataset script contributor.
+#
+# Licensed under the Apache License, Version 2.0 (the "License");
+# you may not use this file except in compliance with the License.
+# You may obtain a copy of the License at
+#
+# http://www.apache.org/licenses/LICENSE-2.0
+#
+# Unless required by applicable law or agreed to in writing, software
+# distributed under the License is distributed on an "AS IS" BASIS,
+# WITHOUT WARRANTIES OR CONDITIONS OF ANY KIND, either express or implied.
+# See the License for the specific language governing permissions and
+# limitations under the License.
+"""The CodeEval metric estimates the pass@k metric for code synthesis.
+This is an evaluation harness for the HumanEval problem solving dataset
+described in the paper "Evaluating Large Language Models Trained on Code"
+(https://arxiv.org/abs/2107.03374)."""
+
+import itertools
+import os
+from collections import Counter, defaultdict
+from concurrent.futures import ThreadPoolExecutor, as_completed
+
+import numpy as np
+
+import datasets
+
+from .execute import check_correctness
+
+
+_CITATION = """\
+@misc{chen2021evaluating,
+ title={Evaluating Large Language Models Trained on Code},
+ author={Mark Chen and Jerry Tworek and Heewoo Jun and Qiming Yuan \
+and Henrique Ponde de Oliveira Pinto and Jared Kaplan and Harri Edwards \
+and Yuri Burda and Nicholas Joseph and Greg Brockman and Alex Ray \
+and Raul Puri and Gretchen Krueger and Michael Petrov and Heidy Khlaaf \
+and Girish Sastry and Pamela Mishkin and Brooke Chan and Scott Gray \
+and Nick Ryder and Mikhail Pavlov and Alethea Power and Lukasz Kaiser \
+and Mohammad Bavarian and Clemens Winter and Philippe Tillet \
+and Felipe Petroski Such and Dave Cummings and Matthias Plappert \
+and Fotios Chantzis and Elizabeth Barnes and Ariel Herbert-Voss \
+and William Hebgen Guss and Alex Nichol and Alex Paino and Nikolas Tezak \
+and Jie Tang and Igor Babuschkin and Suchir Balaji and Shantanu Jain \
+and William Saunders and Christopher Hesse and Andrew N. Carr \
+and Jan Leike and Josh Achiam and Vedant Misra and Evan Morikawa \
+and Alec Radford and Matthew Knight and Miles Brundage and Mira Murati \
+and Katie Mayer and Peter Welinder and Bob McGrew and Dario Amodei \
+and Sam McCandlish and Ilya Sutskever and Wojciech Zaremba},
+ year={2021},
+ eprint={2107.03374},
+ archivePrefix={arXiv},
+ primaryClass={cs.LG}
+}
+"""
+
+_DESCRIPTION = """\
+This metric implements the evaluation harness for the HumanEval problem solving dataset
+described in the paper "Evaluating Large Language Models Trained on Code"
+(https://arxiv.org/abs/2107.03374).
+"""
+
+
+_KWARGS_DESCRIPTION = """
+Calculates how good are predictions given some references, using certain scores
+Args:
+ predictions: list of candidates to evaluate. Each candidates should be a list
+ of strings with several code candidates to solve the problem.
+ references: a list with a test for each prediction. Each test should evaluate the
+ correctness of a code candidate.
+ k: number of code candidates to consider in the evaluation (Default: [1, 10, 100])
+ num_workers: number of workers used to evaluate the canidate programs (Default: 4).
+ timeout:
+Returns:
+ pass_at_k: dict with pass rates for each k
+ results: dict with granular results of each unittest
+Examples:
+ >>> code_eval = datasets.load_metric("code_eval")
+ >>> test_cases = ["assert add(2,3)==5"]
+ >>> candidates = [["def add(a,b): return a*b", "def add(a, b): return a+b"]]
+ >>> pass_at_k, results = code_eval.compute(references=test_cases, predictions=candidates, k=[1, 2])
+ >>> print(pass_at_k)
+ {'pass@1': 0.5, 'pass@2': 1.0}
+"""
+
+
+_WARNING = """
+################################################################################
+ !!!WARNING!!!
+################################################################################
+The "code_eval" metric executes untrusted model-generated code in Python.
+Although it is highly unlikely that model-generated code will do something
+overtly malicious in response to this test suite, model-generated code may act
+destructively due to a lack of model capability or alignment.
+Users are strongly encouraged to sandbox this evaluation suite so that it
+does not perform destructive actions on their host or network. For more
+information on how OpenAI sandboxes its code, see the paper "Evaluating Large
+Language Models Trained on Code" (https://arxiv.org/abs/2107.03374).
+
+Once you have read this disclaimer and taken appropriate precautions,
+set the environment variable HF_ALLOW_CODE_EVAL="1". Within Python you can to this
+with:
+
+>>> import os
+>>> os.environ["HF_ALLOW_CODE_EVAL"] = "1"
+
+################################################################################\
+"""
+
+_LICENSE = """The MIT License
+
+Copyright (c) OpenAI (https://openai.com)
+
+Permission is hereby granted, free of charge, to any person obtaining a copy
+of this software and associated documentation files (the "Software"), to deal
+in the Software without restriction, including without limitation the rights
+to use, copy, modify, merge, publish, distribute, sublicense, and/or sell
+copies of the Software, and to permit persons to whom the Software is
+furnished to do so, subject to the following conditions:
+
+The above copyright notice and this permission notice shall be included in
+all copies or substantial portions of the Software.
+
+THE SOFTWARE IS PROVIDED "AS IS", WITHOUT WARRANTY OF ANY KIND, EXPRESS OR
+IMPLIED, INCLUDING BUT NOT LIMITED TO THE WARRANTIES OF MERCHANTABILITY,
+FITNESS FOR A PARTICULAR PURPOSE AND NONINFRINGEMENT. IN NO EVENT SHALL THE
+AUTHORS OR COPYRIGHT HOLDERS BE LIABLE FOR ANY CLAIM, DAMAGES OR OTHER
+LIABILITY, WHETHER IN AN ACTION OF CONTRACT, TORT OR OTHERWISE, ARISING FROM,
+OUT OF OR IN CONNECTION WITH THE SOFTWARE OR THE USE OR OTHER DEALINGS IN
+THE SOFTWARE."""
+
+
+@datasets.utils.file_utils.add_start_docstrings(_DESCRIPTION, _KWARGS_DESCRIPTION)
+class CodeEval(datasets.Metric):
+ def _info(self):
+ return datasets.MetricInfo(
+ # This is the description that will appear on the metrics page.
+ description=_DESCRIPTION,
+ citation=_CITATION,
+ inputs_description=_KWARGS_DESCRIPTION,
+ # This defines the format of each prediction and reference
+ features=datasets.Features(
+ {
+ "predictions": datasets.Sequence(datasets.Value("string")),
+ "references": datasets.Value("string"),
+ }
+ ),
+ homepage="https://github.com/openai/human-eval",
+ codebase_urls=["https://github.com/openai/human-eval"],
+ reference_urls=["https://github.com/openai/human-eval"],
+ license=_LICENSE,
+ )
+
+ def _compute(self, predictions, references, k=[1, 10, 100], num_workers=4, timeout=3.0):
+ """Returns the scores"""
+
+ if os.getenv("HF_ALLOW_CODE_EVAL", 0) != "1":
+ raise ValueError(_WARNING)
+
+ if os.name == "nt":
+ raise NotImplementedError("This metric is currently not supported on Windows.")
+
+ with ThreadPoolExecutor(max_workers=num_workers) as executor:
+ futures = []
+ completion_id = Counter()
+ n_samples = 0
+ results = defaultdict(list)
+
+ for task_id, (candidates, test_case) in enumerate(zip(predictions, references)):
+ for candidate in candidates:
+ test_program = candidate + "\n" + test_case
+ args = (test_program, timeout, task_id, completion_id[task_id])
+ future = executor.submit(check_correctness, *args)
+ futures.append(future)
+ completion_id[task_id] += 1
+ n_samples += 1
+
+ for future in as_completed(futures):
+ result = future.result()
+ results[result["task_id"]].append((result["completion_id"], result))
+
+ total, correct = [], []
+ for result in results.values():
+ result.sort()
+ passed = [r[1]["passed"] for r in result]
+ total.append(len(passed))
+ correct.append(sum(passed))
+ total = np.array(total)
+ correct = np.array(correct)
+
+ ks = k
+ pass_at_k = {f"pass@{k}": estimate_pass_at_k(total, correct, k).mean() for k in ks if (total >= k).all()}
+
+ return pass_at_k, results
+
+
+def estimate_pass_at_k(num_samples, num_correct, k):
+ """Estimates pass@k of each problem and returns them in an array."""
+
+ def estimator(n: int, c: int, k: int) -> float:
+ """Calculates 1 - comb(n - c, k) / comb(n, k)."""
+ if n - c < k:
+ return 1.0
+ return 1.0 - np.prod(1.0 - k / np.arange(n - c + 1, n + 1))
+
+ if isinstance(num_samples, int):
+ num_samples_it = itertools.repeat(num_samples, len(num_correct))
+ else:
+ assert len(num_samples) == len(num_correct)
+ num_samples_it = iter(num_samples)
+
+ return np.array([estimator(int(n), int(c), k) for n, c in zip(num_samples_it, num_correct)])
diff --git a/metrics/code_eval/execute.py b/metrics/code_eval/execute.py
new file mode 100644
index 0000000..53517a8
--- /dev/null
+++ b/metrics/code_eval/execute.py
@@ -0,0 +1,236 @@
+# Copyright 2020 The HuggingFace Datasets Authors and the current dataset script contributor.
+#
+# Licensed under the Apache License, Version 2.0 (the "License");
+# you may not use this file except in compliance with the License.
+# You may obtain a copy of the License at
+#
+# http://www.apache.org/licenses/LICENSE-2.0
+#
+# Unless required by applicable law or agreed to in writing, software
+# distributed under the License is distributed on an "AS IS" BASIS,
+# WITHOUT WARRANTIES OR CONDITIONS OF ANY KIND, either express or implied.
+# See the License for the specific language governing permissions and
+# limitations under the License.
+
+# This code is adapted from OpenAI's release
+# https://github.com/openai/human-eval/blob/master/human_eval/execution.py
+
+import contextlib
+import faulthandler
+import io
+import multiprocessing
+import os
+import platform
+import signal
+import tempfile
+
+
+def check_correctness(check_program, timeout, task_id, completion_id):
+ """
+ Evaluates the functional correctness of a completion by running the test
+ suite provided in the problem.
+
+ :param completion_id: an optional completion ID so we can match
+ the results later even if execution finishes asynchronously.
+ """
+ manager = multiprocessing.Manager()
+ result = manager.list()
+
+ p = multiprocessing.Process(target=unsafe_execute, args=(check_program, result, timeout))
+ p.start()
+ p.join(timeout=timeout + 1)
+ if p.is_alive():
+ p.kill()
+
+ if not result:
+ result.append("timed out")
+
+ return dict(
+ task_id=task_id,
+ passed=result[0] == "passed",
+ result=result[0],
+ completion_id=completion_id,
+ )
+
+
+def unsafe_execute(check_program, result, timeout):
+
+ with create_tempdir():
+
+ # These system calls are needed when cleaning up tempdir.
+ import os
+ import shutil
+
+ rmtree = shutil.rmtree
+ rmdir = os.rmdir
+ chdir = os.chdir
+
+ # Disable functionalities that can make destructive changes to the test.
+ reliability_guard()
+
+ # Run program.
+ try:
+ exec_globals = {}
+ with swallow_io():
+ with time_limit(timeout):
+ exec(check_program, exec_globals)
+ result.append("passed")
+ except TimeoutException:
+ result.append("timed out")
+ except BaseException as e:
+ result.append(f"failed: {e}")
+
+ # Needed for cleaning up.
+ shutil.rmtree = rmtree
+ os.rmdir = rmdir
+ os.chdir = chdir
+
+
+@contextlib.contextmanager
+def time_limit(seconds):
+ def signal_handler(signum, frame):
+ raise TimeoutException("Timed out!")
+
+ signal.setitimer(signal.ITIMER_REAL, seconds)
+ signal.signal(signal.SIGALRM, signal_handler)
+ try:
+ yield
+ finally:
+ signal.setitimer(signal.ITIMER_REAL, 0)
+
+
+@contextlib.contextmanager
+def swallow_io():
+ stream = WriteOnlyStringIO()
+ with contextlib.redirect_stdout(stream):
+ with contextlib.redirect_stderr(stream):
+ with redirect_stdin(stream):
+ yield
+
+
+@contextlib.contextmanager
+def create_tempdir():
+ with tempfile.TemporaryDirectory() as dirname:
+ with chdir(dirname):
+ yield dirname
+
+
+class TimeoutException(Exception):
+ pass
+
+
+class WriteOnlyStringIO(io.StringIO):
+ """StringIO that throws an exception when it's read from"""
+
+ def read(self, *args, **kwargs):
+ raise OSError
+
+ def readline(self, *args, **kwargs):
+ raise OSError
+
+ def readlines(self, *args, **kwargs):
+ raise OSError
+
+ def readable(self, *args, **kwargs):
+ """Returns True if the IO object can be read."""
+ return False
+
+
+class redirect_stdin(contextlib._RedirectStream): # type: ignore
+ _stream = "stdin"
+
+
+@contextlib.contextmanager
+def chdir(root):
+ if root == ".":
+ yield
+ return
+ cwd = os.getcwd()
+ os.chdir(root)
+ try:
+ yield
+ except BaseException as exc:
+ raise exc
+ finally:
+ os.chdir(cwd)
+
+
+def reliability_guard(maximum_memory_bytes=None):
+ """
+ This disables various destructive functions and prevents the generated code
+ from interfering with the test (e.g. fork bomb, killing other processes,
+ removing filesystem files, etc.)
+
+ WARNING
+ This function is NOT a security sandbox. Untrusted code, including, model-
+ generated code, should not be blindly executed outside of one. See the
+ Codex paper for more information about OpenAI's code sandbox, and proceed
+ with caution.
+ """
+
+ if maximum_memory_bytes is not None:
+ import resource
+
+ resource.setrlimit(resource.RLIMIT_AS, (maximum_memory_bytes, maximum_memory_bytes))
+ resource.setrlimit(resource.RLIMIT_DATA, (maximum_memory_bytes, maximum_memory_bytes))
+ if not platform.uname().system == "Darwin":
+ resource.setrlimit(resource.RLIMIT_STACK, (maximum_memory_bytes, maximum_memory_bytes))
+
+ faulthandler.disable()
+
+ import builtins
+
+ builtins.exit = None
+ builtins.quit = None
+
+ import os
+
+ os.environ["OMP_NUM_THREADS"] = "1"
+
+ os.kill = None
+ os.system = None
+ os.putenv = None
+ os.remove = None
+ os.removedirs = None
+ os.rmdir = None
+ os.fchdir = None
+ os.setuid = None
+ os.fork = None
+ os.forkpty = None
+ os.killpg = None
+ os.rename = None
+ os.renames = None
+ os.truncate = None
+ os.replace = None
+ os.unlink = None
+ os.fchmod = None
+ os.fchown = None
+ os.chmod = None
+ os.chown = None
+ os.chroot = None
+ os.fchdir = None
+ os.lchflags = None
+ os.lchmod = None
+ os.lchown = None
+ os.getcwd = None
+ os.chdir = None
+
+ import shutil
+
+ shutil.rmtree = None
+ shutil.move = None
+ shutil.chown = None
+
+ import subprocess
+
+ subprocess.Popen = None # type: ignore
+
+ __builtins__["help"] = None
+
+ import sys
+
+ sys.modules["ipdb"] = None
+ sys.modules["joblib"] = None
+ sys.modules["resource"] = None
+ sys.modules["psutil"] = None
+ sys.modules["tkinter"] = None
diff --git a/metrics/comet/README.md b/metrics/comet/README.md
new file mode 100644
index 0000000..fb6b24e
--- /dev/null
+++ b/metrics/comet/README.md
@@ -0,0 +1,128 @@
+# Metric Card for COMET
+
+## Metric description
+
+Crosslingual Optimized Metric for Evaluation of Translation (COMET) is an open-source framework used to train Machine Translation metrics that achieve high levels of correlation with different types of human judgments.
+
+## How to use
+
+COMET takes 3 lists of strings as input: `sources` (a list of source sentences), `predictions` (a list of candidate translations) and `references` (a list of reference translations).
+
+```python
+from datasets import load_metric
+comet_metric = load_metric('comet')
+source = ["Dem Feuer konnte Einhalt geboten werden", "Schulen und Kindergärten wurden eröffnet."]
+hypothesis = ["The fire could be stopped", "Schools and kindergartens were open"]
+reference = ["They were able to control the fire.", "Schools and kindergartens opened"]
+comet_score = comet_metric.compute(predictions=hypothesis, references=reference, sources=source)
+```
+
+It has several configurations, named after the COMET model to be used. It will default to `wmt20-comet-da` (previously known as `wmt-large-da-estimator-1719`). Alternate models that can be chosen include `wmt20-comet-qe-da`, `wmt21-comet-mqm`, `wmt21-cometinho-da`, `wmt21-comet-qe-mqm` and `emnlp20-comet-rank`.
+
+It also has several optional arguments:
+
+`gpus`: optional, an integer (number of GPUs to train on) or a list of integers (which GPUs to train on). Set to 0 to use CPU. The default value is `None` (uses one GPU if possible, else use CPU).
+
+`progress_bar`a boolean -- if set to `True`, progress updates will be printed out. The default value is `False`.
+
+More information about model characteristics can be found on the [COMET website](https://unbabel.github.io/COMET/html/models.html).
+
+## Output values
+
+The COMET metric outputs two lists:
+
+`scores`: a list of COMET scores for each of the input sentences, ranging from 0-1.
+
+`mean_score`: the mean value of COMET scores `scores` over all the input sentences, ranging from 0-1.
+
+### Values from popular papers
+
+The [original COMET paper](https://arxiv.org/pdf/2009.09025.pdf) reported average COMET scores ranging from 0.4 to 0.6, depending on the language pairs used for evaluating translation models. They also illustrate that COMET correlates well with human judgements compared to other metrics such as [BLEU](https://huggingface.co/metrics/bleu) and [CHRF](https://huggingface.co/metrics/chrf).
+
+## Examples
+
+Full match:
+
+```python
+from datasets import load_metric
+comet_metric = load_metric('comet')
+source = ["Dem Feuer konnte Einhalt geboten werden", "Schulen und Kindergärten wurden eröffnet."]
+hypothesis = ["They were able to control the fire.", "Schools and kindergartens opened"]
+reference = ["They were able to control the fire.", "Schools and kindergartens opened"]
+results = comet_metric.compute(predictions=hypothesis, references=reference, sources=source)
+print([round(v, 1) for v in results["scores"]])
+[1.0, 1.0]
+```
+
+Partial match:
+
+```python
+from datasets import load_metric
+comet_metric = load_metric('comet')
+source = ["Dem Feuer konnte Einhalt geboten werden", "Schulen und Kindergärten wurden eröffnet."]
+hypothesis = ["The fire could be stopped", "Schools and kindergartens were open"]
+reference = ["They were able to control the fire", "Schools and kindergartens opened"]
+results = comet_metric.compute(predictions=hypothesis, references=reference, sources=source)
+print([round(v, 2) for v in results["scores"]])
+[0.19, 0.92]
+```
+
+No match:
+
+```python
+from datasets import load_metric
+comet_metric = load_metric('comet')
+source = ["Dem Feuer konnte Einhalt geboten werden", "Schulen und Kindergärten wurden eröffnet."]
+hypothesis = ["The girl went for a walk", "The boy was sleeping"]
+reference = ["They were able to control the fire", "Schools and kindergartens opened"]
+results = comet_metric.compute(predictions=hypothesis, references=reference, sources=source)
+print([round(v, 2) for v in results["scores"]])
+[0.00, 0.00]
+```
+
+## Limitations and bias
+
+The models provided for calculating the COMET metric are built on top of XLM-R and cover the following languages:
+
+Afrikaans, Albanian, Amharic, Arabic, Armenian, Assamese, Azerbaijani, Basque, Belarusian, Bengali, Bengali Romanized, Bosnian, Breton, Bulgarian, Burmese, Burmese, Catalan, Chinese (Simplified), Chinese (Traditional), Croatian, Czech, Danish, Dutch, English, Esperanto, Estonian, Filipino, Finnish, French, Galician, Georgian, German, Greek, Gujarati, Hausa, Hebrew, Hindi, Hindi Romanized, Hungarian, Icelandic, Indonesian, Irish, Italian, Japanese, Javanese, Kannada, Kazakh, Khmer, Korean, Kurdish (Kurmanji), Kyrgyz, Lao, Latin, Latvian, Lithuanian, Macedonian, Malagasy, Malay, Malayalam, Marathi, Mongolian, Nepali, Norwegian, Oriya, Oromo, Pashto, Persian, Polish, Portuguese, Punjabi, Romanian, Russian, Sanskri, Scottish, Gaelic, Serbian, Sindhi, Sinhala, Slovak, Slovenian, Somali, Spanish, Sundanese, Swahili, Swedish, Tamil, Tamil Romanized, Telugu, Telugu Romanized, Thai, Turkish, Ukrainian, Urdu, Urdu Romanized, Uyghur, Uzbek, Vietnamese, Welsh, Western, Frisian, Xhosa, Yiddish.
+
+Thus, results for language pairs containing uncovered languages are unreliable, as per the [COMET website](https://github.com/Unbabel/COMET)
+
+Also, calculating the COMET metric involves downloading the model from which features are obtained -- the default model, `wmt20-comet-da`, takes over 1.79GB of storage space and downloading it can take a significant amount of time depending on the speed of your internet connection. If this is an issue, choose a smaller model; for instance `wmt21-cometinho-da` is 344MB.
+
+## Citation
+
+```bibtex
+@inproceedings{rei-EtAl:2020:WMT,
+ author = {Rei, Ricardo and Stewart, Craig and Farinha, Ana C and Lavie, Alon},
+ title = {Unbabel's Participation in the WMT20 Metrics Shared Task},
+ booktitle = {Proceedings of the Fifth Conference on Machine Translation},
+ month = {November},
+ year = {2020},
+ address = {Online},
+ publisher = {Association for Computational Linguistics},
+ pages = {909--918},
+}
+```
+
+```bibtex
+@inproceedings{rei-etal-2020-comet,
+ title = "{COMET}: A Neural Framework for {MT} Evaluation",
+ author = "Rei, Ricardo and
+ Stewart, Craig and
+ Farinha, Ana C and
+ Lavie, Alon",
+ booktitle = "Proceedings of the 2020 Conference on Empirical Methods in Natural Language Processing (EMNLP)",
+ month = nov,
+ year = "2020",
+ address = "Online",
+ publisher = "Association for Computational Linguistics",
+ url = "https://www.aclweb.org/anthology/2020.emnlp-main.213",
+ pages = "2685--2702",
+
+```
+
+## Further References
+
+- [COMET website](https://unbabel.github.io/COMET/html/index.html)
+- [Hugging Face Tasks - Machine Translation](https://huggingface.co/tasks/translation)
diff --git a/metrics/comet/comet.py b/metrics/comet/comet.py
new file mode 100644
index 0000000..585bb30
--- /dev/null
+++ b/metrics/comet/comet.py
@@ -0,0 +1,144 @@
+# Copyright 2020 The HuggingFace Datasets Authors.
+#
+# Licensed under the Apache License, Version 2.0 (the "License");
+# you may not use this file except in compliance with the License.
+# You may obtain a copy of the License at
+#
+# http://www.apache.org/licenses/LICENSE-2.0
+#
+# Unless required by applicable law or agreed to in writing, software
+# distributed under the License is distributed on an "AS IS" BASIS,
+# WITHOUT WARRANTIES OR CONDITIONS OF ANY KIND, either express or implied.
+# See the License for the specific language governing permissions and
+# limitations under the License.
+""" COMET metric.
+
+Requirements:
+pip install unbabel-comet
+
+Usage:
+
+```python
+from datasets import load_metric
+comet_metric = load_metric('metrics/comet/comet.py')
+#comet_metric = load_metric('comet')
+#comet_metric = load_metric('comet', 'wmt-large-hter-estimator')
+
+
+source = ["Dem Feuer konnte Einhalt geboten werden", "Schulen und Kindergärten wurden eröffnet."]
+hypothesis = ["The fire could be stopped", "Schools and kindergartens were open"]
+reference = ["They were able to control the fire.", "Schools and kindergartens opened"]
+
+predictions = comet_metric.compute(predictions=hypothesis, references=reference, sources=source)
+predictions['scores']
+```
+"""
+
+import comet # From: unbabel-comet
+import torch
+
+import datasets
+
+
+logger = datasets.logging.get_logger(__name__)
+
+_CITATION = """\
+@inproceedings{rei-EtAl:2020:WMT,
+ author = {Rei, Ricardo and Stewart, Craig and Farinha, Ana C and Lavie, Alon},
+ title = {Unbabel's Participation in the WMT20 Metrics Shared Task},
+ booktitle = {Proceedings of the Fifth Conference on Machine Translation},
+ month = {November},
+ year = {2020},
+ address = {Online},
+ publisher = {Association for Computational Linguistics},
+ pages = {909--918},
+}
+@inproceedings{rei-etal-2020-comet,
+ title = "{COMET}: A Neural Framework for {MT} Evaluation",
+ author = "Rei, Ricardo and
+ Stewart, Craig and
+ Farinha, Ana C and
+ Lavie, Alon",
+ booktitle = "Proceedings of the 2020 Conference on Empirical Methods in Natural Language Processing (EMNLP)",
+ month = nov,
+ year = "2020",
+ address = "Online",
+ publisher = "Association for Computational Linguistics",
+ url = "https://www.aclweb.org/anthology/2020.emnlp-main.213",
+ pages = "2685--2702",
+}
+"""
+
+_DESCRIPTION = """\
+Crosslingual Optimized Metric for Evaluation of Translation (COMET) is an open-source framework used to train Machine Translation metrics that achieve high levels of correlation with different types of human judgments (HTER, DA's or MQM).
+With the release of the framework the authors also released fully trained models that were used to compete in the WMT20 Metrics Shared Task achieving SOTA in that years competition.
+
+See the [README.md] file at https://unbabel.github.io/COMET/html/models.html for more information.
+"""
+
+_KWARGS_DESCRIPTION = """
+COMET score.
+
+Args:
+
+`sources` (list of str): Source sentences
+`predictions` (list of str): candidate translations
+`references` (list of str): reference translations
+`cuda` (bool): If set to True, runs COMET using GPU
+`show_progress` (bool): Shows progress
+`model`: COMET model to be used. Will default to `wmt-large-da-estimator-1719` if None.
+
+Returns:
+ `samples`: List of dictionaries with `src`, `mt`, `ref` and `score`.
+ `scores`: List of scores.
+
+Examples:
+
+ >>> comet_metric = datasets.load_metric('comet')
+ >>> # comet_metric = load_metric('comet', 'wmt20-comet-da') # you can also choose which model to use
+ >>> source = ["Dem Feuer konnte Einhalt geboten werden", "Schulen und Kindergärten wurden eröffnet."]
+ >>> hypothesis = ["The fire could be stopped", "Schools and kindergartens were open"]
+ >>> reference = ["They were able to control the fire.", "Schools and kindergartens opened"]
+ >>> results = comet_metric.compute(predictions=hypothesis, references=reference, sources=source)
+ >>> print([round(v, 2) for v in results["scores"]])
+ [0.19, 0.92]
+"""
+
+
+@datasets.utils.file_utils.add_start_docstrings(_DESCRIPTION, _KWARGS_DESCRIPTION)
+class COMET(datasets.Metric):
+ def _info(self):
+
+ return datasets.MetricInfo(
+ description=_DESCRIPTION,
+ citation=_CITATION,
+ homepage="https://unbabel.github.io/COMET/html/index.html",
+ inputs_description=_KWARGS_DESCRIPTION,
+ features=datasets.Features(
+ {
+ "sources": datasets.Value("string", id="sequence"),
+ "predictions": datasets.Value("string", id="sequence"),
+ "references": datasets.Value("string", id="sequence"),
+ }
+ ),
+ codebase_urls=["https://github.com/Unbabel/COMET"],
+ reference_urls=[
+ "https://github.com/Unbabel/COMET",
+ "https://www.aclweb.org/anthology/2020.emnlp-main.213/",
+ "http://www.statmt.org/wmt20/pdf/2020.wmt-1.101.pdf6",
+ ],
+ )
+
+ def _download_and_prepare(self, dl_manager):
+ if self.config_name == "default":
+ self.scorer = comet.load_from_checkpoint(comet.download_model("wmt20-comet-da"))
+ else:
+ self.scorer = comet.load_from_checkpoint(comet.download_model(self.config_name))
+
+ def _compute(self, sources, predictions, references, gpus=None, progress_bar=False):
+ if gpus is None:
+ gpus = 1 if torch.cuda.is_available() else 0
+ data = {"src": sources, "mt": predictions, "ref": references}
+ data = [dict(zip(data, t)) for t in zip(*data.values())]
+ scores, mean_score = self.scorer.predict(data, gpus=gpus, progress_bar=progress_bar)
+ return {"mean_score": mean_score, "scores": scores}
diff --git a/metrics/competition_math/README.md b/metrics/competition_math/README.md
new file mode 100644
index 0000000..a7d9893
--- /dev/null
+++ b/metrics/competition_math/README.md
@@ -0,0 +1,103 @@
+# Metric Card for Competition MATH
+
+## Metric description
+
+This metric is used to assess performance on the [Mathematics Aptitude Test of Heuristics (MATH) dataset](https://huggingface.co/datasets/competition_math).
+
+It first canonicalizes the inputs (e.g., converting `1/2` to `\\frac{1}{2}`) and then computes accuracy.
+
+## How to use
+
+This metric takes two arguments:
+
+`predictions`: a list of predictions to score. Each prediction is a string that contains natural language and LaTeX.
+
+`references`: list of reference for each prediction. Each reference is a string that contains natural language and LaTeX.
+
+
+```python
+>>> from datasets import load_metric
+>>> math = load_metric("competition_math")
+>>> references = ["\\frac{1}{2}"]
+>>> predictions = ["1/2"]
+>>> results = math.compute(references=references, predictions=predictions)
+```
+
+N.B. To be able to use Competition MATH, you need to install the `math_equivalence` dependency using `pip install git+https://github.com/hendrycks/math.git`.
+
+
+## Output values
+
+This metric returns a dictionary that contains the [accuracy](https://huggingface.co/metrics/accuracy) after canonicalizing inputs, on a scale between 0.0 and 1.0.
+
+### Values from popular papers
+The [original MATH dataset paper](https://arxiv.org/abs/2103.03874) reported accuracies ranging from 3.0% to 6.9% by different large language models.
+
+More recent progress on the dataset can be found on the [dataset leaderboard](https://paperswithcode.com/sota/math-word-problem-solving-on-math).
+
+## Examples
+
+Maximal values (full match):
+
+```python
+>>> from datasets import load_metric
+>>> math = load_metric("competition_math")
+>>> references = ["\\frac{1}{2}"]
+>>> predictions = ["1/2"]
+>>> results = math.compute(references=references, predictions=predictions)
+>>> print(results)
+{'accuracy': 1.0}
+```
+
+Minimal values (no match):
+
+```python
+>>> from datasets import load_metric
+>>> math = load_metric("competition_math")
+>>> references = ["\\frac{1}{2}"]
+>>> predictions = ["3/4"]
+>>> results = math.compute(references=references, predictions=predictions)
+>>> print(results)
+{'accuracy': 0.0}
+```
+
+Partial match:
+
+```python
+>>> from datasets import load_metric
+>>> math = load_metric("competition_math")
+>>> references = ["\\frac{1}{2}","\\frac{3}{4}"]
+>>> predictions = ["1/5", "3/4"]
+>>> results = math.compute(references=references, predictions=predictions)
+>>> print(results)
+{'accuracy': 0.5}
+```
+
+## Limitations and bias
+
+This metric is limited to datasets with the same format as the [Mathematics Aptitude Test of Heuristics (MATH) dataset](https://huggingface.co/datasets/competition_math), and is meant to evaluate the performance of large language models at solving mathematical problems.
+
+N.B. The MATH dataset also assigns levels of difficulty to different problems, so disagregating model performance by difficulty level (similarly to what was done in the [original paper](https://arxiv.org/abs/2103.03874) can give a better indication of how a given model does on a given difficulty of math problem, compared to overall accuracy.
+
+## Citation
+
+```bibtex
+@article{hendrycksmath2021,
+ title={Measuring Mathematical Problem Solving With the MATH Dataset},
+ author={Dan Hendrycks
+ and Collin Burns
+ and Saurav Kadavath
+ and Akul Arora
+ and Steven Basart
+ and Eric Tang
+ and Dawn Song
+ and Jacob Steinhardt},
+ journal={arXiv preprint arXiv:2103.03874},
+ year={2021}
+}
+```
+
+## Further References
+- [MATH dataset](https://huggingface.co/datasets/competition_math)
+- [MATH leaderboard](https://paperswithcode.com/sota/math-word-problem-solving-on-math)
+- [MATH paper](https://arxiv.org/abs/2103.03874)
diff --git a/metrics/competition_math/competition_math.py b/metrics/competition_math/competition_math.py
new file mode 100644
index 0000000..baca345
--- /dev/null
+++ b/metrics/competition_math/competition_math.py
@@ -0,0 +1,94 @@
+# Copyright 2020 The HuggingFace Datasets Authors and the current dataset script contributor.
+#
+# Licensed under the Apache License, Version 2.0 (the "License");
+# you may not use this file except in compliance with the License.
+# You may obtain a copy of the License at
+#
+# http://www.apache.org/licenses/LICENSE-2.0
+#
+# Unless required by applicable law or agreed to in writing, software
+# distributed under the License is distributed on an "AS IS" BASIS,
+# WITHOUT WARRANTIES OR CONDITIONS OF ANY KIND, either express or implied.
+# See the License for the specific language governing permissions and
+# limitations under the License.
+"""Accuracy metric for the Mathematics Aptitude Test of Heuristics (MATH) dataset."""
+
+import math_equivalence # From: git+https://github.com/hendrycks/math.git
+
+import datasets
+
+
+_CITATION = """\
+@article{hendrycksmath2021,
+ title={Measuring Mathematical Problem Solving With the MATH Dataset},
+ author={Dan Hendrycks
+ and Collin Burns
+ and Saurav Kadavath
+ and Akul Arora
+ and Steven Basart
+ and Eric Tang
+ and Dawn Song
+ and Jacob Steinhardt},
+ journal={arXiv preprint arXiv:2103.03874},
+ year={2021}
+}
+"""
+
+
+_DESCRIPTION = """\
+This metric is used to assess performance on the Mathematics Aptitude Test of Heuristics (MATH) dataset.
+It first canonicalizes the inputs (e.g., converting "1/2" to "\\frac{1}{2}") and then computes accuracy.
+"""
+
+
+_KWARGS_DESCRIPTION = r"""
+Calculates accuracy after canonicalizing inputs.
+
+Args:
+ predictions: list of predictions to score. Each prediction
+ is a string that contains natural language and LaTex.
+ references: list of reference for each prediction. Each
+ reference is a string that contains natural language
+ and LaTex.
+Returns:
+ accuracy: accuracy after canonicalizing inputs
+ (e.g., converting "1/2" to "\\frac{1}{2}")
+
+Examples:
+ >>> metric = datasets.load_metric("competition_math")
+ >>> results = metric.compute(references=["\\frac{1}{2}"], predictions=["1/2"])
+ >>> print(results)
+ {'accuracy': 1.0}
+"""
+
+
+@datasets.utils.file_utils.add_end_docstrings(_DESCRIPTION, _KWARGS_DESCRIPTION)
+class CompetitionMathMetric(datasets.Metric):
+ """Accuracy metric for the MATH dataset."""
+
+ def _info(self):
+ return datasets.MetricInfo(
+ description=_DESCRIPTION,
+ citation=_CITATION,
+ inputs_description=_KWARGS_DESCRIPTION,
+ features=datasets.Features(
+ {
+ "predictions": datasets.Value("string"),
+ "references": datasets.Value("string"),
+ }
+ ),
+ # Homepage of the metric for documentation
+ homepage="https://github.com/hendrycks/math",
+ # Additional links to the codebase or references
+ codebase_urls=["https://github.com/hendrycks/math"],
+ )
+
+ def _compute(self, predictions, references):
+ """Returns the scores"""
+ n_correct = 0.0
+ for i, j in zip(predictions, references):
+ n_correct += 1.0 if math_equivalence.is_equiv(i, j) else 0.0
+ accuracy = n_correct / len(predictions)
+ return {
+ "accuracy": accuracy,
+ }
diff --git a/metrics/coval/README.md b/metrics/coval/README.md
new file mode 100644
index 0000000..bd9fc0f
--- /dev/null
+++ b/metrics/coval/README.md
@@ -0,0 +1,170 @@
+# Metric Card for COVAL
+
+## Metric description
+
+CoVal is a coreference evaluation tool for the [CoNLL](https://huggingface.co/datasets/conll2003) and [ARRAU](https://catalog.ldc.upenn.edu/LDC2013T22) datasets which implements of the common evaluation metrics including MUC [Vilain et al, 1995](https://aclanthology.org/M95-1005.pdf), B-cubed [Bagga and Baldwin, 1998](https://citeseerx.ist.psu.edu/viewdoc/download?doi=10.1.1.34.2578&rep=rep1&type=pdf), CEAFe [Luo et al., 2005](https://aclanthology.org/H05-1004.pdf), LEA [Moosavi and Strube, 2016](https://aclanthology.org/P16-1060.pdf) and the averaged CoNLL score (the average of the F1 values of MUC, B-cubed and CEAFe).
+
+CoVal code was written by [`@ns-moosavi`](https://github.com/ns-moosavi), with some parts borrowed from [Deep Coref](https://github.com/clarkkev/deep-coref/blob/master/evaluation.py). The test suite is taken from the [official CoNLL code](https://github.com/conll/reference-coreference-scorers/), with additions by [`@andreasvc`](https://github.com/andreasvc) and file parsing developed by Leo Born.
+
+## How to use
+
+The metric takes two lists of sentences as input: one representing `predictions` and `references`, with the sentences consisting of words in the CoNLL format (see the [Limitations and bias](#Limitations-and-bias) section below for more details on the CoNLL format).
+
+```python
+from datasets import load_metric
+coval = load_metric('coval')
+words = ['bc/cctv/00/cctv_0005 0 0 Thank VBP (TOP(S(VP* thank 01 1 Xu_li * (V*) * -',
+... 'bc/cctv/00/cctv_0005 0 1 you PRP (NP*) - - - Xu_li * (ARG1*) (ARG0*) (116)',
+... 'bc/cctv/00/cctv_0005 0 2 everyone NN (NP*) - - - Xu_li * (ARGM-DIS*) * (116)',
+... 'bc/cctv/00/cctv_0005 0 3 for IN (PP* - - - Xu_li * (ARG2* * -',
+... 'bc/cctv/00/cctv_0005 0 4 watching VBG (S(VP*)))) watch 01 1 Xu_li * *) (V*) -',
+... 'bc/cctv/00/cctv_0005 0 5 . . *)) - - - Xu_li * * * -']
+references = [words]
+predictions = [words]
+results = coval.compute(predictions=predictions, references=references)
+```
+It also has several optional arguments:
+
+`keep_singletons`: After extracting all mentions of key or system file mentions whose corresponding coreference chain is of size one are considered as singletons. The default evaluation mode will include singletons in evaluations if they are included in the key or the system files. By setting `keep_singletons=False`, all singletons in the key and system files will be excluded from the evaluation.
+
+`NP_only`: Most of the recent coreference resolvers only resolve NP mentions and leave out the resolution of VPs. By setting the `NP_only` option, the scorer will only evaluate the resolution of NPs.
+
+`min_span`: By setting `min_span`, the scorer reports the results based on automatically detected minimum spans. Minimum spans are determined using the [MINA algorithm](https://arxiv.org/pdf/1906.06703.pdf).
+
+
+## Output values
+
+The metric outputs a dictionary with the following key-value pairs:
+
+`mentions`: number of mentions, ranges from 0-1
+
+`muc`: MUC metric, which expresses performance in terms of recall and precision, ranging from 0-1.
+
+`bcub`: B-cubed metric, which is the averaged precision of all items in the distribution, ranging from 0-1.
+
+`ceafe`: CEAFe (Constrained Entity Alignment F-Measure) is computed by aligning reference and system entities with the constraint that a reference entity is aligned with at most one reference entity. It ranges from 0-1
+
+`lea`: LEA is a Link-Based Entity-Aware metric which, for each entity, considers how important the entity is and how well it is resolved. It ranges from 0-1.
+
+`conll_score`: averaged CoNLL score (the average of the F1 values of `muc`, `bcub` and `ceafe`), ranging from 0 to 100.
+
+
+### Values from popular papers
+
+Given that many of the metrics returned by COVAL come from different sources, is it hard to cite reference values for all of them.
+
+The CoNLL score is used to track progress on different datasets such as the [ARRAU corpus](https://paperswithcode.com/sota/coreference-resolution-on-the-arrau-corpus) and [CoNLL 2012](https://paperswithcode.com/sota/coreference-resolution-on-conll-2012).
+
+## Examples
+
+Maximal values
+
+```python
+from datasets import load_metric
+coval = load_metric('coval')
+words = ['bc/cctv/00/cctv_0005 0 0 Thank VBP (TOP(S(VP* thank 01 1 Xu_li * (V*) * -',
+... 'bc/cctv/00/cctv_0005 0 1 you PRP (NP*) - - - Xu_li * (ARG1*) (ARG0*) (116)',
+... 'bc/cctv/00/cctv_0005 0 2 everyone NN (NP*) - - - Xu_li * (ARGM-DIS*) * (116)',
+... 'bc/cctv/00/cctv_0005 0 3 for IN (PP* - - - Xu_li * (ARG2* * -',
+... 'bc/cctv/00/cctv_0005 0 4 watching VBG (S(VP*)))) watch 01 1 Xu_li * *) (V*) -',
+... 'bc/cctv/00/cctv_0005 0 5 . . *)) - - - Xu_li * * * -']
+references = [words]
+predictions = [words]
+results = coval.compute(predictions=predictions, references=references)
+print(results)
+{'mentions/recall': 1.0, 'mentions/precision': 1.0, 'mentions/f1': 1.0, 'muc/recall': 1.0, 'muc/precision': 1.0, 'muc/f1': 1.0, 'bcub/recall': 1.0, 'bcub/precision': 1.0, 'bcub/f1': 1.0, 'ceafe/recall': 1.0, 'ceafe/precision': 1.0, 'ceafe/f1': 1.0, 'lea/recall': 1.0, 'lea/precision': 1.0, 'lea/f1': 1.0, 'conll_score': 100.0}
+```
+
+## Limitations and bias
+
+This wrapper of CoVal currently only works with [CoNLL line format](https://huggingface.co/datasets/conll2003), which has one word per line with all the annotation for this word in column separated by spaces:
+
+| Column | Type | Description |
+|:-------|:----------------------|:--------------------------------------------------------------------------------------------------------------------------------------------------------------------------------------------------------------------------------------------------------------------------------------------------------|
+| 1 | Document ID | This is a variation on the document filename |
+| 2 | Part number | Some files are divided into multiple parts numbered as 000, 001, 002, ... etc. |
+| 3 | Word number | |
+| 4 | Word | This is the token as segmented/tokenized in the Treebank. Initially the *_skel file contain the placeholder [WORD] which gets replaced by the actual token from the Treebank which is part of the OntoNotes release. |
+| 5 | Part-of-Speech | |
+| 6 | Parse bit | This is the bracketed structure broken before the first open parenthesis in the parse, and the word/part-of-speech leaf replaced with a *. The full parse can be created by substituting the asterix with the "([pos] [word])" string (or leaf) and concatenating the items in the rows of that column. |
+| 7 | Predicate lemma | The predicate lemma is mentioned for the rows for which we have semantic role information. All other rows are marked with a "-". |
+| 8 | Predicate Frameset ID | This is the PropBank frameset ID of the predicate in Column 7. |
+| 9 | Word sense | This is the word sense of the word in Column 3. |
+| 10 | Speaker/Author | This is the speaker or author name where available. Mostly in Broadcast Conversation and Web Log data. |
+| 11 | Named Entities | These columns identifies the spans representing various named entities. |
+| 12:N | Predicate Arguments | There is one column each of predicate argument structure information for the predicate mentioned in Column 7. |
+| N | Coreference | Coreference chain information encoded in a parenthesis structure. |
+
+## Citations
+
+```bibtex
+@InProceedings{moosavi2019minimum,
+ author = { Nafise Sadat Moosavi, Leo Born, Massimo Poesio and Michael Strube},
+ title = {Using Automatically Extracted Minimum Spans to Disentangle Coreference Evaluation from Boundary Detection},
+ year = {2019},
+ booktitle = {Proceedings of the 57th Annual Meeting of
+ the Association for Computational Linguistics (Volume 1: Long Papers)},
+ publisher = {Association for Computational Linguistics},
+ address = {Florence, Italy},
+}
+```
+```bibtex
+@inproceedings{10.3115/1072399.1072405,
+ author = {Vilain, Marc and Burger, John and Aberdeen, John and Connolly, Dennis and Hirschman, Lynette},
+ title = {A Model-Theoretic Coreference Scoring Scheme},
+ year = {1995},
+ isbn = {1558604022},
+ publisher = {Association for Computational Linguistics},
+ address = {USA},
+ url = {https://doi.org/10.3115/1072399.1072405},
+ doi = {10.3115/1072399.1072405},
+ booktitle = {Proceedings of the 6th Conference on Message Understanding},
+ pages = {45–52},
+ numpages = {8},
+ location = {Columbia, Maryland},
+ series = {MUC6 ’95}
+}
+```
+
+```bibtex
+@INPROCEEDINGS{Bagga98algorithmsfor,
+ author = {Amit Bagga and Breck Baldwin},
+ title = {Algorithms for Scoring Coreference Chains},
+ booktitle = {In The First International Conference on Language Resources and Evaluation Workshop on Linguistics Coreference},
+ year = {1998},
+ pages = {563--566}
+}
+```
+```bibtex
+@INPROCEEDINGS{Luo05oncoreference,
+ author = {Xiaoqiang Luo},
+ title = {On coreference resolution performance metrics},
+ booktitle = {In Proc. of HLT/EMNLP},
+ year = {2005},
+ pages = {25--32},
+ publisher = {URL}
+}
+```
+
+```bibtex
+@inproceedings{moosavi-strube-2016-coreference,
+ title = "Which Coreference Evaluation Metric Do You Trust? A Proposal for a Link-based Entity Aware Metric",
+ author = "Moosavi, Nafise Sadat and
+ Strube, Michael",
+ booktitle = "Proceedings of the 54th Annual Meeting of the Association for Computational Linguistics (Volume 1: Long Papers)",
+ month = aug,
+ year = "2016",
+ address = "Berlin, Germany",
+ publisher = "Association for Computational Linguistics",
+ url = "https://www.aclweb.org/anthology/P16-1060",
+ doi = "10.18653/v1/P16-1060",
+ pages = "632--642",
+}
+```
+
+## Further References
+
+- [CoNLL 2012 Task Description](http://www.conll.cemantix.org/2012/data.html): for information on the format (section "*_conll File Format")
+- [CoNLL Evaluation details](https://github.com/ns-moosavi/coval/blob/master/conll/README.md)
+- [Hugging Face - Neural Coreference Resolution (Neuralcoref)](https://huggingface.co/coref/)
+
diff --git a/metrics/coval/coval.py b/metrics/coval/coval.py
new file mode 100644
index 0000000..9db06da
--- /dev/null
+++ b/metrics/coval/coval.py
@@ -0,0 +1,320 @@
+# Copyright 2020 The HuggingFace Datasets Authors.
+#
+# Licensed under the Apache License, Version 2.0 (the "License");
+# you may not use this file except in compliance with the License.
+# You may obtain a copy of the License at
+#
+# http://www.apache.org/licenses/LICENSE-2.0
+#
+# Unless required by applicable law or agreed to in writing, software
+# distributed under the License is distributed on an "AS IS" BASIS,
+# WITHOUT WARRANTIES OR CONDITIONS OF ANY KIND, either express or implied.
+# See the License for the specific language governing permissions and
+# limitations under the License.
+""" CoVal metric. """
+import coval # From: git+https://github.com/ns-moosavi/coval.git noqa: F401
+from coval.conll import reader, util
+from coval.eval import evaluator
+
+import datasets
+
+
+logger = datasets.logging.get_logger(__name__)
+
+
+_CITATION = """\
+@InProceedings{moosavi2019minimum,
+ author = { Nafise Sadat Moosavi, Leo Born, Massimo Poesio and Michael Strube},
+ title = {Using Automatically Extracted Minimum Spans to Disentangle Coreference Evaluation from Boundary Detection},
+ year = {2019},
+ booktitle = {Proceedings of the 57th Annual Meeting of
+ the Association for Computational Linguistics (Volume 1: Long Papers)},
+ publisher = {Association for Computational Linguistics},
+ address = {Florence, Italy},
+}
+
+@inproceedings{10.3115/1072399.1072405,
+author = {Vilain, Marc and Burger, John and Aberdeen, John and Connolly, Dennis and Hirschman, Lynette},
+title = {A Model-Theoretic Coreference Scoring Scheme},
+year = {1995},
+isbn = {1558604022},
+publisher = {Association for Computational Linguistics},
+address = {USA},
+url = {https://doi.org/10.3115/1072399.1072405},
+doi = {10.3115/1072399.1072405},
+booktitle = {Proceedings of the 6th Conference on Message Understanding},
+pages = {45–52},
+numpages = {8},
+location = {Columbia, Maryland},
+series = {MUC6 ’95}
+}
+
+@INPROCEEDINGS{Bagga98algorithmsfor,
+ author = {Amit Bagga and Breck Baldwin},
+ title = {Algorithms for Scoring Coreference Chains},
+ booktitle = {In The First International Conference on Language Resources and Evaluation Workshop on Linguistics Coreference},
+ year = {1998},
+ pages = {563--566}
+}
+
+@INPROCEEDINGS{Luo05oncoreference,
+ author = {Xiaoqiang Luo},
+ title = {On coreference resolution performance metrics},
+ booktitle = {In Proc. of HLT/EMNLP},
+ year = {2005},
+ pages = {25--32},
+ publisher = {URL}
+}
+
+@inproceedings{moosavi-strube-2016-coreference,
+ title = "Which Coreference Evaluation Metric Do You Trust? A Proposal for a Link-based Entity Aware Metric",
+ author = "Moosavi, Nafise Sadat and
+ Strube, Michael",
+ booktitle = "Proceedings of the 54th Annual Meeting of the Association for Computational Linguistics (Volume 1: Long Papers)",
+ month = aug,
+ year = "2016",
+ address = "Berlin, Germany",
+ publisher = "Association for Computational Linguistics",
+ url = "https://www.aclweb.org/anthology/P16-1060",
+ doi = "10.18653/v1/P16-1060",
+ pages = "632--642",
+}
+
+"""
+
+_DESCRIPTION = """\
+CoVal is a coreference evaluation tool for the CoNLL and ARRAU datasets which
+implements of the common evaluation metrics including MUC [Vilain et al, 1995],
+B-cubed [Bagga and Baldwin, 1998], CEAFe [Luo et al., 2005],
+LEA [Moosavi and Strube, 2016] and the averaged CoNLL score
+(the average of the F1 values of MUC, B-cubed and CEAFe)
+[Denis and Baldridge, 2009a; Pradhan et al., 2011].
+
+This wrapper of CoVal currently only work with CoNLL line format:
+The CoNLL format has one word per line with all the annotation for this word in column separated by spaces:
+Column Type Description
+1 Document ID This is a variation on the document filename
+2 Part number Some files are divided into multiple parts numbered as 000, 001, 002, ... etc.
+3 Word number
+4 Word itself This is the token as segmented/tokenized in the Treebank. Initially the *_skel file contain the placeholder [WORD] which gets replaced by the actual token from the Treebank which is part of the OntoNotes release.
+5 Part-of-Speech
+6 Parse bit This is the bracketed structure broken before the first open parenthesis in the parse, and the word/part-of-speech leaf replaced with a *. The full parse can be created by substituting the asterix with the "([pos] [word])" string (or leaf) and concatenating the items in the rows of that column.
+7 Predicate lemma The predicate lemma is mentioned for the rows for which we have semantic role information. All other rows are marked with a "-"
+8 Predicate Frameset ID This is the PropBank frameset ID of the predicate in Column 7.
+9 Word sense This is the word sense of the word in Column 3.
+10 Speaker/Author This is the speaker or author name where available. Mostly in Broadcast Conversation and Web Log data.
+11 Named Entities These columns identifies the spans representing various named entities.
+12:N Predicate Arguments There is one column each of predicate argument structure information for the predicate mentioned in Column 7.
+N Coreference Coreference chain information encoded in a parenthesis structure.
+More informations on the format can be found here (section "*_conll File Format"): http://www.conll.cemantix.org/2012/data.html
+
+Details on the evaluation on CoNLL can be found here: https://github.com/ns-moosavi/coval/blob/master/conll/README.md
+
+CoVal code was written by @ns-moosavi.
+Some parts are borrowed from https://github.com/clarkkev/deep-coref/blob/master/evaluation.py
+The test suite is taken from https://github.com/conll/reference-coreference-scorers/
+Mention evaluation and the test suite are added by @andreasvc.
+Parsing CoNLL files is developed by Leo Born.
+"""
+
+_KWARGS_DESCRIPTION = """
+Calculates coreference evaluation metrics.
+Args:
+ predictions: list of sentences. Each sentence is a list of word predictions to score in the CoNLL format.
+ Each prediction is a word with its annotations as a string made of columns joined with spaces.
+ Only columns 4, 5, 6 and the last column are used (word, POS, Pars and coreference annotation)
+ See the details on the format in the description of the metric.
+ references: list of sentences. Each sentence is a list of word reference to score in the CoNLL format.
+ Each reference is a word with its annotations as a string made of columns joined with spaces.
+ Only columns 4, 5, 6 and the last column are used (word, POS, Pars and coreference annotation)
+ See the details on the format in the description of the metric.
+ keep_singletons: After extracting all mentions of key or system files,
+ mentions whose corresponding coreference chain is of size one,
+ are considered as singletons. The default evaluation mode will include
+ singletons in evaluations if they are included in the key or the system files.
+ By setting 'keep_singletons=False', all singletons in the key and system files
+ will be excluded from the evaluation.
+ NP_only: Most of the recent coreference resolvers only resolve NP mentions and
+ leave out the resolution of VPs. By setting the 'NP_only' option, the scorer will only evaluate the resolution of NPs.
+ min_span: By setting 'min_span', the scorer reports the results based on automatically detected minimum spans.
+ Minimum spans are determined using the MINA algorithm.
+
+Returns:
+ 'mentions': mentions
+ 'muc': MUC metric [Vilain et al, 1995]
+ 'bcub': B-cubed [Bagga and Baldwin, 1998]
+ 'ceafe': CEAFe [Luo et al., 2005]
+ 'lea': LEA [Moosavi and Strube, 2016]
+ 'conll_score': averaged CoNLL score (the average of the F1 values of MUC, B-cubed and CEAFe)
+
+Examples:
+
+ >>> coval = datasets.load_metric('coval')
+ >>> words = ['bc/cctv/00/cctv_0005 0 0 Thank VBP (TOP(S(VP* thank 01 1 Xu_li * (V*) * -',
+ ... 'bc/cctv/00/cctv_0005 0 1 you PRP (NP*) - - - Xu_li * (ARG1*) (ARG0*) (116)',
+ ... 'bc/cctv/00/cctv_0005 0 2 everyone NN (NP*) - - - Xu_li * (ARGM-DIS*) * (116)',
+ ... 'bc/cctv/00/cctv_0005 0 3 for IN (PP* - - - Xu_li * (ARG2* * -',
+ ... 'bc/cctv/00/cctv_0005 0 4 watching VBG (S(VP*)))) watch 01 1 Xu_li * *) (V*) -',
+ ... 'bc/cctv/00/cctv_0005 0 5 . . *)) - - - Xu_li * * * -']
+ >>> references = [words]
+ >>> predictions = [words]
+ >>> results = coval.compute(predictions=predictions, references=references)
+ >>> print(results) # doctest:+ELLIPSIS
+ {'mentions/recall': 1.0,[...] 'conll_score': 100.0}
+"""
+
+
+def get_coref_infos(
+ key_lines, sys_lines, NP_only=False, remove_nested=False, keep_singletons=True, min_span=False, doc="dummy_doc"
+):
+
+ key_doc_lines = {doc: key_lines}
+ sys_doc_lines = {doc: sys_lines}
+
+ doc_coref_infos = {}
+
+ key_nested_coref_num = 0
+ sys_nested_coref_num = 0
+ key_removed_nested_clusters = 0
+ sys_removed_nested_clusters = 0
+ key_singletons_num = 0
+ sys_singletons_num = 0
+
+ key_clusters, singletons_num = reader.get_doc_mentions(doc, key_doc_lines[doc], keep_singletons)
+ key_singletons_num += singletons_num
+
+ if NP_only or min_span:
+ key_clusters = reader.set_annotated_parse_trees(key_clusters, key_doc_lines[doc], NP_only, min_span)
+
+ sys_clusters, singletons_num = reader.get_doc_mentions(doc, sys_doc_lines[doc], keep_singletons)
+ sys_singletons_num += singletons_num
+
+ if NP_only or min_span:
+ sys_clusters = reader.set_annotated_parse_trees(sys_clusters, key_doc_lines[doc], NP_only, min_span)
+
+ if remove_nested:
+ nested_mentions, removed_clusters = reader.remove_nested_coref_mentions(key_clusters, keep_singletons)
+ key_nested_coref_num += nested_mentions
+ key_removed_nested_clusters += removed_clusters
+
+ nested_mentions, removed_clusters = reader.remove_nested_coref_mentions(sys_clusters, keep_singletons)
+ sys_nested_coref_num += nested_mentions
+ sys_removed_nested_clusters += removed_clusters
+
+ sys_mention_key_cluster = reader.get_mention_assignments(sys_clusters, key_clusters)
+ key_mention_sys_cluster = reader.get_mention_assignments(key_clusters, sys_clusters)
+
+ doc_coref_infos[doc] = (key_clusters, sys_clusters, key_mention_sys_cluster, sys_mention_key_cluster)
+
+ if remove_nested:
+ logger.info(
+ "Number of removed nested coreferring mentions in the key "
+ f"annotation: {key_nested_coref_num}; and system annotation: {sys_nested_coref_num}"
+ )
+ logger.info(
+ "Number of resulting singleton clusters in the key "
+ f"annotation: {key_removed_nested_clusters}; and system annotation: {sys_removed_nested_clusters}"
+ )
+
+ if not keep_singletons:
+ logger.info(
+ f"{key_singletons_num:d} and {sys_singletons_num:d} singletons are removed from the key and system "
+ "files, respectively"
+ )
+
+ return doc_coref_infos
+
+
+def evaluate(key_lines, sys_lines, metrics, NP_only, remove_nested, keep_singletons, min_span):
+ doc_coref_infos = get_coref_infos(key_lines, sys_lines, NP_only, remove_nested, keep_singletons, min_span)
+
+ output_scores = {}
+ conll = 0
+ conll_subparts_num = 0
+
+ for name, metric in metrics:
+ recall, precision, f1 = evaluator.evaluate_documents(doc_coref_infos, metric, beta=1)
+ if name in ["muc", "bcub", "ceafe"]:
+ conll += f1
+ conll_subparts_num += 1
+ output_scores.update({f"{name}/recall": recall, f"{name}/precision": precision, f"{name}/f1": f1})
+
+ logger.info(
+ name.ljust(10),
+ f"Recall: {recall * 100:.2f}",
+ f" Precision: {precision * 100:.2f}",
+ f" F1: {f1 * 100:.2f}",
+ )
+
+ if conll_subparts_num == 3:
+ conll = (conll / 3) * 100
+ logger.info(f"CoNLL score: {conll:.2f}")
+ output_scores.update({"conll_score": conll})
+
+ return output_scores
+
+
+def check_gold_parse_annotation(key_lines):
+ has_gold_parse = False
+ for line in key_lines:
+ if not line.startswith("#"):
+ if len(line.split()) > 6:
+ parse_col = line.split()[5]
+ if not parse_col == "-":
+ has_gold_parse = True
+ break
+ else:
+ break
+ return has_gold_parse
+
+
+@datasets.utils.file_utils.add_start_docstrings(_DESCRIPTION, _KWARGS_DESCRIPTION)
+class Coval(datasets.Metric):
+ def _info(self):
+ return datasets.MetricInfo(
+ description=_DESCRIPTION,
+ citation=_CITATION,
+ inputs_description=_KWARGS_DESCRIPTION,
+ features=datasets.Features(
+ {
+ "predictions": datasets.Sequence(datasets.Value("string")),
+ "references": datasets.Sequence(datasets.Value("string")),
+ }
+ ),
+ codebase_urls=["https://github.com/ns-moosavi/coval"],
+ reference_urls=[
+ "https://github.com/ns-moosavi/coval",
+ "https://www.aclweb.org/anthology/P16-1060",
+ "http://www.conll.cemantix.org/2012/data.html",
+ ],
+ )
+
+ def _compute(
+ self, predictions, references, keep_singletons=True, NP_only=False, min_span=False, remove_nested=False
+ ):
+ allmetrics = [
+ ("mentions", evaluator.mentions),
+ ("muc", evaluator.muc),
+ ("bcub", evaluator.b_cubed),
+ ("ceafe", evaluator.ceafe),
+ ("lea", evaluator.lea),
+ ]
+
+ if min_span:
+ has_gold_parse = util.check_gold_parse_annotation(references)
+ if not has_gold_parse:
+ raise NotImplementedError("References should have gold parse annotation to use 'min_span'.")
+ # util.parse_key_file(key_file)
+ # key_file = key_file + ".parsed"
+
+ score = evaluate(
+ key_lines=references,
+ sys_lines=predictions,
+ metrics=allmetrics,
+ NP_only=NP_only,
+ remove_nested=remove_nested,
+ keep_singletons=keep_singletons,
+ min_span=min_span,
+ )
+
+ return score
diff --git a/metrics/cuad/README.md b/metrics/cuad/README.md
new file mode 100644
index 0000000..309cc97
--- /dev/null
+++ b/metrics/cuad/README.md
@@ -0,0 +1,112 @@
+# Metric Card for CUAD
+
+## Metric description
+
+This metric wraps the official scoring script for version 1 of the [Contract Understanding Atticus Dataset (CUAD)](https://huggingface.co/datasets/cuad), which is a corpus of more than 13,000 labels in 510 commercial legal contracts that have been manually labeled to identify 41 categories of important clauses that lawyers look for when reviewing contracts in connection with corporate transactions.
+
+The CUAD metric computes several scores: [Exact Match](https://huggingface.co/metrics/exact_match), [F1 score](https://huggingface.co/metrics/f1), Area Under the Precision-Recall Curve, [Precision](https://huggingface.co/metrics/precision) at 80% [recall](https://huggingface.co/metrics/recall) and Precision at 90% recall.
+
+## How to use
+
+The CUAD metric takes two inputs :
+
+
+`predictions`, a list of question-answer dictionaries with the following key-values:
+- `id`: the id of the question-answer pair as given in the references.
+- `prediction_text`: a list of possible texts for the answer, as a list of strings depending on a threshold on the confidence probability of each prediction.
+
+
+`references`: a list of question-answer dictionaries with the following key-values:
+ - `id`: the id of the question-answer pair (the same as above).
+ - `answers`: a dictionary *in the CUAD dataset format* with the following keys:
+ - `text`: a list of possible texts for the answer, as a list of strings.
+ - `answer_start`: a list of start positions for the answer, as a list of ints.
+
+ Note that `answer_start` values are not taken into account to compute the metric.
+
+```python
+from datasets import load_metric
+cuad_metric = load_metric("cuad")
+predictions = [{'prediction_text': ['The seller:', 'The buyer/End-User: Shenzhen LOHAS Supply Chain Management Co., Ltd.'], 'id': 'LohaCompanyltd_20191209_F-1_EX-10.16_11917878_EX-10.16_Supply Agreement__Parties'}]
+references = [{'answers': {'answer_start': [143, 49], 'text': ['The seller:', 'The buyer/End-User: Shenzhen LOHAS Supply Chain Management Co., Ltd.']}, 'id': 'LohaCompanyltd_20191209_F-1_EX-10.16_11917878_EX-10.16_Supply Agreement__Parties'}]
+results = cuad_metric.compute(predictions=predictions, references=references)
+```
+## Output values
+
+The output of the CUAD metric consists of a dictionary that contains one or several of the following metrics:
+
+`exact_match`: The normalized answers that exactly match the reference answer, with a range between 0.0 and 1.0 (see [exact match](https://huggingface.co/metrics/exact_match) for more information).
+
+`f1`: The harmonic mean of the precision and recall (see [F1 score](https://huggingface.co/metrics/f1) for more information). Its range is between 0.0 and 1.0 -- its lowest possible value is 0, if either the precision or the recall is 0, and its highest possible value is 1.0, which means perfect precision and recall.
+
+`aupr`: The Area Under the Precision-Recall curve, with a range between 0.0 and 1.0, with a higher value representing both high recall and high precision, and a low value representing low values for both. See the [Wikipedia article](https://en.wikipedia.org/wiki/Receiver_operating_characteristic#Area_under_the_curve) for more information.
+
+`prec_at_80_recall`: The fraction of true examples among the predicted examples at a recall rate of 80%. Its range is between 0.0 and 1.0. For more information, see [precision](https://huggingface.co/metrics/precision) and [recall](https://huggingface.co/metrics/recall).
+
+`prec_at_90_recall`: The fraction of true examples among the predicted examples at a recall rate of 90%. Its range is between 0.0 and 1.0.
+
+
+### Values from popular papers
+The [original CUAD paper](https://arxiv.org/pdf/2103.06268.pdf) reports that a [DeBERTa model](https://huggingface.co/microsoft/deberta-base) attains
+an AUPR of 47.8%, a Precision at 80% Recall of 44.0%, and a Precision at 90% Recall of 17.8% (they do not report F1 or Exact Match separately).
+
+For more recent model performance, see the [dataset leaderboard](https://paperswithcode.com/dataset/cuad).
+
+## Examples
+
+Maximal values :
+
+```python
+from datasets import load_metric
+cuad_metric = load_metric("cuad")
+predictions = [{'prediction_text': ['The seller:', 'The buyer/End-User: Shenzhen LOHAS Supply Chain Management Co., Ltd.'], 'id': 'LohaCompanyltd_20191209_F-1_EX-10.16_11917878_EX-10.16_Supply Agreement__Parties'}]
+references = [{'answers': {'answer_start': [143, 49], 'text': ['The seller:', 'The buyer/End-User: Shenzhen LOHAS Supply Chain Management Co., Ltd.']}, 'id': 'LohaCompanyltd_20191209_F-1_EX-10.16_11917878_EX-10.16_Supply Agreement__Parties'}]
+results = cuad_metric.compute(predictions=predictions, references=references)
+print(results)
+{'exact_match': 100.0, 'f1': 100.0, 'aupr': 0.0, 'prec_at_80_recall': 1.0, 'prec_at_90_recall': 1.0}
+```
+
+Minimal values:
+
+```python
+from datasets import load_metric
+cuad_metric = load_metric("cuad")
+predictions = [{'prediction_text': ['The Company appoints the Distributor as an exclusive distributor of Products in the Market, subject to the terms and conditions of this Agreement.'], 'id': 'LIMEENERGYCO_09_09_1999-EX-10-DISTRIBUTOR AGREEMENT__Exclusivity_0'}]
+references = [{'answers': {'answer_start': [143], 'text': 'The seller'}, 'id': 'LIMEENERGYCO_09_09_1999-EX-10-DISTRIBUTOR AGREEMENT__Exclusivity_0'}]
+results = cuad_metric.compute(predictions=predictions, references=references)
+print(results)
+{'exact_match': 0.0, 'f1': 0.0, 'aupr': 0.0, 'prec_at_80_recall': 0, 'prec_at_90_recall': 0}
+```
+
+Partial match:
+
+```python
+from datasets import load_metric
+cuad_metric = load_metric("cuad")
+predictions = [{'prediction_text': ['The seller:', 'The buyer/End-User: Shenzhen LOHAS Supply Chain Management Co., Ltd.'], 'id': 'LohaCompanyltd_20191209_F-1_EX-10.16_11917878_EX-10.16_Supply Agreement__Parties'}]
+predictions = [{'prediction_text': ['The Company appoints the Distributor as an exclusive distributor of Products in the Market, subject to the terms and conditions of this Agreement.', 'The buyer/End-User: Shenzhen LOHAS Supply Chain Management Co., Ltd.'], 'id': 'LohaCompanyltd_20191209_F-1_EX-10.16_11917878_EX-10.16_Supply Agreement__Parties'}]
+results = cuad_metric.compute(predictions=predictions, references=references)
+print(results)
+{'exact_match': 100.0, 'f1': 50.0, 'aupr': 0.0, 'prec_at_80_recall': 0, 'prec_at_90_recall': 0}
+```
+
+## Limitations and bias
+This metric works only with datasets that have the same format as the [CUAD dataset](https://huggingface.co/datasets/cuad). The limitations of the biases of this dataset are not discussed, but could exhibit annotation bias given the homogeneity of annotators for this dataset.
+
+In terms of the metric itself, the accuracy of AUPR has been debated because its estimates are quite noisy and because of the fact that reducing the Precision-Recall Curve to a single number ignores the fact that it is about the tradeoffs between the different systems or performance points plotted and not the performance of an individual system. Reporting the original F1 and exact match scores is therefore useful to ensure a more complete representation of system performance.
+
+
+## Citation
+
+```bibtex
+@article{hendrycks2021cuad,
+ title={CUAD: An Expert-Annotated NLP Dataset for Legal Contract Review},
+ author={Dan Hendrycks and Collin Burns and Anya Chen and Spencer Ball},
+ journal={arXiv preprint arXiv:2103.06268},
+ year={2021}
+}
+```
+
+## Further References
+
+- [CUAD dataset homepage](https://www.atticusprojectai.org/cuad-v1-performance-announcements)
diff --git a/metrics/cuad/cuad.py b/metrics/cuad/cuad.py
new file mode 100644
index 0000000..78cc114
--- /dev/null
+++ b/metrics/cuad/cuad.py
@@ -0,0 +1,115 @@
+# Copyright 2020 The HuggingFace Datasets Authors.
+#
+# Licensed under the Apache License, Version 2.0 (the "License");
+# you may not use this file except in compliance with the License.
+# You may obtain a copy of the License at
+#
+# http://www.apache.org/licenses/LICENSE-2.0
+#
+# Unless required by applicable law or agreed to in writing, software
+# distributed under the License is distributed on an "AS IS" BASIS,
+# WITHOUT WARRANTIES OR CONDITIONS OF ANY KIND, either express or implied.
+# See the License for the specific language governing permissions and
+# limitations under the License.
+""" CUAD metric. """
+
+import datasets
+
+from .evaluate import evaluate
+
+
+_CITATION = """\
+@article{hendrycks2021cuad,
+ title={CUAD: An Expert-Annotated NLP Dataset for Legal Contract Review},
+ author={Dan Hendrycks and Collin Burns and Anya Chen and Spencer Ball},
+ journal={arXiv preprint arXiv:2103.06268},
+ year={2021}
+}
+"""
+
+_DESCRIPTION = """
+This metric wrap the official scoring script for version 1 of the Contract
+Understanding Atticus Dataset (CUAD).
+Contract Understanding Atticus Dataset (CUAD) v1 is a corpus of more than 13,000 labels in 510
+commercial legal contracts that have been manually labeled to identify 41 categories of important
+clauses that lawyers look for when reviewing contracts in connection with corporate transactions.
+"""
+
+_KWARGS_DESCRIPTION = """
+Computes CUAD scores (EM, F1, AUPR, Precision@80%Recall, and Precision@90%Recall).
+Args:
+ predictions: List of question-answers dictionaries with the following key-values:
+ - 'id': id of the question-answer pair as given in the references (see below)
+ - 'prediction_text': list of possible texts for the answer, as a list of strings
+ depending on a threshold on the confidence probability of each prediction.
+ references: List of question-answers dictionaries with the following key-values:
+ - 'id': id of the question-answer pair (see above),
+ - 'answers': a Dict in the CUAD dataset format
+ {
+ 'text': list of possible texts for the answer, as a list of strings
+ 'answer_start': list of start positions for the answer, as a list of ints
+ }
+ Note that answer_start values are not taken into account to compute the metric.
+Returns:
+ 'exact_match': Exact match (the normalized answer exactly match the gold answer)
+ 'f1': The F-score of predicted tokens versus the gold answer
+ 'aupr': Area Under the Precision-Recall curve
+ 'prec_at_80_recall': Precision at 80% recall
+ 'prec_at_90_recall': Precision at 90% recall
+Examples:
+ >>> predictions = [{'prediction_text': ['The seller:', 'The buyer/End-User: Shenzhen LOHAS Supply Chain Management Co., Ltd.'], 'id': 'LohaCompanyltd_20191209_F-1_EX-10.16_11917878_EX-10.16_Supply Agreement__Parties'}]
+ >>> references = [{'answers': {'answer_start': [143, 49], 'text': ['The seller:', 'The buyer/End-User: Shenzhen LOHAS Supply Chain Management Co., Ltd.']}, 'id': 'LohaCompanyltd_20191209_F-1_EX-10.16_11917878_EX-10.16_Supply Agreement__Parties'}]
+ >>> cuad_metric = datasets.load_metric("cuad")
+ >>> results = cuad_metric.compute(predictions=predictions, references=references)
+ >>> print(results)
+ {'exact_match': 100.0, 'f1': 100.0, 'aupr': 0.0, 'prec_at_80_recall': 1.0, 'prec_at_90_recall': 1.0}
+"""
+
+
+@datasets.utils.file_utils.add_start_docstrings(_DESCRIPTION, _KWARGS_DESCRIPTION)
+class CUAD(datasets.Metric):
+ def _info(self):
+ return datasets.MetricInfo(
+ description=_DESCRIPTION,
+ citation=_CITATION,
+ inputs_description=_KWARGS_DESCRIPTION,
+ features=datasets.Features(
+ {
+ "predictions": {
+ "id": datasets.Value("string"),
+ "prediction_text": datasets.features.Sequence(datasets.Value("string")),
+ },
+ "references": {
+ "id": datasets.Value("string"),
+ "answers": datasets.features.Sequence(
+ {
+ "text": datasets.Value("string"),
+ "answer_start": datasets.Value("int32"),
+ }
+ ),
+ },
+ }
+ ),
+ codebase_urls=["https://www.atticusprojectai.org/cuad"],
+ reference_urls=["https://www.atticusprojectai.org/cuad"],
+ )
+
+ def _compute(self, predictions, references):
+ pred_dict = {prediction["id"]: prediction["prediction_text"] for prediction in predictions}
+ dataset = [
+ {
+ "paragraphs": [
+ {
+ "qas": [
+ {
+ "answers": [{"text": answer_text} for answer_text in ref["answers"]["text"]],
+ "id": ref["id"],
+ }
+ for ref in references
+ ]
+ }
+ ]
+ }
+ ]
+ score = evaluate(dataset=dataset, predictions=pred_dict)
+ return score
diff --git a/metrics/cuad/evaluate.py b/metrics/cuad/evaluate.py
new file mode 100644
index 0000000..3fbe88c
--- /dev/null
+++ b/metrics/cuad/evaluate.py
@@ -0,0 +1,205 @@
+""" Official evaluation script for CUAD dataset. """
+
+import argparse
+import json
+import re
+import string
+import sys
+
+import numpy as np
+
+
+IOU_THRESH = 0.5
+
+
+def get_jaccard(prediction, ground_truth):
+ remove_tokens = [".", ",", ";", ":"]
+
+ for token in remove_tokens:
+ ground_truth = ground_truth.replace(token, "")
+ prediction = prediction.replace(token, "")
+
+ ground_truth, prediction = ground_truth.lower(), prediction.lower()
+ ground_truth, prediction = ground_truth.replace("/", " "), prediction.replace("/", " ")
+ ground_truth, prediction = set(ground_truth.split(" ")), set(prediction.split(" "))
+
+ intersection = ground_truth.intersection(prediction)
+ union = ground_truth.union(prediction)
+ jaccard = len(intersection) / len(union)
+ return jaccard
+
+
+def normalize_answer(s):
+ """Lower text and remove punctuation, articles and extra whitespace."""
+
+ def remove_articles(text):
+ return re.sub(r"\b(a|an|the)\b", " ", text)
+
+ def white_space_fix(text):
+ return " ".join(text.split())
+
+ def remove_punc(text):
+ exclude = set(string.punctuation)
+ return "".join(ch for ch in text if ch not in exclude)
+
+ def lower(text):
+ return text.lower()
+
+ return white_space_fix(remove_articles(remove_punc(lower(s))))
+
+
+def compute_precision_recall(predictions, ground_truths, qa_id):
+ tp, fp, fn = 0, 0, 0
+
+ substr_ok = "Parties" in qa_id
+
+ # first check if ground truth is empty
+ if len(ground_truths) == 0:
+ if len(predictions) > 0:
+ fp += len(predictions) # false positive for each one
+ else:
+ for ground_truth in ground_truths:
+ assert len(ground_truth) > 0
+ # check if there is a match
+ match_found = False
+ for pred in predictions:
+ if substr_ok:
+ is_match = get_jaccard(pred, ground_truth) >= IOU_THRESH or ground_truth in pred
+ else:
+ is_match = get_jaccard(pred, ground_truth) >= IOU_THRESH
+ if is_match:
+ match_found = True
+
+ if match_found:
+ tp += 1
+ else:
+ fn += 1
+
+ # now also get any fps by looping through preds
+ for pred in predictions:
+ # Check if there's a match. if so, don't count (don't want to double count based on the above)
+ # but if there's no match, then this is a false positive.
+ # (Note: we get the true positives in the above loop instead of this loop so that we don't double count
+ # multiple predictions that are matched with the same answer.)
+ match_found = False
+ for ground_truth in ground_truths:
+ assert len(ground_truth) > 0
+ if substr_ok:
+ is_match = get_jaccard(pred, ground_truth) >= IOU_THRESH or ground_truth in pred
+ else:
+ is_match = get_jaccard(pred, ground_truth) >= IOU_THRESH
+ if is_match:
+ match_found = True
+
+ if not match_found:
+ fp += 1
+
+ precision = tp / (tp + fp) if tp + fp > 0 else np.nan
+ recall = tp / (tp + fn) if tp + fn > 0 else np.nan
+
+ return precision, recall
+
+
+def process_precisions(precisions):
+ """
+ Processes precisions to ensure that precision and recall don't both get worse.
+ Assumes the list precision is sorted in order of recalls
+ """
+ precision_best = precisions[::-1]
+ for i in range(1, len(precision_best)):
+ precision_best[i] = max(precision_best[i - 1], precision_best[i])
+ precisions = precision_best[::-1]
+ return precisions
+
+
+def get_aupr(precisions, recalls):
+ processed_precisions = process_precisions(precisions)
+ aupr = np.trapz(processed_precisions, recalls)
+ if np.isnan(aupr):
+ return 0
+ return aupr
+
+
+def get_prec_at_recall(precisions, recalls, recall_thresh):
+ """Assumes recalls are sorted in increasing order"""
+ processed_precisions = process_precisions(precisions)
+ prec_at_recall = 0
+ for prec, recall in zip(processed_precisions, recalls):
+ if recall >= recall_thresh:
+ prec_at_recall = prec
+ break
+ return prec_at_recall
+
+
+def exact_match_score(prediction, ground_truth):
+ return normalize_answer(prediction) == normalize_answer(ground_truth)
+
+
+def metric_max_over_ground_truths(metric_fn, predictions, ground_truths):
+ score = 0
+ for pred in predictions:
+ for ground_truth in ground_truths:
+ score = metric_fn(pred, ground_truth)
+ if score == 1: # break the loop when one prediction matches the ground truth
+ break
+ if score == 1:
+ break
+ return score
+
+
+def evaluate(dataset, predictions):
+ f1 = exact_match = total = 0
+ precisions = []
+ recalls = []
+ for article in dataset:
+ for paragraph in article["paragraphs"]:
+ for qa in paragraph["qas"]:
+ total += 1
+ if qa["id"] not in predictions:
+ message = "Unanswered question " + qa["id"] + " will receive score 0."
+ print(message, file=sys.stderr)
+ continue
+ ground_truths = list(map(lambda x: x["text"], qa["answers"]))
+ prediction = predictions[qa["id"]]
+ precision, recall = compute_precision_recall(prediction, ground_truths, qa["id"])
+
+ precisions.append(precision)
+ recalls.append(recall)
+
+ if precision == 0 and recall == 0:
+ f1 += 0
+ else:
+ f1 += 2 * (precision * recall) / (precision + recall)
+
+ exact_match += metric_max_over_ground_truths(exact_match_score, prediction, ground_truths)
+
+ precisions = [x for _, x in sorted(zip(recalls, precisions))]
+ recalls.sort()
+
+ f1 = 100.0 * f1 / total
+ exact_match = 100.0 * exact_match / total
+ aupr = get_aupr(precisions, recalls)
+
+ prec_at_90_recall = get_prec_at_recall(precisions, recalls, recall_thresh=0.9)
+ prec_at_80_recall = get_prec_at_recall(precisions, recalls, recall_thresh=0.8)
+
+ return {
+ "exact_match": exact_match,
+ "f1": f1,
+ "aupr": aupr,
+ "prec_at_80_recall": prec_at_80_recall,
+ "prec_at_90_recall": prec_at_90_recall,
+ }
+
+
+if __name__ == "__main__":
+ parser = argparse.ArgumentParser(description="Evaluation for CUAD")
+ parser.add_argument("dataset_file", help="Dataset file")
+ parser.add_argument("prediction_file", help="Prediction File")
+ args = parser.parse_args()
+ with open(args.dataset_file) as dataset_file:
+ dataset_json = json.load(dataset_file)
+ dataset = dataset_json["data"]
+ with open(args.prediction_file) as prediction_file:
+ predictions = json.load(prediction_file)
+ print(json.dumps(evaluate(dataset, predictions)))
diff --git a/metrics/exact_match/README.md b/metrics/exact_match/README.md
new file mode 100644
index 0000000..47df0ba
--- /dev/null
+++ b/metrics/exact_match/README.md
@@ -0,0 +1,103 @@
+# Metric Card for Exact Match
+
+
+## Metric Description
+A given predicted string's exact match score is 1 if it is the exact same as its reference string, and is 0 otherwise.
+
+- **Example 1**: The exact match score of prediction "Happy Birthday!" is 0, given its reference is "Happy New Year!".
+- **Example 2**: The exact match score of prediction "The Colour of Magic (1983)" is 1, given its reference is also "The Colour of Magic (1983)".
+
+The exact match score of a set of predictions is the sum of all of the individual exact match scores in the set, divided by the total number of predictions in the set.
+
+- **Example**: The exact match score of the set {Example 1, Example 2} (above) is 0.5.
+
+
+## How to Use
+At minimum, this metric takes as input predictions and references:
+```python
+>>> from datasets import load_metric
+>>> exact_match_metric = load_metric("exact_match")
+>>> results = exact_match_metric.compute(predictions=predictions, references=references)
+```
+
+### Inputs
+- **`predictions`** (`list` of `str`): List of predicted texts.
+- **`references`** (`list` of `str`): List of reference texts.
+- **`regexes_to_ignore`** (`list` of `str`): Regex expressions of characters to ignore when calculating the exact matches. Defaults to `None`. Note: the regex changes are applied before capitalization is normalized.
+- **`ignore_case`** (`bool`): If `True`, turns everything to lowercase so that capitalization differences are ignored. Defaults to `False`.
+- **`ignore_punctuation`** (`bool`): If `True`, removes punctuation before comparing strings. Defaults to `False`.
+- **`ignore_numbers`** (`bool`): If `True`, removes all digits before comparing strings. Defaults to `False`.
+
+
+### Output Values
+This metric outputs a dictionary with one value: the average exact match score.
+
+```python
+{'exact_match': 100.0}
+```
+
+This metric's range is 0-100, inclusive. Here, 0.0 means no prediction/reference pairs were matches, while 100.0 means they all were.
+
+#### Values from Popular Papers
+The exact match metric is often included in other metrics, such as SQuAD. For example, the [original SQuAD paper](https://nlp.stanford.edu/pubs/rajpurkar2016squad.pdf) reported an Exact Match score of 40.0%. They also report that the human performance Exact Match score on the dataset was 80.3%.
+
+### Examples
+Without including any regexes to ignore:
+```python
+>>> exact_match = datasets.load_metric("exact_match")
+>>> refs = ["the cat", "theater", "YELLING", "agent007"]
+>>> preds = ["cat?", "theater", "yelling", "agent"]
+>>> results = exact_match.compute(references=refs, predictions=preds)
+>>> print(round(results["exact_match"], 1))
+25.0
+```
+
+Ignoring regexes "the" and "yell", as well as ignoring case and punctuation:
+```python
+>>> exact_match = datasets.load_metric("exact_match")
+>>> refs = ["the cat", "theater", "YELLING", "agent007"]
+>>> preds = ["cat?", "theater", "yelling", "agent"]
+>>> results = exact_match.compute(references=refs, predictions=preds, regexes_to_ignore=["the ", "yell"], ignore_case=True, ignore_punctuation=True)
+>>> print(round(results["exact_match"], 1))
+50.0
+```
+Note that in the example above, because the regexes are ignored before the case is normalized, "yell" from "YELLING" is not deleted.
+
+Ignoring "the", "yell", and "YELL", as well as ignoring case and punctuation:
+```python
+>>> exact_match = datasets.load_metric("exact_match")
+>>> refs = ["the cat", "theater", "YELLING", "agent007"]
+>>> preds = ["cat?", "theater", "yelling", "agent"]
+>>> results = exact_match.compute(references=refs, predictions=preds, regexes_to_ignore=["the ", "yell", "YELL"], ignore_case=True, ignore_punctuation=True)
+>>> print(round(results["exact_match"], 1))
+75.0
+```
+
+Ignoring "the", "yell", and "YELL", as well as ignoring case, punctuation, and numbers:
+```python
+>>> exact_match = datasets.load_metric("exact_match")
+>>> refs = ["the cat", "theater", "YELLING", "agent007"]
+>>> preds = ["cat?", "theater", "yelling", "agent"]
+>>> results = exact_match.compute(references=refs, predictions=preds, regexes_to_ignore=["the ", "yell", "YELL"], ignore_case=True, ignore_punctuation=True, ignore_numbers=True)
+>>> print(round(results["exact_match"], 1))
+100.0
+```
+
+An example that includes sentences:
+```python
+>>> exact_match = datasets.load_metric("exact_match")
+>>> refs = ["The cat sat on the mat.", "Theaters are great.", "It's like comparing oranges and apples."]
+>>> preds = ["The cat sat on the mat?", "Theaters are great.", "It's like comparing apples and oranges."]
+>>> results = exact_match.compute(references=refs, predictions=preds)
+>>> print(round(results["exact_match"], 1))
+33.3
+```
+
+
+## Limitations and Bias
+This metric is limited in that it outputs the same score for something that is completely wrong as for something that is correct except for a single character. In other words, there is no award for being *almost* right.
+
+## Citation
+
+## Further References
+- Also used in the [SQuAD metric](https://github.com/huggingface/datasets/tree/main/metrics/squad)
diff --git a/metrics/exact_match/exact_match.py b/metrics/exact_match/exact_match.py
new file mode 100644
index 0000000..00f8619
--- /dev/null
+++ b/metrics/exact_match/exact_match.py
@@ -0,0 +1,136 @@
+# Copyright 2020 The HuggingFace Datasets Authors and the current dataset script contributor.
+#
+# Licensed under the Apache License, Version 2.0 (the "License");
+# you may not use this file except in compliance with the License.
+# You may obtain a copy of the License at
+#
+# http://www.apache.org/licenses/LICENSE-2.0
+#
+# Unless required by applicable law or agreed to in writing, software
+# distributed under the License is distributed on an "AS IS" BASIS,
+# WITHOUT WARRANTIES OR CONDITIONS OF ANY KIND, either express or implied.
+# See the License for the specific language governing permissions and
+# limitations under the License.
+"""Exact Match metric."""
+import re
+import string
+
+import numpy as np
+
+import datasets
+
+
+_DESCRIPTION = """
+Returns the rate at which the input predicted strings exactly match their references, ignoring any strings input as part of the regexes_to_ignore list.
+"""
+
+_KWARGS_DESCRIPTION = """
+Args:
+ predictions: List of predicted texts.
+ references: List of reference texts.
+ regexes_to_ignore: List, defaults to None. Regex expressions of characters to
+ ignore when calculating the exact matches. Note: these regexes are removed
+ from the input data before the changes based on the options below (e.g. ignore_case,
+ ignore_punctuation, ignore_numbers) are applied.
+ ignore_case: Boolean, defaults to False. If true, turns everything
+ to lowercase so that capitalization differences are ignored.
+ ignore_punctuation: Boolean, defaults to False. If true, removes all punctuation before
+ comparing predictions and references.
+ ignore_numbers: Boolean, defaults to False. If true, removes all punctuation before
+ comparing predictions and references.
+Returns:
+ exact_match: Dictionary containing exact_match rate. Possible values are between 0.0 and 100.0, inclusive.
+Examples:
+ >>> exact_match = datasets.load_metric("exact_match")
+ >>> refs = ["the cat", "theater", "YELLING", "agent007"]
+ >>> preds = ["cat?", "theater", "yelling", "agent"]
+ >>> results = exact_match.compute(references=refs, predictions=preds)
+ >>> print(round(results["exact_match"], 1))
+ 25.0
+
+ >>> exact_match = datasets.load_metric("exact_match")
+ >>> refs = ["the cat", "theater", "YELLING", "agent007"]
+ >>> preds = ["cat?", "theater", "yelling", "agent"]
+ >>> results = exact_match.compute(references=refs, predictions=preds, regexes_to_ignore=["the ", "yell"], ignore_case=True, ignore_punctuation=True)
+ >>> print(round(results["exact_match"], 1))
+ 50.0
+
+
+ >>> exact_match = datasets.load_metric("exact_match")
+ >>> refs = ["the cat", "theater", "YELLING", "agent007"]
+ >>> preds = ["cat?", "theater", "yelling", "agent"]
+ >>> results = exact_match.compute(references=refs, predictions=preds, regexes_to_ignore=["the ", "yell", "YELL"], ignore_case=True, ignore_punctuation=True)
+ >>> print(round(results["exact_match"], 1))
+ 75.0
+
+ >>> exact_match = datasets.load_metric("exact_match")
+ >>> refs = ["the cat", "theater", "YELLING", "agent007"]
+ >>> preds = ["cat?", "theater", "yelling", "agent"]
+ >>> results = exact_match.compute(references=refs, predictions=preds, regexes_to_ignore=["the ", "yell", "YELL"], ignore_case=True, ignore_punctuation=True, ignore_numbers=True)
+ >>> print(round(results["exact_match"], 1))
+ 100.0
+
+ >>> exact_match = datasets.load_metric("exact_match")
+ >>> refs = ["The cat sat on the mat.", "Theaters are great.", "It's like comparing oranges and apples."]
+ >>> preds = ["The cat sat on the mat?", "Theaters are great.", "It's like comparing apples and oranges."]
+ >>> results = exact_match.compute(references=refs, predictions=preds)
+ >>> print(round(results["exact_match"], 1))
+ 33.3
+
+"""
+
+_CITATION = """
+"""
+
+
+@datasets.utils.file_utils.add_start_docstrings(_DESCRIPTION, _KWARGS_DESCRIPTION)
+class ExactMatch(datasets.Metric):
+ def _info(self):
+ return datasets.MetricInfo(
+ description=_DESCRIPTION,
+ citation=_CITATION,
+ inputs_description=_KWARGS_DESCRIPTION,
+ features=datasets.Features(
+ {
+ "predictions": datasets.Value("string", id="sequence"),
+ "references": datasets.Value("string", id="sequence"),
+ }
+ ),
+ reference_urls=[],
+ )
+
+ def _compute(
+ self,
+ predictions,
+ references,
+ regexes_to_ignore=None,
+ ignore_case=False,
+ ignore_punctuation=False,
+ ignore_numbers=False,
+ ):
+
+ if regexes_to_ignore is not None:
+ for s in regexes_to_ignore:
+ predictions = np.array([re.sub(s, "", x) for x in predictions])
+ references = np.array([re.sub(s, "", x) for x in references])
+ else:
+ predictions = np.asarray(predictions)
+ references = np.asarray(references)
+
+ if ignore_case:
+ predictions = np.char.lower(predictions)
+ references = np.char.lower(references)
+
+ if ignore_punctuation:
+ repl_table = string.punctuation.maketrans("", "", string.punctuation)
+ predictions = np.char.translate(predictions, table=repl_table)
+ references = np.char.translate(references, table=repl_table)
+
+ if ignore_numbers:
+ repl_table = string.digits.maketrans("", "", string.digits)
+ predictions = np.char.translate(predictions, table=repl_table)
+ references = np.char.translate(references, table=repl_table)
+
+ score_list = predictions == references
+
+ return {"exact_match": np.mean(score_list) * 100}
diff --git a/metrics/f1/README.md b/metrics/f1/README.md
new file mode 100644
index 0000000..5cf4f25
--- /dev/null
+++ b/metrics/f1/README.md
@@ -0,0 +1,120 @@
+# Metric Card for F1
+
+
+## Metric Description
+
+The F1 score is the harmonic mean of the precision and recall. It can be computed with the equation:
+F1 = 2 * (precision * recall) / (precision + recall)
+
+
+## How to Use
+
+At minimum, this metric requires predictions and references as input
+
+```python
+>>> f1_metric = datasets.load_metric("f1")
+>>> results = f1_metric.compute(predictions=[0, 1], references=[0, 1])
+>>> print(results)
+["{'f1': 1.0}"]
+```
+
+
+### Inputs
+- **predictions** (`list` of `int`): Predicted labels.
+- **references** (`list` of `int`): Ground truth labels.
+- **labels** (`list` of `int`): The set of labels to include when `average` is not set to `'binary'`, and the order of the labels if `average` is `None`. Labels present in the data can be excluded, for example to calculate a multiclass average ignoring a majority negative class. Labels not present in the data will result in 0 components in a macro average. For multilabel targets, labels are column indices. By default, all labels in `predictions` and `references` are used in sorted order. Defaults to None.
+- **pos_label** (`int`): The class to be considered the positive class, in the case where `average` is set to `binary`. Defaults to 1.
+- **average** (`string`): This parameter is required for multiclass/multilabel targets. If set to `None`, the scores for each class are returned. Otherwise, this determines the type of averaging performed on the data. Defaults to `'binary'`.
+ - 'binary': Only report results for the class specified by `pos_label`. This is applicable only if the classes found in `predictions` and `references` are binary.
+ - 'micro': Calculate metrics globally by counting the total true positives, false negatives and false positives.
+ - 'macro': Calculate metrics for each label, and find their unweighted mean. This does not take label imbalance into account.
+ - 'weighted': Calculate metrics for each label, and find their average weighted by support (the number of true instances for each label). This alters `'macro'` to account for label imbalance. This option can result in an F-score that is not between precision and recall.
+ - 'samples': Calculate metrics for each instance, and find their average (only meaningful for multilabel classification).
+- **sample_weight** (`list` of `float`): Sample weights Defaults to None.
+
+
+### Output Values
+- **f1**(`float` or `array` of `float`): F1 score or list of f1 scores, depending on the value passed to `average`. Minimum possible value is 0. Maximum possible value is 1. Higher f1 scores are better.
+
+Output Example(s):
+```python
+{'f1': 0.26666666666666666}
+```
+```python
+{'f1': array([0.8, 0.0, 0.0])}
+```
+
+This metric outputs a dictionary, with either a single f1 score, of type `float`, or an array of f1 scores, with entries of type `float`.
+
+
+#### Values from Popular Papers
+
+
+
+
+### Examples
+
+Example 1-A simple binary example
+```python
+>>> f1_metric = datasets.load_metric("f1")
+>>> results = f1_metric.compute(references=[0, 1, 0, 1, 0], predictions=[0, 0, 1, 1, 0])
+>>> print(results)
+{'f1': 0.5}
+```
+
+Example 2-The same simple binary example as in Example 1, but with `pos_label` set to `0`.
+```python
+>>> f1_metric = datasets.load_metric("f1")
+>>> results = f1_metric.compute(references=[0, 1, 0, 1, 0], predictions=[0, 0, 1, 1, 0], pos_label=0)
+>>> print(round(results['f1'], 2))
+0.67
+```
+
+Example 3-The same simple binary example as in Example 1, but with `sample_weight` included.
+```python
+>>> f1_metric = datasets.load_metric("f1")
+>>> results = f1_metric.compute(references=[0, 1, 0, 1, 0], predictions=[0, 0, 1, 1, 0], sample_weight=[0.9, 0.5, 3.9, 1.2, 0.3])
+>>> print(round(results['f1'], 2))
+0.35
+```
+
+Example 4-A multiclass example, with different values for the `average` input.
+```python
+>>> predictions = [0, 2, 1, 0, 0, 1]
+>>> references = [0, 1, 2, 0, 1, 2]
+>>> results = f1_metric.compute(predictions=predictions, references=references, average="macro")
+>>> print(round(results['f1'], 2))
+0.27
+>>> results = f1_metric.compute(predictions=predictions, references=references, average="micro")
+>>> print(round(results['f1'], 2))
+0.33
+>>> results = f1_metric.compute(predictions=predictions, references=references, average="weighted")
+>>> print(round(results['f1'], 2))
+0.27
+>>> results = f1_metric.compute(predictions=predictions, references=references, average=None)
+>>> print(results)
+{'f1': array([0.8, 0. , 0. ])}
+```
+
+
+## Limitations and Bias
+
+
+
+## Citation(s)
+```bibtex
+@article{scikit-learn,
+ title={Scikit-learn: Machine Learning in {P}ython},
+ author={Pedregosa, F. and Varoquaux, G. and Gramfort, A. and Michel, V.
+ and Thirion, B. and Grisel, O. and Blondel, M. and Prettenhofer, P.
+ and Weiss, R. and Dubourg, V. and Vanderplas, J. and Passos, A. and
+ Cournapeau, D. and Brucher, M. and Perrot, M. and Duchesnay, E.},
+ journal={Journal of Machine Learning Research},
+ volume={12},
+ pages={2825--2830},
+ year={2011}
+}
+```
+
+
+## Further References
\ No newline at end of file
diff --git a/metrics/f1/f1.py b/metrics/f1/f1.py
new file mode 100644
index 0000000..513c8fb
--- /dev/null
+++ b/metrics/f1/f1.py
@@ -0,0 +1,123 @@
+# Copyright 2020 The HuggingFace Datasets Authors and the current dataset script contributor.
+#
+# Licensed under the Apache License, Version 2.0 (the "License");
+# you may not use this file except in compliance with the License.
+# You may obtain a copy of the License at
+#
+# http://www.apache.org/licenses/LICENSE-2.0
+#
+# Unless required by applicable law or agreed to in writing, software
+# distributed under the License is distributed on an "AS IS" BASIS,
+# WITHOUT WARRANTIES OR CONDITIONS OF ANY KIND, either express or implied.
+# See the License for the specific language governing permissions and
+# limitations under the License.
+"""F1 metric."""
+
+from sklearn.metrics import f1_score
+
+import datasets
+
+
+_DESCRIPTION = """
+The F1 score is the harmonic mean of the precision and recall. It can be computed with the equation:
+F1 = 2 * (precision * recall) / (precision + recall)
+"""
+
+
+_KWARGS_DESCRIPTION = """
+Args:
+ predictions (`list` of `int`): Predicted labels.
+ references (`list` of `int`): Ground truth labels.
+ labels (`list` of `int`): The set of labels to include when `average` is not set to `'binary'`, and the order of the labels if `average` is `None`. Labels present in the data can be excluded, for example to calculate a multiclass average ignoring a majority negative class. Labels not present in the data will result in 0 components in a macro average. For multilabel targets, labels are column indices. By default, all labels in `predictions` and `references` are used in sorted order. Defaults to None.
+ pos_label (`int`): The class to be considered the positive class, in the case where `average` is set to `binary`. Defaults to 1.
+ average (`string`): This parameter is required for multiclass/multilabel targets. If set to `None`, the scores for each class are returned. Otherwise, this determines the type of averaging performed on the data. Defaults to `'binary'`.
+
+ - 'binary': Only report results for the class specified by `pos_label`. This is applicable only if the classes found in `predictions` and `references` are binary.
+ - 'micro': Calculate metrics globally by counting the total true positives, false negatives and false positives.
+ - 'macro': Calculate metrics for each label, and find their unweighted mean. This does not take label imbalance into account.
+ - 'weighted': Calculate metrics for each label, and find their average weighted by support (the number of true instances for each label). This alters `'macro'` to account for label imbalance. This option can result in an F-score that is not between precision and recall.
+ - 'samples': Calculate metrics for each instance, and find their average (only meaningful for multilabel classification).
+ sample_weight (`list` of `float`): Sample weights Defaults to None.
+
+Returns:
+ f1 (`float` or `array` of `float`): F1 score or list of f1 scores, depending on the value passed to `average`. Minimum possible value is 0. Maximum possible value is 1. Higher f1 scores are better.
+
+Examples:
+
+ Example 1-A simple binary example
+ >>> f1_metric = datasets.load_metric("f1")
+ >>> results = f1_metric.compute(references=[0, 1, 0, 1, 0], predictions=[0, 0, 1, 1, 0])
+ >>> print(results)
+ {'f1': 0.5}
+
+ Example 2-The same simple binary example as in Example 1, but with `pos_label` set to `0`.
+ >>> f1_metric = datasets.load_metric("f1")
+ >>> results = f1_metric.compute(references=[0, 1, 0, 1, 0], predictions=[0, 0, 1, 1, 0], pos_label=0)
+ >>> print(round(results['f1'], 2))
+ 0.67
+
+ Example 3-The same simple binary example as in Example 1, but with `sample_weight` included.
+ >>> f1_metric = datasets.load_metric("f1")
+ >>> results = f1_metric.compute(references=[0, 1, 0, 1, 0], predictions=[0, 0, 1, 1, 0], sample_weight=[0.9, 0.5, 3.9, 1.2, 0.3])
+ >>> print(round(results['f1'], 2))
+ 0.35
+
+ Example 4-A multiclass example, with different values for the `average` input.
+ >>> predictions = [0, 2, 1, 0, 0, 1]
+ >>> references = [0, 1, 2, 0, 1, 2]
+ >>> results = f1_metric.compute(predictions=predictions, references=references, average="macro")
+ >>> print(round(results['f1'], 2))
+ 0.27
+ >>> results = f1_metric.compute(predictions=predictions, references=references, average="micro")
+ >>> print(round(results['f1'], 2))
+ 0.33
+ >>> results = f1_metric.compute(predictions=predictions, references=references, average="weighted")
+ >>> print(round(results['f1'], 2))
+ 0.27
+ >>> results = f1_metric.compute(predictions=predictions, references=references, average=None)
+ >>> print(results)
+ {'f1': array([0.8, 0. , 0. ])}
+"""
+
+
+_CITATION = """
+@article{scikit-learn,
+ title={Scikit-learn: Machine Learning in {P}ython},
+ author={Pedregosa, F. and Varoquaux, G. and Gramfort, A. and Michel, V.
+ and Thirion, B. and Grisel, O. and Blondel, M. and Prettenhofer, P.
+ and Weiss, R. and Dubourg, V. and Vanderplas, J. and Passos, A. and
+ Cournapeau, D. and Brucher, M. and Perrot, M. and Duchesnay, E.},
+ journal={Journal of Machine Learning Research},
+ volume={12},
+ pages={2825--2830},
+ year={2011}
+}
+"""
+
+
+@datasets.utils.file_utils.add_start_docstrings(_DESCRIPTION, _KWARGS_DESCRIPTION)
+class F1(datasets.Metric):
+ def _info(self):
+ return datasets.MetricInfo(
+ description=_DESCRIPTION,
+ citation=_CITATION,
+ inputs_description=_KWARGS_DESCRIPTION,
+ features=datasets.Features(
+ {
+ "predictions": datasets.Sequence(datasets.Value("int32")),
+ "references": datasets.Sequence(datasets.Value("int32")),
+ }
+ if self.config_name == "multilabel"
+ else {
+ "predictions": datasets.Value("int32"),
+ "references": datasets.Value("int32"),
+ }
+ ),
+ reference_urls=["https://scikit-learn.org/stable/modules/generated/sklearn.metrics.f1_score.html"],
+ )
+
+ def _compute(self, predictions, references, labels=None, pos_label=1, average="binary", sample_weight=None):
+ score = f1_score(
+ references, predictions, labels=labels, pos_label=pos_label, average=average, sample_weight=sample_weight
+ )
+ return {"f1": float(score) if score.size == 1 else score}
diff --git a/metrics/frugalscore/README.md b/metrics/frugalscore/README.md
new file mode 100644
index 0000000..977731a
--- /dev/null
+++ b/metrics/frugalscore/README.md
@@ -0,0 +1,105 @@
+# Metric Card for FrugalScore
+
+
+## Metric Description
+FrugalScore is a reference-based metric for Natural Language Generation (NLG) model evaluation. It is based on a distillation approach that allows to learn a fixed, low cost version of any expensive NLG metric, while retaining most of its original performance.
+
+The FrugalScore models are obtained by continuing the pretraining of small models on a synthetic dataset constructed using summarization, backtranslation and denoising models. During the training, the small models learn the internal mapping of the expensive metric, including any similarity function.
+
+## How to use
+
+When loading FrugalScore, you can indicate the model you wish to use to compute the score. The default model is `moussaKam/frugalscore_tiny_bert-base_bert-score`, and a full list of models can be found in the [Limitations and bias](#Limitations-and-bias) section.
+
+```python
+>>> from datasets import load_metric
+>>> frugalscore = load_metric("frugalscore", "moussaKam/frugalscore_medium_bert-base_mover-score")
+```
+
+FrugalScore calculates how good are the predictions given some references, based on a set of scores.
+
+The inputs it takes are:
+
+`predictions`: a list of strings representing the predictions to score.
+
+`references`: a list of string representing the references for each prediction.
+
+Its optional arguments are:
+
+`batch_size`: the batch size for predictions (default value is `32`).
+
+`max_length`: the maximum sequence length (default value is `128`).
+
+`device`: either "gpu" or "cpu" (default value is `None`).
+
+```python
+>>> results = frugalscore.compute(predictions=['hello there', 'huggingface'], references=['hello world', 'hugging face'], batch_size=16, max_length=64, device="gpu")
+```
+
+## Output values
+
+The output of FrugalScore is a dictionary with the list of scores for each prediction-reference pair:
+```python
+{'scores': [0.6307541, 0.6449357]}
+```
+
+### Values from popular papers
+The [original FrugalScore paper](https://arxiv.org/abs/2110.08559) reported that FrugalScore-Tiny retains 97.7/94.7% of the original performance compared to [BertScore](https://huggingface.co/metrics/bertscore) while running 54 times faster and having 84 times less parameters.
+
+## Examples
+
+Maximal values (exact match between `references` and `predictions`):
+
+```python
+>>> from datasets import load_metric
+>>> frugalscore = load_metric("frugalscore")
+>>> results = frugalscore.compute(predictions=['hello world'], references=['hello world'])
+>>> print(results)
+{'scores': [0.9891098]}
+```
+
+Partial values:
+
+```python
+>>> from datasets import load_metric
+>>> frugalscore = load_metric("frugalscore")
+>>> results = frugalscore.compute(predictions=['hello world'], references=['hugging face'])
+>>> print(results)
+{'scores': [0.42482382]}
+```
+
+## Limitations and bias
+
+FrugalScore is based on [BertScore](https://huggingface.co/metrics/bertscore) and [MoverScore](https://arxiv.org/abs/1909.02622), and the models used are based on the original models used for these scores.
+
+The full list of available models for FrugalScore is:
+
+| FrugalScore | Student | Teacher | Method |
+|----------------------------------------------------|-------------|----------------|------------|
+| [moussaKam/frugalscore_tiny_bert-base_bert-score](https://huggingface.co/moussaKam/frugalscore_tiny_bert-base_bert-score) | BERT-tiny | BERT-Base | BERTScore |
+| [moussaKam/frugalscore_small_bert-base_bert-score](https://huggingface.co/moussaKam/frugalscore_small_bert-base_bert-score) | BERT-small | BERT-Base | BERTScore |
+| [moussaKam/frugalscore_medium_bert-base_bert-score](https://huggingface.co/moussaKam/frugalscore_medium_bert-base_bert-score) | BERT-medium | BERT-Base | BERTScore |
+| [moussaKam/frugalscore_tiny_roberta_bert-score](https://huggingface.co/moussaKam/frugalscore_tiny_roberta_bert-score) | BERT-tiny | RoBERTa-Large | BERTScore |
+| [moussaKam/frugalscore_small_roberta_bert-score](https://huggingface.co/moussaKam/frugalscore_small_roberta_bert-score) | BERT-small | RoBERTa-Large | BERTScore |
+| [moussaKam/frugalscore_medium_roberta_bert-score](https://huggingface.co/moussaKam/frugalscore_medium_roberta_bert-score) | BERT-medium | RoBERTa-Large | BERTScore |
+| [moussaKam/frugalscore_tiny_deberta_bert-score](https://huggingface.co/moussaKam/frugalscore_tiny_deberta_bert-score) | BERT-tiny | DeBERTa-XLarge | BERTScore |
+| [moussaKam/frugalscore_small_deberta_bert-score](https://huggingface.co/moussaKam/frugalscore_small_deberta_bert-score) | BERT-small | DeBERTa-XLarge | BERTScore |
+| [moussaKam/frugalscore_medium_deberta_bert-score](https://huggingface.co/moussaKam/frugalscore_medium_deberta_bert-score) | BERT-medium | DeBERTa-XLarge | BERTScore |
+| [moussaKam/frugalscore_tiny_bert-base_mover-score](https://huggingface.co/moussaKam/frugalscore_tiny_bert-base_mover-score) | BERT-tiny | BERT-Base | MoverScore |
+| [moussaKam/frugalscore_small_bert-base_mover-score](https://huggingface.co/moussaKam/frugalscore_small_bert-base_mover-score) | BERT-small | BERT-Base | MoverScore |
+| [moussaKam/frugalscore_medium_bert-base_mover-score](https://huggingface.co/moussaKam/frugalscore_medium_bert-base_mover-score) | BERT-medium | BERT-Base | MoverScore |
+
+Depending on the size of the model picked, the loading time will vary: the `tiny` models will load very quickly, whereas the `medium` ones can take several minutes, depending on your Internet connection.
+
+## Citation
+```bibtex
+@article{eddine2021frugalscore,
+ title={FrugalScore: Learning Cheaper, Lighter and Faster Evaluation Metrics for Automatic Text Generation},
+ author={Eddine, Moussa Kamal and Shang, Guokan and Tixier, Antoine J-P and Vazirgiannis, Michalis},
+ journal={arXiv preprint arXiv:2110.08559},
+ year={2021}
+}
+```
+
+## Further References
+- [Original FrugalScore code](https://github.com/moussaKam/FrugalScore)
+- [FrugalScore paper](https://arxiv.org/abs/2110.08559)
diff --git a/metrics/frugalscore/frugalscore.py b/metrics/frugalscore/frugalscore.py
new file mode 100644
index 0000000..35180b1
--- /dev/null
+++ b/metrics/frugalscore/frugalscore.py
@@ -0,0 +1,116 @@
+# Copyright 2022 The HuggingFace Datasets Authors and the current metric script contributor.
+#
+# Licensed under the Apache License, Version 2.0 (the "License");
+# you may not use this file except in compliance with the License.
+# You may obtain a copy of the License at
+#
+# http://www.apache.org/licenses/LICENSE-2.0
+#
+# Unless required by applicable law or agreed to in writing, software
+# distributed under the License is distributed on an "AS IS" BASIS,
+# WITHOUT WARRANTIES OR CONDITIONS OF ANY KIND, either express or implied.
+# See the License for the specific language governing permissions and
+# limitations under the License.
+"""FrugalScore metric."""
+
+import torch
+from transformers import AutoModelForSequenceClassification, AutoTokenizer, Trainer, TrainingArguments
+
+import datasets
+
+
+_CITATION = """\
+@article{eddine2021frugalscore,
+ title={FrugalScore: Learning Cheaper, Lighter and Faster Evaluation Metrics for Automatic Text Generation},
+ author={Eddine, Moussa Kamal and Shang, Guokan and Tixier, Antoine J-P and Vazirgiannis, Michalis},
+ journal={arXiv preprint arXiv:2110.08559},
+ year={2021}
+}
+"""
+
+_DESCRIPTION = """\
+FrugalScore is a reference-based metric for NLG models evaluation. It is based on a distillation approach that allows to learn a fixed, low cost version of any expensive NLG metric, while retaining most of its original performance.
+"""
+
+
+_KWARGS_DESCRIPTION = """
+Calculates how good are predictions given some references, using certain scores.
+Args:
+ predictions (list of str): list of predictions to score. Each predictions
+ should be a string.
+ references (list of str): list of reference for each prediction. Each
+ reference should be a string.
+ batch_size (int): the batch size for predictions.
+ max_length (int): maximum sequence length.
+ device (str): either gpu or cpu
+Returns:
+ scores (list of int): list of scores.
+Examples:
+ >>> frugalscore = datasets.load_metric("frugalscore")
+ >>> results = frugalscore.compute(predictions=['hello there', 'huggingface'], references=['hello world', 'hugging face'])
+ >>> print([round(s, 3) for s in results["scores"]])
+ [0.631, 0.645]
+"""
+
+
+@datasets.utils.file_utils.add_start_docstrings(_DESCRIPTION, _KWARGS_DESCRIPTION)
+class FRUGALSCORE(datasets.Metric):
+ def _info(self):
+ return datasets.MetricInfo(
+ description=_DESCRIPTION,
+ citation=_CITATION,
+ inputs_description=_KWARGS_DESCRIPTION,
+ features=datasets.Features(
+ {
+ "predictions": datasets.Value("string"),
+ "references": datasets.Value("string"),
+ }
+ ),
+ homepage="https://github.com/moussaKam/FrugalScore",
+ )
+
+ def _download_and_prepare(self, dl_manager):
+ if self.config_name == "default":
+ checkpoint = "moussaKam/frugalscore_tiny_bert-base_bert-score"
+ else:
+ checkpoint = self.config_name
+ self.model = AutoModelForSequenceClassification.from_pretrained(checkpoint)
+ self.tokenizer = AutoTokenizer.from_pretrained(checkpoint)
+
+ def _compute(
+ self,
+ predictions,
+ references,
+ batch_size=32,
+ max_length=128,
+ device=None,
+ ):
+ """Returns the scores"""
+ assert len(predictions) == len(
+ references
+ ), "predictions and references should have the same number of sentences."
+ if device is not None:
+ assert device in ["gpu", "cpu"], "device should be either gpu or cpu."
+ else:
+ device = "gpu" if torch.cuda.is_available() else "cpu"
+ training_args = TrainingArguments(
+ "trainer",
+ fp16=(device == "gpu"),
+ per_device_eval_batch_size=batch_size,
+ report_to="all",
+ no_cuda=(device == "cpu"),
+ log_level="warning",
+ )
+ dataset = {"sentence1": predictions, "sentence2": references}
+ raw_datasets = datasets.Dataset.from_dict(dataset)
+
+ def tokenize_function(data):
+ return self.tokenizer(
+ data["sentence1"], data["sentence2"], max_length=max_length, truncation=True, padding=True
+ )
+
+ tokenized_datasets = raw_datasets.map(tokenize_function, batched=True)
+ tokenized_datasets.remove_columns(["sentence1", "sentence2"])
+ trainer = Trainer(self.model, training_args, tokenizer=self.tokenizer)
+ predictions = trainer.predict(tokenized_datasets)
+ return {"scores": list(predictions.predictions.squeeze(-1))}
diff --git a/metrics/glue/README.md b/metrics/glue/README.md
new file mode 100644
index 0000000..f7f60a7
--- /dev/null
+++ b/metrics/glue/README.md
@@ -0,0 +1,105 @@
+# Metric Card for GLUE
+
+## Metric description
+This metric is used to compute the GLUE evaluation metric associated to each [GLUE dataset](https://huggingface.co/datasets/glue).
+
+GLUE, the General Language Understanding Evaluation benchmark is a collection of resources for training, evaluating, and analyzing natural language understanding systems.
+
+## How to use
+
+There are two steps: (1) loading the GLUE metric relevant to the subset of the GLUE dataset being used for evaluation; and (2) calculating the metric.
+
+1. **Loading the relevant GLUE metric** : the subsets of GLUE are the following: `sst2`, `mnli`, `mnli_mismatched`, `mnli_matched`, `qnli`, `rte`, `wnli`, `cola`,`stsb`, `mrpc`, `qqp`, and `hans`.
+
+More information about the different subsets of the GLUE dataset can be found on the [GLUE dataset page](https://huggingface.co/datasets/glue).
+
+2. **Calculating the metric**: the metric takes two inputs : one list with the predictions of the model to score and one lists of references for each translation.
+
+```python
+from datasets import load_metric
+glue_metric = load_metric('glue', 'sst2')
+references = [0, 1]
+predictions = [0, 1]
+results = glue_metric.compute(predictions=predictions, references=references)
+```
+## Output values
+
+The output of the metric depends on the GLUE subset chosen, consisting of a dictionary that contains one or several of the following metrics:
+
+`accuracy`: the proportion of correct predictions among the total number of cases processed, with a range between 0 and 1 (see [accuracy](https://huggingface.co/metrics/accuracy) for more information).
+
+`f1`: the harmonic mean of the precision and recall (see [F1 score](https://huggingface.co/metrics/f1) for more information). Its range is 0-1 -- its lowest possible value is 0, if either the precision or the recall is 0, and its highest possible value is 1.0, which means perfect precision and recall.
+
+`pearson`: a measure of the linear relationship between two datasets (see [Pearson correlation](https://huggingface.co/metrics/pearsonr) for more information). Its range is between -1 and +1, with 0 implying no correlation, and -1/+1 implying an exact linear relationship. Positive correlations imply that as x increases, so does y, whereas negative correlations imply that as x increases, y decreases.
+
+`spearmanr`: a nonparametric measure of the monotonicity of the relationship between two datasets(see [Spearman Correlation](https://huggingface.co/metrics/spearmanr) for more information). `spearmanr` has the same range as `pearson`.
+
+`matthews_correlation`: a measure of the quality of binary and multiclass classifications (see [Matthews Correlation](https://huggingface.co/metrics/matthews_correlation) for more information). Its range of values is between -1 and +1, where a coefficient of +1 represents a perfect prediction, 0 an average random prediction and -1 an inverse prediction.
+
+The `cola` subset returns `matthews_correlation`, the `stsb` subset returns `pearson` and `spearmanr`, the `mrpc` and `qqp` subsets return both `accuracy` and `f1`, and all other subsets of GLUE return only accuracy.
+
+### Values from popular papers
+The [original GLUE paper](https://huggingface.co/datasets/glue) reported average scores ranging from 58 to 64%, depending on the model used (with all evaluation values scaled by 100 to make computing the average possible).
+
+For more recent model performance, see the [dataset leaderboard](https://paperswithcode.com/dataset/glue).
+
+## Examples
+
+Maximal values for the MRPC subset (which outputs `accuracy` and `f1`):
+
+```python
+from datasets import load_metric
+glue_metric = load_metric('glue', 'mrpc') # 'mrpc' or 'qqp'
+references = [0, 1]
+predictions = [0, 1]
+results = glue_metric.compute(predictions=predictions, references=references)
+print(results)
+{'accuracy': 1.0, 'f1': 1.0}
+```
+
+Minimal values for the STSB subset (which outputs `pearson` and `spearmanr`):
+
+```python
+from datasets import load_metric
+glue_metric = load_metric('glue', 'stsb')
+references = [0., 1., 2., 3., 4., 5.]
+predictions = [-10., -11., -12., -13., -14., -15.]
+results = glue_metric.compute(predictions=predictions, references=references)
+print(results)
+{'pearson': -1.0, 'spearmanr': -1.0}
+```
+
+Partial match for the COLA subset (which outputs `matthews_correlation`)
+
+```python
+from datasets import load_metric
+glue_metric = load_metric('glue', 'cola')
+references = [0, 1]
+predictions = [1, 1]
+results = glue_metric.compute(predictions=predictions, references=references)
+results
+{'matthews_correlation': 0.0}
+```
+
+## Limitations and bias
+This metric works only with datasets that have the same format as the [GLUE dataset](https://huggingface.co/datasets/glue).
+
+While the GLUE dataset is meant to represent "General Language Understanding", the tasks represented in it are not necessarily representative of language understanding, and should not be interpreted as such.
+
+Also, while the GLUE subtasks were considered challenging during its creation in 2019, they are no longer considered as such given the impressive progress made since then. A more complex (or "stickier") version of it, called [SuperGLUE](https://huggingface.co/datasets/super_glue), was subsequently created.
+
+## Citation
+
+```bibtex
+ @inproceedings{wang2019glue,
+ title={{GLUE}: A Multi-Task Benchmark and Analysis Platform for Natural Language Understanding},
+ author={Wang, Alex and Singh, Amanpreet and Michael, Julian and Hill, Felix and Levy, Omer and Bowman, Samuel R.},
+ note={In the Proceedings of ICLR.},
+ year={2019}
+}
+```
+
+## Further References
+
+- [GLUE benchmark homepage](https://gluebenchmark.com/)
+- [Fine-tuning a model with the Trainer API](https://huggingface.co/course/chapter3/3?)
diff --git a/metrics/glue/glue.py b/metrics/glue/glue.py
new file mode 100644
index 0000000..42f1738
--- /dev/null
+++ b/metrics/glue/glue.py
@@ -0,0 +1,155 @@
+# Copyright 2020 The HuggingFace Datasets Authors.
+#
+# Licensed under the Apache License, Version 2.0 (the "License");
+# you may not use this file except in compliance with the License.
+# You may obtain a copy of the License at
+#
+# http://www.apache.org/licenses/LICENSE-2.0
+#
+# Unless required by applicable law or agreed to in writing, software
+# distributed under the License is distributed on an "AS IS" BASIS,
+# WITHOUT WARRANTIES OR CONDITIONS OF ANY KIND, either express or implied.
+# See the License for the specific language governing permissions and
+# limitations under the License.
+""" GLUE benchmark metric. """
+
+from scipy.stats import pearsonr, spearmanr
+from sklearn.metrics import f1_score, matthews_corrcoef
+
+import datasets
+
+
+_CITATION = """\
+@inproceedings{wang2019glue,
+ title={{GLUE}: A Multi-Task Benchmark and Analysis Platform for Natural Language Understanding},
+ author={Wang, Alex and Singh, Amanpreet and Michael, Julian and Hill, Felix and Levy, Omer and Bowman, Samuel R.},
+ note={In the Proceedings of ICLR.},
+ year={2019}
+}
+"""
+
+_DESCRIPTION = """\
+GLUE, the General Language Understanding Evaluation benchmark
+(https://gluebenchmark.com/) is a collection of resources for training,
+evaluating, and analyzing natural language understanding systems.
+"""
+
+_KWARGS_DESCRIPTION = """
+Compute GLUE evaluation metric associated to each GLUE dataset.
+Args:
+ predictions: list of predictions to score.
+ Each translation should be tokenized into a list of tokens.
+ references: list of lists of references for each translation.
+ Each reference should be tokenized into a list of tokens.
+Returns: depending on the GLUE subset, one or several of:
+ "accuracy": Accuracy
+ "f1": F1 score
+ "pearson": Pearson Correlation
+ "spearmanr": Spearman Correlation
+ "matthews_correlation": Matthew Correlation
+Examples:
+
+ >>> glue_metric = datasets.load_metric('glue', 'sst2') # 'sst2' or any of ["mnli", "mnli_mismatched", "mnli_matched", "qnli", "rte", "wnli", "hans"]
+ >>> references = [0, 1]
+ >>> predictions = [0, 1]
+ >>> results = glue_metric.compute(predictions=predictions, references=references)
+ >>> print(results)
+ {'accuracy': 1.0}
+
+ >>> glue_metric = datasets.load_metric('glue', 'mrpc') # 'mrpc' or 'qqp'
+ >>> references = [0, 1]
+ >>> predictions = [0, 1]
+ >>> results = glue_metric.compute(predictions=predictions, references=references)
+ >>> print(results)
+ {'accuracy': 1.0, 'f1': 1.0}
+
+ >>> glue_metric = datasets.load_metric('glue', 'stsb')
+ >>> references = [0., 1., 2., 3., 4., 5.]
+ >>> predictions = [0., 1., 2., 3., 4., 5.]
+ >>> results = glue_metric.compute(predictions=predictions, references=references)
+ >>> print({"pearson": round(results["pearson"], 2), "spearmanr": round(results["spearmanr"], 2)})
+ {'pearson': 1.0, 'spearmanr': 1.0}
+
+ >>> glue_metric = datasets.load_metric('glue', 'cola')
+ >>> references = [0, 1]
+ >>> predictions = [0, 1]
+ >>> results = glue_metric.compute(predictions=predictions, references=references)
+ >>> print(results)
+ {'matthews_correlation': 1.0}
+"""
+
+
+def simple_accuracy(preds, labels):
+ return float((preds == labels).mean())
+
+
+def acc_and_f1(preds, labels):
+ acc = simple_accuracy(preds, labels)
+ f1 = float(f1_score(y_true=labels, y_pred=preds))
+ return {
+ "accuracy": acc,
+ "f1": f1,
+ }
+
+
+def pearson_and_spearman(preds, labels):
+ pearson_corr = float(pearsonr(preds, labels)[0])
+ spearman_corr = float(spearmanr(preds, labels)[0])
+ return {
+ "pearson": pearson_corr,
+ "spearmanr": spearman_corr,
+ }
+
+
+@datasets.utils.file_utils.add_start_docstrings(_DESCRIPTION, _KWARGS_DESCRIPTION)
+class Glue(datasets.Metric):
+ def _info(self):
+ if self.config_name not in [
+ "sst2",
+ "mnli",
+ "mnli_mismatched",
+ "mnli_matched",
+ "cola",
+ "stsb",
+ "mrpc",
+ "qqp",
+ "qnli",
+ "rte",
+ "wnli",
+ "hans",
+ ]:
+ raise KeyError(
+ "You should supply a configuration name selected in "
+ '["sst2", "mnli", "mnli_mismatched", "mnli_matched", '
+ '"cola", "stsb", "mrpc", "qqp", "qnli", "rte", "wnli", "hans"]'
+ )
+ return datasets.MetricInfo(
+ description=_DESCRIPTION,
+ citation=_CITATION,
+ inputs_description=_KWARGS_DESCRIPTION,
+ features=datasets.Features(
+ {
+ "predictions": datasets.Value("int64" if self.config_name != "stsb" else "float32"),
+ "references": datasets.Value("int64" if self.config_name != "stsb" else "float32"),
+ }
+ ),
+ codebase_urls=[],
+ reference_urls=[],
+ format="numpy",
+ )
+
+ def _compute(self, predictions, references):
+ if self.config_name == "cola":
+ return {"matthews_correlation": matthews_corrcoef(references, predictions)}
+ elif self.config_name == "stsb":
+ return pearson_and_spearman(predictions, references)
+ elif self.config_name in ["mrpc", "qqp"]:
+ return acc_and_f1(predictions, references)
+ elif self.config_name in ["sst2", "mnli", "mnli_mismatched", "mnli_matched", "qnli", "rte", "wnli", "hans"]:
+ return {"accuracy": simple_accuracy(predictions, references)}
+ else:
+ raise KeyError(
+ "You should supply a configuration name selected in "
+ '["sst2", "mnli", "mnli_mismatched", "mnli_matched", '
+ '"cola", "stsb", "mrpc", "qqp", "qnli", "rte", "wnli", "hans"]'
+ )
diff --git a/metrics/google_bleu/README.md b/metrics/google_bleu/README.md
new file mode 100644
index 0000000..08659e6
--- /dev/null
+++ b/metrics/google_bleu/README.md
@@ -0,0 +1,186 @@
+# Metric Card for Google BLEU (GLEU)
+
+
+## Metric Description
+The BLEU score has some undesirable properties when used for single
+sentences, as it was designed to be a corpus measure. The Google BLEU score, also known as GLEU score, is designed to limit these undesirable properties when used for single sentences.
+
+To calculate this score, all sub-sequences of 1, 2, 3 or 4 tokens in output and target sequence (n-grams) are recorded. The precision and recall, described below, are then computed.
+
+- **precision:** the ratio of the number of matching n-grams to the number of total n-grams in the generated output sequence
+- **recall:** the ratio of the number of matching n-grams to the number of total n-grams in the target (ground truth) sequence
+
+The minimum value of precision and recall is then returned as the score.
+
+
+## Intended Uses
+This metric is generally used to evaluate machine translation models. It is especially used when scores of individual (prediction, reference) sentence pairs are needed, as opposed to when averaging over the (prediction, reference) scores for a whole corpus. That being said, it can also be used when averaging over the scores for a whole corpus.
+
+Because it performs better on individual sentence pairs as compared to BLEU, Google BLEU has also been used in RL experiments.
+
+## How to Use
+At minimum, this metric takes predictions and references:
+```python
+>>> sentence1 = "the cat sat on the mat".split()
+>>> sentence2 = "the cat ate the mat".split()
+>>> google_bleu = datasets.load_metric("google_bleu")
+>>> result = google_bleu.compute(predictions=[sentence1], references=[[sentence2]])
+>>> print(result)
+{'google_bleu': 0.3333333333333333}
+```
+
+### Inputs
+- **predictions** (list of list of str): list of translations to score. Each translation should be tokenized into a list of tokens.
+- **references** (list of list of list of str): list of lists of references for each translation. Each reference should be tokenized into a list of tokens.
+- **min_len** (int): The minimum order of n-gram this function should extract. Defaults to 1.
+- **max_len** (int): The maximum order of n-gram this function should extract. Defaults to 4.
+
+### Output Values
+This metric returns the following in a dict:
+- **google_bleu** (float): google_bleu score
+
+The output format is as follows:
+```python
+{'google_bleu': google_bleu score}
+```
+
+This metric can take on values from 0 to 1, inclusive. Higher scores are better, with 0 indicating no matches, and 1 indicating a perfect match.
+
+Note that this score is symmetrical when switching output and target. This means that, given two sentences, `sentence1` and `sentence2`, whatever score is output when `sentence1` is the predicted sentence and `sencence2` is the reference sentence will be the same as when the sentences are swapped and `sentence2` is the predicted sentence while `sentence1` is the reference sentence. In code, this looks like:
+
+```python
+sentence1 = "the cat sat on the mat".split()
+sentence2 = "the cat ate the mat".split()
+google_bleu = datasets.load_metric("google_bleu")
+result_a = google_bleu.compute(predictions=[sentence1], references=[[sentence2]])
+result_b = google_bleu.compute(predictions=[sentence2], references=[[sentence1]])
+print(result_a == result_b)
+>>> True
+```
+
+#### Values from Popular Papers
+
+
+### Examples
+Example with one reference per sample:
+```python
+>>> hyp1 = ['It', 'is', 'a', 'guide', 'to', 'action', 'which',
+... 'ensures', 'that', 'the', 'rubber', 'duck', 'always',
+... 'disobeys', 'the', 'commands', 'of', 'the', 'cat']
+>>> ref1a = ['It', 'is', 'the', 'guiding', 'principle', 'which',
+... 'guarantees', 'the', 'rubber', 'duck', 'forces', 'never',
+... 'being', 'under', 'the', 'command', 'of', 'the', 'cat']
+
+>>> hyp2 = ['he', 'read', 'the', 'book', 'because', 'he', 'was',
+... 'interested', 'in', 'world', 'history']
+>>> ref2a = ['he', 'was', 'interested', 'in', 'world', 'history',
+... 'because', 'he', 'read', 'the', 'book']
+
+>>> list_of_references = [[ref1a], [ref2a]]
+>>> hypotheses = [hyp1, hyp2]
+>>> google_bleu = datasets.load_metric("google_bleu")
+>>> results = google_bleu.compute(predictions=hypotheses, references=list_of_references)
+>>> print(round(results["google_bleu"], 2))
+0.44
+```
+
+Example with multiple references for the first sample:
+```python
+>>> hyp1 = ['It', 'is', 'a', 'guide', 'to', 'action', 'which',
+... 'ensures', 'that', 'the', 'rubber', 'duck', 'always',
+... 'disobeys', 'the', 'commands', 'of', 'the', 'cat']
+>>> ref1a = ['It', 'is', 'the', 'guiding', 'principle', 'which',
+... 'guarantees', 'the', 'rubber', 'duck', 'forces', 'never',
+... 'being', 'under', 'the', 'command', 'of', 'the', 'cat']
+>>> ref1b = ['It', 'is', 'a', 'guide', 'to', 'action', 'that',
+... 'ensures', 'that', 'the', 'rubber', 'duck', 'will', 'never',
+... 'heed', 'the', 'cat', 'commands']
+>>> ref1c = ['It', 'is', 'the', 'practical', 'guide', 'for', 'the',
+... 'rubber', 'duck', 'army', 'never', 'to', 'heed', 'the', 'directions',
+... 'of', 'the', 'cat']
+
+>>> hyp2 = ['he', 'read', 'the', 'book', 'because', 'he', 'was',
+... 'interested', 'in', 'world', 'history']
+>>> ref2a = ['he', 'was', 'interested', 'in', 'world', 'history',
+... 'because', 'he', 'read', 'the', 'book']
+
+>>> list_of_references = [[ref1a, ref1b, ref1c], [ref2a]]
+>>> hypotheses = [hyp1, hyp2]
+>>> google_bleu = datasets.load_metric("google_bleu")
+>>> results = google_bleu.compute(predictions=hypotheses, references=list_of_references)
+>>> print(round(results["google_bleu"], 2))
+0.61
+```
+
+Example with multiple references for the first sample, and with `min_len` adjusted to `2`, instead of the default `1`:
+```python
+>>> hyp1 = ['It', 'is', 'a', 'guide', 'to', 'action', 'which',
+... 'ensures', 'that', 'the', 'rubber', 'duck', 'always',
+... 'disobeys', 'the', 'commands', 'of', 'the', 'cat']
+>>> ref1a = ['It', 'is', 'the', 'guiding', 'principle', 'which',
+... 'guarantees', 'the', 'rubber', 'duck', 'forces', 'never',
+... 'being', 'under', 'the', 'command', 'of', 'the', 'cat']
+>>> ref1b = ['It', 'is', 'a', 'guide', 'to', 'action', 'that',
+... 'ensures', 'that', 'the', 'rubber', 'duck', 'will', 'never',
+... 'heed', 'the', 'cat', 'commands']
+>>> ref1c = ['It', 'is', 'the', 'practical', 'guide', 'for', 'the',
+... 'rubber', 'duck', 'army', 'never', 'to', 'heed', 'the', 'directions',
+... 'of', 'the', 'cat']
+
+>>> hyp2 = ['he', 'read', 'the', 'book', 'because', 'he', 'was',
+... 'interested', 'in', 'world', 'history']
+>>> ref2a = ['he', 'was', 'interested', 'in', 'world', 'history',
+... 'because', 'he', 'read', 'the', 'book']
+
+>>> list_of_references = [[ref1a, ref1b, ref1c], [ref2a]]
+>>> hypotheses = [hyp1, hyp2]
+>>> google_bleu = datasets.load_metric("google_bleu")
+>>> results = google_bleu.compute(predictions=hypotheses, references=list_of_references, min_len=2)
+>>> print(round(results["google_bleu"], 2))
+0.53
+```
+
+Example with multiple references for the first sample, with `min_len` adjusted to `2`, instead of the default `1`, and `max_len` adjusted to `6` instead of the default `4`:
+```python
+>>> hyp1 = ['It', 'is', 'a', 'guide', 'to', 'action', 'which',
+... 'ensures', 'that', 'the', 'rubber', 'duck', 'always',
+... 'disobeys', 'the', 'commands', 'of', 'the', 'cat']
+>>> ref1a = ['It', 'is', 'the', 'guiding', 'principle', 'which',
+... 'guarantees', 'the', 'rubber', 'duck', 'forces', 'never',
+... 'being', 'under', 'the', 'command', 'of', 'the', 'cat']
+>>> ref1b = ['It', 'is', 'a', 'guide', 'to', 'action', 'that',
+... 'ensures', 'that', 'the', 'rubber', 'duck', 'will', 'never',
+... 'heed', 'the', 'cat', 'commands']
+>>> ref1c = ['It', 'is', 'the', 'practical', 'guide', 'for', 'the',
+... 'rubber', 'duck', 'army', 'never', 'to', 'heed', 'the', 'directions',
+... 'of', 'the', 'cat']
+
+>>> hyp2 = ['he', 'read', 'the', 'book', 'because', 'he', 'was',
+... 'interested', 'in', 'world', 'history']
+>>> ref2a = ['he', 'was', 'interested', 'in', 'world', 'history',
+... 'because', 'he', 'read', 'the', 'book']
+
+>>> list_of_references = [[ref1a, ref1b, ref1c], [ref2a]]
+>>> hypotheses = [hyp1, hyp2]
+>>> google_bleu = datasets.load_metric("google_bleu")
+>>> results = google_bleu.compute(predictions=hypotheses,references=list_of_references, min_len=2, max_len=6)
+>>> print(round(results["google_bleu"], 2))
+0.4
+```
+
+## Limitations and Bias
+
+
+## Citation
+```bibtex
+@misc{wu2016googles,
+title={Google's Neural Machine Translation System: Bridging the Gap between Human and Machine Translation},
+author={Yonghui Wu and Mike Schuster and Zhifeng Chen and Quoc V. Le and Mohammad Norouzi and Wolfgang Macherey and Maxim Krikun and Yuan Cao and Qin Gao and Klaus Macherey and Jeff Klingner and Apurva Shah and Melvin Johnson and Xiaobing Liu and Łukasz Kaiser and Stephan Gouws and Yoshikiyo Kato and Taku Kudo and Hideto Kazawa and Keith Stevens and George Kurian and Nishant Patil and Wei Wang and Cliff Young and Jason Smith and Jason Riesa and Alex Rudnick and Oriol Vinyals and Greg Corrado and Macduff Hughes and Jeffrey Dean},
+year={2016},
+eprint={1609.08144},
+archivePrefix={arXiv},
+primaryClass={cs.CL}
+}
+```
+## Further References
+- This Hugging Face implementation uses the [nltk.translate.gleu_score implementation](https://www.nltk.org/_modules/nltk/translate/gleu_score.html)
\ No newline at end of file
diff --git a/metrics/google_bleu/google_bleu.py b/metrics/google_bleu/google_bleu.py
new file mode 100644
index 0000000..09b6660
--- /dev/null
+++ b/metrics/google_bleu/google_bleu.py
@@ -0,0 +1,203 @@
+# Copyright 2020 The HuggingFace Datasets Authors.
+#
+# Licensed under the Apache License, Version 2.0 (the "License");
+# you may not use this file except in compliance with the License.
+# You may obtain a copy of the License at
+#
+# http://www.apache.org/licenses/LICENSE-2.0
+#
+# Unless required by applicable law or agreed to in writing, software
+# distributed under the License is distributed on an "AS IS" BASIS,
+# WITHOUT WARRANTIES OR CONDITIONS OF ANY KIND, either express or implied.
+# See the License for the specific language governing permissions and
+# limitations under the License.
+""" Google BLEU (aka GLEU) metric. """
+
+from typing import Dict, List
+
+from nltk.translate import gleu_score
+
+import datasets
+from datasets import MetricInfo
+
+
+_CITATION = """\
+@misc{wu2016googles,
+ title={Google's Neural Machine Translation System: Bridging the Gap between Human and Machine Translation},
+ author={Yonghui Wu and Mike Schuster and Zhifeng Chen and Quoc V. Le and Mohammad Norouzi and Wolfgang Macherey
+ and Maxim Krikun and Yuan Cao and Qin Gao and Klaus Macherey and Jeff Klingner and Apurva Shah and Melvin
+ Johnson and Xiaobing Liu and Łukasz Kaiser and Stephan Gouws and Yoshikiyo Kato and Taku Kudo and Hideto
+ Kazawa and Keith Stevens and George Kurian and Nishant Patil and Wei Wang and Cliff Young and
+ Jason Smith and Jason Riesa and Alex Rudnick and Oriol Vinyals and Greg Corrado and Macduff Hughes
+ and Jeffrey Dean},
+ year={2016},
+ eprint={1609.08144},
+ archivePrefix={arXiv},
+ primaryClass={cs.CL}
+}
+"""
+
+_DESCRIPTION = """\
+The BLEU score has some undesirable properties when used for single
+sentences, as it was designed to be a corpus measure. We therefore
+use a slightly different score for our RL experiments which we call
+the 'GLEU score'. For the GLEU score, we record all sub-sequences of
+1, 2, 3 or 4 tokens in output and target sequence (n-grams). We then
+compute a recall, which is the ratio of the number of matching n-grams
+to the number of total n-grams in the target (ground truth) sequence,
+and a precision, which is the ratio of the number of matching n-grams
+to the number of total n-grams in the generated output sequence. Then
+GLEU score is simply the minimum of recall and precision. This GLEU
+score's range is always between 0 (no matches) and 1 (all match) and
+it is symmetrical when switching output and target. According to
+our experiments, GLEU score correlates quite well with the BLEU
+metric on a corpus level but does not have its drawbacks for our per
+sentence reward objective.
+"""
+
+_KWARGS_DESCRIPTION = """\
+Computes corpus-level Google BLEU (GLEU) score of translated segments against one or more references.
+Instead of averaging the sentence level GLEU scores (i.e. macro-average precision), Wu et al. (2016) sum up the matching
+tokens and the max of hypothesis and reference tokens for each sentence, then compute using the aggregate values.
+
+Args:
+ predictions (list of str): list of translations to score.
+ Each translation should be tokenized into a list of tokens.
+ references (list of list of str): list of lists of references for each translation.
+ Each reference should be tokenized into a list of tokens.
+ min_len (int): The minimum order of n-gram this function should extract. Defaults to 1.
+ max_len (int): The maximum order of n-gram this function should extract. Defaults to 4.
+
+Returns:
+ 'google_bleu': google_bleu score
+
+Examples:
+ Example 1:
+ >>> hyp1 = ['It', 'is', 'a', 'guide', 'to', 'action', 'which',
+ ... 'ensures', 'that', 'the', 'rubber', 'duck', 'always',
+ ... 'disobeys', 'the', 'commands', 'of', 'the', 'cat']
+ >>> ref1a = ['It', 'is', 'the', 'guiding', 'principle', 'which',
+ ... 'guarantees', 'the', 'rubber', 'duck', 'forces', 'never',
+ ... 'being', 'under', 'the', 'command', 'of', 'the', 'cat']
+
+ >>> hyp2 = ['he', 'read', 'the', 'book', 'because', 'he', 'was',
+ ... 'interested', 'in', 'world', 'history']
+ >>> ref2a = ['he', 'was', 'interested', 'in', 'world', 'history',
+ ... 'because', 'he', 'read', 'the', 'book']
+
+ >>> list_of_references = [[ref1a], [ref2a]]
+ >>> hypotheses = [hyp1, hyp2]
+ >>> google_bleu = datasets.load_metric("google_bleu")
+ >>> results = google_bleu.compute(predictions=hypotheses, references=list_of_references)
+ >>> print(round(results["google_bleu"], 2))
+ 0.44
+
+ Example 2:
+ >>> hyp1 = ['It', 'is', 'a', 'guide', 'to', 'action', 'which',
+ ... 'ensures', 'that', 'the', 'rubber', 'duck', 'always',
+ ... 'disobeys', 'the', 'commands', 'of', 'the', 'cat']
+ >>> ref1a = ['It', 'is', 'the', 'guiding', 'principle', 'which',
+ ... 'guarantees', 'the', 'rubber', 'duck', 'forces', 'never',
+ ... 'being', 'under', 'the', 'command', 'of', 'the', 'cat']
+ >>> ref1b = ['It', 'is', 'a', 'guide', 'to', 'action', 'that',
+ ... 'ensures', 'that', 'the', 'rubber', 'duck', 'will', 'never',
+ ... 'heed', 'the', 'cat', 'commands']
+ >>> ref1c = ['It', 'is', 'the', 'practical', 'guide', 'for', 'the',
+ ... 'rubber', 'duck', 'army', 'never', 'to', 'heed', 'the', 'directions',
+ ... 'of', 'the', 'cat']
+
+ >>> hyp2 = ['he', 'read', 'the', 'book', 'because', 'he', 'was',
+ ... 'interested', 'in', 'world', 'history']
+ >>> ref2a = ['he', 'was', 'interested', 'in', 'world', 'history',
+ ... 'because', 'he', 'read', 'the', 'book']
+
+ >>> list_of_references = [[ref1a, ref1b, ref1c], [ref2a]]
+ >>> hypotheses = [hyp1, hyp2]
+ >>> google_bleu = datasets.load_metric("google_bleu")
+ >>> results = google_bleu.compute(predictions=hypotheses, references=list_of_references)
+ >>> print(round(results["google_bleu"], 2))
+ 0.61
+
+ Example 3:
+ >>> hyp1 = ['It', 'is', 'a', 'guide', 'to', 'action', 'which',
+ ... 'ensures', 'that', 'the', 'rubber', 'duck', 'always',
+ ... 'disobeys', 'the', 'commands', 'of', 'the', 'cat']
+ >>> ref1a = ['It', 'is', 'the', 'guiding', 'principle', 'which',
+ ... 'guarantees', 'the', 'rubber', 'duck', 'forces', 'never',
+ ... 'being', 'under', 'the', 'command', 'of', 'the', 'cat']
+ >>> ref1b = ['It', 'is', 'a', 'guide', 'to', 'action', 'that',
+ ... 'ensures', 'that', 'the', 'rubber', 'duck', 'will', 'never',
+ ... 'heed', 'the', 'cat', 'commands']
+ >>> ref1c = ['It', 'is', 'the', 'practical', 'guide', 'for', 'the',
+ ... 'rubber', 'duck', 'army', 'never', 'to', 'heed', 'the', 'directions',
+ ... 'of', 'the', 'cat']
+
+ >>> hyp2 = ['he', 'read', 'the', 'book', 'because', 'he', 'was',
+ ... 'interested', 'in', 'world', 'history']
+ >>> ref2a = ['he', 'was', 'interested', 'in', 'world', 'history',
+ ... 'because', 'he', 'read', 'the', 'book']
+
+ >>> list_of_references = [[ref1a, ref1b, ref1c], [ref2a]]
+ >>> hypotheses = [hyp1, hyp2]
+ >>> google_bleu = datasets.load_metric("google_bleu")
+ >>> results = google_bleu.compute(predictions=hypotheses, references=list_of_references, min_len=2)
+ >>> print(round(results["google_bleu"], 2))
+ 0.53
+
+ Example 4:
+ >>> hyp1 = ['It', 'is', 'a', 'guide', 'to', 'action', 'which',
+ ... 'ensures', 'that', 'the', 'rubber', 'duck', 'always',
+ ... 'disobeys', 'the', 'commands', 'of', 'the', 'cat']
+ >>> ref1a = ['It', 'is', 'the', 'guiding', 'principle', 'which',
+ ... 'guarantees', 'the', 'rubber', 'duck', 'forces', 'never',
+ ... 'being', 'under', 'the', 'command', 'of', 'the', 'cat']
+ >>> ref1b = ['It', 'is', 'a', 'guide', 'to', 'action', 'that',
+ ... 'ensures', 'that', 'the', 'rubber', 'duck', 'will', 'never',
+ ... 'heed', 'the', 'cat', 'commands']
+ >>> ref1c = ['It', 'is', 'the', 'practical', 'guide', 'for', 'the',
+ ... 'rubber', 'duck', 'army', 'never', 'to', 'heed', 'the', 'directions',
+ ... 'of', 'the', 'cat']
+
+ >>> hyp2 = ['he', 'read', 'the', 'book', 'because', 'he', 'was',
+ ... 'interested', 'in', 'world', 'history']
+ >>> ref2a = ['he', 'was', 'interested', 'in', 'world', 'history',
+ ... 'because', 'he', 'read', 'the', 'book']
+
+ >>> list_of_references = [[ref1a, ref1b, ref1c], [ref2a]]
+ >>> hypotheses = [hyp1, hyp2]
+ >>> google_bleu = datasets.load_metric("google_bleu")
+ >>> results = google_bleu.compute(predictions=hypotheses,references=list_of_references, min_len=2, max_len=6)
+ >>> print(round(results["google_bleu"], 2))
+ 0.4
+"""
+
+
+@datasets.utils.file_utils.add_start_docstrings(_DESCRIPTION, _KWARGS_DESCRIPTION)
+class GoogleBleu(datasets.Metric):
+ def _info(self) -> MetricInfo:
+ return datasets.MetricInfo(
+ description=_DESCRIPTION,
+ citation=_CITATION,
+ inputs_description=_KWARGS_DESCRIPTION,
+ features=datasets.Features(
+ {
+ "predictions": datasets.Sequence(datasets.Value("string", id="token"), id="sequence"),
+ "references": datasets.Sequence(
+ datasets.Sequence(datasets.Value("string", id="token"), id="sequence"), id="references"
+ ),
+ }
+ ),
+ )
+
+ def _compute(
+ self,
+ predictions: List[List[List[str]]],
+ references: List[List[str]],
+ min_len: int = 1,
+ max_len: int = 4,
+ ) -> Dict[str, float]:
+ return {
+ "google_bleu": gleu_score.corpus_gleu(
+ list_of_references=references, hypotheses=predictions, min_len=min_len, max_len=max_len
+ )
+ }
diff --git a/metrics/indic_glue/README.md b/metrics/indic_glue/README.md
new file mode 100644
index 0000000..e3c768d
--- /dev/null
+++ b/metrics/indic_glue/README.md
@@ -0,0 +1,96 @@
+# Metric Card for IndicGLUE
+
+## Metric description
+This metric is used to compute the evaluation metric for the [IndicGLUE dataset](https://huggingface.co/datasets/indic_glue).
+
+IndicGLUE is a natural language understanding benchmark for Indian languages. It contains a wide variety of tasks and covers 11 major Indian languages - Assamese (`as`), Bengali (`bn`), Gujarati (`gu`), Hindi (`hi`), Kannada (`kn`), Malayalam (`ml`), Marathi(`mr`), Oriya(`or`), Panjabi (`pa`), Tamil(`ta`) and Telugu (`te`).
+
+## How to use
+
+There are two steps: (1) loading the IndicGLUE metric relevant to the subset of the dataset being used for evaluation; and (2) calculating the metric.
+
+1. **Loading the relevant IndicGLUE metric** : the subsets of IndicGLUE are the following: `wnli`, `copa`, `sna`, `csqa`, `wstp`, `inltkh`, `bbca`, `cvit-mkb-clsr`, `iitp-mr`, `iitp-pr`, `actsa-sc`, `md`, and`wiki-ner`.
+
+More information about the different subsets of the Indic GLUE dataset can be found on the [IndicGLUE dataset page](https://indicnlp.ai4bharat.org/indic-glue/).
+
+2. **Calculating the metric**: the metric takes two inputs : one list with the predictions of the model to score and one lists of references for each translation for all subsets of the dataset except for `cvit-mkb-clsr`, where each prediction and reference is a vector of floats.
+
+```python
+from datasets import load_metric
+indic_glue_metric = load_metric('indic_glue', 'wnli')
+references = [0, 1]
+predictions = [0, 1]
+results = indic_glue_metric.compute(predictions=predictions, references=references)
+```
+
+## Output values
+
+The output of the metric depends on the IndicGLUE subset chosen, consisting of a dictionary that contains one or several of the following metrics:
+
+`accuracy`: the proportion of correct predictions among the total number of cases processed, with a range between 0 and 1 (see [accuracy](https://huggingface.co/metrics/accuracy) for more information).
+
+`f1`: the harmonic mean of the precision and recall (see [F1 score](https://huggingface.co/metrics/f1) for more information). Its range is 0-1 -- its lowest possible value is 0, if either the precision or the recall is 0, and its highest possible value is 1.0, which means perfect precision and recall.
+
+`precision@10`: the fraction of the true examples among the top 10 predicted examples, with a range between 0 and 1 (see [precision](https://huggingface.co/metrics/precision) for more information).
+
+The `cvit-mkb-clsr` subset returns `precision@10`, the `wiki-ner` subset returns `accuracy` and `f1`, and all other subsets of Indic GLUE return only accuracy.
+
+### Values from popular papers
+
+The [original IndicGlue paper](https://aclanthology.org/2020.findings-emnlp.445.pdf) reported an average accuracy of 0.766 on the dataset, which varies depending on the subset selected.
+
+## Examples
+
+Maximal values for the WNLI subset (which outputs `accuracy`):
+
+```python
+from datasets import load_metric
+indic_glue_metric = load_metric('indic_glue', 'wnli')
+references = [0, 1]
+predictions = [0, 1]
+results = indic_glue_metric.compute(predictions=predictions, references=references)
+print(results)
+{'accuracy': 1.0}
+```
+
+Minimal values for the Wiki-NER subset (which outputs `accuracy` and `f1`):
+
+```python
+>>> from datasets import load_metric
+>>> indic_glue_metric = load_metric('indic_glue', 'wiki-ner')
+>>> references = [0, 1]
+>>> predictions = [1,0]
+>>> results = indic_glue_metric.compute(predictions=predictions, references=references)
+>>> print(results)
+{'accuracy': 1.0, 'f1': 1.0}
+```
+
+Partial match for the CVIT-Mann Ki Baat subset (which outputs `precision@10`)
+
+```python
+>>> from datasets import load_metric
+>>> indic_glue_metric = load_metric('indic_glue', 'cvit-mkb-clsr')
+>>> references = [[0.5, 0.5, 0.5], [0.1, 0.2, 0.3]]
+>>> predictions = [[0.5, 0.5, 0.5], [0.1, 0.2, 0.3]]
+>>> results = indic_glue_metric.compute(predictions=predictions, references=references)
+>>> print(results)
+{'precision@10': 1.0}
+```
+
+## Limitations and bias
+This metric works only with datasets that have the same format as the [IndicGLUE dataset](https://huggingface.co/datasets/glue).
+
+## Citation
+
+```bibtex
+ @inproceedings{kakwani2020indicnlpsuite,
+ title={{IndicNLPSuite: Monolingual Corpora, Evaluation Benchmarks and Pre-trained Multilingual Language Models for Indian Languages}},
+ author={Divyanshu Kakwani and Anoop Kunchukuttan and Satish Golla and Gokul N.C. and Avik Bhattacharyya and Mitesh M. Khapra and Pratyush Kumar},
+ year={2020},
+ booktitle={Findings of EMNLP},
+}
+```
+
+## Further References
+- [IndicNLP website](https://indicnlp.ai4bharat.org/home/)
+-
diff --git a/metrics/indic_glue/indic_glue.py b/metrics/indic_glue/indic_glue.py
new file mode 100644
index 0000000..0cbf3aa
--- /dev/null
+++ b/metrics/indic_glue/indic_glue.py
@@ -0,0 +1,172 @@
+# Copyright 2020 The HuggingFace Datasets Authors.
+#
+# Licensed under the Apache License, Version 2.0 (the "License");
+# you may not use this file except in compliance with the License.
+# You may obtain a copy of the License at
+#
+# http://www.apache.org/licenses/LICENSE-2.0
+#
+# Unless required by applicable law or agreed to in writing, software
+# distributed under the License is distributed on an "AS IS" BASIS,
+# WITHOUT WARRANTIES OR CONDITIONS OF ANY KIND, either express or implied.
+# See the License for the specific language governing permissions and
+# limitations under the License.
+""" IndicGLUE benchmark metric. """
+
+import numpy as np
+from scipy.spatial.distance import cdist
+from scipy.stats import pearsonr, spearmanr
+from sklearn.metrics import f1_score
+
+import datasets
+
+
+_CITATION = """\
+ @inproceedings{kakwani2020indicnlpsuite,
+ title={{IndicNLPSuite: Monolingual Corpora, Evaluation Benchmarks and Pre-trained Multilingual Language Models for Indian Languages}},
+ author={Divyanshu Kakwani and Anoop Kunchukuttan and Satish Golla and Gokul N.C. and Avik Bhattacharyya and Mitesh M. Khapra and Pratyush Kumar},
+ year={2020},
+ booktitle={Findings of EMNLP},
+}
+"""
+
+_DESCRIPTION = """\
+ IndicGLUE is a natural language understanding benchmark for Indian languages. It contains a wide
+ variety of tasks and covers 11 major Indian languages - as, bn, gu, hi, kn, ml, mr, or, pa, ta, te.
+"""
+
+_KWARGS_DESCRIPTION = """
+Compute IndicGLUE evaluation metric associated to each IndicGLUE dataset.
+Args:
+ predictions: list of predictions to score (as int64),
+ except for 'cvit-mkb-clsr' where each prediction is a vector (of float32).
+ references: list of ground truth labels corresponding to the predictions (as int64),
+ except for 'cvit-mkb-clsr' where each reference is a vector (of float32).
+Returns: depending on the IndicGLUE subset, one or several of:
+ "accuracy": Accuracy
+ "f1": F1 score
+ "precision": Precision@10
+Examples:
+
+ >>> indic_glue_metric = datasets.load_metric('indic_glue', 'wnli') # 'wnli' or any of ["copa", "sna", "csqa", "wstp", "inltkh", "bbca", "iitp-mr", "iitp-pr", "actsa-sc", "md"]
+ >>> references = [0, 1]
+ >>> predictions = [0, 1]
+ >>> results = indic_glue_metric.compute(predictions=predictions, references=references)
+ >>> print(results)
+ {'accuracy': 1.0}
+
+ >>> indic_glue_metric = datasets.load_metric('indic_glue', 'wiki-ner')
+ >>> references = [0, 1]
+ >>> predictions = [0, 1]
+ >>> results = indic_glue_metric.compute(predictions=predictions, references=references)
+ >>> print(results)
+ {'accuracy': 1.0, 'f1': 1.0}
+
+ >>> indic_glue_metric = datasets.load_metric('indic_glue', 'cvit-mkb-clsr')
+ >>> references = [[0.5, 0.5, 0.5], [0.1, 0.2, 0.3]]
+ >>> predictions = [[0.5, 0.5, 0.5], [0.1, 0.2, 0.3]]
+ >>> results = indic_glue_metric.compute(predictions=predictions, references=references)
+ >>> print(results)
+ {'precision@10': 1.0}
+
+"""
+
+
+def simple_accuracy(preds, labels):
+ return float((preds == labels).mean())
+
+
+def acc_and_f1(preds, labels):
+ acc = simple_accuracy(preds, labels)
+ f1 = float(f1_score(y_true=labels, y_pred=preds))
+ return {
+ "accuracy": acc,
+ "f1": f1,
+ }
+
+
+def precision_at_10(en_sentvecs, in_sentvecs):
+ en_sentvecs = np.array(en_sentvecs)
+ in_sentvecs = np.array(in_sentvecs)
+ n = en_sentvecs.shape[0]
+
+ # mean centering
+ en_sentvecs = en_sentvecs - np.mean(en_sentvecs, axis=0)
+ in_sentvecs = in_sentvecs - np.mean(in_sentvecs, axis=0)
+
+ sim = cdist(en_sentvecs, in_sentvecs, "cosine")
+ actual = np.array(range(n))
+ preds = sim.argsort(axis=1)[:, :10]
+ matches = np.any(preds == actual[:, None], axis=1)
+ return float(matches.mean())
+
+
+@datasets.utils.file_utils.add_start_docstrings(_DESCRIPTION, _KWARGS_DESCRIPTION)
+class IndicGlue(datasets.Metric):
+ def _info(self):
+ if self.config_name not in [
+ "wnli",
+ "copa",
+ "sna",
+ "csqa",
+ "wstp",
+ "inltkh",
+ "bbca",
+ "cvit-mkb-clsr",
+ "iitp-mr",
+ "iitp-pr",
+ "actsa-sc",
+ "md",
+ "wiki-ner",
+ ]:
+ raise KeyError(
+ "You should supply a configuration name selected in "
+ '["wnli", "copa", "sna", "csqa", "wstp", "inltkh", "bbca", '
+ '"cvit-mkb-clsr", "iitp-mr", "iitp-pr", "actsa-sc", "md", '
+ '"wiki-ner"]'
+ )
+ return datasets.MetricInfo(
+ description=_DESCRIPTION,
+ citation=_CITATION,
+ inputs_description=_KWARGS_DESCRIPTION,
+ features=datasets.Features(
+ {
+ "predictions": datasets.Value("int64")
+ if self.config_name != "cvit-mkb-clsr"
+ else datasets.Sequence(datasets.Value("float32")),
+ "references": datasets.Value("int64")
+ if self.config_name != "cvit-mkb-clsr"
+ else datasets.Sequence(datasets.Value("float32")),
+ }
+ ),
+ codebase_urls=[],
+ reference_urls=[],
+ format="numpy" if self.config_name != "cvit-mkb-clsr" else None,
+ )
+
+ def _compute(self, predictions, references):
+ if self.config_name == "cvit-mkb-clsr":
+ return {"precision@10": precision_at_10(predictions, references)}
+ elif self.config_name in ["wiki-ner"]:
+ return acc_and_f1(predictions, references)
+ elif self.config_name in [
+ "wnli",
+ "copa",
+ "sna",
+ "csqa",
+ "wstp",
+ "inltkh",
+ "bbca",
+ "iitp-mr",
+ "iitp-pr",
+ "actsa-sc",
+ "md",
+ ]:
+ return {"accuracy": simple_accuracy(predictions, references)}
+ else:
+ raise KeyError(
+ "You should supply a configuration name selected in "
+ '["wnli", "copa", "sna", "csqa", "wstp", "inltkh", "bbca", '
+ '"cvit-mkb-clsr", "iitp-mr", "iitp-pr", "actsa-sc", "md", '
+ '"wiki-ner"]'
+ )
diff --git a/metrics/mae/README.md b/metrics/mae/README.md
new file mode 100644
index 0000000..0765e42
--- /dev/null
+++ b/metrics/mae/README.md
@@ -0,0 +1,114 @@
+# Metric Card for MAE
+
+
+## Metric Description
+
+Mean Absolute Error (MAE) is the mean of the magnitude of difference between the predicted and actual numeric values:
+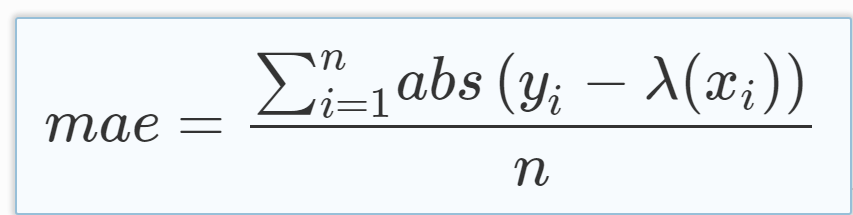
+
+
+## How to Use
+
+At minimum, this metric requires predictions and references as inputs.
+
+```python
+>>> mae_metric = datasets.load_metric("mae")
+>>> predictions = [2.5, 0.0, 2, 8]
+>>> references = [3, -0.5, 2, 7]
+>>> results = mae_metric.compute(predictions=predictions, references=references)
+```
+
+### Inputs
+
+Mandatory inputs:
+- `predictions`: numeric array-like of shape (`n_samples,`) or (`n_samples`, `n_outputs`), representing the estimated target values.
+- `references`: numeric array-like of shape (`n_samples,`) or (`n_samples`, `n_outputs`), representing the ground truth (correct) target values.
+
+Optional arguments:
+- `sample_weight`: numeric array-like of shape (`n_samples,`) representing sample weights. The default is `None`.
+- `multioutput`: `raw_values`, `uniform_average` or numeric array-like of shape (`n_outputs,`), which defines the aggregation of multiple output values. The default value is `uniform_average`.
+ - `raw_values` returns a full set of errors in case of multioutput input.
+ - `uniform_average` means that the errors of all outputs are averaged with uniform weight.
+ - the array-like value defines weights used to average errors.
+
+### Output Values
+This metric outputs a dictionary, containing the mean absolute error score, which is of type:
+- `float`: if multioutput is `uniform_average` or an ndarray of weights, then the weighted average of all output errors is returned.
+- numeric array-like of shape (`n_outputs,`): if multioutput is `raw_values`, then the score is returned for each output separately.
+
+Each MAE `float` value ranges from `0.0` to `1.0`, with the best value being 0.0.
+
+Output Example(s):
+```python
+{'mae': 0.5}
+```
+
+If `multioutput="raw_values"`:
+```python
+{'mae': array([0.5, 1. ])}
+```
+
+#### Values from Popular Papers
+
+
+### Examples
+
+Example with the `uniform_average` config:
+```python
+>>> from datasets import load_metric
+>>> mae_metric = load_metric("mae")
+>>> predictions = [2.5, 0.0, 2, 8]
+>>> references = [3, -0.5, 2, 7]
+>>> results = mae_metric.compute(predictions=predictions, references=references)
+>>> print(results)
+{'mae': 0.5}
+```
+
+Example with multi-dimensional lists, and the `raw_values` config:
+```python
+>>> from datasets import load_metric
+>>> mae_metric = datasets.load_metric("mae", "multilist")
+>>> predictions = [[0.5, 1], [-1, 1], [7, -6]]
+>>> references = [[0, 2], [-1, 2], [8, -5]]
+>>> results = mae_metric.compute(predictions=predictions, references=references)
+>>> print(results)
+{'mae': 0.75}
+>>> results = mae_metric.compute(predictions=predictions, references=references, multioutput='raw_values')
+>>> print(results)
+{'mae': array([0.5, 1. ])}
+```
+
+## Limitations and Bias
+One limitation of MAE is that the relative size of the error is not always obvious, meaning that it can be difficult to tell a big error from a smaller one -- metrics such as Mean Absolute Percentage Error (MAPE) have been proposed to calculate MAE in percentage terms.
+
+Also, since it calculates the mean, MAE may underestimate the impact of big, but infrequent, errors -- metrics such as the Root Mean Square Error (RMSE) compensate for this by taking the root of error values.
+
+## Citation(s)
+```bibtex
+@article{scikit-learn,
+ title={Scikit-learn: Machine Learning in {P}ython},
+ author={Pedregosa, F. and Varoquaux, G. and Gramfort, A. and Michel, V.
+ and Thirion, B. and Grisel, O. and Blondel, M. and Prettenhofer, P.
+ and Weiss, R. and Dubourg, V. and Vanderplas, J. and Passos, A. and
+ Cournapeau, D. and Brucher, M. and Perrot, M. and Duchesnay, E.},
+ journal={Journal of Machine Learning Research},
+ volume={12},
+ pages={2825--2830},
+ year={2011}
+}
+```
+
+```bibtex
+@article{willmott2005advantages,
+ title={Advantages of the mean absolute error (MAE) over the root mean square error (RMSE) in assessing average model performance},
+ author={Willmott, Cort J and Matsuura, Kenji},
+ journal={Climate research},
+ volume={30},
+ number={1},
+ pages={79--82},
+ year={2005}
+}
+```
+
+## Further References
+- [Mean Absolute Error - Wikipedia](https://en.wikipedia.org/wiki/Mean_absolute_error)
diff --git a/metrics/mae/mae.py b/metrics/mae/mae.py
new file mode 100644
index 0000000..aa51cd0
--- /dev/null
+++ b/metrics/mae/mae.py
@@ -0,0 +1,112 @@
+# Copyright 2022 The HuggingFace Datasets Authors and the current dataset script contributor.
+#
+# Licensed under the Apache License, Version 2.0 (the "License");
+# you may not use this file except in compliance with the License.
+# You may obtain a copy of the License at
+#
+# http://www.apache.org/licenses/LICENSE-2.0
+#
+# Unless required by applicable law or agreed to in writing, software
+# distributed under the License is distributed on an "AS IS" BASIS,
+# WITHOUT WARRANTIES OR CONDITIONS OF ANY KIND, either express or implied.
+# See the License for the specific language governing permissions and
+# limitations under the License.
+"""MAE - Mean Absolute Error Metric"""
+
+from sklearn.metrics import mean_absolute_error
+
+import datasets
+
+
+_CITATION = """\
+@article{scikit-learn,
+ title={Scikit-learn: Machine Learning in {P}ython},
+ author={Pedregosa, F. and Varoquaux, G. and Gramfort, A. and Michel, V.
+ and Thirion, B. and Grisel, O. and Blondel, M. and Prettenhofer, P.
+ and Weiss, R. and Dubourg, V. and Vanderplas, J. and Passos, A. and
+ Cournapeau, D. and Brucher, M. and Perrot, M. and Duchesnay, E.},
+ journal={Journal of Machine Learning Research},
+ volume={12},
+ pages={2825--2830},
+ year={2011}
+}
+"""
+
+_DESCRIPTION = """\
+Mean Absolute Error (MAE) is the mean of the magnitude of difference between the predicted and actual
+values.
+"""
+
+
+_KWARGS_DESCRIPTION = """
+Args:
+ predictions: array-like of shape (n_samples,) or (n_samples, n_outputs)
+ Estimated target values.
+ references: array-like of shape (n_samples,) or (n_samples, n_outputs)
+ Ground truth (correct) target values.
+ sample_weight: array-like of shape (n_samples,), default=None
+ Sample weights.
+ multioutput: {"raw_values", "uniform_average"} or array-like of shape (n_outputs,), default="uniform_average"
+ Defines aggregating of multiple output values. Array-like value defines weights used to average errors.
+
+ "raw_values" : Returns a full set of errors in case of multioutput input.
+
+ "uniform_average" : Errors of all outputs are averaged with uniform weight.
+
+Returns:
+ mae : mean absolute error.
+ If multioutput is "raw_values", then mean absolute error is returned for each output separately. If multioutput is "uniform_average" or an ndarray of weights, then the weighted average of all output errors is returned.
+ MAE output is non-negative floating point. The best value is 0.0.
+Examples:
+
+ >>> mae_metric = datasets.load_metric("mae")
+ >>> predictions = [2.5, 0.0, 2, 8]
+ >>> references = [3, -0.5, 2, 7]
+ >>> results = mae_metric.compute(predictions=predictions, references=references)
+ >>> print(results)
+ {'mae': 0.5}
+
+ If you're using multi-dimensional lists, then set the config as follows :
+
+ >>> mae_metric = datasets.load_metric("mae", "multilist")
+ >>> predictions = [[0.5, 1], [-1, 1], [7, -6]]
+ >>> references = [[0, 2], [-1, 2], [8, -5]]
+ >>> results = mae_metric.compute(predictions=predictions, references=references)
+ >>> print(results)
+ {'mae': 0.75}
+ >>> results = mae_metric.compute(predictions=predictions, references=references, multioutput='raw_values')
+ >>> print(results)
+ {'mae': array([0.5, 1. ])}
+"""
+
+
+@datasets.utils.file_utils.add_start_docstrings(_DESCRIPTION, _KWARGS_DESCRIPTION)
+class Mae(datasets.Metric):
+ def _info(self):
+ return datasets.MetricInfo(
+ description=_DESCRIPTION,
+ citation=_CITATION,
+ inputs_description=_KWARGS_DESCRIPTION,
+ features=datasets.Features(self._get_feature_types()),
+ reference_urls=[
+ "https://scikit-learn.org/stable/modules/generated/sklearn.metrics.mean_absolute_error.html"
+ ],
+ )
+
+ def _get_feature_types(self):
+ if self.config_name == "multilist":
+ return {
+ "predictions": datasets.Sequence(datasets.Value("float")),
+ "references": datasets.Sequence(datasets.Value("float")),
+ }
+ else:
+ return {
+ "predictions": datasets.Value("float"),
+ "references": datasets.Value("float"),
+ }
+
+ def _compute(self, predictions, references, sample_weight=None, multioutput="uniform_average"):
+
+ mae_score = mean_absolute_error(references, predictions, sample_weight=sample_weight, multioutput=multioutput)
+
+ return {"mae": mae_score}
diff --git a/metrics/mahalanobis/README.md b/metrics/mahalanobis/README.md
new file mode 100644
index 0000000..a25d116
--- /dev/null
+++ b/metrics/mahalanobis/README.md
@@ -0,0 +1,70 @@
+# Metric Card for Mahalanobis Distance
+
+## Metric Description
+Mahalonobis distance is the distance between a point and a distribution (as opposed to the distance between two points), making it the multivariate equivalent of the Euclidean distance.
+
+It is often used in multivariate anomaly detection, classification on highly imbalanced datasets and one-class classification.
+
+## How to Use
+At minimum, this metric requires two `list`s of datapoints:
+
+```python
+>>> mahalanobis_metric = datasets.load_metric("mahalanobis")
+>>> results = mahalanobis_metric.compute(reference_distribution=[[0, 1], [1, 0]], X=[[0, 1]])
+```
+
+### Inputs
+- `X` (`list`): data points to be compared with the `reference_distribution`.
+- `reference_distribution` (`list`): data points from the reference distribution that we want to compare to.
+
+### Output Values
+`mahalanobis` (`array`): the Mahalonobis distance for each data point in `X`.
+
+```python
+>>> print(results)
+{'mahalanobis': array([0.5])}
+```
+
+#### Values from Popular Papers
+*N/A*
+
+### Example
+
+```python
+>>> mahalanobis_metric = datasets.load_metric("mahalanobis")
+>>> results = mahalanobis_metric.compute(reference_distribution=[[0, 1], [1, 0]], X=[[0, 1]])
+>>> print(results)
+{'mahalanobis': array([0.5])}
+```
+
+## Limitations and Bias
+
+The Mahalanobis distance is only able to capture linear relationships between the variables, which means it cannot capture all types of outliers. Mahalanobis distance also fails to faithfully represent data that is highly skewed or multimodal.
+
+## Citation
+```bibtex
+@inproceedings{mahalanobis1936generalized,
+ title={On the generalized distance in statistics},
+ author={Mahalanobis, Prasanta Chandra},
+ year={1936},
+ organization={National Institute of Science of India}
+}
+```
+
+```bibtex
+@article{de2000mahalanobis,
+ title={The Mahalanobis distance},
+ author={De Maesschalck, Roy and Jouan-Rimbaud, Delphine and Massart, D{\'e}sir{\'e} L},
+ journal={Chemometrics and intelligent laboratory systems},
+ volume={50},
+ number={1},
+ pages={1--18},
+ year={2000},
+ publisher={Elsevier}
+}
+```
+
+## Further References
+-[Wikipedia -- Mahalanobis Distance](https://en.wikipedia.org/wiki/Mahalanobis_distance)
+
+-[Machine Learning Plus -- Mahalanobis Distance](https://www.machinelearningplus.com/statistics/mahalanobis-distance/)
diff --git a/metrics/mahalanobis/mahalanobis.py b/metrics/mahalanobis/mahalanobis.py
new file mode 100644
index 0000000..3cb4309
--- /dev/null
+++ b/metrics/mahalanobis/mahalanobis.py
@@ -0,0 +1,99 @@
+# Copyright 2021 The HuggingFace Datasets Authors and the current dataset script contributor.
+#
+# Licensed under the Apache License, Version 2.0 (the "License");
+# you may not use this file except in compliance with the License.
+# You may obtain a copy of the License at
+#
+# http://www.apache.org/licenses/LICENSE-2.0
+#
+# Unless required by applicable law or agreed to in writing, software
+# distributed under the License is distributed on an "AS IS" BASIS,
+# WITHOUT WARRANTIES OR CONDITIONS OF ANY KIND, either express or implied.
+# See the License for the specific language governing permissions and
+# limitations under the License.
+"""Mahalanobis metric."""
+
+import numpy as np
+
+import datasets
+
+
+_DESCRIPTION = """
+Compute the Mahalanobis Distance
+
+Mahalonobis distance is the distance between a point and a distribution.
+And not between two distinct points. It is effectively a multivariate equivalent of the Euclidean distance.
+It was introduced by Prof. P. C. Mahalanobis in 1936
+and has been used in various statistical applications ever since
+[source: https://www.machinelearningplus.com/statistics/mahalanobis-distance/]
+"""
+
+_CITATION = """\
+@article{de2000mahalanobis,
+ title={The mahalanobis distance},
+ author={De Maesschalck, Roy and Jouan-Rimbaud, Delphine and Massart, D{\'e}sir{\'e} L},
+ journal={Chemometrics and intelligent laboratory systems},
+ volume={50},
+ number={1},
+ pages={1--18},
+ year={2000},
+ publisher={Elsevier}
+}
+"""
+
+_KWARGS_DESCRIPTION = """
+Args:
+ X: List of datapoints to be compared with the `reference_distribution`.
+ reference_distribution: List of datapoints from the reference distribution we want to compare to.
+Returns:
+ mahalanobis: The Mahalonobis distance for each datapoint in `X`.
+Examples:
+
+ >>> mahalanobis_metric = datasets.load_metric("mahalanobis")
+ >>> results = mahalanobis_metric.compute(reference_distribution=[[0, 1], [1, 0]], X=[[0, 1]])
+ >>> print(results)
+ {'mahalanobis': array([0.5])}
+"""
+
+
+@datasets.utils.file_utils.add_start_docstrings(_DESCRIPTION, _KWARGS_DESCRIPTION)
+class Mahalanobis(datasets.Metric):
+ def _info(self):
+ return datasets.MetricInfo(
+ description=_DESCRIPTION,
+ citation=_CITATION,
+ inputs_description=_KWARGS_DESCRIPTION,
+ features=datasets.Features(
+ {
+ "X": datasets.Sequence(datasets.Value("float", id="sequence"), id="X"),
+ }
+ ),
+ )
+
+ def _compute(self, X, reference_distribution):
+
+ # convert to numpy arrays
+ X = np.array(X)
+ reference_distribution = np.array(reference_distribution)
+
+ # Assert that arrays are 2D
+ if len(X.shape) != 2:
+ raise ValueError("Expected `X` to be a 2D vector")
+ if len(reference_distribution.shape) != 2:
+ raise ValueError("Expected `reference_distribution` to be a 2D vector")
+ if reference_distribution.shape[0] < 2:
+ raise ValueError(
+ "Expected `reference_distribution` to be a 2D vector with more than one element in the first dimension"
+ )
+
+ # Get mahalanobis distance for each prediction
+ X_minus_mu = X - np.mean(reference_distribution)
+ cov = np.cov(reference_distribution.T)
+ try:
+ inv_covmat = np.linalg.inv(cov)
+ except np.linalg.LinAlgError:
+ inv_covmat = np.linalg.pinv(cov)
+ left_term = np.dot(X_minus_mu, inv_covmat)
+ mahal_dist = np.dot(left_term, X_minus_mu.T).diagonal()
+
+ return {"mahalanobis": mahal_dist}
diff --git a/metrics/matthews_correlation/README.md b/metrics/matthews_correlation/README.md
new file mode 100644
index 0000000..f4c5a64
--- /dev/null
+++ b/metrics/matthews_correlation/README.md
@@ -0,0 +1,91 @@
+# Metric Card for Matthews Correlation Coefficient
+
+## Metric Description
+The Matthews correlation coefficient is used in machine learning as a
+measure of the quality of binary and multiclass classifications. It takes
+into account true and false positives and negatives and is generally
+regarded as a balanced measure which can be used even if the classes are of
+very different sizes. The MCC is in essence a correlation coefficient value
+between -1 and +1. A coefficient of +1 represents a perfect prediction, 0
+an average random prediction and -1 an inverse prediction. The statistic
+is also known as the phi coefficient. [source: Wikipedia]
+
+## How to Use
+At minimum, this metric requires a list of predictions and a list of references:
+```python
+>>> matthews_metric = datasets.load_metric("matthews_correlation")
+>>> results = matthews_metric.compute(references=[0, 1], predictions=[0, 1])
+>>> print(results)
+{'matthews_correlation': 1.0}
+```
+
+### Inputs
+- **`predictions`** (`list` of `int`s): Predicted class labels.
+- **`references`** (`list` of `int`s): Ground truth labels.
+- **`sample_weight`** (`list` of `int`s, `float`s, or `bool`s): Sample weights. Defaults to `None`.
+
+### Output Values
+- **`matthews_correlation`** (`float`): Matthews correlation coefficient.
+
+The metric output takes the following form:
+```python
+{'matthews_correlation': 0.54}
+```
+
+This metric can be any value from -1 to +1, inclusive.
+
+#### Values from Popular Papers
+
+
+### Examples
+A basic example with only predictions and references as inputs:
+```python
+>>> matthews_metric = datasets.load_metric("matthews_correlation")
+>>> results = matthews_metric.compute(references=[1, 3, 2, 0, 3, 2],
+... predictions=[1, 2, 2, 0, 3, 3])
+>>> print(results)
+{'matthews_correlation': 0.5384615384615384}
+```
+
+The same example as above, but also including sample weights:
+```python
+>>> matthews_metric = datasets.load_metric("matthews_correlation")
+>>> results = matthews_metric.compute(references=[1, 3, 2, 0, 3, 2],
+... predictions=[1, 2, 2, 0, 3, 3],
+... sample_weight=[0.5, 3, 1, 1, 1, 2])
+>>> print(results)
+{'matthews_correlation': 0.09782608695652174}
+```
+
+The same example as above, with sample weights that cause a negative correlation:
+```python
+>>> matthews_metric = datasets.load_metric("matthews_correlation")
+>>> results = matthews_metric.compute(references=[1, 3, 2, 0, 3, 2],
+... predictions=[1, 2, 2, 0, 3, 3],
+... sample_weight=[0.5, 1, 0, 0, 0, 1])
+>>> print(results)
+{'matthews_correlation': -0.25}
+```
+
+## Limitations and Bias
+*Note any limitations or biases that the metric has.*
+
+
+## Citation
+```bibtex
+@article{scikit-learn,
+ title={Scikit-learn: Machine Learning in {P}ython},
+ author={Pedregosa, F. and Varoquaux, G. and Gramfort, A. and Michel, V.
+ and Thirion, B. and Grisel, O. and Blondel, M. and Prettenhofer, P.
+ and Weiss, R. and Dubourg, V. and Vanderplas, J. and Passos, A. and
+ Cournapeau, D. and Brucher, M. and Perrot, M. and Duchesnay, E.},
+ journal={Journal of Machine Learning Research},
+ volume={12},
+ pages={2825--2830},
+ year={2011}
+}
+```
+
+## Further References
+
+- This Hugging Face implementation uses [this scikit-learn implementation](https://scikit-learn.org/stable/modules/generated/sklearn.metrics.matthews_corrcoef.html)
\ No newline at end of file
diff --git a/metrics/matthews_correlation/matthews_correlation.py b/metrics/matthews_correlation/matthews_correlation.py
new file mode 100644
index 0000000..1c7f6bf
--- /dev/null
+++ b/metrics/matthews_correlation/matthews_correlation.py
@@ -0,0 +1,102 @@
+# Copyright 2021 The HuggingFace Datasets Authors and the current dataset script contributor.
+#
+# Licensed under the Apache License, Version 2.0 (the "License");
+# you may not use this file except in compliance with the License.
+# You may obtain a copy of the License at
+#
+# http://www.apache.org/licenses/LICENSE-2.0
+#
+# Unless required by applicable law or agreed to in writing, software
+# distributed under the License is distributed on an "AS IS" BASIS,
+# WITHOUT WARRANTIES OR CONDITIONS OF ANY KIND, either express or implied.
+# See the License for the specific language governing permissions and
+# limitations under the License.
+"""Matthews Correlation metric."""
+
+from sklearn.metrics import matthews_corrcoef
+
+import datasets
+
+
+_DESCRIPTION = """
+Compute the Matthews correlation coefficient (MCC)
+
+The Matthews correlation coefficient is used in machine learning as a
+measure of the quality of binary and multiclass classifications. It takes
+into account true and false positives and negatives and is generally
+regarded as a balanced measure which can be used even if the classes are of
+very different sizes. The MCC is in essence a correlation coefficient value
+between -1 and +1. A coefficient of +1 represents a perfect prediction, 0
+an average random prediction and -1 an inverse prediction. The statistic
+is also known as the phi coefficient. [source: Wikipedia]
+"""
+
+_KWARGS_DESCRIPTION = """
+Args:
+ predictions (list of int): Predicted labels, as returned by a model.
+ references (list of int): Ground truth labels.
+ sample_weight (list of int, float, or bool): Sample weights. Defaults to `None`.
+Returns:
+ matthews_correlation (dict containing float): Matthews correlation.
+Examples:
+ Example 1, a basic example with only predictions and references as inputs:
+ >>> matthews_metric = datasets.load_metric("matthews_correlation")
+ >>> results = matthews_metric.compute(references=[1, 3, 2, 0, 3, 2],
+ ... predictions=[1, 2, 2, 0, 3, 3])
+ >>> print(round(results['matthews_correlation'], 2))
+ 0.54
+
+ Example 2, the same example as above, but also including sample weights:
+ >>> matthews_metric = datasets.load_metric("matthews_correlation")
+ >>> results = matthews_metric.compute(references=[1, 3, 2, 0, 3, 2],
+ ... predictions=[1, 2, 2, 0, 3, 3],
+ ... sample_weight=[0.5, 3, 1, 1, 1, 2])
+ >>> print(round(results['matthews_correlation'], 2))
+ 0.1
+
+ Example 3, the same example as above, but with sample weights that cause a negative correlation:
+ >>> matthews_metric = datasets.load_metric("matthews_correlation")
+ >>> results = matthews_metric.compute(references=[1, 3, 2, 0, 3, 2],
+ ... predictions=[1, 2, 2, 0, 3, 3],
+ ... sample_weight=[0.5, 1, 0, 0, 0, 1])
+ >>> print(round(results['matthews_correlation'], 2))
+ -0.25
+"""
+
+_CITATION = """\
+@article{scikit-learn,
+ title={Scikit-learn: Machine Learning in {P}ython},
+ author={Pedregosa, F. and Varoquaux, G. and Gramfort, A. and Michel, V.
+ and Thirion, B. and Grisel, O. and Blondel, M. and Prettenhofer, P.
+ and Weiss, R. and Dubourg, V. and Vanderplas, J. and Passos, A. and
+ Cournapeau, D. and Brucher, M. and Perrot, M. and Duchesnay, E.},
+ journal={Journal of Machine Learning Research},
+ volume={12},
+ pages={2825--2830},
+ year={2011}
+}
+"""
+
+
+@datasets.utils.file_utils.add_start_docstrings(_DESCRIPTION, _KWARGS_DESCRIPTION)
+class MatthewsCorrelation(datasets.Metric):
+ def _info(self):
+ return datasets.MetricInfo(
+ description=_DESCRIPTION,
+ citation=_CITATION,
+ inputs_description=_KWARGS_DESCRIPTION,
+ features=datasets.Features(
+ {
+ "predictions": datasets.Value("int32"),
+ "references": datasets.Value("int32"),
+ }
+ ),
+ reference_urls=[
+ "https://scikit-learn.org/stable/modules/generated/sklearn.metrics.matthews_corrcoef.html"
+ ],
+ )
+
+ def _compute(self, predictions, references, sample_weight=None):
+ return {
+ "matthews_correlation": float(matthews_corrcoef(references, predictions, sample_weight=sample_weight)),
+ }
diff --git a/metrics/mauve/README.md b/metrics/mauve/README.md
new file mode 100644
index 0000000..d9f5ce4
--- /dev/null
+++ b/metrics/mauve/README.md
@@ -0,0 +1,118 @@
+# Metric Card for MAUVE
+
+## Metric description
+
+MAUVE is a library built on PyTorch and HuggingFace Transformers to measure the gap between neural text and human text with the eponymous MAUVE measure. It summarizes both Type I and Type II errors measured softly using [Kullback–Leibler (KL) divergences](https://en.wikipedia.org/wiki/Kullback%E2%80%93Leibler_divergence).
+
+This metric is a wrapper around the [official implementation](https://github.com/krishnap25/mauve) of MAUVE.
+
+For more details, consult the [MAUVE paper](https://arxiv.org/abs/2102.01454).
+
+
+## How to use
+
+The metric takes two lists of strings of tokens separated by spaces: one representing `predictions` (i.e. the text generated by the model) and the second representing `references` (a reference text for each prediction):
+
+```python
+from datasets import load_metric
+mauve = load_metric('mauve')
+predictions = ["hello world", "goodnight moon"]
+references = ["hello world", "goodnight moon"]
+mauve_results = mauve.compute(predictions=predictions, references=references)
+```
+
+It also has several optional arguments:
+
+`num_buckets`: the size of the histogram to quantize P and Q. Options: `auto` (default) or an integer.
+
+`pca_max_data`: the number data points to use for PCA dimensionality reduction prior to clustering. If -1, use all the data. The default is `-1`.
+
+`kmeans_explained_var`: amount of variance of the data to keep in dimensionality reduction by PCA. The default is `0.9`.
+
+`kmeans_num_redo`: number of times to redo k-means clustering (the best objective is kept). The default is `5`.
+
+`kmeans_max_iter`: maximum number of k-means iterations. The default is `500`.
+
+`featurize_model_name`: name of the model from which features are obtained, from one of the following: `gpt2`, `gpt2-medium`, `gpt2-large`, `gpt2-xl`. The default is `gpt2-large`.
+
+`device_id`: Device for featurization. Supply a GPU id (e.g. `0` or `3`) to use GPU. If no GPU with this id is found, the metric will use CPU.
+
+`max_text_length`: maximum number of tokens to consider. The default is `1024`.
+
+`divergence_curve_discretization_size` Number of points to consider on the divergence curve. The default is `25`.
+
+`mauve_scaling_factor`: Hyperparameter for scaling. The default is `5`.
+
+`verbose`: If `True` (default), running the metric will print running time updates.
+
+`seed`: random seed to initialize k-means cluster assignments, randomly assigned by default.
+
+
+
+## Output values
+
+This metric outputs a dictionary with 5 key-value pairs:
+
+`mauve`: MAUVE score, which ranges between 0 and 1. **Larger** values indicate that P and Q are closer.
+
+`frontier_integral`: Frontier Integral, which ranges between 0 and 1. **Smaller** values indicate that P and Q are closer.
+
+`divergence_curve`: a numpy.ndarray of shape (m, 2); plot it with `matplotlib` to view the divergence curve.
+
+`p_hist`: a discrete distribution, which is a quantized version of the text distribution `p_text`.
+
+`q_hist`: same as above, but with `q_text`.
+
+
+### Values from popular papers
+
+The [original MAUVE paper](https://arxiv.org/abs/2102.01454) reported values ranging from 0.88 to 0.94 for open-ended text generation using a text completion task in the web text domain. The authors found that bigger models resulted in higher MAUVE scores, and that MAUVE is correlated with human judgments.
+
+
+## Examples
+
+Perfect match between prediction and reference:
+
+```python
+from datasets import load_metric
+mauve = load_metric('mauve')
+predictions = ["hello world", "goodnight moon"]
+references = ["hello world", "goodnight moon"]
+mauve_results = mauve.compute(predictions=predictions, references=references)
+print(mauve_results.mauve)
+1.0
+```
+
+Partial match between prediction and reference:
+
+```python
+from datasets import load_metric
+mauve = load_metric('mauve')
+predictions = ["hello world", "goodnight moon"]
+references = ["hello there", "general kenobi"]
+mauve_results = mauve.compute(predictions=predictions, references=references)
+print(mauve_results.mauve)
+0.27811372536724027
+```
+
+## Limitations and bias
+
+The [original MAUVE paper](https://arxiv.org/abs/2102.01454) did not analyze the inductive biases present in different embedding models, but related work has shown different kinds of biases exist in many popular generative language models including GPT-2 (see [Kirk et al., 2021](https://arxiv.org/pdf/2102.04130.pdf), [Abid et al., 2021](https://arxiv.org/abs/2101.05783)). The extent to which these biases can impact the MAUVE score has not been quantified.
+
+Also, calculating the MAUVE metric involves downloading the model from which features are obtained -- the default model, `gpt2-large`, takes over 3GB of storage space and downloading it can take a significant amount of time depending on the speed of your internet connection. If this is an issue, choose a smaller model; for instance `gpt` is 523MB.
+
+
+## Citation
+
+```bibtex
+@inproceedings{pillutla-etal:mauve:neurips2021,
+ title={MAUVE: Measuring the Gap Between Neural Text and Human Text using Divergence Frontiers},
+ author={Pillutla, Krishna and Swayamdipta, Swabha and Zellers, Rowan and Thickstun, John and Welleck, Sean and Choi, Yejin and Harchaoui, Zaid},
+ booktitle = {NeurIPS},
+ year = {2021}
+}
+```
+
+## Further References
+- [Official MAUVE implementation](https://github.com/krishnap25/mauve)
+- [Hugging Face Tasks - Text Generation](https://huggingface.co/tasks/text-generation)
diff --git a/metrics/mauve/mauve.py b/metrics/mauve/mauve.py
new file mode 100644
index 0000000..c281fd8
--- /dev/null
+++ b/metrics/mauve/mauve.py
@@ -0,0 +1,149 @@
+# coding=utf-8
+# Copyright 2020 The HuggingFace Datasets Authors.
+#
+# Licensed under the Apache License, Version 2.0 (the "License");
+# you may not use this file except in compliance with the License.
+# You may obtain a copy of the License at
+#
+# http://www.apache.org/licenses/LICENSE-2.0
+#
+# Unless required by applicable law or agreed to in writing, software
+# distributed under the License is distributed on an "AS IS" BASIS,
+# WITHOUT WARRANTIES OR CONDITIONS OF ANY KIND, either express or implied.
+# See the License for the specific language governing permissions and
+# limitations under the License.
+""" MAUVE metric from https://github.com/krishnap25/mauve. """
+
+import faiss # Here to have a nice missing dependency error message early on
+import numpy # Here to have a nice missing dependency error message early on
+import requests # Here to have a nice missing dependency error message early on
+import sklearn # Here to have a nice missing dependency error message early on
+import tqdm # Here to have a nice missing dependency error message early on
+from mauve import compute_mauve # From: mauve-text
+
+import datasets
+
+
+_CITATION = """\
+@inproceedings{pillutla-etal:mauve:neurips2021,
+ title={MAUVE: Measuring the Gap Between Neural Text and Human Text using Divergence Frontiers},
+ author={Pillutla, Krishna and Swayamdipta, Swabha and Zellers, Rowan and Thickstun, John and Welleck, Sean and Choi, Yejin and Harchaoui, Zaid},
+ booktitle = {NeurIPS},
+ year = {2021}
+}
+
+"""
+
+_DESCRIPTION = """\
+MAUVE is a library built on PyTorch and HuggingFace Transformers to measure the gap between neural text and human text with the eponymous MAUVE measure.
+
+MAUVE summarizes both Type I and Type II errors measured softly using Kullback–Leibler (KL) divergences.
+
+For details, see the MAUVE paper: https://arxiv.org/abs/2102.01454 (Neurips, 2021).
+
+This metrics is a wrapper around the official implementation of MAUVE:
+https://github.com/krishnap25/mauve
+"""
+
+_KWARGS_DESCRIPTION = """
+Calculates MAUVE scores between two lists of generated text and reference text.
+Args:
+ predictions: list of generated text to score. Each predictions
+ should be a string with tokens separated by spaces.
+ references: list of reference for each prediction. Each
+ reference should be a string with tokens separated by spaces.
+Optional Args:
+ num_buckets: the size of the histogram to quantize P and Q. Options: 'auto' (default) or an integer
+ pca_max_data: the number data points to use for PCA dimensionality reduction prior to clustering. If -1, use all the data. Default -1
+ kmeans_explained_var: amount of variance of the data to keep in dimensionality reduction by PCA. Default 0.9
+ kmeans_num_redo: number of times to redo k-means clustering (the best objective is kept). Default 5
+ kmeans_max_iter: maximum number of k-means iterations. Default 500
+ featurize_model_name: name of the model from which features are obtained. Default 'gpt2-large' Use one of ['gpt2', 'gpt2-medium', 'gpt2-large', 'gpt2-xl'].
+ device_id: Device for featurization. Supply a GPU id (e.g. 0 or 3) to use GPU. If no GPU with this id is found, use CPU
+ max_text_length: maximum number of tokens to consider. Default 1024
+ divergence_curve_discretization_size: Number of points to consider on the divergence curve. Default 25
+ mauve_scaling_factor: "c" from the paper. Default 5.
+ verbose: If True (default), print running time updates
+ seed: random seed to initialize k-means cluster assignments.
+Returns:
+ mauve: MAUVE score, a number between 0 and 1. Larger values indicate that P and Q are closer,
+ frontier_integral: Frontier Integral, a number between 0 and 1. Smaller values indicate that P and Q are closer,
+ divergence_curve: a numpy.ndarray of shape (m, 2); plot it with matplotlib to view the divergence curve,
+ p_hist: a discrete distribution, which is a quantized version of the text distribution p_text,
+ q_hist: same as above, but with q_text.
+Examples:
+
+ >>> # faiss segfaults in doctest for some reason, so the .compute call is not tested with doctest
+ >>> import datasets
+ >>> mauve = datasets.load_metric('mauve')
+ >>> predictions = ["hello there", "general kenobi"]
+ >>> references = ["hello there", "general kenobi"]
+ >>> out = mauve.compute(predictions=predictions, references=references) # doctest: +SKIP
+ >>> print(out.mauve) # doctest: +SKIP
+ 1.0
+"""
+
+
+@datasets.utils.file_utils.add_start_docstrings(_DESCRIPTION, _KWARGS_DESCRIPTION)
+class Mauve(datasets.Metric):
+ def _info(self):
+ return datasets.MetricInfo(
+ description=_DESCRIPTION,
+ citation=_CITATION,
+ homepage="https://github.com/krishnap25/mauve",
+ inputs_description=_KWARGS_DESCRIPTION,
+ features=datasets.Features(
+ {
+ "predictions": datasets.Value("string", id="sequence"),
+ "references": datasets.Value("string", id="sequence"),
+ }
+ ),
+ codebase_urls=["https://github.com/krishnap25/mauve"],
+ reference_urls=[
+ "https://arxiv.org/abs/2102.01454",
+ "https://github.com/krishnap25/mauve",
+ ],
+ )
+
+ def _compute(
+ self,
+ predictions,
+ references,
+ p_features=None,
+ q_features=None,
+ p_tokens=None,
+ q_tokens=None,
+ num_buckets="auto",
+ pca_max_data=-1,
+ kmeans_explained_var=0.9,
+ kmeans_num_redo=5,
+ kmeans_max_iter=500,
+ featurize_model_name="gpt2-large",
+ device_id=-1,
+ max_text_length=1024,
+ divergence_curve_discretization_size=25,
+ mauve_scaling_factor=5,
+ verbose=True,
+ seed=25,
+ ):
+ out = compute_mauve(
+ p_text=predictions,
+ q_text=references,
+ p_features=p_features,
+ q_features=q_features,
+ p_tokens=p_tokens,
+ q_tokens=q_tokens,
+ num_buckets=num_buckets,
+ pca_max_data=pca_max_data,
+ kmeans_explained_var=kmeans_explained_var,
+ kmeans_num_redo=kmeans_num_redo,
+ kmeans_max_iter=kmeans_max_iter,
+ featurize_model_name=featurize_model_name,
+ device_id=device_id,
+ max_text_length=max_text_length,
+ divergence_curve_discretization_size=divergence_curve_discretization_size,
+ mauve_scaling_factor=mauve_scaling_factor,
+ verbose=verbose,
+ seed=seed,
+ )
+ return out
diff --git a/metrics/mean_iou/README.md b/metrics/mean_iou/README.md
new file mode 100644
index 0000000..b1abe2c
--- /dev/null
+++ b/metrics/mean_iou/README.md
@@ -0,0 +1,91 @@
+# Metric Card for Mean IoU
+
+
+## Metric Description
+
+IoU (Intersection over Union) is the area of overlap between the predicted segmentation and the ground truth divided by the area of union between the predicted segmentation and the ground truth.
+
+For binary (two classes) or multi-class segmentation, the *mean IoU* of the image is calculated by taking the IoU of each class and averaging them.
+
+## How to Use
+
+The Mean IoU metric takes two numeric arrays as input corresponding to the predicted and ground truth segmentations:
+```python
+>>> import numpy as np
+>>> mean_iou = datasets.load_metric("mean_iou")
+>>> predicted = np.array([[2, 2, 3], [8, 2, 4], [3, 255, 2]])
+>>> ground_truth = np.array([[1, 2, 2], [8, 2, 1], [3, 255, 1]])
+>>> results = mean_iou.compute(predictions=predicted, references=ground_truth, num_labels=10, ignore_index=255)
+```
+
+### Inputs
+**Mandatory inputs**
+- `predictions` (`List[ndarray]`): List of predicted segmentation maps, each of shape (height, width). Each segmentation map can be of a different size.
+- `references` (`List[ndarray]`): List of ground truth segmentation maps, each of shape (height, width). Each segmentation map can be of a different size.
+- `num_labels` (`int`): Number of classes (categories).
+- `ignore_index` (`int`): Index that will be ignored during evaluation.
+
+**Optional inputs**
+- `nan_to_num` (`int`): If specified, NaN values will be replaced by the number defined by the user.
+- `label_map` (`dict`): If specified, dictionary mapping old label indices to new label indices.
+- `reduce_labels` (`bool`): Whether or not to reduce all label values of segmentation maps by 1. Usually used for datasets where 0 is used for background, and background itself is not included in all classes of a dataset (e.g. ADE20k). The background label will be replaced by 255. The default value is `False`.
+
+### Output Values
+The metric returns a dictionary with the following elements:
+- `mean_iou` (`float`): Mean Intersection-over-Union (IoU averaged over all categories).
+- `mean_accuracy` (`float`): Mean accuracy (averaged over all categories).
+- `overall_accuracy` (`float`): Overall accuracy on all images.
+- `per_category_accuracy` (`ndarray` of shape `(num_labels,)`): Per category accuracy.
+- `per_category_iou` (`ndarray` of shape `(num_labels,)`): Per category IoU.
+
+The values of all of the scores reported range from from `0.0` (minimum) and `1.0` (maximum).
+
+Output Example:
+```python
+{'mean_iou': 0.47750000000000004, 'mean_accuracy': 0.5916666666666666, 'overall_accuracy': 0.5263157894736842, 'per_category_iou': array([0. , 0. , 0.375, 0.4 , 0.5 , 0. , 0.5 , 1. , 1. , 1. ]), 'per_category_accuracy': array([0. , 0. , 0.75 , 0.66666667, 1. , 0. , 0.5 , 1. , 1. , 1. ])}
+```
+
+#### Values from Popular Papers
+
+The [leaderboard for the CityScapes dataset](https://paperswithcode.com/sota/semantic-segmentation-on-cityscapes) reports a Mean IOU ranging from 64 to 84; that of [ADE20k](https://paperswithcode.com/sota/semantic-segmentation-on-ade20k) ranges from 30 to a peak of 59.9, indicating that the dataset is more difficult for current approaches (as of 2022).
+
+
+### Examples
+
+```python
+>>> from datasets import load_metric
+>>> import numpy as np
+>>> mean_iou = load_metric("mean_iou")
+>>> # suppose one has 3 different segmentation maps predicted
+>>> predicted_1 = np.array([[1, 2], [3, 4], [5, 255]])
+>>> actual_1 = np.array([[0, 3], [5, 4], [6, 255]])
+>>> predicted_2 = np.array([[2, 7], [9, 2], [3, 6]])
+>>> actual_2 = np.array([[1, 7], [9, 2], [3, 6]])
+>>> predicted_3 = np.array([[2, 2, 3], [8, 2, 4], [3, 255, 2]])
+>>> actual_3 = np.array([[1, 2, 2], [8, 2, 1], [3, 255, 1]])
+>>> predictions = [predicted_1, predicted_2, predicted_3]
+>>> references = [actual_1, actual_2, actual_3]
+>>> results = mean_iou.compute(predictions=predictions, references=references, num_labels=10, ignore_index=255, reduce_labels=False)
+>>> print(results) # doctest: +NORMALIZE_WHITESPACE
+{'mean_iou': 0.47750000000000004, 'mean_accuracy': 0.5916666666666666, 'overall_accuracy': 0.5263157894736842, 'per_category_iou': array([0. , 0. , 0.375, 0.4 , 0.5 , 0. , 0.5 , 1. , 1. , 1. ]), 'per_category_accuracy': array([0. , 0. , 0.75 , 0.66666667, 1. , 0. , 0.5 , 1. , 1. , 1. ])}
+```
+
+
+## Limitations and Bias
+Mean IOU is an average metric, so it will not show you where model predictions differ from the ground truth (i.e. if there are particular regions or classes that the model does poorly on). Further error analysis is needed to gather actional insights that can be used to inform model improvements.
+
+## Citation(s)
+```bibtex
+@software{MMSegmentation_Contributors_OpenMMLab_Semantic_Segmentation_2020,
+author = {{MMSegmentation Contributors}},
+license = {Apache-2.0},
+month = {7},
+title = {{OpenMMLab Semantic Segmentation Toolbox and Benchmark}},
+url = {https://github.com/open-mmlab/mmsegmentation},
+year = {2020}
+}"
+```
+
+
+## Further References
+- [Wikipedia article - Jaccard Index](https://en.wikipedia.org/wiki/Jaccard_index)
diff --git a/metrics/mean_iou/mean_iou.py b/metrics/mean_iou/mean_iou.py
new file mode 100644
index 0000000..36139e8
--- /dev/null
+++ b/metrics/mean_iou/mean_iou.py
@@ -0,0 +1,313 @@
+# Copyright 2022 The HuggingFace Datasets Authors.
+#
+# Licensed under the Apache License, Version 2.0 (the "License");
+# you may not use this file except in compliance with the License.
+# You may obtain a copy of the License at
+#
+# http://www.apache.org/licenses/LICENSE-2.0
+#
+# Unless required by applicable law or agreed to in writing, software
+# distributed under the License is distributed on an "AS IS" BASIS,
+# WITHOUT WARRANTIES OR CONDITIONS OF ANY KIND, either express or implied.
+# See the License for the specific language governing permissions and
+# limitations under the License.
+"""Mean IoU (Intersection-over-Union) metric."""
+
+from typing import Dict, Optional
+
+import numpy as np
+
+import datasets
+
+
+_DESCRIPTION = """
+IoU is the area of overlap between the predicted segmentation and the ground truth divided by the area of union
+between the predicted segmentation and the ground truth. For binary (two classes) or multi-class segmentation,
+the mean IoU of the image is calculated by taking the IoU of each class and averaging them.
+"""
+
+_KWARGS_DESCRIPTION = """
+Args:
+ predictions (`List[ndarray]`):
+ List of predicted segmentation maps, each of shape (height, width). Each segmentation map can be of a different size.
+ references (`List[ndarray]`):
+ List of ground truth segmentation maps, each of shape (height, width). Each segmentation map can be of a different size.
+ num_labels (`int`):
+ Number of classes (categories).
+ ignore_index (`int`):
+ Index that will be ignored during evaluation.
+ nan_to_num (`int`, *optional*):
+ If specified, NaN values will be replaced by the number defined by the user.
+ label_map (`dict`, *optional*):
+ If specified, dictionary mapping old label indices to new label indices.
+ reduce_labels (`bool`, *optional*, defaults to `False`):
+ Whether or not to reduce all label values of segmentation maps by 1. Usually used for datasets where 0 is used for background,
+ and background itself is not included in all classes of a dataset (e.g. ADE20k). The background label will be replaced by 255.
+
+Returns:
+ `Dict[str, float | ndarray]` comprising various elements:
+ - *mean_iou* (`float`):
+ Mean Intersection-over-Union (IoU averaged over all categories).
+ - *mean_accuracy* (`float`):
+ Mean accuracy (averaged over all categories).
+ - *overall_accuracy* (`float`):
+ Overall accuracy on all images.
+ - *per_category_accuracy* (`ndarray` of shape `(num_labels,)`):
+ Per category accuracy.
+ - *per_category_iou* (`ndarray` of shape `(num_labels,)`):
+ Per category IoU.
+
+Examples:
+
+ >>> import numpy as np
+
+ >>> mean_iou = datasets.load_metric("mean_iou")
+
+ >>> # suppose one has 3 different segmentation maps predicted
+ >>> predicted_1 = np.array([[1, 2], [3, 4], [5, 255]])
+ >>> actual_1 = np.array([[0, 3], [5, 4], [6, 255]])
+
+ >>> predicted_2 = np.array([[2, 7], [9, 2], [3, 6]])
+ >>> actual_2 = np.array([[1, 7], [9, 2], [3, 6]])
+
+ >>> predicted_3 = np.array([[2, 2, 3], [8, 2, 4], [3, 255, 2]])
+ >>> actual_3 = np.array([[1, 2, 2], [8, 2, 1], [3, 255, 1]])
+
+ >>> predicted = [predicted_1, predicted_2, predicted_3]
+ >>> ground_truth = [actual_1, actual_2, actual_3]
+
+ >>> results = mean_iou.compute(predictions=predicted, references=ground_truth, num_labels=10, ignore_index=255, reduce_labels=False)
+ >>> print(results) # doctest: +NORMALIZE_WHITESPACE
+ {'mean_iou': 0.47750000000000004, 'mean_accuracy': 0.5916666666666666, 'overall_accuracy': 0.5263157894736842, 'per_category_iou': array([0. , 0. , 0.375, 0.4 , 0.5 , 0. , 0.5 , 1. , 1. , 1. ]), 'per_category_accuracy': array([0. , 0. , 0.75 , 0.66666667, 1. , 0. , 0.5 , 1. , 1. , 1. ])}
+"""
+
+_CITATION = """\
+@software{MMSegmentation_Contributors_OpenMMLab_Semantic_Segmentation_2020,
+author = {{MMSegmentation Contributors}},
+license = {Apache-2.0},
+month = {7},
+title = {{OpenMMLab Semantic Segmentation Toolbox and Benchmark}},
+url = {https://github.com/open-mmlab/mmsegmentation},
+year = {2020}
+}"""
+
+
+def intersect_and_union(
+ pred_label,
+ label,
+ num_labels,
+ ignore_index: bool,
+ label_map: Optional[Dict[int, int]] = None,
+ reduce_labels: bool = False,
+):
+ """Calculate intersection and Union.
+
+ Args:
+ pred_label (`ndarray`):
+ Prediction segmentation map of shape (height, width).
+ label (`ndarray`):
+ Ground truth segmentation map of shape (height, width).
+ num_labels (`int`):
+ Number of categories.
+ ignore_index (`int`):
+ Index that will be ignored during evaluation.
+ label_map (`dict`, *optional*):
+ Mapping old labels to new labels. The parameter will work only when label is str.
+ reduce_labels (`bool`, *optional*, defaults to `False`):
+ Whether or not to reduce all label values of segmentation maps by 1. Usually used for datasets where 0 is used for background,
+ and background itself is not included in all classes of a dataset (e.g. ADE20k). The background label will be replaced by 255.
+
+ Returns:
+ area_intersect (`ndarray`):
+ The intersection of prediction and ground truth histogram on all classes.
+ area_union (`ndarray`):
+ The union of prediction and ground truth histogram on all classes.
+ area_pred_label (`ndarray`):
+ The prediction histogram on all classes.
+ area_label (`ndarray`):
+ The ground truth histogram on all classes.
+ """
+ if label_map is not None:
+ for old_id, new_id in label_map.items():
+ label[label == old_id] = new_id
+
+ # turn into Numpy arrays
+ pred_label = np.array(pred_label)
+ label = np.array(label)
+
+ if reduce_labels:
+ label[label == 0] = 255
+ label = label - 1
+ label[label == 254] = 255
+
+ mask = label != ignore_index
+ mask = np.not_equal(label, ignore_index)
+ pred_label = pred_label[mask]
+ label = np.array(label)[mask]
+
+ intersect = pred_label[pred_label == label]
+
+ area_intersect = np.histogram(intersect, bins=num_labels, range=(0, num_labels - 1))[0]
+ area_pred_label = np.histogram(pred_label, bins=num_labels, range=(0, num_labels - 1))[0]
+ area_label = np.histogram(label, bins=num_labels, range=(0, num_labels - 1))[0]
+
+ area_union = area_pred_label + area_label - area_intersect
+
+ return area_intersect, area_union, area_pred_label, area_label
+
+
+def total_intersect_and_union(
+ results,
+ gt_seg_maps,
+ num_labels,
+ ignore_index: bool,
+ label_map: Optional[Dict[int, int]] = None,
+ reduce_labels: bool = False,
+):
+ """Calculate Total Intersection and Union, by calculating `intersect_and_union` for each (predicted, ground truth) pair.
+
+ Args:
+ results (`ndarray`):
+ List of prediction segmentation maps, each of shape (height, width).
+ gt_seg_maps (`ndarray`):
+ List of ground truth segmentation maps, each of shape (height, width).
+ num_labels (`int`):
+ Number of categories.
+ ignore_index (`int`):
+ Index that will be ignored during evaluation.
+ label_map (`dict`, *optional*):
+ Mapping old labels to new labels. The parameter will work only when label is str.
+ reduce_labels (`bool`, *optional*, defaults to `False`):
+ Whether or not to reduce all label values of segmentation maps by 1. Usually used for datasets where 0 is used for background,
+ and background itself is not included in all classes of a dataset (e.g. ADE20k). The background label will be replaced by 255.
+
+ Returns:
+ total_area_intersect (`ndarray`):
+ The intersection of prediction and ground truth histogram on all classes.
+ total_area_union (`ndarray`):
+ The union of prediction and ground truth histogram on all classes.
+ total_area_pred_label (`ndarray`):
+ The prediction histogram on all classes.
+ total_area_label (`ndarray`):
+ The ground truth histogram on all classes.
+ """
+ total_area_intersect = np.zeros((num_labels,), dtype=np.float64)
+ total_area_union = np.zeros((num_labels,), dtype=np.float64)
+ total_area_pred_label = np.zeros((num_labels,), dtype=np.float64)
+ total_area_label = np.zeros((num_labels,), dtype=np.float64)
+ for result, gt_seg_map in zip(results, gt_seg_maps):
+ area_intersect, area_union, area_pred_label, area_label = intersect_and_union(
+ result, gt_seg_map, num_labels, ignore_index, label_map, reduce_labels
+ )
+ total_area_intersect += area_intersect
+ total_area_union += area_union
+ total_area_pred_label += area_pred_label
+ total_area_label += area_label
+ return total_area_intersect, total_area_union, total_area_pred_label, total_area_label
+
+
+def mean_iou(
+ results,
+ gt_seg_maps,
+ num_labels,
+ ignore_index: bool,
+ nan_to_num: Optional[int] = None,
+ label_map: Optional[Dict[int, int]] = None,
+ reduce_labels: bool = False,
+):
+ """Calculate Mean Intersection and Union (mIoU).
+
+ Args:
+ results (`ndarray`):
+ List of prediction segmentation maps, each of shape (height, width).
+ gt_seg_maps (`ndarray`):
+ List of ground truth segmentation maps, each of shape (height, width).
+ num_labels (`int`):
+ Number of categories.
+ ignore_index (`int`):
+ Index that will be ignored during evaluation.
+ nan_to_num (`int`, *optional*):
+ If specified, NaN values will be replaced by the number defined by the user.
+ label_map (`dict`, *optional*):
+ Mapping old labels to new labels. The parameter will work only when label is str.
+ reduce_labels (`bool`, *optional*, defaults to `False`):
+ Whether or not to reduce all label values of segmentation maps by 1. Usually used for datasets where 0 is used for background,
+ and background itself is not included in all classes of a dataset (e.g. ADE20k). The background label will be replaced by 255.
+
+ Returns:
+ `Dict[str, float | ndarray]` comprising various elements:
+ - *mean_iou* (`float`):
+ Mean Intersection-over-Union (IoU averaged over all categories).
+ - *mean_accuracy* (`float`):
+ Mean accuracy (averaged over all categories).
+ - *overall_accuracy* (`float`):
+ Overall accuracy on all images.
+ - *per_category_accuracy* (`ndarray` of shape `(num_labels,)`):
+ Per category accuracy.
+ - *per_category_iou* (`ndarray` of shape `(num_labels,)`):
+ Per category IoU.
+ """
+ total_area_intersect, total_area_union, total_area_pred_label, total_area_label = total_intersect_and_union(
+ results, gt_seg_maps, num_labels, ignore_index, label_map, reduce_labels
+ )
+
+ # compute metrics
+ metrics = dict()
+
+ all_acc = total_area_intersect.sum() / total_area_label.sum()
+ iou = total_area_intersect / total_area_union
+ acc = total_area_intersect / total_area_label
+
+ metrics["mean_iou"] = np.nanmean(iou)
+ metrics["mean_accuracy"] = np.nanmean(acc)
+ metrics["overall_accuracy"] = all_acc
+ metrics["per_category_iou"] = iou
+ metrics["per_category_accuracy"] = acc
+
+ if nan_to_num is not None:
+ metrics = dict(
+ {metric: np.nan_to_num(metric_value, nan=nan_to_num) for metric, metric_value in metrics.items()}
+ )
+
+ return metrics
+
+
+@datasets.utils.file_utils.add_start_docstrings(_DESCRIPTION, _KWARGS_DESCRIPTION)
+class MeanIoU(datasets.Metric):
+ def _info(self):
+ return datasets.MetricInfo(
+ description=_DESCRIPTION,
+ citation=_CITATION,
+ inputs_description=_KWARGS_DESCRIPTION,
+ features=datasets.Features(
+ # 1st Seq - height dim, 2nd - width dim
+ {
+ "predictions": datasets.Sequence(datasets.Sequence(datasets.Value("uint16"))),
+ "references": datasets.Sequence(datasets.Sequence(datasets.Value("uint16"))),
+ }
+ ),
+ reference_urls=[
+ "https://github.com/open-mmlab/mmsegmentation/blob/71c201b1813267d78764f306a297ca717827c4bf/mmseg/core/evaluation/metrics.py"
+ ],
+ )
+
+ def _compute(
+ self,
+ predictions,
+ references,
+ num_labels: int,
+ ignore_index: bool,
+ nan_to_num: Optional[int] = None,
+ label_map: Optional[Dict[int, int]] = None,
+ reduce_labels: bool = False,
+ ):
+ iou_result = mean_iou(
+ results=predictions,
+ gt_seg_maps=references,
+ num_labels=num_labels,
+ ignore_index=ignore_index,
+ nan_to_num=nan_to_num,
+ label_map=label_map,
+ reduce_labels=reduce_labels,
+ )
+ return iou_result
diff --git a/metrics/meteor/README.md b/metrics/meteor/README.md
new file mode 100644
index 0000000..ba54a21
--- /dev/null
+++ b/metrics/meteor/README.md
@@ -0,0 +1,109 @@
+# Metric Card for METEOR
+
+## Metric description
+
+METEOR (Metric for Evaluation of Translation with Explicit ORdering) is a machine translation evaluation metric, which is calculated based on the harmonic mean of precision and recall, with recall weighted more than precision.
+
+METEOR is based on a generalized concept of unigram matching between the machine-produced translation and human-produced reference translations. Unigrams can be matched based on their surface forms, stemmed forms, and meanings. Once all generalized unigram matches between the two strings have been found, METEOR computes a score for this matching using a combination of unigram-precision, unigram-recall, and a measure of fragmentation that is designed to directly capture how well-ordered the matched words in the machine translation are in relation to the reference.
+
+
+## How to use
+
+METEOR has two mandatory arguments:
+
+`predictions`: a list of predictions to score. Each prediction should be a string with tokens separated by spaces.
+
+`references`: a list of references for each prediction. Each reference should be a string with tokens separated by spaces.
+
+It also has several optional parameters:
+
+`alpha`: Parameter for controlling relative weights of precision and recall. The default value is `0.9`.
+
+`beta`: Parameter for controlling shape of penalty as a function of fragmentation. The default value is `3`.
+
+`gamma`: The relative weight assigned to fragmentation penalty. The default is `0.5`.
+
+Refer to the [METEOR paper](https://aclanthology.org/W05-0909.pdf) for more information about parameter values and ranges.
+
+```python
+>>> from datasets import load_metric
+>>> meteor = load_metric('meteor')
+>>> predictions = ["It is a guide to action which ensures that the military always obeys the commands of the party"]
+>>> references = ["It is a guide to action that ensures that the military will forever heed Party commands"]
+>>> results = meteor.compute(predictions=predictions, references=references)
+```
+
+## Output values
+
+The metric outputs a dictionary containing the METEOR score. Its values range from 0 to 1.
+
+
+### Values from popular papers
+The [METEOR paper](https://aclanthology.org/W05-0909.pdf) does not report METEOR score values for different models, but it does report that METEOR gets an R correlation value of 0.347 with human evaluation on the Arabic data and 0.331 on the Chinese data.
+
+
+## Examples
+
+Maximal values :
+
+```python
+>>> from datasets import load_metric
+>>> meteor = load_metric('meteor')
+>>> predictions = ["It is a guide to action which ensures that the military always obeys the commands of the party"]
+>>> references = ["It is a guide to action which ensures that the military always obeys the commands of the party"]
+>>> results = meteor.compute(predictions=predictions, references=references)
+>>> print(round(results['meteor'], 2))
+1.0
+```
+
+Minimal values:
+
+```python
+>>> from datasets import load_metric
+>>> meteor = load_metric('meteor')
+>>> predictions = ["It is a guide to action which ensures that the military always obeys the commands of the party"]
+>>> references = ["Hello world"]
+>>> results = meteor.compute(predictions=predictions, references=references)
+>>> print(round(results['meteor'], 2))
+0.0
+```
+
+Partial match:
+
+```python
+>>> from datasets import load_metric
+>>> meteor = load_metric('meteor')
+>>> predictions = ["It is a guide to action which ensures that the military always obeys the commands of the party"]
+>>> references = ["It is a guide to action that ensures that the military will forever heed Party commands"]
+>>> results = meteor.compute(predictions=predictions, references=references)
+>>> print(round(results['meteor'], 2))
+0.69
+```
+
+## Limitations and bias
+
+While the correlation between METEOR and human judgments was measured for Chinese and Arabic and found to be significant, further experimentation is needed to check its correlation for other languages.
+
+Furthermore, while the alignment and matching done in METEOR is based on unigrams, using multiple word entities (e.g. bigrams) could contribute to improving its accuracy -- this has been proposed in [more recent publications](https://www.cs.cmu.edu/~alavie/METEOR/pdf/meteor-naacl-2010.pdf) on the subject.
+
+
+## Citation
+
+```bibtex
+@inproceedings{banarjee2005,
+ title = {{METEOR}: An Automatic Metric for {MT} Evaluation with Improved Correlation with Human Judgments},
+ author = {Banerjee, Satanjeev and Lavie, Alon},
+ booktitle = {Proceedings of the {ACL} Workshop on Intrinsic and Extrinsic Evaluation Measures for Machine Translation and/or Summarization},
+ month = jun,
+ year = {2005},
+ address = {Ann Arbor, Michigan},
+ publisher = {Association for Computational Linguistics},
+ url = {https://www.aclweb.org/anthology/W05-0909},
+ pages = {65--72},
+}
+```
+
+## Further References
+- [METEOR -- Wikipedia](https://en.wikipedia.org/wiki/METEOR)
+- [METEOR score -- NLTK](https://www.nltk.org/_modules/nltk/translate/meteor_score.html)
+
diff --git a/metrics/meteor/meteor.py b/metrics/meteor/meteor.py
new file mode 100644
index 0000000..835e5de
--- /dev/null
+++ b/metrics/meteor/meteor.py
@@ -0,0 +1,127 @@
+# Copyright 2020 The HuggingFace Datasets Authors.
+#
+# Licensed under the Apache License, Version 2.0 (the "License");
+# you may not use this file except in compliance with the License.
+# You may obtain a copy of the License at
+#
+# http://www.apache.org/licenses/LICENSE-2.0
+#
+# Unless required by applicable law or agreed to in writing, software
+# distributed under the License is distributed on an "AS IS" BASIS,
+# WITHOUT WARRANTIES OR CONDITIONS OF ANY KIND, either express or implied.
+# See the License for the specific language governing permissions and
+# limitations under the License.
+""" METEOR metric. """
+
+import numpy as np
+from nltk.translate import meteor_score
+
+import datasets
+from datasets.config import importlib_metadata, version
+
+
+NLTK_VERSION = version.parse(importlib_metadata.version("nltk"))
+if NLTK_VERSION >= version.Version("3.6.4"):
+ from nltk import word_tokenize
+
+
+_CITATION = """\
+@inproceedings{banarjee2005,
+ title = {{METEOR}: An Automatic Metric for {MT} Evaluation with Improved Correlation with Human Judgments},
+ author = {Banerjee, Satanjeev and Lavie, Alon},
+ booktitle = {Proceedings of the {ACL} Workshop on Intrinsic and Extrinsic Evaluation Measures for Machine Translation and/or Summarization},
+ month = jun,
+ year = {2005},
+ address = {Ann Arbor, Michigan},
+ publisher = {Association for Computational Linguistics},
+ url = {https://www.aclweb.org/anthology/W05-0909},
+ pages = {65--72},
+}
+"""
+
+_DESCRIPTION = """\
+METEOR, an automatic metric for machine translation evaluation
+that is based on a generalized concept of unigram matching between the
+machine-produced translation and human-produced reference translations.
+Unigrams can be matched based on their surface forms, stemmed forms,
+and meanings; furthermore, METEOR can be easily extended to include more
+advanced matching strategies. Once all generalized unigram matches
+between the two strings have been found, METEOR computes a score for
+this matching using a combination of unigram-precision, unigram-recall, and
+a measure of fragmentation that is designed to directly capture how
+well-ordered the matched words in the machine translation are in relation
+to the reference.
+
+METEOR gets an R correlation value of 0.347 with human evaluation on the Arabic
+data and 0.331 on the Chinese data. This is shown to be an improvement on
+using simply unigram-precision, unigram-recall and their harmonic F1
+combination.
+"""
+
+_KWARGS_DESCRIPTION = """
+Computes METEOR score of translated segments against one or more references.
+Args:
+ predictions: list of predictions to score. Each prediction
+ should be a string with tokens separated by spaces.
+ references: list of reference for each prediction. Each
+ reference should be a string with tokens separated by spaces.
+ alpha: Parameter for controlling relative weights of precision and recall. default: 0.9
+ beta: Parameter for controlling shape of penalty as a function of fragmentation. default: 3
+ gamma: Relative weight assigned to fragmentation penalty. default: 0.5
+Returns:
+ 'meteor': meteor score.
+Examples:
+
+ >>> meteor = datasets.load_metric('meteor')
+ >>> predictions = ["It is a guide to action which ensures that the military always obeys the commands of the party"]
+ >>> references = ["It is a guide to action that ensures that the military will forever heed Party commands"]
+ >>> results = meteor.compute(predictions=predictions, references=references)
+ >>> print(round(results["meteor"], 4))
+ 0.6944
+"""
+
+
+@datasets.utils.file_utils.add_start_docstrings(_DESCRIPTION, _KWARGS_DESCRIPTION)
+class Meteor(datasets.Metric):
+ def _info(self):
+ return datasets.MetricInfo(
+ description=_DESCRIPTION,
+ citation=_CITATION,
+ inputs_description=_KWARGS_DESCRIPTION,
+ features=datasets.Features(
+ {
+ "predictions": datasets.Value("string", id="sequence"),
+ "references": datasets.Value("string", id="sequence"),
+ }
+ ),
+ codebase_urls=["https://github.com/nltk/nltk/blob/develop/nltk/translate/meteor_score.py"],
+ reference_urls=[
+ "https://www.nltk.org/api/nltk.translate.html#module-nltk.translate.meteor_score",
+ "https://en.wikipedia.org/wiki/METEOR",
+ ],
+ )
+
+ def _download_and_prepare(self, dl_manager):
+ import nltk
+
+ nltk.download("wordnet")
+ if NLTK_VERSION >= version.Version("3.6.5"):
+ nltk.download("punkt")
+ if NLTK_VERSION >= version.Version("3.6.6"):
+ nltk.download("omw-1.4")
+
+ def _compute(self, predictions, references, alpha=0.9, beta=3, gamma=0.5):
+ if NLTK_VERSION >= version.Version("3.6.5"):
+ scores = [
+ meteor_score.single_meteor_score(
+ word_tokenize(ref), word_tokenize(pred), alpha=alpha, beta=beta, gamma=gamma
+ )
+ for ref, pred in zip(references, predictions)
+ ]
+ else:
+ scores = [
+ meteor_score.single_meteor_score(ref, pred, alpha=alpha, beta=beta, gamma=gamma)
+ for ref, pred in zip(references, predictions)
+ ]
+
+ return {"meteor": np.mean(scores)}
diff --git a/metrics/mse/README.md b/metrics/mse/README.md
new file mode 100644
index 0000000..421b1b8
--- /dev/null
+++ b/metrics/mse/README.md
@@ -0,0 +1,123 @@
+# Metric Card for MSE
+
+
+## Metric Description
+
+Mean Squared Error(MSE) represents the average of the squares of errors -- i.e. the average squared difference between the estimated values and the actual values.
+
+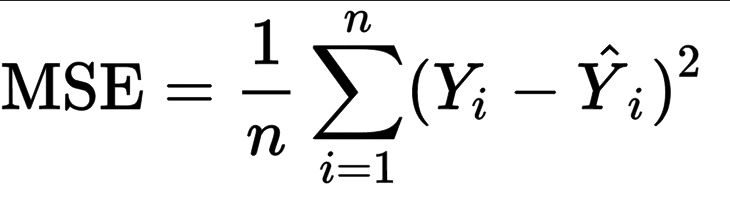
+
+## How to Use
+
+At minimum, this metric requires predictions and references as inputs.
+
+```python
+>>> mse_metric = datasets.load_metric("mse")
+>>> predictions = [2.5, 0.0, 2, 8]
+>>> references = [3, -0.5, 2, 7]
+>>> results = mse_metric.compute(predictions=predictions, references=references)
+```
+
+### Inputs
+
+Mandatory inputs:
+- `predictions`: numeric array-like of shape (`n_samples,`) or (`n_samples`, `n_outputs`), representing the estimated target values.
+- `references`: numeric array-like of shape (`n_samples,`) or (`n_samples`, `n_outputs`), representing the ground truth (correct) target values.
+
+Optional arguments:
+- `sample_weight`: numeric array-like of shape (`n_samples,`) representing sample weights. The default is `None`.
+- `multioutput`: `raw_values`, `uniform_average` or numeric array-like of shape (`n_outputs,`), which defines the aggregation of multiple output values. The default value is `uniform_average`.
+ - `raw_values` returns a full set of errors in case of multioutput input.
+ - `uniform_average` means that the errors of all outputs are averaged with uniform weight.
+ - the array-like value defines weights used to average errors.
+- `squared` (`bool`): If `True` returns MSE value, if `False` returns RMSE (Root Mean Squared Error). The default value is `True`.
+
+
+### Output Values
+This metric outputs a dictionary, containing the mean squared error score, which is of type:
+- `float`: if multioutput is `uniform_average` or an ndarray of weights, then the weighted average of all output errors is returned.
+- numeric array-like of shape (`n_outputs,`): if multioutput is `raw_values`, then the score is returned for each output separately.
+
+Each MSE `float` value ranges from `0.0` to `1.0`, with the best value being `0.0`.
+
+Output Example(s):
+```python
+{'mse': 0.5}
+```
+
+If `multioutput="raw_values"`:
+```python
+{'mse': array([0.41666667, 1. ])}
+```
+
+#### Values from Popular Papers
+
+
+### Examples
+
+Example with the `uniform_average` config:
+```python
+>>> from datasets import load_metric
+>>> mse_metric = load_metric("mse")
+>>> predictions = [2.5, 0.0, 2, 8]
+>>> references = [3, -0.5, 2, 7]
+>>> results = mse_metric.compute(predictions=predictions, references=references)
+>>> print(results)
+{'mse': 0.375}
+```
+
+Example with `squared = True`, which returns the RMSE:
+```python
+>>> from datasets import load_metric
+>>> mse_metric = load_metric("mse")
+>>> predictions = [2.5, 0.0, 2, 8]
+>>> references = [3, -0.5, 2, 7]
+>>> rmse_result = mse_metric.compute(predictions=predictions, references=references, squared=False)
+>>> print(rmse_result)
+{'mse': 0.6123724356957945}
+```
+
+Example with multi-dimensional lists, and the `raw_values` config:
+```python
+>>> from datasets import load_metric
+>>> mse_metric = load_metric("mse", "multilist")
+>>> predictions = [[0.5, 1], [-1, 1], [7, -6]]
+>>> references = [[0, 2], [-1, 2], [8, -5]]
+>>> results = mse_metric.compute(predictions=predictions, references=references, multioutput='raw_values')
+>>> print(results)
+{'mse': array([0.41666667, 1. ])}
+"""
+```
+
+## Limitations and Bias
+MSE has the disadvantage of heavily weighting outliers -- given that it squares them, this results in large errors weighing more heavily than small ones. It can be used alongside [MAE](https://huggingface.co/metrics/mae), which is complementary given that it does not square the errors.
+
+## Citation(s)
+```bibtex
+@article{scikit-learn,
+ title={Scikit-learn: Machine Learning in {P}ython},
+ author={Pedregosa, F. and Varoquaux, G. and Gramfort, A. and Michel, V.
+ and Thirion, B. and Grisel, O. and Blondel, M. and Prettenhofer, P.
+ and Weiss, R. and Dubourg, V. and Vanderplas, J. and Passos, A. and
+ Cournapeau, D. and Brucher, M. and Perrot, M. and Duchesnay, E.},
+ journal={Journal of Machine Learning Research},
+ volume={12},
+ pages={2825--2830},
+ year={2011}
+}
+```
+
+```bibtex
+@article{willmott2005advantages,
+ title={Advantages of the mean absolute error (MAE) over the root mean square error (RMSE) in assessing average model performance},
+ author={Willmott, Cort J and Matsuura, Kenji},
+ journal={Climate research},
+ volume={30},
+ number={1},
+ pages={79--82},
+ year={2005}
+}
+```
+
+## Further References
+- [Mean Squared Error - Wikipedia](https://en.wikipedia.org/wiki/Mean_squared_error)
diff --git a/metrics/mse/mse.py b/metrics/mse/mse.py
new file mode 100644
index 0000000..b395c46
--- /dev/null
+++ b/metrics/mse/mse.py
@@ -0,0 +1,118 @@
+# Copyright 2022 The HuggingFace Datasets Authors and the current dataset script contributor.
+#
+# Licensed under the Apache License, Version 2.0 (the "License");
+# you may not use this file except in compliance with the License.
+# You may obtain a copy of the License at
+#
+# http://www.apache.org/licenses/LICENSE-2.0
+#
+# Unless required by applicable law or agreed to in writing, software
+# distributed under the License is distributed on an "AS IS" BASIS,
+# WITHOUT WARRANTIES OR CONDITIONS OF ANY KIND, either express or implied.
+# See the License for the specific language governing permissions and
+# limitations under the License.
+"""MSE - Mean Squared Error Metric"""
+
+from sklearn.metrics import mean_squared_error
+
+import datasets
+
+
+_CITATION = """\
+@article{scikit-learn,
+ title={Scikit-learn: Machine Learning in {P}ython},
+ author={Pedregosa, F. and Varoquaux, G. and Gramfort, A. and Michel, V.
+ and Thirion, B. and Grisel, O. and Blondel, M. and Prettenhofer, P.
+ and Weiss, R. and Dubourg, V. and Vanderplas, J. and Passos, A. and
+ Cournapeau, D. and Brucher, M. and Perrot, M. and Duchesnay, E.},
+ journal={Journal of Machine Learning Research},
+ volume={12},
+ pages={2825--2830},
+ year={2011}
+}
+"""
+
+_DESCRIPTION = """\
+Mean Squared Error(MSE) is the average of the square of difference between the predicted
+and actual values.
+"""
+
+
+_KWARGS_DESCRIPTION = """
+Args:
+ predictions: array-like of shape (n_samples,) or (n_samples, n_outputs)
+ Estimated target values.
+ references: array-like of shape (n_samples,) or (n_samples, n_outputs)
+ Ground truth (correct) target values.
+ sample_weight: array-like of shape (n_samples,), default=None
+ Sample weights.
+ multioutput: {"raw_values", "uniform_average"} or array-like of shape (n_outputs,), default="uniform_average"
+ Defines aggregating of multiple output values. Array-like value defines weights used to average errors.
+
+ "raw_values" : Returns a full set of errors in case of multioutput input.
+
+ "uniform_average" : Errors of all outputs are averaged with uniform weight.
+
+ squared : bool, default=True
+ If True returns MSE value, if False returns RMSE (Root Mean Squared Error) value.
+
+Returns:
+ mse : mean squared error.
+Examples:
+
+ >>> mse_metric = datasets.load_metric("mse")
+ >>> predictions = [2.5, 0.0, 2, 8]
+ >>> references = [3, -0.5, 2, 7]
+ >>> results = mse_metric.compute(predictions=predictions, references=references)
+ >>> print(results)
+ {'mse': 0.375}
+ >>> rmse_result = mse_metric.compute(predictions=predictions, references=references, squared=False)
+ >>> print(rmse_result)
+ {'mse': 0.6123724356957945}
+
+ If you're using multi-dimensional lists, then set the config as follows :
+
+ >>> mse_metric = datasets.load_metric("mse", "multilist")
+ >>> predictions = [[0.5, 1], [-1, 1], [7, -6]]
+ >>> references = [[0, 2], [-1, 2], [8, -5]]
+ >>> results = mse_metric.compute(predictions=predictions, references=references)
+ >>> print(results)
+ {'mse': 0.7083333333333334}
+ >>> results = mse_metric.compute(predictions=predictions, references=references, multioutput='raw_values')
+ >>> print(results) # doctest: +NORMALIZE_WHITESPACE
+ {'mse': array([0.41666667, 1. ])}
+"""
+
+
+@datasets.utils.file_utils.add_start_docstrings(_DESCRIPTION, _KWARGS_DESCRIPTION)
+class Mse(datasets.Metric):
+ def _info(self):
+ return datasets.MetricInfo(
+ description=_DESCRIPTION,
+ citation=_CITATION,
+ inputs_description=_KWARGS_DESCRIPTION,
+ features=datasets.Features(self._get_feature_types()),
+ reference_urls=[
+ "https://scikit-learn.org/stable/modules/generated/sklearn.metrics.mean_squared_error.html"
+ ],
+ )
+
+ def _get_feature_types(self):
+ if self.config_name == "multilist":
+ return {
+ "predictions": datasets.Sequence(datasets.Value("float")),
+ "references": datasets.Sequence(datasets.Value("float")),
+ }
+ else:
+ return {
+ "predictions": datasets.Value("float"),
+ "references": datasets.Value("float"),
+ }
+
+ def _compute(self, predictions, references, sample_weight=None, multioutput="uniform_average", squared=True):
+
+ mse = mean_squared_error(
+ references, predictions, sample_weight=sample_weight, multioutput=multioutput, squared=squared
+ )
+
+ return {"mse": mse}
diff --git a/metrics/pearsonr/README.md b/metrics/pearsonr/README.md
new file mode 100644
index 0000000..48f259e
--- /dev/null
+++ b/metrics/pearsonr/README.md
@@ -0,0 +1,101 @@
+# Metric Card for Pearson Correlation Coefficient (pearsonr)
+
+
+## Metric Description
+
+Pearson correlation coefficient and p-value for testing non-correlation.
+The Pearson correlation coefficient measures the linear relationship between two datasets. The calculation of the p-value relies on the assumption that each dataset is normally distributed. Like other correlation coefficients, this one varies between -1 and +1 with 0 implying no correlation. Correlations of -1 or +1 imply an exact linear relationship. Positive correlations imply that as x increases, so does y. Negative correlations imply that as x increases, y decreases.
+The p-value roughly indicates the probability of an uncorrelated system producing datasets that have a Pearson correlation at least as extreme as the one computed from these datasets.
+
+
+## How to Use
+
+This metric takes a list of predictions and a list of references as input
+
+```python
+>>> pearsonr_metric = datasets.load_metric("pearsonr")
+>>> results = pearsonr_metric.compute(predictions=[10, 9, 2.5, 6, 4], references=[1, 2, 3, 4, 5])
+>>> print(round(results['pearsonr']), 2)
+['-0.74']
+```
+
+
+### Inputs
+- **predictions** (`list` of `int`): Predicted class labels, as returned by a model.
+- **references** (`list` of `int`): Ground truth labels.
+- **return_pvalue** (`boolean`): If `True`, returns the p-value, along with the correlation coefficient. If `False`, returns only the correlation coefficient. Defaults to `False`.
+
+
+### Output Values
+- **pearsonr**(`float`): Pearson correlation coefficient. Minimum possible value is -1. Maximum possible value is 1. Values of 1 and -1 indicate exact linear positive and negative relationships, respectively. A value of 0 implies no correlation.
+- **p-value**(`float`): P-value, which roughly indicates the probability of an The p-value roughly indicates the probability of an uncorrelated system producing datasets that have a Pearson correlation at least as extreme as the one computed from these datasets. Minimum possible value is 0. Maximum possible value is 1. Higher values indicate higher probabilities.
+
+Like other correlation coefficients, this one varies between -1 and +1 with 0 implying no correlation. Correlations of -1 or +1 imply an exact linear relationship. Positive correlations imply that as x increases, so does y. Negative correlations imply that as x increases, y decreases.
+
+Output Example(s):
+```python
+{'pearsonr': -0.7}
+```
+```python
+{'p-value': 0.15}
+```
+
+#### Values from Popular Papers
+
+### Examples
+
+Example 1-A simple example using only predictions and references.
+```python
+>>> pearsonr_metric = datasets.load_metric("pearsonr")
+>>> results = pearsonr_metric.compute(predictions=[10, 9, 2.5, 6, 4], references=[1, 2, 3, 4, 5])
+>>> print(round(results['pearsonr'], 2))
+-0.74
+```
+
+Example 2-The same as Example 1, but that also returns the `p-value`.
+```python
+>>> pearsonr_metric = datasets.load_metric("pearsonr")
+>>> results = pearsonr_metric.compute(predictions=[10, 9, 2.5, 6, 4], references=[1, 2, 3, 4, 5], return_pvalue=True)
+>>> print(sorted(list(results.keys())))
+['p-value', 'pearsonr']
+>>> print(round(results['pearsonr'], 2))
+-0.74
+>>> print(round(results['p-value'], 2))
+0.15
+```
+
+
+## Limitations and Bias
+
+As stated above, the calculation of the p-value relies on the assumption that each data set is normally distributed. This is not always the case, so verifying the true distribution of datasets is recommended.
+
+
+## Citation(s)
+```bibtex
+@article{2020SciPy-NMeth,
+author = {Virtanen, Pauli and Gommers, Ralf and Oliphant, Travis E. and
+ Haberland, Matt and Reddy, Tyler and Cournapeau, David and
+ Burovski, Evgeni and Peterson, Pearu and Weckesser, Warren and
+ Bright, Jonathan and {van der Walt}, St{\'e}fan J. and
+ Brett, Matthew and Wilson, Joshua and Millman, K. Jarrod and
+ Mayorov, Nikolay and Nelson, Andrew R. J. and Jones, Eric and
+ Kern, Robert and Larson, Eric and Carey, C J and
+ Polat, {\.I}lhan and Feng, Yu and Moore, Eric W. and
+ {VanderPlas}, Jake and Laxalde, Denis and Perktold, Josef and
+ Cimrman, Robert and Henriksen, Ian and Quintero, E. A. and
+ Harris, Charles R. and Archibald, Anne M. and
+ Ribeiro, Ant{\^o}nio H. and Pedregosa, Fabian and
+ {van Mulbregt}, Paul and {SciPy 1.0 Contributors}},
+title = {{{SciPy} 1.0: Fundamental Algorithms for Scientific
+ Computing in Python}},
+journal = {Nature Methods},
+year = {2020},
+volume = {17},
+pages = {261--272},
+adsurl = {https://rdcu.be/b08Wh},
+doi = {10.1038/s41592-019-0686-2},
+}
+```
+
+
+## Further References
diff --git a/metrics/pearsonr/pearsonr.py b/metrics/pearsonr/pearsonr.py
new file mode 100644
index 0000000..34c3169
--- /dev/null
+++ b/metrics/pearsonr/pearsonr.py
@@ -0,0 +1,106 @@
+# Copyright 2021 The HuggingFace Datasets Authors and the current dataset script contributor.
+#
+# Licensed under the Apache License, Version 2.0 (the "License");
+# you may not use this file except in compliance with the License.
+# You may obtain a copy of the License at
+#
+# http://www.apache.org/licenses/LICENSE-2.0
+#
+# Unless required by applicable law or agreed to in writing, software
+# distributed under the License is distributed on an "AS IS" BASIS,
+# WITHOUT WARRANTIES OR CONDITIONS OF ANY KIND, either express or implied.
+# See the License for the specific language governing permissions and
+# limitations under the License.
+"""Pearson correlation coefficient metric."""
+
+from scipy.stats import pearsonr
+
+import datasets
+
+
+_DESCRIPTION = """
+Pearson correlation coefficient and p-value for testing non-correlation.
+The Pearson correlation coefficient measures the linear relationship between two datasets. The calculation of the p-value relies on the assumption that each dataset is normally distributed. Like other correlation coefficients, this one varies between -1 and +1 with 0 implying no correlation. Correlations of -1 or +1 imply an exact linear relationship. Positive correlations imply that as x increases, so does y. Negative correlations imply that as x increases, y decreases.
+The p-value roughly indicates the probability of an uncorrelated system producing datasets that have a Pearson correlation at least as extreme as the one computed from these datasets.
+"""
+
+
+_KWARGS_DESCRIPTION = """
+Args:
+ predictions (`list` of `int`): Predicted class labels, as returned by a model.
+ references (`list` of `int`): Ground truth labels.
+ return_pvalue (`boolean`): If `True`, returns the p-value, along with the correlation coefficient. If `False`, returns only the correlation coefficient. Defaults to `False`.
+
+Returns:
+ pearsonr (`float`): Pearson correlation coefficient. Minimum possible value is -1. Maximum possible value is 1. Values of 1 and -1 indicate exact linear positive and negative relationships, respectively. A value of 0 implies no correlation.
+ p-value (`float`): P-value, which roughly indicates the probability of an The p-value roughly indicates the probability of an uncorrelated system producing datasets that have a Pearson correlation at least as extreme as the one computed from these datasets. Minimum possible value is 0. Maximum possible value is 1. Higher values indicate higher probabilities.
+
+Examples:
+
+ Example 1-A simple example using only predictions and references.
+ >>> pearsonr_metric = datasets.load_metric("pearsonr")
+ >>> results = pearsonr_metric.compute(predictions=[10, 9, 2.5, 6, 4], references=[1, 2, 3, 4, 5])
+ >>> print(round(results['pearsonr'], 2))
+ -0.74
+
+ Example 2-The same as Example 1, but that also returns the `p-value`.
+ >>> pearsonr_metric = datasets.load_metric("pearsonr")
+ >>> results = pearsonr_metric.compute(predictions=[10, 9, 2.5, 6, 4], references=[1, 2, 3, 4, 5], return_pvalue=True)
+ >>> print(sorted(list(results.keys())))
+ ['p-value', 'pearsonr']
+ >>> print(round(results['pearsonr'], 2))
+ -0.74
+ >>> print(round(results['p-value'], 2))
+ 0.15
+"""
+
+
+_CITATION = """
+@article{2020SciPy-NMeth,
+author = {Virtanen, Pauli and Gommers, Ralf and Oliphant, Travis E. and
+ Haberland, Matt and Reddy, Tyler and Cournapeau, David and
+ Burovski, Evgeni and Peterson, Pearu and Weckesser, Warren and
+ Bright, Jonathan and {van der Walt}, St{\'e}fan J. and
+ Brett, Matthew and Wilson, Joshua and Millman, K. Jarrod and
+ Mayorov, Nikolay and Nelson, Andrew R. J. and Jones, Eric and
+ Kern, Robert and Larson, Eric and Carey, C J and
+ Polat, Ilhan and Feng, Yu and Moore, Eric W. and
+ {VanderPlas}, Jake and Laxalde, Denis and Perktold, Josef and
+ Cimrman, Robert and Henriksen, Ian and Quintero, E. A. and
+ Harris, Charles R. and Archibald, Anne M. and
+ Ribeiro, Antonio H. and Pedregosa, Fabian and
+ {van Mulbregt}, Paul and {SciPy 1.0 Contributors}},
+title = {{{SciPy} 1.0: Fundamental Algorithms for Scientific
+ Computing in Python}},
+journal = {Nature Methods},
+year = {2020},
+volume = {17},
+pages = {261--272},
+adsurl = {https://rdcu.be/b08Wh},
+doi = {10.1038/s41592-019-0686-2},
+}
+"""
+
+
+@datasets.utils.file_utils.add_start_docstrings(_DESCRIPTION, _KWARGS_DESCRIPTION)
+class Pearsonr(datasets.Metric):
+ def _info(self):
+ return datasets.MetricInfo(
+ description=_DESCRIPTION,
+ citation=_CITATION,
+ inputs_description=_KWARGS_DESCRIPTION,
+ features=datasets.Features(
+ {
+ "predictions": datasets.Value("float"),
+ "references": datasets.Value("float"),
+ }
+ ),
+ reference_urls=["https://docs.scipy.org/doc/scipy/reference/generated/scipy.stats.pearsonr.html"],
+ )
+
+ def _compute(self, predictions, references, return_pvalue=False):
+ if return_pvalue:
+ results = pearsonr(references, predictions)
+ return {"pearsonr": results[0], "p-value": results[1]}
+ else:
+ return {"pearsonr": float(pearsonr(references, predictions)[0])}
diff --git a/metrics/perplexity/README.md b/metrics/perplexity/README.md
new file mode 100644
index 0000000..efa5216
--- /dev/null
+++ b/metrics/perplexity/README.md
@@ -0,0 +1,96 @@
+# Metric Card for Perplexity
+
+## Metric Description
+Given a model and an input text sequence, perplexity measures how likely the model is to generate the input text sequence. This can be used in two main ways:
+1. to evaluate how well the model has learned the distribution of the text it was trained on
+ - In this case, the model input should be the trained model to be evaluated, and the input texts should be the text that the model was trained on.
+2. to evaluate how well a selection of text matches the distribution of text that the input model was trained on
+ - In this case, the model input should be a trained model, and the input texts should be the text to be evaluated.
+
+## Intended Uses
+Any language generation task.
+
+## How to Use
+
+The metric takes a list of text as input, as well as the name of the model used to compute the metric:
+
+```python
+from datasets import load_metric
+perplexity = load_metric("perplexity")
+results = perplexity.compute(input_texts=input_texts, model_id='gpt2')
+```
+
+### Inputs
+- **model_id** (str): model used for calculating Perplexity. NOTE: Perplexity can only be calculated for causal language models.
+ - This includes models such as gpt2, causal variations of bert, causal versions of t5, and more (the full list can be found in the AutoModelForCausalLM documentation here: https://huggingface.co/docs/transformers/master/en/model_doc/auto#transformers.AutoModelForCausalLM )
+- **input_texts** (list of str): input text, each separate text snippet is one list entry.
+- **batch_size** (int): the batch size to run texts through the model. Defaults to 16.
+- **add_start_token** (bool): whether to add the start token to the texts, so the perplexity can include the probability of the first word. Defaults to True.
+- **device** (str): device to run on, defaults to 'cuda' when available
+
+### Output Values
+This metric outputs a dictionary with the perplexity scores for the text input in the list, and the average perplexity.
+If one of the input texts is longer than the max input length of the model, then it is truncated to the max length for the perplexity computation.
+
+```
+{'perplexities': [8.182524681091309, 33.42122268676758, 27.012239456176758], 'mean_perplexity': 22.871995608011883}
+```
+
+This metric's range is 0 and up. A lower score is better.
+
+#### Values from Popular Papers
+
+
+### Examples
+Calculating perplexity on input_texts defined here:
+```python
+perplexity = datasets.load_metric("perplexity")
+input_texts = ["lorem ipsum", "Happy Birthday!", "Bienvenue"]
+results = perplexity.compute(model_id='gpt2',
+ add_start_token=False,
+ input_texts=input_texts)
+print(list(results.keys()))
+>>>['perplexities', 'mean_perplexity']
+print(round(results["mean_perplexity"], 2))
+>>>78.22
+print(round(results["perplexities"][0], 2))
+>>>11.11
+```
+Calculating perplexity on input_texts loaded in from a dataset:
+```python
+perplexity = datasets.load_metric("perplexity")
+input_texts = datasets.load_dataset("wikitext",
+ "wikitext-2-raw-v1",
+ split="test")["text"][:50]
+input_texts = [s for s in input_texts if s!='']
+results = perplexity.compute(model_id='gpt2',
+ input_texts=input_texts)
+print(list(results.keys()))
+>>>['perplexities', 'mean_perplexity']
+print(round(results["mean_perplexity"], 2))
+>>>60.35
+print(round(results["perplexities"][0], 2))
+>>>81.12
+```
+
+## Limitations and Bias
+Note that the output value is based heavily on what text the model was trained on. This means that perplexity scores are not comparable between models or datasets.
+
+
+## Citation
+
+```bibtex
+@article{jelinek1977perplexity,
+title={Perplexity—a measure of the difficulty of speech recognition tasks},
+author={Jelinek, Fred and Mercer, Robert L and Bahl, Lalit R and Baker, James K},
+journal={The Journal of the Acoustical Society of America},
+volume={62},
+number={S1},
+pages={S63--S63},
+year={1977},
+publisher={Acoustical Society of America}
+}
+```
+
+## Further References
+- [Hugging Face Perplexity Blog Post](https://huggingface.co/docs/transformers/perplexity)
diff --git a/metrics/perplexity/perplexity.py b/metrics/perplexity/perplexity.py
new file mode 100644
index 0000000..9197b27
--- /dev/null
+++ b/metrics/perplexity/perplexity.py
@@ -0,0 +1,190 @@
+# Copyright 2022 The HuggingFace Datasets Authors and the current dataset script contributor.
+#
+# Licensed under the Apache License, Version 2.0 (the "License");
+# you may not use this file except in compliance with the License.
+# You may obtain a copy of the License at
+#
+# http://www.apache.org/licenses/LICENSE-2.0
+#
+# Unless required by applicable law or agreed to in writing, software
+# distributed under the License is distributed on an "AS IS" BASIS,
+# WITHOUT WARRANTIES OR CONDITIONS OF ANY KIND, either express or implied.
+# See the License for the specific language governing permissions and
+# limitations under the License.
+"""Perplexity Metric."""
+
+import numpy as np
+import torch
+from torch.nn import CrossEntropyLoss
+from transformers import AutoModelForCausalLM, AutoTokenizer
+
+import datasets
+from datasets import logging
+
+
+_CITATION = """\
+
+"""
+
+_DESCRIPTION = """
+Perplexity (PPL) is one of the most common metrics for evaluating language models.
+It is defined as the exponentiated average negative log-likelihood of a sequence.
+
+For more information, see https://huggingface.co/docs/transformers/perplexity
+"""
+
+_KWARGS_DESCRIPTION = """
+Args:
+ model_id (str): model used for calculating Perplexity
+ NOTE: Perplexity can only be calculated for causal language models.
+ This includes models such as gpt2, causal variations of bert,
+ causal versions of t5, and more (the full list can be found
+ in the AutoModelForCausalLM documentation here:
+ https://huggingface.co/docs/transformers/master/en/model_doc/auto#transformers.AutoModelForCausalLM )
+
+ input_texts (list of str): input text, each separate text snippet
+ is one list entry.
+ batch_size (int): the batch size to run texts through the model. Defaults to 16.
+ add_start_token (bool): whether to add the start token to the texts,
+ so the perplexity can include the probability of the first word. Defaults to True.
+ device (str): device to run on, defaults to 'cuda' when available
+Returns:
+ perplexity: dictionary containing the perplexity scores for the texts
+ in the input list, as well as the mean perplexity. If one of the input texts is
+ longer than the max input length of the model, then it is truncated to the
+ max length for the perplexity computation.
+Examples:
+ Example 1:
+ >>> perplexity = datasets.load_metric("perplexity")
+ >>> input_texts = ["lorem ipsum", "Happy Birthday!", "Bienvenue"]
+ >>> results = perplexity.compute(model_id='gpt2',
+ ... add_start_token=False,
+ ... input_texts=input_texts) # doctest:+ELLIPSIS
+ >>> print(list(results.keys()))
+ ['perplexities', 'mean_perplexity']
+ >>> print(round(results["mean_perplexity"], 2))
+ 78.22
+ >>> print(round(results["perplexities"][0], 2))
+ 11.11
+
+ Example 2:
+ >>> perplexity = datasets.load_metric("perplexity")
+ >>> input_texts = datasets.load_dataset("wikitext",
+ ... "wikitext-2-raw-v1",
+ ... split="test")["text"][:50] # doctest:+ELLIPSIS
+ [...]
+ >>> input_texts = [s for s in input_texts if s!='']
+ >>> results = perplexity.compute(model_id='gpt2',
+ ... input_texts=input_texts) # doctest:+ELLIPSIS
+ >>> print(list(results.keys()))
+ ['perplexities', 'mean_perplexity']
+ >>> print(round(results["mean_perplexity"], 2))
+ 60.35
+ >>> print(round(results["perplexities"][0], 2))
+ 81.12
+"""
+
+
+@datasets.utils.file_utils.add_start_docstrings(_DESCRIPTION, _KWARGS_DESCRIPTION)
+class Perplexity(datasets.Metric):
+ def _info(self):
+ return datasets.MetricInfo(
+ description=_DESCRIPTION,
+ citation=_CITATION,
+ inputs_description=_KWARGS_DESCRIPTION,
+ features=datasets.Features(
+ {
+ "input_texts": datasets.Value("string"),
+ }
+ ),
+ reference_urls=["https://huggingface.co/docs/transformers/perplexity"],
+ )
+
+ def _compute(self, input_texts, model_id, batch_size: int = 16, add_start_token: bool = True, device=None):
+
+ if device is not None:
+ assert device in ["gpu", "cpu", "cuda"], "device should be either gpu or cpu."
+ if device == "gpu":
+ device = "cuda"
+ else:
+ device = "cuda" if torch.cuda.is_available() else "cpu"
+
+ model = AutoModelForCausalLM.from_pretrained(model_id)
+ model = model.to(device)
+
+ tokenizer = AutoTokenizer.from_pretrained(model_id)
+
+ # if batch_size > 1 (which generally leads to padding being required), and
+ # if there is not an already assigned pad_token, assign an existing
+ # special token to also be the padding token
+ if tokenizer.pad_token is None and batch_size > 1:
+ existing_special_tokens = list(tokenizer.special_tokens_map_extended.values())
+ # check that the model already has at least one special token defined
+ assert (
+ len(existing_special_tokens) > 0
+ ), "If batch_size > 1, model must have at least one special token to use for padding. Please use a different model or set batch_size=1."
+ # assign one of the special tokens to also be the pad token
+ tokenizer.add_special_tokens({"pad_token": existing_special_tokens[0]})
+
+ if add_start_token:
+ # leave room for token to be added:
+ assert (
+ tokenizer.bos_token is not None
+ ), "Input model must already have a BOS token if using add_start_token=True. Please use a different model, or set add_start_token=False"
+ max_tokenized_len = model.config.max_length - 1
+ else:
+ max_tokenized_len = model.config.max_length
+
+ encodings = tokenizer(
+ input_texts,
+ add_special_tokens=False,
+ padding=True,
+ truncation=True,
+ max_length=max_tokenized_len,
+ return_tensors="pt",
+ return_attention_mask=True,
+ ).to(device)
+
+ encoded_texts = encodings["input_ids"]
+ attn_masks = encodings["attention_mask"]
+
+ # check that each input is long enough:
+ if add_start_token:
+ assert torch.all(torch.ge(attn_masks.sum(1), 1)), "Each input text must be at least one token long."
+ else:
+ assert torch.all(
+ torch.ge(attn_masks.sum(1), 2)
+ ), "When add_start_token=False, each input text must be at least two tokens long. Run with add_start_token=True if inputting strings of only one token, and remove all empty input strings."
+
+ ppls = []
+ loss_fct = CrossEntropyLoss(reduction="none")
+
+ for start_index in logging.tqdm(range(0, len(encoded_texts), batch_size)):
+ end_index = min(start_index + batch_size, len(encoded_texts))
+ encoded_batch = encoded_texts[start_index:end_index]
+ attn_mask = attn_masks[start_index:end_index]
+
+ if add_start_token:
+ bos_tokens_tensor = torch.tensor([[tokenizer.bos_token_id]] * encoded_batch.size(dim=0)).to(device)
+ encoded_batch = torch.cat([bos_tokens_tensor, encoded_batch], dim=1)
+ attn_mask = torch.cat(
+ [torch.ones(bos_tokens_tensor.size(), dtype=torch.int64).to(device), attn_mask], dim=1
+ )
+
+ labels = encoded_batch
+
+ with torch.no_grad():
+ out_logits = model(encoded_batch, attention_mask=attn_mask).logits
+
+ shift_logits = out_logits[..., :-1, :].contiguous()
+ shift_labels = labels[..., 1:].contiguous()
+ shift_attention_mask_batch = attn_mask[..., 1:].contiguous()
+
+ perplexity_batch = torch.exp2(
+ (loss_fct(shift_logits.transpose(1, 2), shift_labels) * shift_attention_mask_batch).sum(1)
+ / shift_attention_mask_batch.sum(1)
+ )
+
+ ppls += perplexity_batch.tolist()
+
+ return {"perplexities": ppls, "mean_perplexity": np.mean(ppls)}
diff --git a/metrics/precision/README.md b/metrics/precision/README.md
new file mode 100644
index 0000000..14b5998
--- /dev/null
+++ b/metrics/precision/README.md
@@ -0,0 +1,124 @@
+# Metric Card for Precision
+
+
+## Metric Description
+
+Precision is the fraction of correctly labeled positive examples out of all of the examples that were labeled as positive. It is computed via the equation:
+Precision = TP / (TP + FP)
+where TP is the True positives (i.e. the examples correctly labeled as positive) and FP is the False positive examples (i.e. the examples incorrectly labeled as positive).
+
+
+## How to Use
+
+At minimum, precision takes as input a list of predicted labels, `predictions`, and a list of output labels, `references`.
+
+```python
+>>> precision_metric = datasets.load_metric("precision")
+>>> results = precision_metric.compute(references=[0, 1], predictions=[0, 1])
+>>> print(results)
+{'precision': 1.0}
+```
+
+
+### Inputs
+- **predictions** (`list` of `int`): Predicted class labels.
+- **references** (`list` of `int`): Actual class labels.
+- **labels** (`list` of `int`): The set of labels to include when `average` is not set to `'binary'`. If `average` is `None`, it should be the label order. Labels present in the data can be excluded, for example to calculate a multiclass average ignoring a majority negative class. Labels not present in the data will result in 0 components in a macro average. For multilabel targets, labels are column indices. By default, all labels in `predictions` and `references` are used in sorted order. Defaults to None.
+- **pos_label** (`int`): The class to be considered the positive class, in the case where `average` is set to `binary`. Defaults to 1.
+- **average** (`string`): This parameter is required for multiclass/multilabel targets. If set to `None`, the scores for each class are returned. Otherwise, this determines the type of averaging performed on the data. Defaults to `'binary'`.
+ - 'binary': Only report results for the class specified by `pos_label`. This is applicable only if the classes found in `predictions` and `references` are binary.
+ - 'micro': Calculate metrics globally by counting the total true positives, false negatives and false positives.
+ - 'macro': Calculate metrics for each label, and find their unweighted mean. This does not take label imbalance into account.
+ - 'weighted': Calculate metrics for each label, and find their average weighted by support (the number of true instances for each label). This alters `'macro'` to account for label imbalance. This option can result in an F-score that is not between precision and recall.
+ - 'samples': Calculate metrics for each instance, and find their average (only meaningful for multilabel classification).
+- **sample_weight** (`list` of `float`): Sample weights Defaults to None.
+- **zero_division** (): Sets the value to return when there is a zero division. Defaults to .
+ - 0: Returns 0 when there is a zero division.
+ - 1: Returns 1 when there is a zero division.
+ - 'warn': Raises warnings and then returns 0 when there is a zero division.
+
+
+### Output Values
+- **precision**(`float` or `array` of `float`): Precision score or list of precision scores, depending on the value passed to `average`. Minimum possible value is 0. Maximum possible value is 1. Higher values indicate that fewer negative examples were incorrectly labeled as positive, which means that, generally, higher scores are better.
+
+Output Example(s):
+```python
+{'precision': 0.2222222222222222}
+```
+```python
+{'precision': array([0.66666667, 0.0, 0.0])}
+```
+
+
+
+
+#### Values from Popular Papers
+
+
+### Examples
+
+Example 1-A simple binary example
+```python
+>>> precision_metric = datasets.load_metric("precision")
+>>> results = precision_metric.compute(references=[0, 1, 0, 1, 0], predictions=[0, 0, 1, 1, 0])
+>>> print(results)
+{'precision': 0.5}
+```
+
+Example 2-The same simple binary example as in Example 1, but with `pos_label` set to `0`.
+```python
+>>> precision_metric = datasets.load_metric("precision")
+>>> results = precision_metric.compute(references=[0, 1, 0, 1, 0], predictions=[0, 0, 1, 1, 0], pos_label=0)
+>>> print(round(results['precision'], 2))
+0.67
+```
+
+Example 3-The same simple binary example as in Example 1, but with `sample_weight` included.
+```python
+>>> precision_metric = datasets.load_metric("precision")
+>>> results = precision_metric.compute(references=[0, 1, 0, 1, 0], predictions=[0, 0, 1, 1, 0], sample_weight=[0.9, 0.5, 3.9, 1.2, 0.3])
+>>> print(results)
+{'precision': 0.23529411764705882}
+```
+
+Example 4-A multiclass example, with different values for the `average` input.
+```python
+>>> predictions = [0, 2, 1, 0, 0, 1]
+>>> references = [0, 1, 2, 0, 1, 2]
+>>> results = precision_metric.compute(predictions=predictions, references=references, average='macro')
+>>> print(results)
+{'precision': 0.2222222222222222}
+>>> results = precision_metric.compute(predictions=predictions, references=references, average='micro')
+>>> print(results)
+{'precision': 0.3333333333333333}
+>>> results = precision_metric.compute(predictions=predictions, references=references, average='weighted')
+>>> print(results)
+{'precision': 0.2222222222222222}
+>>> results = precision_metric.compute(predictions=predictions, references=references, average=None)
+>>> print([round(res, 2) for res in results['precision']])
+[0.67, 0.0, 0.0]
+```
+
+
+## Limitations and Bias
+
+[Precision](https://huggingface.co/metrics/precision) and [recall](https://huggingface.co/metrics/recall) are complementary and can be used to measure different aspects of model performance -- using both of them (or an averaged measure like [F1 score](https://huggingface.co/metrics/F1) to better represent different aspects of performance. See [Wikipedia](https://en.wikipedia.org/wiki/Precision_and_recall) for more information.
+
+## Citation(s)
+```bibtex
+@article{scikit-learn,
+ title={Scikit-learn: Machine Learning in {P}ython},
+ author={Pedregosa, F. and Varoquaux, G. and Gramfort, A. and Michel, V.
+ and Thirion, B. and Grisel, O. and Blondel, M. and Prettenhofer, P.
+ and Weiss, R. and Dubourg, V. and Vanderplas, J. and Passos, A. and
+ Cournapeau, D. and Brucher, M. and Perrot, M. and Duchesnay, E.},
+ journal={Journal of Machine Learning Research},
+ volume={12},
+ pages={2825--2830},
+ year={2011}
+}
+```
+
+
+## Further References
+- [Wikipedia -- Precision and recall](https://en.wikipedia.org/wiki/Precision_and_recall)
diff --git a/metrics/precision/precision.py b/metrics/precision/precision.py
new file mode 100644
index 0000000..95d0b89
--- /dev/null
+++ b/metrics/precision/precision.py
@@ -0,0 +1,144 @@
+# Copyright 2020 The HuggingFace Datasets Authors and the current dataset script contributor.
+#
+# Licensed under the Apache License, Version 2.0 (the "License");
+# you may not use this file except in compliance with the License.
+# You may obtain a copy of the License at
+#
+# http://www.apache.org/licenses/LICENSE-2.0
+#
+# Unless required by applicable law or agreed to in writing, software
+# distributed under the License is distributed on an "AS IS" BASIS,
+# WITHOUT WARRANTIES OR CONDITIONS OF ANY KIND, either express or implied.
+# See the License for the specific language governing permissions and
+# limitations under the License.
+"""Precision metric."""
+
+from sklearn.metrics import precision_score
+
+import datasets
+
+
+_DESCRIPTION = """
+Precision is the fraction of correctly labeled positive examples out of all of the examples that were labeled as positive. It is computed via the equation:
+Precision = TP / (TP + FP)
+where TP is the True positives (i.e. the examples correctly labeled as positive) and FP is the False positive examples (i.e. the examples incorrectly labeled as positive).
+"""
+
+
+_KWARGS_DESCRIPTION = """
+Args:
+ predictions (`list` of `int`): Predicted class labels.
+ references (`list` of `int`): Actual class labels.
+ labels (`list` of `int`): The set of labels to include when `average` is not set to `'binary'`. If `average` is `None`, it should be the label order. Labels present in the data can be excluded, for example to calculate a multiclass average ignoring a majority negative class. Labels not present in the data will result in 0 components in a macro average. For multilabel targets, labels are column indices. By default, all labels in `predictions` and `references` are used in sorted order. Defaults to None.
+ pos_label (`int`): The class to be considered the positive class, in the case where `average` is set to `binary`. Defaults to 1.
+ average (`string`): This parameter is required for multiclass/multilabel targets. If set to `None`, the scores for each class are returned. Otherwise, this determines the type of averaging performed on the data. Defaults to `'binary'`.
+
+ - 'binary': Only report results for the class specified by `pos_label`. This is applicable only if the classes found in `predictions` and `references` are binary.
+ - 'micro': Calculate metrics globally by counting the total true positives, false negatives and false positives.
+ - 'macro': Calculate metrics for each label, and find their unweighted mean. This does not take label imbalance into account.
+ - 'weighted': Calculate metrics for each label, and find their average weighted by support (the number of true instances for each label). This alters `'macro'` to account for label imbalance. This option can result in an F-score that is not between precision and recall.
+ - 'samples': Calculate metrics for each instance, and find their average (only meaningful for multilabel classification).
+ sample_weight (`list` of `float`): Sample weights Defaults to None.
+ zero_division (`int` or `string`): Sets the value to return when there is a zero division. Defaults to 'warn'.
+
+ - 0: Returns 0 when there is a zero division.
+ - 1: Returns 1 when there is a zero division.
+ - 'warn': Raises warnings and then returns 0 when there is a zero division.
+
+Returns:
+ precision (`float` or `array` of `float`): Precision score or list of precision scores, depending on the value passed to `average`. Minimum possible value is 0. Maximum possible value is 1. Higher values indicate that fewer negative examples were incorrectly labeled as positive, which means that, generally, higher scores are better.
+
+Examples:
+
+ Example 1-A simple binary example
+ >>> precision_metric = datasets.load_metric("precision")
+ >>> results = precision_metric.compute(references=[0, 1, 0, 1, 0], predictions=[0, 0, 1, 1, 0])
+ >>> print(results)
+ {'precision': 0.5}
+
+ Example 2-The same simple binary example as in Example 1, but with `pos_label` set to `0`.
+ >>> precision_metric = datasets.load_metric("precision")
+ >>> results = precision_metric.compute(references=[0, 1, 0, 1, 0], predictions=[0, 0, 1, 1, 0], pos_label=0)
+ >>> print(round(results['precision'], 2))
+ 0.67
+
+ Example 3-The same simple binary example as in Example 1, but with `sample_weight` included.
+ >>> precision_metric = datasets.load_metric("precision")
+ >>> results = precision_metric.compute(references=[0, 1, 0, 1, 0], predictions=[0, 0, 1, 1, 0], sample_weight=[0.9, 0.5, 3.9, 1.2, 0.3])
+ >>> print(results)
+ {'precision': 0.23529411764705882}
+
+ Example 4-A multiclass example, with different values for the `average` input.
+ >>> predictions = [0, 2, 1, 0, 0, 1]
+ >>> references = [0, 1, 2, 0, 1, 2]
+ >>> results = precision_metric.compute(predictions=predictions, references=references, average='macro')
+ >>> print(results)
+ {'precision': 0.2222222222222222}
+ >>> results = precision_metric.compute(predictions=predictions, references=references, average='micro')
+ >>> print(results)
+ {'precision': 0.3333333333333333}
+ >>> results = precision_metric.compute(predictions=predictions, references=references, average='weighted')
+ >>> print(results)
+ {'precision': 0.2222222222222222}
+ >>> results = precision_metric.compute(predictions=predictions, references=references, average=None)
+ >>> print([round(res, 2) for res in results['precision']])
+ [0.67, 0.0, 0.0]
+"""
+
+
+_CITATION = """
+@article{scikit-learn,
+ title={Scikit-learn: Machine Learning in {P}ython},
+ author={Pedregosa, F. and Varoquaux, G. and Gramfort, A. and Michel, V.
+ and Thirion, B. and Grisel, O. and Blondel, M. and Prettenhofer, P.
+ and Weiss, R. and Dubourg, V. and Vanderplas, J. and Passos, A. and
+ Cournapeau, D. and Brucher, M. and Perrot, M. and Duchesnay, E.},
+ journal={Journal of Machine Learning Research},
+ volume={12},
+ pages={2825--2830},
+ year={2011}
+}
+"""
+
+
+@datasets.utils.file_utils.add_start_docstrings(_DESCRIPTION, _KWARGS_DESCRIPTION)
+class Precision(datasets.Metric):
+ def _info(self):
+ return datasets.MetricInfo(
+ description=_DESCRIPTION,
+ citation=_CITATION,
+ inputs_description=_KWARGS_DESCRIPTION,
+ features=datasets.Features(
+ {
+ "predictions": datasets.Sequence(datasets.Value("int32")),
+ "references": datasets.Sequence(datasets.Value("int32")),
+ }
+ if self.config_name == "multilabel"
+ else {
+ "predictions": datasets.Value("int32"),
+ "references": datasets.Value("int32"),
+ }
+ ),
+ reference_urls=["https://scikit-learn.org/stable/modules/generated/sklearn.metrics.precision_score.html"],
+ )
+
+ def _compute(
+ self,
+ predictions,
+ references,
+ labels=None,
+ pos_label=1,
+ average="binary",
+ sample_weight=None,
+ zero_division="warn",
+ ):
+ score = precision_score(
+ references,
+ predictions,
+ labels=labels,
+ pos_label=pos_label,
+ average=average,
+ sample_weight=sample_weight,
+ zero_division=zero_division,
+ )
+ return {"precision": float(score) if score.size == 1 else score}
diff --git a/metrics/recall/README.md b/metrics/recall/README.md
new file mode 100644
index 0000000..cae1459
--- /dev/null
+++ b/metrics/recall/README.md
@@ -0,0 +1,114 @@
+# Metric Card for Recall
+
+
+## Metric Description
+
+Recall is the fraction of the positive examples that were correctly labeled by the model as positive. It can be computed with the equation:
+Recall = TP / (TP + FN)
+Where TP is the number of true positives and FN is the number of false negatives.
+
+
+## How to Use
+
+At minimum, this metric takes as input two `list`s, each containing `int`s: predictions and references.
+
+```python
+>>> recall_metric = datasets.load_metric('recall')
+>>> results = recall_metric.compute(references=[0, 1], predictions=[0, 1])
+>>> print(results)
+["{'recall': 1.0}"]
+```
+
+
+### Inputs
+- **predictions** (`list` of `int`): The predicted labels.
+- **references** (`list` of `int`): The ground truth labels.
+- **labels** (`list` of `int`): The set of labels to include when `average` is not set to `binary`, and their order when average is `None`. Labels present in the data can be excluded in this input, for example to calculate a multiclass average ignoring a majority negative class, while labels not present in the data will result in 0 components in a macro average. For multilabel targets, labels are column indices. By default, all labels in y_true and y_pred are used in sorted order. Defaults to None.
+- **pos_label** (`int`): The class label to use as the 'positive class' when calculating the recall. Defaults to `1`.
+- **average** (`string`): This parameter is required for multiclass/multilabel targets. If None, the scores for each class are returned. Otherwise, this determines the type of averaging performed on the data. Defaults to `'binary'`.
+ - `'binary'`: Only report results for the class specified by `pos_label`. This is applicable only if the target labels and predictions are binary.
+ - `'micro'`: Calculate metrics globally by counting the total true positives, false negatives, and false positives.
+ - `'macro'`: Calculate metrics for each label, and find their unweighted mean. This does not take label imbalance into account.
+ - `'weighted'`: Calculate metrics for each label, and find their average weighted by support (the number of true instances for each label). This alters `'macro'` to account for label imbalance. Note that it can result in an F-score that is not between precision and recall.
+ - `'samples'`: Calculate metrics for each instance, and find their average (only meaningful for multilabel classification).
+- **sample_weight** (`list` of `float`): Sample weights Defaults to `None`.
+- **zero_division** (): Sets the value to return when there is a zero division. Defaults to .
+ - `'warn'`: If there is a zero division, the return value is `0`, but warnings are also raised.
+ - `0`: If there is a zero division, the return value is `0`.
+ - `1`: If there is a zero division, the return value is `1`.
+
+
+### Output Values
+- **recall**(`float`, or `array` of `float`, for multiclass targets): Either the general recall score, or the recall scores for individual classes, depending on the values input to `labels` and `average`. Minimum possible value is 0. Maximum possible value is 1. A higher recall means that more of the positive examples have been labeled correctly. Therefore, a higher recall is generally considered better.
+
+Output Example(s):
+```python
+{'recall': 1.0}
+```
+```python
+{'recall': array([1., 0., 0.])}
+```
+
+This metric outputs a dictionary with one entry, `'recall'`.
+
+
+#### Values from Popular Papers
+
+
+### Examples
+
+Example 1-A simple example with some errors
+```python
+>>> recall_metric = datasets.load_metric('recall')
+>>> results = recall_metric.compute(references=[0, 0, 1, 1, 1], predictions=[0, 1, 0, 1, 1])
+>>> print(results)
+{'recall': 0.6666666666666666}
+```
+
+Example 2-The same example as Example 1, but with `pos_label=0` instead of the default `pos_label=1`.
+```python
+>>> recall_metric = datasets.load_metric('recall')
+>>> results = recall_metric.compute(references=[0, 0, 1, 1, 1], predictions=[0, 1, 0, 1, 1], pos_label=0)
+>>> print(results)
+{'recall': 0.5}
+```
+
+Example 3-The same example as Example 1, but with `sample_weight` included.
+```python
+>>> recall_metric = datasets.load_metric('recall')
+>>> sample_weight = [0.9, 0.2, 0.9, 0.3, 0.8]
+>>> results = recall_metric.compute(references=[0, 0, 1, 1, 1], predictions=[0, 1, 0, 1, 1], sample_weight=sample_weight)
+>>> print(results)
+{'recall': 0.55}
+```
+
+Example 4-A multiclass example, using different averages.
+```python
+>>> recall_metric = datasets.load_metric('recall')
+>>> predictions = [0, 2, 1, 0, 0, 1]
+>>> references = [0, 1, 2, 0, 1, 2]
+>>> results = recall_metric.compute(predictions=predictions, references=references, average='macro')
+>>> print(results)
+{'recall': 0.3333333333333333}
+>>> results = recall_metric.compute(predictions=predictions, references=references, average='micro')
+>>> print(results)
+{'recall': 0.3333333333333333}
+>>> results = recall_metric.compute(predictions=predictions, references=references, average='weighted')
+>>> print(results)
+{'recall': 0.3333333333333333}
+>>> results = recall_metric.compute(predictions=predictions, references=references, average=None)
+>>> print(results)
+{'recall': array([1., 0., 0.])}
+```
+
+
+## Limitations and Bias
+
+
+## Citation(s)
+```bibtex
+@article{scikit-learn, title={Scikit-learn: Machine Learning in {P}ython}, author={Pedregosa, F. and Varoquaux, G. and Gramfort, A. and Michel, V. and Thirion, B. and Grisel, O. and Blondel, M. and Prettenhofer, P. and Weiss, R. and Dubourg, V. and Vanderplas, J. and Passos, A. and Cournapeau, D. and Brucher, M. and Perrot, M. and Duchesnay, E.}, journal={Journal of Machine Learning Research}, volume={12}, pages={2825--2830}, year={2011}
+```
+
+
+## Further References
diff --git a/metrics/recall/recall.py b/metrics/recall/recall.py
new file mode 100644
index 0000000..3ba1149
--- /dev/null
+++ b/metrics/recall/recall.py
@@ -0,0 +1,134 @@
+# Copyright 2020 The HuggingFace Datasets Authors and the current dataset script contributor.
+#
+# Licensed under the Apache License, Version 2.0 (the "License");
+# you may not use this file except in compliance with the License.
+# You may obtain a copy of the License at
+#
+# http://www.apache.org/licenses/LICENSE-2.0
+#
+# Unless required by applicable law or agreed to in writing, software
+# distributed under the License is distributed on an "AS IS" BASIS,
+# WITHOUT WARRANTIES OR CONDITIONS OF ANY KIND, either express or implied.
+# See the License for the specific language governing permissions and
+# limitations under the License.
+"""Recall metric."""
+
+from sklearn.metrics import recall_score
+
+import datasets
+
+
+_DESCRIPTION = """
+Recall is the fraction of the positive examples that were correctly labeled by the model as positive. It can be computed with the equation:
+Recall = TP / (TP + FN)
+Where TP is the true positives and FN is the false negatives.
+"""
+
+
+_KWARGS_DESCRIPTION = """
+Args:
+- **predictions** (`list` of `int`): The predicted labels.
+- **references** (`list` of `int`): The ground truth labels.
+- **labels** (`list` of `int`): The set of labels to include when `average` is not set to `binary`, and their order when average is `None`. Labels present in the data can be excluded in this input, for example to calculate a multiclass average ignoring a majority negative class, while labels not present in the data will result in 0 components in a macro average. For multilabel targets, labels are column indices. By default, all labels in y_true and y_pred are used in sorted order. Defaults to None.
+- **pos_label** (`int`): The class label to use as the 'positive class' when calculating the recall. Defaults to `1`.
+- **average** (`string`): This parameter is required for multiclass/multilabel targets. If None, the scores for each class are returned. Otherwise, this determines the type of averaging performed on the data. Defaults to `'binary'`.
+ - `'binary'`: Only report results for the class specified by `pos_label`. This is applicable only if the target labels and predictions are binary.
+ - `'micro'`: Calculate metrics globally by counting the total true positives, false negatives, and false positives.
+ - `'macro'`: Calculate metrics for each label, and find their unweighted mean. This does not take label imbalance into account.
+ - `'weighted'`: Calculate metrics for each label, and find their average weighted by support (the number of true instances for each label). This alters `'macro'` to account for label imbalance. Note that it can result in an F-score that is not between precision and recall.
+ - `'samples'`: Calculate metrics for each instance, and find their average (only meaningful for multilabel classification).
+- **sample_weight** (`list` of `float`): Sample weights Defaults to `None`.
+- **zero_division** (): Sets the value to return when there is a zero division. Defaults to .
+ - `'warn'`: If there is a zero division, the return value is `0`, but warnings are also raised.
+ - `0`: If there is a zero division, the return value is `0`.
+ - `1`: If there is a zero division, the return value is `1`.
+
+Returns:
+- **recall** (`float`, or `array` of `float`): Either the general recall score, or the recall scores for individual classes, depending on the values input to `labels` and `average`. Minimum possible value is 0. Maximum possible value is 1. A higher recall means that more of the positive examples have been labeled correctly. Therefore, a higher recall is generally considered better.
+
+Examples:
+
+ Example 1-A simple example with some errors
+ >>> recall_metric = datasets.load_metric('recall')
+ >>> results = recall_metric.compute(references=[0, 0, 1, 1, 1], predictions=[0, 1, 0, 1, 1])
+ >>> print(results)
+ {'recall': 0.6666666666666666}
+
+ Example 2-The same example as Example 1, but with `pos_label=0` instead of the default `pos_label=1`.
+ >>> recall_metric = datasets.load_metric('recall')
+ >>> results = recall_metric.compute(references=[0, 0, 1, 1, 1], predictions=[0, 1, 0, 1, 1], pos_label=0)
+ >>> print(results)
+ {'recall': 0.5}
+
+ Example 3-The same example as Example 1, but with `sample_weight` included.
+ >>> recall_metric = datasets.load_metric('recall')
+ >>> sample_weight = [0.9, 0.2, 0.9, 0.3, 0.8]
+ >>> results = recall_metric.compute(references=[0, 0, 1, 1, 1], predictions=[0, 1, 0, 1, 1], sample_weight=sample_weight)
+ >>> print(results)
+ {'recall': 0.55}
+
+ Example 4-A multiclass example, using different averages.
+ >>> recall_metric = datasets.load_metric('recall')
+ >>> predictions = [0, 2, 1, 0, 0, 1]
+ >>> references = [0, 1, 2, 0, 1, 2]
+ >>> results = recall_metric.compute(predictions=predictions, references=references, average='macro')
+ >>> print(results)
+ {'recall': 0.3333333333333333}
+ >>> results = recall_metric.compute(predictions=predictions, references=references, average='micro')
+ >>> print(results)
+ {'recall': 0.3333333333333333}
+ >>> results = recall_metric.compute(predictions=predictions, references=references, average='weighted')
+ >>> print(results)
+ {'recall': 0.3333333333333333}
+ >>> results = recall_metric.compute(predictions=predictions, references=references, average=None)
+ >>> print(results)
+ {'recall': array([1., 0., 0.])}
+"""
+
+
+_CITATION = """
+@article{scikit-learn, title={Scikit-learn: Machine Learning in {P}ython}, author={Pedregosa, F. and Varoquaux, G. and Gramfort, A. and Michel, V. and Thirion, B. and Grisel, O. and Blondel, M. and Prettenhofer, P. and Weiss, R. and Dubourg, V. and Vanderplas, J. and Passos, A. and Cournapeau, D. and Brucher, M. and Perrot, M. and Duchesnay, E.}, journal={Journal of Machine Learning Research}, volume={12}, pages={2825--2830}, year={2011}
+"""
+
+
+@datasets.utils.file_utils.add_start_docstrings(_DESCRIPTION, _KWARGS_DESCRIPTION)
+class Recall(datasets.Metric):
+ def _info(self):
+ return datasets.MetricInfo(
+ description=_DESCRIPTION,
+ citation=_CITATION,
+ inputs_description=_KWARGS_DESCRIPTION,
+ features=datasets.Features(
+ {
+ "predictions": datasets.Sequence(datasets.Value("int32")),
+ "references": datasets.Sequence(datasets.Value("int32")),
+ }
+ if self.config_name == "multilabel"
+ else {
+ "predictions": datasets.Value("int32"),
+ "references": datasets.Value("int32"),
+ }
+ ),
+ reference_urls=["https://scikit-learn.org/stable/modules/generated/sklearn.metrics.recall_score.html"],
+ )
+
+ def _compute(
+ self,
+ predictions,
+ references,
+ labels=None,
+ pos_label=1,
+ average="binary",
+ sample_weight=None,
+ zero_division="warn",
+ ):
+ score = recall_score(
+ references,
+ predictions,
+ labels=labels,
+ pos_label=pos_label,
+ average=average,
+ sample_weight=sample_weight,
+ zero_division=zero_division,
+ )
+ return {"recall": float(score) if score.size == 1 else score}
diff --git a/metrics/roc_auc/README.md b/metrics/roc_auc/README.md
new file mode 100644
index 0000000..0b1e65e
--- /dev/null
+++ b/metrics/roc_auc/README.md
@@ -0,0 +1,183 @@
+# Metric Card for ROC AUC
+
+
+## Metric Description
+This metric computes the area under the curve (AUC) for the Receiver Operating Characteristic Curve (ROC). The return values represent how well the model used is predicting the correct classes, based on the input data. A score of `0.5` means that the model is predicting exactly at chance, i.e. the model's predictions are correct at the same rate as if the predictions were being decided by the flip of a fair coin or the roll of a fair die. A score above `0.5` indicates that the model is doing better than chance, while a score below `0.5` indicates that the model is doing worse than chance.
+
+This metric has three separate use cases:
+- **binary**: The case in which there are only two different label classes, and each example gets only one label. This is the default implementation.
+- **multiclass**: The case in which there can be more than two different label classes, but each example still gets only one label.
+- **multilabel**: The case in which there can be more than two different label classes, and each example can have more than one label.
+
+
+## How to Use
+At minimum, this metric requires references and prediction scores:
+```python
+>>> roc_auc_score = datasets.load_metric("roc_auc")
+>>> refs = [1, 0, 1, 1, 0, 0]
+>>> pred_scores = [0.5, 0.2, 0.99, 0.3, 0.1, 0.7]
+>>> results = roc_auc_score.compute(references=refs, prediction_scores=pred_scores)
+>>> print(round(results['roc_auc'], 2))
+0.78
+```
+
+The default implementation of this metric is the **binary** implementation. If employing the **multiclass** or **multilabel** use cases, the keyword `"multiclass"` or `"multilabel"` must be specified when loading the metric:
+- In the **multiclass** case, the metric is loaded with:
+ ```python
+ >>> roc_auc_score = datasets.load_metric("roc_auc", "multiclass")
+ ```
+- In the **multilabel** case, the metric is loaded with:
+ ```python
+ >>> roc_auc_score = datasets.load_metric("roc_auc", "multilabel")
+ ```
+
+See the [Examples Section Below](#examples_section) for more extensive examples.
+
+
+### Inputs
+- **`references`** (array-like of shape (n_samples,) or (n_samples, n_classes)): Ground truth labels. Expects different inputs based on use case:
+ - binary: expects an array-like of shape (n_samples,)
+ - multiclass: expects an array-like of shape (n_samples,)
+ - multilabel: expects an array-like of shape (n_samples, n_classes)
+- **`prediction_scores`** (array-like of shape (n_samples,) or (n_samples, n_classes)): Model predictions. Expects different inputs based on use case:
+ - binary: expects an array-like of shape (n_samples,)
+ - multiclass: expects an array-like of shape (n_samples, n_classes). The probability estimates must sum to 1 across the possible classes.
+ - multilabel: expects an array-like of shape (n_samples, n_classes)
+- **`average`** (`str`): Type of average, and is ignored in the binary use case. Defaults to `'macro'`. Options are:
+ - `'micro'`: Calculates metrics globally by considering each element of the label indicator matrix as a label. Only works with the multilabel use case.
+ - `'macro'`: Calculate metrics for each label, and find their unweighted mean. This does not take label imbalance into account.
+ - `'weighted'`: Calculate metrics for each label, and find their average, weighted by support (i.e. the number of true instances for each label).
+ - `'samples'`: Calculate metrics for each instance, and find their average. Only works with the multilabel use case.
+ - `None`: No average is calculated, and scores for each class are returned. Only works with the multilabels use case.
+- **`sample_weight`** (array-like of shape (n_samples,)): Sample weights. Defaults to None.
+- **`max_fpr`** (`float`): If not None, the standardized partial AUC over the range [0, `max_fpr`] is returned. Must be greater than `0` and less than or equal to `1`. Defaults to `None`. Note: For the multiclass use case, `max_fpr` should be either `None` or `1.0` as ROC AUC partial computation is not currently supported for `multiclass`.
+- **`multi_class`** (`str`): Only used for multiclass targets, in which case it is required. Determines the type of configuration to use. Options are:
+ - `'ovr'`: Stands for One-vs-rest. Computes the AUC of each class against the rest. This treats the multiclass case in the same way as the multilabel case. Sensitive to class imbalance even when `average == 'macro'`, because class imbalance affects the composition of each of the 'rest' groupings.
+ - `'ovo'`: Stands for One-vs-one. Computes the average AUC of all possible pairwise combinations of classes. Insensitive to class imbalance when `average == 'macro'`.
+- **`labels`** (array-like of shape (n_classes,)): Only used for multiclass targets. List of labels that index the classes in `prediction_scores`. If `None`, the numerical or lexicographical order of the labels in `prediction_scores` is used. Defaults to `None`.
+
+### Output Values
+This metric returns a dict containing the `roc_auc` score. The score is a `float`, unless it is the multilabel case with `average=None`, in which case the score is a numpy `array` with entries of type `float`.
+
+The output therefore generally takes the following format:
+```python
+{'roc_auc': 0.778}
+```
+
+In contrast, though, the output takes the following format in the multilabel case when `average=None`:
+```python
+{'roc_auc': array([0.83333333, 0.375, 0.94444444])}
+```
+
+ROC AUC scores can take on any value between `0` and `1`, inclusive.
+
+#### Values from Popular Papers
+
+
+### Examples
+Example 1, the **binary** use case:
+```python
+>>> roc_auc_score = datasets.load_metric("roc_auc")
+>>> refs = [1, 0, 1, 1, 0, 0]
+>>> pred_scores = [0.5, 0.2, 0.99, 0.3, 0.1, 0.7]
+>>> results = roc_auc_score.compute(references=refs, prediction_scores=pred_scores)
+>>> print(round(results['roc_auc'], 2))
+0.78
+```
+
+Example 2, the **multiclass** use case:
+```python
+>>> roc_auc_score = datasets.load_metric("roc_auc", "multiclass")
+>>> refs = [1, 0, 1, 2, 2, 0]
+>>> pred_scores = [[0.3, 0.5, 0.2],
+... [0.7, 0.2, 0.1],
+... [0.005, 0.99, 0.005],
+... [0.2, 0.3, 0.5],
+... [0.1, 0.1, 0.8],
+... [0.1, 0.7, 0.2]]
+>>> results = roc_auc_score.compute(references=refs,
+... prediction_scores=pred_scores,
+... multi_class='ovr')
+>>> print(round(results['roc_auc'], 2))
+0.85
+```
+
+Example 3, the **multilabel** use case:
+```python
+>>> roc_auc_score = datasets.load_metric("roc_auc", "multilabel")
+>>> refs = [[1, 1, 0],
+... [1, 1, 0],
+... [0, 1, 0],
+... [0, 0, 1],
+... [0, 1, 1],
+... [1, 0, 1]]
+>>> pred_scores = [[0.3, 0.5, 0.2],
+... [0.7, 0.2, 0.1],
+... [0.005, 0.99, 0.005],
+... [0.2, 0.3, 0.5],
+... [0.1, 0.1, 0.8],
+... [0.1, 0.7, 0.2]]
+>>> results = roc_auc_score.compute(references=refs,
+... prediction_scores=pred_scores,
+... average=None)
+>>> print([round(res, 2) for res in results['roc_auc'])
+[0.83, 0.38, 0.94]
+```
+
+
+## Limitations and Bias
+
+
+## Citation
+```bibtex
+@article{doi:10.1177/0272989X8900900307,
+author = {Donna Katzman McClish},
+title ={Analyzing a Portion of the ROC Curve},
+journal = {Medical Decision Making},
+volume = {9},
+number = {3},
+pages = {190-195},
+year = {1989},
+doi = {10.1177/0272989X8900900307},
+ note ={PMID: 2668680},
+URL = {https://doi.org/10.1177/0272989X8900900307},
+eprint = {https://doi.org/10.1177/0272989X8900900307}
+}
+```
+
+```bibtex
+@article{10.1023/A:1010920819831,
+author = {Hand, David J. and Till, Robert J.},
+title = {A Simple Generalisation of the Area Under the ROC Curve for Multiple Class Classification Problems},
+year = {2001},
+issue_date = {November 2001},
+publisher = {Kluwer Academic Publishers},
+address = {USA},
+volume = {45},
+number = {2},
+issn = {0885-6125},
+url = {https://doi.org/10.1023/A:1010920819831},
+doi = {10.1023/A:1010920819831},
+journal = {Mach. Learn.},
+month = {oct},
+pages = {171–186},
+numpages = {16},
+keywords = {Gini index, AUC, error rate, ROC curve, receiver operating characteristic}
+}
+```
+
+```bibtex
+@article{scikit-learn,
+title={Scikit-learn: Machine Learning in {P}ython},
+author={Pedregosa, F. and Varoquaux, G. and Gramfort, A. and Michel, V. and Thirion, B. and Grisel, O. and Blondel, M. and Prettenhofer, P. and Weiss, R. and Dubourg, V. and Vanderplas, J. and Passos, A. and Cournapeau, D. and Brucher, M. and Perrot, M. and Duchesnay, E.},
+journal={Journal of Machine Learning Research},
+volume={12},
+pages={2825--2830},
+year={2011}
+}
+```
+
+## Further References
+This implementation is a wrapper around the [Scikit-learn implementation](https://scikit-learn.org/stable/modules/generated/sklearn.metrics.roc_auc_score.html). Much of the documentation here was adapted from their existing documentation, as well.
+
+The [Guide to ROC and AUC](https://youtu.be/iCZJfO-7C5Q) video from the channel Data Science Bits is also very informative.
diff --git a/metrics/roc_auc/roc_auc.py b/metrics/roc_auc/roc_auc.py
new file mode 100644
index 0000000..8acf257
--- /dev/null
+++ b/metrics/roc_auc/roc_auc.py
@@ -0,0 +1,190 @@
+# Copyright 2020 The HuggingFace Datasets Authors and the current dataset script contributor.
+#
+# Licensed under the Apache License, Version 2.0 (the "License");
+# you may not use this file except in compliance with the License.
+# You may obtain a copy of the License at
+#
+# http://www.apache.org/licenses/LICENSE-2.0
+#
+# Unless required by applicable law or agreed to in writing, software
+# distributed under the License is distributed on an "AS IS" BASIS,
+# WITHOUT WARRANTIES OR CONDITIONS OF ANY KIND, either express or implied.
+# See the License for the specific language governing permissions and
+# limitations under the License.
+"""Accuracy metric."""
+
+from sklearn.metrics import roc_auc_score
+
+import datasets
+
+
+_DESCRIPTION = """
+This metric computes the area under the curve (AUC) for the Receiver Operating Characteristic Curve (ROC). The return values represent how well the model used is predicting the correct classes, based on the input data. A score of `0.5` means that the model is predicting exactly at chance, i.e. the model's predictions are correct at the same rate as if the predictions were being decided by the flip of a fair coin or the roll of a fair die. A score above `0.5` indicates that the model is doing better than chance, while a score below `0.5` indicates that the model is doing worse than chance.
+
+This metric has three separate use cases:
+ - binary: The case in which there are only two different label classes, and each example gets only one label. This is the default implementation.
+ - multiclass: The case in which there can be more than two different label classes, but each example still gets only one label.
+ - multilabel: The case in which there can be more than two different label classes, and each example can have more than one label.
+"""
+
+_KWARGS_DESCRIPTION = """
+Args:
+- references (array-like of shape (n_samples,) or (n_samples, n_classes)): Ground truth labels. Expects different input based on use case:
+ - binary: expects an array-like of shape (n_samples,)
+ - multiclass: expects an array-like of shape (n_samples,)
+ - multilabel: expects an array-like of shape (n_samples, n_classes)
+- prediction_scores (array-like of shape (n_samples,) or (n_samples, n_classes)): Model predictions. Expects different inputs based on use case:
+ - binary: expects an array-like of shape (n_samples,)
+ - multiclass: expects an array-like of shape (n_samples, n_classes)
+ - multilabel: expects an array-like of shape (n_samples, n_classes)
+- average (`str`): Type of average, and is ignored in the binary use case. Defaults to 'macro'. Options are:
+ - `'micro'`: Calculates metrics globally by considering each element of the label indicator matrix as a label. Only works with the multilabel use case.
+ - `'macro'`: Calculate metrics for each label, and find their unweighted mean. This does not take label imbalance into account.
+ - `'weighted'`: Calculate metrics for each label, and find their average, weighted by support (i.e. the number of true instances for each label).
+ - `'samples'`: Calculate metrics for each instance, and find their average. Only works with the multilabel use case.
+ - `None`: No average is calculated, and scores for each class are returned. Only works with the multilabels use case.
+- sample_weight (array-like of shape (n_samples,)): Sample weights. Defaults to None.
+- max_fpr (`float`): If not None, the standardized partial AUC over the range [0, `max_fpr`] is returned. Must be greater than `0` and less than or equal to `1`. Defaults to `None`. Note: For the multiclass use case, `max_fpr` should be either `None` or `1.0` as ROC AUC partial computation is not currently supported for `multiclass`.
+- multi_class (`str`): Only used for multiclass targets, where it is required. Determines the type of configuration to use. Options are:
+ - `'ovr'`: Stands for One-vs-rest. Computes the AUC of each class against the rest. This treats the multiclass case in the same way as the multilabel case. Sensitive to class imbalance even when `average == 'macro'`, because class imbalance affects the composition of each of the 'rest' groupings.
+ - `'ovo'`: Stands for One-vs-one. Computes the average AUC of all possible pairwise combinations of classes. Insensitive to class imbalance when `average == 'macro'`.
+- labels (array-like of shape (n_classes,)): Only used for multiclass targets. List of labels that index the classes in
+ `prediction_scores`. If `None`, the numerical or lexicographical order of the labels in
+ `prediction_scores` is used. Defaults to `None`.
+Returns:
+ roc_auc (`float` or array-like of shape (n_classes,)): Returns array if in multilabel use case and `average='None'`. Otherwise, returns `float`.
+Examples:
+ Example 1:
+ >>> roc_auc_score = datasets.load_metric("roc_auc")
+ >>> refs = [1, 0, 1, 1, 0, 0]
+ >>> pred_scores = [0.5, 0.2, 0.99, 0.3, 0.1, 0.7]
+ >>> results = roc_auc_score.compute(references=refs, prediction_scores=pred_scores)
+ >>> print(round(results['roc_auc'], 2))
+ 0.78
+
+ Example 2:
+ >>> roc_auc_score = datasets.load_metric("roc_auc", "multiclass")
+ >>> refs = [1, 0, 1, 2, 2, 0]
+ >>> pred_scores = [[0.3, 0.5, 0.2],
+ ... [0.7, 0.2, 0.1],
+ ... [0.005, 0.99, 0.005],
+ ... [0.2, 0.3, 0.5],
+ ... [0.1, 0.1, 0.8],
+ ... [0.1, 0.7, 0.2]]
+ >>> results = roc_auc_score.compute(references=refs, prediction_scores=pred_scores, multi_class='ovr')
+ >>> print(round(results['roc_auc'], 2))
+ 0.85
+
+ Example 3:
+ >>> roc_auc_score = datasets.load_metric("roc_auc", "multilabel")
+ >>> refs = [[1, 1, 0],
+ ... [1, 1, 0],
+ ... [0, 1, 0],
+ ... [0, 0, 1],
+ ... [0, 1, 1],
+ ... [1, 0, 1]]
+ >>> pred_scores = [[0.3, 0.5, 0.2],
+ ... [0.7, 0.2, 0.1],
+ ... [0.005, 0.99, 0.005],
+ ... [0.2, 0.3, 0.5],
+ ... [0.1, 0.1, 0.8],
+ ... [0.1, 0.7, 0.2]]
+ >>> results = roc_auc_score.compute(references=refs, prediction_scores=pred_scores, average=None)
+ >>> print([round(res, 2) for res in results['roc_auc']])
+ [0.83, 0.38, 0.94]
+"""
+
+_CITATION = """\
+@article{doi:10.1177/0272989X8900900307,
+author = {Donna Katzman McClish},
+title ={Analyzing a Portion of the ROC Curve},
+journal = {Medical Decision Making},
+volume = {9},
+number = {3},
+pages = {190-195},
+year = {1989},
+doi = {10.1177/0272989X8900900307},
+ note ={PMID: 2668680},
+URL = {https://doi.org/10.1177/0272989X8900900307},
+eprint = {https://doi.org/10.1177/0272989X8900900307}
+}
+
+
+@article{10.1023/A:1010920819831,
+author = {Hand, David J. and Till, Robert J.},
+title = {A Simple Generalisation of the Area Under the ROC Curve for Multiple Class Classification Problems},
+year = {2001},
+issue_date = {November 2001},
+publisher = {Kluwer Academic Publishers},
+address = {USA},
+volume = {45},
+number = {2},
+issn = {0885-6125},
+url = {https://doi.org/10.1023/A:1010920819831},
+doi = {10.1023/A:1010920819831},
+journal = {Mach. Learn.},
+month = {oct},
+pages = {171–186},
+numpages = {16},
+keywords = {Gini index, AUC, error rate, ROC curve, receiver operating characteristic}
+}
+
+
+@article{scikit-learn,
+title={Scikit-learn: Machine Learning in {P}ython},
+author={Pedregosa, F. and Varoquaux, G. and Gramfort, A. and Michel, V. and Thirion, B. and Grisel, O. and Blondel, M. and Prettenhofer, P. and Weiss, R. and Dubourg, V. and Vanderplas, J. and Passos, A. and Cournapeau, D. and Brucher, M. and Perrot, M. and Duchesnay, E.},
+journal={Journal of Machine Learning Research},
+volume={12},
+pages={2825--2830},
+year={2011}
+}
+"""
+
+
+@datasets.utils.file_utils.add_start_docstrings(_DESCRIPTION, _KWARGS_DESCRIPTION)
+class ROCAUC(datasets.Metric):
+ def _info(self):
+ return datasets.MetricInfo(
+ description=_DESCRIPTION,
+ citation=_CITATION,
+ inputs_description=_KWARGS_DESCRIPTION,
+ features=datasets.Features(
+ {
+ "prediction_scores": datasets.Sequence(datasets.Value("float")),
+ "references": datasets.Value("int32"),
+ }
+ if self.config_name == "multiclass"
+ else {
+ "references": datasets.Sequence(datasets.Value("int32")),
+ "prediction_scores": datasets.Sequence(datasets.Value("float")),
+ }
+ if self.config_name == "multilabel"
+ else {
+ "references": datasets.Value("int32"),
+ "prediction_scores": datasets.Value("float"),
+ }
+ ),
+ reference_urls=["https://scikit-learn.org/stable/modules/generated/sklearn.metrics.roc_auc_score.html"],
+ )
+
+ def _compute(
+ self,
+ references,
+ prediction_scores,
+ average="macro",
+ sample_weight=None,
+ max_fpr=None,
+ multi_class="raise",
+ labels=None,
+ ):
+ return {
+ "roc_auc": roc_auc_score(
+ references,
+ prediction_scores,
+ average=average,
+ sample_weight=sample_weight,
+ max_fpr=max_fpr,
+ multi_class=multi_class,
+ labels=labels,
+ )
+ }
diff --git a/metrics/rouge/README.md b/metrics/rouge/README.md
new file mode 100644
index 0000000..3bfb569
--- /dev/null
+++ b/metrics/rouge/README.md
@@ -0,0 +1,121 @@
+# Metric Card for ROUGE
+
+## Metric Description
+ROUGE, or Recall-Oriented Understudy for Gisting Evaluation, is a set of metrics and a software package used for evaluating automatic summarization and machine translation software in natural language processing. The metrics compare an automatically produced summary or translation against a reference or a set of references (human-produced) summary or translation.
+
+Note that ROUGE is case insensitive, meaning that upper case letters are treated the same way as lower case letters.
+
+This metrics is a wrapper around the [Google Research reimplementation of ROUGE](https://github.com/google-research/google-research/tree/master/rouge)
+
+## How to Use
+At minimum, this metric takes as input a list of predictions and a list of references:
+```python
+>>> rouge = datasets.load_metric('rouge')
+>>> predictions = ["hello there", "general kenobi"]
+>>> references = ["hello there", "general kenobi"]
+>>> results = rouge.compute(predictions=predictions,
+... references=references)
+>>> print(list(results.keys()))
+['rouge1', 'rouge2', 'rougeL', 'rougeLsum']
+>>> print(results["rouge1"])
+AggregateScore(low=Score(precision=1.0, recall=1.0, fmeasure=1.0), mid=Score(precision=1.0, recall=1.0, fmeasure=1.0), high=Score(precision=1.0, recall=1.0, fmeasure=1.0))
+>>> print(results["rouge1"].mid.fmeasure)
+1.0
+```
+
+### Inputs
+- **predictions** (`list`): list of predictions to score. Each prediction
+ should be a string with tokens separated by spaces.
+- **references** (`list`): list of reference for each prediction. Each
+ reference should be a string with tokens separated by spaces.
+- **rouge_types** (`list`): A list of rouge types to calculate. Defaults to `['rouge1', 'rouge2', 'rougeL', 'rougeLsum']`.
+ - Valid rouge types:
+ - `"rouge1"`: unigram (1-gram) based scoring
+ - `"rouge2"`: bigram (2-gram) based scoring
+ - `"rougeL"`: Longest common subsequence based scoring.
+ - `"rougeLSum"`: splits text using `"\n"`
+ - See [here](https://github.com/huggingface/datasets/issues/617) for more information
+- **use_aggregator** (`boolean`): If True, returns aggregates. Defaults to `True`.
+- **use_stemmer** (`boolean`): If `True`, uses Porter stemmer to strip word suffixes. Defaults to `False`.
+
+### Output Values
+The output is a dictionary with one entry for each rouge type in the input list `rouge_types`. If `use_aggregator=False`, each dictionary entry is a list of Score objects, with one score for each sentence. Each Score object includes the `precision`, `recall`, and `fmeasure`. E.g. if `rouge_types=['rouge1', 'rouge2']` and `use_aggregator=False`, the output is:
+
+```python
+{'rouge1': [Score(precision=1.0, recall=0.5, fmeasure=0.6666666666666666), Score(precision=1.0, recall=1.0, fmeasure=1.0)], 'rouge2': [Score(precision=0.0, recall=0.0, fmeasure=0.0), Score(precision=1.0, recall=1.0, fmeasure=1.0)]}
+```
+
+If `rouge_types=['rouge1', 'rouge2']` and `use_aggregator=True`, the output is of the following format:
+```python
+{'rouge1': AggregateScore(low=Score(precision=1.0, recall=1.0, fmeasure=1.0), mid=Score(precision=1.0, recall=1.0, fmeasure=1.0), high=Score(precision=1.0, recall=1.0, fmeasure=1.0)), 'rouge2': AggregateScore(low=Score(precision=1.0, recall=1.0, fmeasure=1.0), mid=Score(precision=1.0, recall=1.0, fmeasure=1.0), high=Score(precision=1.0, recall=1.0, fmeasure=1.0))}
+```
+
+The `precision`, `recall`, and `fmeasure` values all have a range of 0 to 1.
+
+
+#### Values from Popular Papers
+
+
+### Examples
+An example without aggregation:
+```python
+>>> rouge = datasets.load_metric('rouge')
+>>> predictions = ["hello goodbye", "ankh morpork"]
+>>> references = ["goodbye", "general kenobi"]
+>>> results = rouge.compute(predictions=predictions,
+... references=references)
+>>> print(list(results.keys()))
+['rouge1', 'rouge2', 'rougeL', 'rougeLsum']
+>>> print(results["rouge1"])
+[Score(precision=0.5, recall=0.5, fmeasure=0.5), Score(precision=0.0, recall=0.0, fmeasure=0.0)]
+```
+
+The same example, but with aggregation:
+```python
+>>> rouge = datasets.load_metric('rouge')
+>>> predictions = ["hello goodbye", "ankh morpork"]
+>>> references = ["goodbye", "general kenobi"]
+>>> results = rouge.compute(predictions=predictions,
+... references=references,
+... use_aggregator=True)
+>>> print(list(results.keys()))
+['rouge1', 'rouge2', 'rougeL', 'rougeLsum']
+>>> print(results["rouge1"])
+AggregateScore(low=Score(precision=0.0, recall=0.0, fmeasure=0.0), mid=Score(precision=0.25, recall=0.25, fmeasure=0.25), high=Score(precision=0.5, recall=0.5, fmeasure=0.5))
+```
+
+The same example, but only calculating `rouge_1`:
+```python
+>>> rouge = datasets.load_metric('rouge')
+>>> predictions = ["hello goodbye", "ankh morpork"]
+>>> references = ["goodbye", "general kenobi"]
+>>> results = rouge.compute(predictions=predictions,
+... references=references,
+... rouge_types=['rouge_1'],
+... use_aggregator=True)
+>>> print(list(results.keys()))
+['rouge1']
+>>> print(results["rouge1"])
+AggregateScore(low=Score(precision=0.0, recall=0.0, fmeasure=0.0), mid=Score(precision=0.25, recall=0.25, fmeasure=0.25), high=Score(precision=0.5, recall=0.5, fmeasure=0.5))
+```
+
+## Limitations and Bias
+See [Schluter (2017)](https://aclanthology.org/E17-2007/) for an in-depth discussion of many of ROUGE's limits.
+
+## Citation
+```bibtex
+@inproceedings{lin-2004-rouge,
+ title = "{ROUGE}: A Package for Automatic Evaluation of Summaries",
+ author = "Lin, Chin-Yew",
+ booktitle = "Text Summarization Branches Out",
+ month = jul,
+ year = "2004",
+ address = "Barcelona, Spain",
+ publisher = "Association for Computational Linguistics",
+ url = "https://www.aclweb.org/anthology/W04-1013",
+ pages = "74--81",
+}
+```
+
+## Further References
+- This metrics is a wrapper around the [Google Research reimplementation of ROUGE](https://github.com/google-research/google-research/tree/master/rouge)
\ No newline at end of file
diff --git a/metrics/rouge/rouge.py b/metrics/rouge/rouge.py
new file mode 100644
index 0000000..df7a5e4
--- /dev/null
+++ b/metrics/rouge/rouge.py
@@ -0,0 +1,130 @@
+# Copyright 2020 The HuggingFace Datasets Authors.
+#
+# Licensed under the Apache License, Version 2.0 (the "License");
+# you may not use this file except in compliance with the License.
+# You may obtain a copy of the License at
+#
+# http://www.apache.org/licenses/LICENSE-2.0
+#
+# Unless required by applicable law or agreed to in writing, software
+# distributed under the License is distributed on an "AS IS" BASIS,
+# WITHOUT WARRANTIES OR CONDITIONS OF ANY KIND, either express or implied.
+# See the License for the specific language governing permissions and
+# limitations under the License.
+""" ROUGE metric from Google Research github repo. """
+
+# The dependencies in https://github.com/google-research/google-research/blob/master/rouge/requirements.txt
+import absl # Here to have a nice missing dependency error message early on
+import nltk # Here to have a nice missing dependency error message early on
+import numpy # Here to have a nice missing dependency error message early on
+import six # Here to have a nice missing dependency error message early on
+from rouge_score import rouge_scorer, scoring
+
+import datasets
+
+
+_CITATION = """\
+@inproceedings{lin-2004-rouge,
+ title = "{ROUGE}: A Package for Automatic Evaluation of Summaries",
+ author = "Lin, Chin-Yew",
+ booktitle = "Text Summarization Branches Out",
+ month = jul,
+ year = "2004",
+ address = "Barcelona, Spain",
+ publisher = "Association for Computational Linguistics",
+ url = "https://www.aclweb.org/anthology/W04-1013",
+ pages = "74--81",
+}
+"""
+
+_DESCRIPTION = """\
+ROUGE, or Recall-Oriented Understudy for Gisting Evaluation, is a set of metrics and a software package used for
+evaluating automatic summarization and machine translation software in natural language processing.
+The metrics compare an automatically produced summary or translation against a reference or a set of references (human-produced) summary or translation.
+
+Note that ROUGE is case insensitive, meaning that upper case letters are treated the same way as lower case letters.
+
+This metrics is a wrapper around Google Research reimplementation of ROUGE:
+https://github.com/google-research/google-research/tree/master/rouge
+"""
+
+_KWARGS_DESCRIPTION = """
+Calculates average rouge scores for a list of hypotheses and references
+Args:
+ predictions: list of predictions to score. Each prediction
+ should be a string with tokens separated by spaces.
+ references: list of reference for each prediction. Each
+ reference should be a string with tokens separated by spaces.
+ rouge_types: A list of rouge types to calculate.
+ Valid names:
+ `"rouge{n}"` (e.g. `"rouge1"`, `"rouge2"`) where: {n} is the n-gram based scoring,
+ `"rougeL"`: Longest common subsequence based scoring.
+ `"rougeLSum"`: rougeLsum splits text using `"\n"`.
+ See details in https://github.com/huggingface/datasets/issues/617
+ use_stemmer: Bool indicating whether Porter stemmer should be used to strip word suffixes.
+ use_aggregator: Return aggregates if this is set to True
+Returns:
+ rouge1: rouge_1 (precision, recall, f1),
+ rouge2: rouge_2 (precision, recall, f1),
+ rougeL: rouge_l (precision, recall, f1),
+ rougeLsum: rouge_lsum (precision, recall, f1)
+Examples:
+
+ >>> rouge = datasets.load_metric('rouge')
+ >>> predictions = ["hello there", "general kenobi"]
+ >>> references = ["hello there", "general kenobi"]
+ >>> results = rouge.compute(predictions=predictions, references=references)
+ >>> print(list(results.keys()))
+ ['rouge1', 'rouge2', 'rougeL', 'rougeLsum']
+ >>> print(results["rouge1"])
+ AggregateScore(low=Score(precision=1.0, recall=1.0, fmeasure=1.0), mid=Score(precision=1.0, recall=1.0, fmeasure=1.0), high=Score(precision=1.0, recall=1.0, fmeasure=1.0))
+ >>> print(results["rouge1"].mid.fmeasure)
+ 1.0
+"""
+
+
+@datasets.utils.file_utils.add_start_docstrings(_DESCRIPTION, _KWARGS_DESCRIPTION)
+class Rouge(datasets.Metric):
+ def _info(self):
+ return datasets.MetricInfo(
+ description=_DESCRIPTION,
+ citation=_CITATION,
+ inputs_description=_KWARGS_DESCRIPTION,
+ features=datasets.Features(
+ {
+ "predictions": datasets.Value("string", id="sequence"),
+ "references": datasets.Value("string", id="sequence"),
+ }
+ ),
+ codebase_urls=["https://github.com/google-research/google-research/tree/master/rouge"],
+ reference_urls=[
+ "https://en.wikipedia.org/wiki/ROUGE_(metric)",
+ "https://github.com/google-research/google-research/tree/master/rouge",
+ ],
+ )
+
+ def _compute(self, predictions, references, rouge_types=None, use_aggregator=True, use_stemmer=False):
+ if rouge_types is None:
+ rouge_types = ["rouge1", "rouge2", "rougeL", "rougeLsum"]
+
+ scorer = rouge_scorer.RougeScorer(rouge_types=rouge_types, use_stemmer=use_stemmer)
+ if use_aggregator:
+ aggregator = scoring.BootstrapAggregator()
+ else:
+ scores = []
+
+ for ref, pred in zip(references, predictions):
+ score = scorer.score(ref, pred)
+ if use_aggregator:
+ aggregator.add_scores(score)
+ else:
+ scores.append(score)
+
+ if use_aggregator:
+ result = aggregator.aggregate()
+ else:
+ result = {}
+ for key in scores[0]:
+ result[key] = list(score[key] for score in scores)
+
+ return result
diff --git a/metrics/sacrebleu/README.md b/metrics/sacrebleu/README.md
new file mode 100644
index 0000000..e2ea7fb
--- /dev/null
+++ b/metrics/sacrebleu/README.md
@@ -0,0 +1,99 @@
+# Metric Card for SacreBLEU
+
+
+## Metric Description
+SacreBLEU provides hassle-free computation of shareable, comparable, and reproducible BLEU scores. Inspired by Rico Sennrich's `multi-bleu-detok.perl`, it produces the official Workshop on Machine Translation (WMT) scores but works with plain text. It also knows all the standard test sets and handles downloading, processing, and tokenization.
+
+See the [README.md] file at https://github.com/mjpost/sacreBLEU for more information.
+
+## How to Use
+This metric takes a set of predictions and a set of references as input, along with various optional parameters.
+
+
+```python
+>>> predictions = ["hello there general kenobi", "foo bar foobar"]
+>>> references = [["hello there general kenobi", "hello there !"],
+... ["foo bar foobar", "foo bar foobar"]]
+>>> sacrebleu = datasets.load_metric("sacrebleu")
+>>> results = sacrebleu.compute(predictions=predictions,
+... references=references)
+>>> print(list(results.keys()))
+['score', 'counts', 'totals', 'precisions', 'bp', 'sys_len', 'ref_len']
+>>> print(round(results["score"], 1))
+100.0
+```
+
+### Inputs
+- **`predictions`** (`list` of `str`): list of translations to score. Each translation should be tokenized into a list of tokens.
+- **`references`** (`list` of `list` of `str`): A list of lists of references. The contents of the first sub-list are the references for the first prediction, the contents of the second sub-list are for the second prediction, etc. Note that there must be the same number of references for each prediction (i.e. all sub-lists must be of the same length).
+- **`smooth_method`** (`str`): The smoothing method to use, defaults to `'exp'`. Possible values are:
+ - `'none'`: no smoothing
+ - `'floor'`: increment zero counts
+ - `'add-k'`: increment num/denom by k for n>1
+ - `'exp'`: exponential decay
+- **`smooth_value`** (`float`): The smoothing value. Only valid when `smooth_method='floor'` (in which case `smooth_value` defaults to `0.1`) or `smooth_method='add-k'` (in which case `smooth_value` defaults to `1`).
+- **`tokenize`** (`str`): Tokenization method to use for BLEU. If not provided, defaults to `'zh'` for Chinese, `'ja-mecab'` for Japanese and `'13a'` (mteval) otherwise. Possible values are:
+ - `'none'`: No tokenization.
+ - `'zh'`: Chinese tokenization.
+ - `'13a'`: mimics the `mteval-v13a` script from Moses.
+ - `'intl'`: International tokenization, mimics the `mteval-v14` script from Moses
+ - `'char'`: Language-agnostic character-level tokenization.
+ - `'ja-mecab'`: Japanese tokenization. Uses the [MeCab tokenizer](https://pypi.org/project/mecab-python3).
+- **`lowercase`** (`bool`): If `True`, lowercases the input, enabling case-insensitivity. Defaults to `False`.
+- **`force`** (`bool`): If `True`, insists that your tokenized input is actually detokenized. Defaults to `False`.
+- **`use_effective_order`** (`bool`): If `True`, stops including n-gram orders for which precision is 0. This should be `True`, if sentence-level BLEU will be computed. Defaults to `False`.
+
+### Output Values
+- `score`: BLEU score
+- `counts`: Counts
+- `totals`: Totals
+- `precisions`: Precisions
+- `bp`: Brevity penalty
+- `sys_len`: predictions length
+- `ref_len`: reference length
+
+The output is in the following format:
+```python
+{'score': 39.76353643835252, 'counts': [6, 4, 2, 1], 'totals': [10, 8, 6, 4], 'precisions': [60.0, 50.0, 33.333333333333336, 25.0], 'bp': 1.0, 'sys_len': 10, 'ref_len': 7}
+```
+The score can take any value between `0.0` and `100.0`, inclusive.
+
+#### Values from Popular Papers
+
+
+### Examples
+
+```python
+>>> predictions = ["hello there general kenobi",
+... "on our way to ankh morpork"]
+>>> references = [["hello there general kenobi", "hello there !"],
+... ["goodbye ankh morpork", "ankh morpork"]]
+>>> sacrebleu = datasets.load_metric("sacrebleu")
+>>> results = sacrebleu.compute(predictions=predictions,
+... references=references)
+>>> print(list(results.keys()))
+['score', 'counts', 'totals', 'precisions', 'bp', 'sys_len', 'ref_len']
+>>> print(round(results["score"], 1))
+39.8
+```
+
+## Limitations and Bias
+Because what this metric calculates is BLEU scores, it has the same limitations as that metric, except that sacreBLEU is more easily reproducible.
+
+## Citation
+```bibtex
+@inproceedings{post-2018-call,
+ title = "A Call for Clarity in Reporting {BLEU} Scores",
+ author = "Post, Matt",
+ booktitle = "Proceedings of the Third Conference on Machine Translation: Research Papers",
+ month = oct,
+ year = "2018",
+ address = "Belgium, Brussels",
+ publisher = "Association for Computational Linguistics",
+ url = "https://www.aclweb.org/anthology/W18-6319",
+ pages = "186--191",
+}
+```
+
+## Further References
+- See the [sacreBLEU README.md file](https://github.com/mjpost/sacreBLEU) for more information.
\ No newline at end of file
diff --git a/metrics/sacrebleu/sacrebleu.py b/metrics/sacrebleu/sacrebleu.py
new file mode 100644
index 0000000..f6f3a5b
--- /dev/null
+++ b/metrics/sacrebleu/sacrebleu.py
@@ -0,0 +1,165 @@
+# Copyright 2020 The HuggingFace Datasets Authors.
+#
+# Licensed under the Apache License, Version 2.0 (the "License");
+# you may not use this file except in compliance with the License.
+# You may obtain a copy of the License at
+#
+# http://www.apache.org/licenses/LICENSE-2.0
+#
+# Unless required by applicable law or agreed to in writing, software
+# distributed under the License is distributed on an "AS IS" BASIS,
+# WITHOUT WARRANTIES OR CONDITIONS OF ANY KIND, either express or implied.
+# See the License for the specific language governing permissions and
+# limitations under the License.
+""" SACREBLEU metric. """
+
+import sacrebleu as scb
+from packaging import version
+
+import datasets
+
+
+_CITATION = """\
+@inproceedings{post-2018-call,
+ title = "A Call for Clarity in Reporting {BLEU} Scores",
+ author = "Post, Matt",
+ booktitle = "Proceedings of the Third Conference on Machine Translation: Research Papers",
+ month = oct,
+ year = "2018",
+ address = "Belgium, Brussels",
+ publisher = "Association for Computational Linguistics",
+ url = "https://www.aclweb.org/anthology/W18-6319",
+ pages = "186--191",
+}
+"""
+
+_DESCRIPTION = """\
+SacreBLEU provides hassle-free computation of shareable, comparable, and reproducible BLEU scores.
+Inspired by Rico Sennrich's `multi-bleu-detok.perl`, it produces the official WMT scores but works with plain text.
+It also knows all the standard test sets and handles downloading, processing, and tokenization for you.
+
+See the [README.md] file at https://github.com/mjpost/sacreBLEU for more information.
+"""
+
+_KWARGS_DESCRIPTION = """
+Produces BLEU scores along with its sufficient statistics
+from a source against one or more references.
+
+Args:
+ predictions (`list` of `str`): list of translations to score. Each translation should be tokenized into a list of tokens.
+ references (`list` of `list` of `str`): A list of lists of references. The contents of the first sub-list are the references for the first prediction, the contents of the second sub-list are for the second prediction, etc. Note that there must be the same number of references for each prediction (i.e. all sub-lists must be of the same length).
+ smooth_method (`str`): The smoothing method to use, defaults to `'exp'`. Possible values are:
+ - `'none'`: no smoothing
+ - `'floor'`: increment zero counts
+ - `'add-k'`: increment num/denom by k for n>1
+ - `'exp'`: exponential decay
+ smooth_value (`float`): The smoothing value. Only valid when `smooth_method='floor'` (in which case `smooth_value` defaults to `0.1`) or `smooth_method='add-k'` (in which case `smooth_value` defaults to `1`).
+ tokenize (`str`): Tokenization method to use for BLEU. If not provided, defaults to `'zh'` for Chinese, `'ja-mecab'` for Japanese and `'13a'` (mteval) otherwise. Possible values are:
+ - `'none'`: No tokenization.
+ - `'zh'`: Chinese tokenization.
+ - `'13a'`: mimics the `mteval-v13a` script from Moses.
+ - `'intl'`: International tokenization, mimics the `mteval-v14` script from Moses
+ - `'char'`: Language-agnostic character-level tokenization.
+ - `'ja-mecab'`: Japanese tokenization. Uses the [MeCab tokenizer](https://pypi.org/project/mecab-python3).
+ lowercase (`bool`): If `True`, lowercases the input, enabling case-insensitivity. Defaults to `False`.
+ force (`bool`): If `True`, insists that your tokenized input is actually detokenized. Defaults to `False`.
+ use_effective_order (`bool`): If `True`, stops including n-gram orders for which precision is 0. This should be `True`, if sentence-level BLEU will be computed. Defaults to `False`.
+
+Returns:
+ 'score': BLEU score,
+ 'counts': Counts,
+ 'totals': Totals,
+ 'precisions': Precisions,
+ 'bp': Brevity penalty,
+ 'sys_len': predictions length,
+ 'ref_len': reference length,
+
+Examples:
+
+ Example 1:
+ >>> predictions = ["hello there general kenobi", "foo bar foobar"]
+ >>> references = [["hello there general kenobi", "hello there !"], ["foo bar foobar", "foo bar foobar"]]
+ >>> sacrebleu = datasets.load_metric("sacrebleu")
+ >>> results = sacrebleu.compute(predictions=predictions, references=references)
+ >>> print(list(results.keys()))
+ ['score', 'counts', 'totals', 'precisions', 'bp', 'sys_len', 'ref_len']
+ >>> print(round(results["score"], 1))
+ 100.0
+
+ Example 2:
+ >>> predictions = ["hello there general kenobi",
+ ... "on our way to ankh morpork"]
+ >>> references = [["hello there general kenobi", "hello there !"],
+ ... ["goodbye ankh morpork", "ankh morpork"]]
+ >>> sacrebleu = datasets.load_metric("sacrebleu")
+ >>> results = sacrebleu.compute(predictions=predictions,
+ ... references=references)
+ >>> print(list(results.keys()))
+ ['score', 'counts', 'totals', 'precisions', 'bp', 'sys_len', 'ref_len']
+ >>> print(round(results["score"], 1))
+ 39.8
+"""
+
+
+@datasets.utils.file_utils.add_start_docstrings(_DESCRIPTION, _KWARGS_DESCRIPTION)
+class Sacrebleu(datasets.Metric):
+ def _info(self):
+ if version.parse(scb.__version__) < version.parse("1.4.12"):
+ raise ImportWarning(
+ "To use `sacrebleu`, the module `sacrebleu>=1.4.12` is required, and the current version of `sacrebleu` doesn't match this condition.\n"
+ 'You can install it with `pip install "sacrebleu>=1.4.12"`.'
+ )
+ return datasets.MetricInfo(
+ description=_DESCRIPTION,
+ citation=_CITATION,
+ homepage="https://github.com/mjpost/sacreBLEU",
+ inputs_description=_KWARGS_DESCRIPTION,
+ features=datasets.Features(
+ {
+ "predictions": datasets.Value("string", id="sequence"),
+ "references": datasets.Sequence(datasets.Value("string", id="sequence"), id="references"),
+ }
+ ),
+ codebase_urls=["https://github.com/mjpost/sacreBLEU"],
+ reference_urls=[
+ "https://github.com/mjpost/sacreBLEU",
+ "https://en.wikipedia.org/wiki/BLEU",
+ "https://towardsdatascience.com/evaluating-text-output-in-nlp-bleu-at-your-own-risk-e8609665a213",
+ ],
+ )
+
+ def _compute(
+ self,
+ predictions,
+ references,
+ smooth_method="exp",
+ smooth_value=None,
+ force=False,
+ lowercase=False,
+ tokenize=None,
+ use_effective_order=False,
+ ):
+ references_per_prediction = len(references[0])
+ if any(len(refs) != references_per_prediction for refs in references):
+ raise ValueError("Sacrebleu requires the same number of references for each prediction")
+ transformed_references = [[refs[i] for refs in references] for i in range(references_per_prediction)]
+ output = scb.corpus_bleu(
+ predictions,
+ transformed_references,
+ smooth_method=smooth_method,
+ smooth_value=smooth_value,
+ force=force,
+ lowercase=lowercase,
+ use_effective_order=use_effective_order,
+ **(dict(tokenize=tokenize) if tokenize else {}),
+ )
+ output_dict = {
+ "score": output.score,
+ "counts": output.counts,
+ "totals": output.totals,
+ "precisions": output.precisions,
+ "bp": output.bp,
+ "sys_len": output.sys_len,
+ "ref_len": output.ref_len,
+ }
+ return output_dict
diff --git a/metrics/sari/README.md b/metrics/sari/README.md
new file mode 100644
index 0000000..d5292b5
--- /dev/null
+++ b/metrics/sari/README.md
@@ -0,0 +1,111 @@
+# Metric Card for SARI
+
+
+## Metric description
+SARI (***s**ystem output **a**gainst **r**eferences and against the **i**nput sentence*) is a metric used for evaluating automatic text simplification systems.
+
+The metric compares the predicted simplified sentences against the reference and the source sentences. It explicitly measures the goodness of words that are added, deleted and kept by the system.
+
+SARI can be computed as:
+
+`sari = ( F1_add + F1_keep + P_del) / 3`
+
+where
+
+`F1_add` is the n-gram F1 score for add operations
+
+`F1_keep` is the n-gram F1 score for keep operations
+
+`P_del` is the n-gram precision score for delete operations
+
+The number of n grams, `n`, is equal to 4, as in the original paper.
+
+This implementation is adapted from [Tensorflow's tensor2tensor implementation](https://github.com/tensorflow/tensor2tensor/blob/master/tensor2tensor/utils/sari_hook.py).
+It has two differences with the [original GitHub implementation](https://github.com/cocoxu/simplification/blob/master/SARI.py):
+
+1) It defines 0/0=1 instead of 0 to give higher scores for predictions that match a target exactly.
+2) It fixes an [alleged bug](https://github.com/cocoxu/simplification/issues/6) in the keep score computation.
+
+
+
+## How to use
+
+The metric takes 3 inputs: sources (a list of source sentence strings), predictions (a list of predicted sentence strings) and references (a list of lists of reference sentence strings)
+
+```python
+from datasets import load_metric
+sari = load_metric("sari")
+sources=["About 95 species are currently accepted."]
+predictions=["About 95 you now get in."]
+references=[["About 95 species are currently known.","About 95 species are now accepted.","95 species are now accepted."]]
+sari_score = sari.compute(sources=sources, predictions=predictions, references=references)
+```
+## Output values
+
+This metric outputs a dictionary with the SARI score:
+
+```
+print(sari_score)
+{'sari': 26.953601953601954}
+```
+
+The range of values for the SARI score is between 0 and 100 -- the higher the value, the better the performance of the model being evaluated, with a SARI of 100 being a perfect score.
+
+### Values from popular papers
+
+The [original paper that proposes the SARI metric](https://aclanthology.org/Q16-1029.pdf) reports scores ranging from 26 to 43 for different simplification systems and different datasets. They also find that the metric ranks all of the simplification systems and human references in the same order as the human assessment used as a comparison, and that it correlates reasonably with human judgments.
+
+More recent SARI scores for text simplification can be found on leaderboards for datasets such as [TurkCorpus](https://paperswithcode.com/sota/text-simplification-on-turkcorpus) and [Newsela](https://paperswithcode.com/sota/text-simplification-on-newsela).
+
+## Examples
+
+Perfect match between prediction and reference:
+
+```python
+from datasets import load_metric
+sari = load_metric("sari")
+sources=["About 95 species are currently accepted ."]
+predictions=["About 95 species are currently accepted ."]
+references=[["About 95 species are currently accepted ."]]
+sari_score = sari.compute(sources=sources, predictions=predictions, references=references)
+print(sari_score)
+{'sari': 100.0}
+```
+
+Partial match between prediction and reference:
+
+```python
+from datasets import load_metric
+sari = load_metric("sari")
+sources=["About 95 species are currently accepted ."]
+predictions=["About 95 you now get in ."]
+references=[["About 95 species are currently known .","About 95 species are now accepted .","95 species are now accepted ."]]
+sari_score = sari.compute(sources=sources, predictions=predictions, references=references)
+print(sari_score)
+{'sari': 26.953601953601954}
+```
+
+## Limitations and bias
+
+SARI is a valuable measure for comparing different text simplification systems as well as one that can assist the iterative development of a system.
+
+However, while the [original paper presenting SARI](https://aclanthology.org/Q16-1029.pdf) states that it captures "the notion of grammaticality and meaning preservation", this is a difficult claim to empirically validate.
+
+## Citation
+
+```bibtex
+@inproceedings{xu-etal-2016-optimizing,
+title = {Optimizing Statistical Machine Translation for Text Simplification},
+authors={Xu, Wei and Napoles, Courtney and Pavlick, Ellie and Chen, Quanze and Callison-Burch, Chris},
+journal = {Transactions of the Association for Computational Linguistics},
+volume = {4},
+year={2016},
+url = {https://www.aclweb.org/anthology/Q16-1029},
+pages = {401--415},
+}
+```
+
+## Further References
+
+- [NLP Progress -- Text Simplification](http://nlpprogress.com/english/simplification.html)
+- [Hugging Face Hub -- Text Simplification Models](https://huggingface.co/datasets?filter=task_ids:text-simplification)
diff --git a/metrics/sari/sari.py b/metrics/sari/sari.py
new file mode 100644
index 0000000..d20aa08
--- /dev/null
+++ b/metrics/sari/sari.py
@@ -0,0 +1,288 @@
+# Copyright 2020 The HuggingFace Datasets Authors and the current dataset script contributor.
+#
+# Licensed under the Apache License, Version 2.0 (the "License");
+# you may not use this file except in compliance with the License.
+# You may obtain a copy of the License at
+#
+# http://www.apache.org/licenses/LICENSE-2.0
+#
+# Unless required by applicable law or agreed to in writing, software
+# distributed under the License is distributed on an "AS IS" BASIS,
+# WITHOUT WARRANTIES OR CONDITIONS OF ANY KIND, either express or implied.
+# See the License for the specific language governing permissions and
+# limitations under the License.
+""" SARI metric."""
+
+from collections import Counter
+
+import sacrebleu
+import sacremoses
+from packaging import version
+
+import datasets
+
+
+_CITATION = """\
+@inproceedings{xu-etal-2016-optimizing,
+title = {Optimizing Statistical Machine Translation for Text Simplification},
+authors={Xu, Wei and Napoles, Courtney and Pavlick, Ellie and Chen, Quanze and Callison-Burch, Chris},
+journal = {Transactions of the Association for Computational Linguistics},
+volume = {4},
+year={2016},
+url = {https://www.aclweb.org/anthology/Q16-1029},
+pages = {401--415},
+}
+"""
+
+_DESCRIPTION = """\
+SARI is a metric used for evaluating automatic text simplification systems.
+The metric compares the predicted simplified sentences against the reference
+and the source sentences. It explicitly measures the goodness of words that are
+added, deleted and kept by the system.
+Sari = (F1_add + F1_keep + P_del) / 3
+where
+F1_add: n-gram F1 score for add operation
+F1_keep: n-gram F1 score for keep operation
+P_del: n-gram precision score for delete operation
+n = 4, as in the original paper.
+
+This implementation is adapted from Tensorflow's tensor2tensor implementation [3].
+It has two differences with the original GitHub [1] implementation:
+ (1) Defines 0/0=1 instead of 0 to give higher scores for predictions that match
+ a target exactly.
+ (2) Fixes an alleged bug [2] in the keep score computation.
+[1] https://github.com/cocoxu/simplification/blob/master/SARI.py
+ (commit 0210f15)
+[2] https://github.com/cocoxu/simplification/issues/6
+[3] https://github.com/tensorflow/tensor2tensor/blob/master/tensor2tensor/utils/sari_hook.py
+"""
+
+
+_KWARGS_DESCRIPTION = """
+Calculates sari score (between 0 and 100) given a list of source and predicted
+sentences, and a list of lists of reference sentences.
+Args:
+ sources: list of source sentences where each sentence should be a string.
+ predictions: list of predicted sentences where each sentence should be a string.
+ references: list of lists of reference sentences where each sentence should be a string.
+Returns:
+ sari: sari score
+Examples:
+ >>> sources=["About 95 species are currently accepted ."]
+ >>> predictions=["About 95 you now get in ."]
+ >>> references=[["About 95 species are currently known .","About 95 species are now accepted .","95 species are now accepted ."]]
+ >>> sari = datasets.load_metric("sari")
+ >>> results = sari.compute(sources=sources, predictions=predictions, references=references)
+ >>> print(results)
+ {'sari': 26.953601953601954}
+"""
+
+
+def SARIngram(sgrams, cgrams, rgramslist, numref):
+ rgramsall = [rgram for rgrams in rgramslist for rgram in rgrams]
+ rgramcounter = Counter(rgramsall)
+
+ sgramcounter = Counter(sgrams)
+ sgramcounter_rep = Counter()
+ for sgram, scount in sgramcounter.items():
+ sgramcounter_rep[sgram] = scount * numref
+
+ cgramcounter = Counter(cgrams)
+ cgramcounter_rep = Counter()
+ for cgram, ccount in cgramcounter.items():
+ cgramcounter_rep[cgram] = ccount * numref
+
+ # KEEP
+ keepgramcounter_rep = sgramcounter_rep & cgramcounter_rep
+ keepgramcountergood_rep = keepgramcounter_rep & rgramcounter
+ keepgramcounterall_rep = sgramcounter_rep & rgramcounter
+
+ keeptmpscore1 = 0
+ keeptmpscore2 = 0
+ for keepgram in keepgramcountergood_rep:
+ keeptmpscore1 += keepgramcountergood_rep[keepgram] / keepgramcounter_rep[keepgram]
+ # Fix an alleged bug [2] in the keep score computation.
+ # keeptmpscore2 += keepgramcountergood_rep[keepgram] / keepgramcounterall_rep[keepgram]
+ keeptmpscore2 += keepgramcountergood_rep[keepgram]
+ # Define 0/0=1 instead of 0 to give higher scores for predictions that match
+ # a target exactly.
+ keepscore_precision = 1
+ keepscore_recall = 1
+ if len(keepgramcounter_rep) > 0:
+ keepscore_precision = keeptmpscore1 / len(keepgramcounter_rep)
+ if len(keepgramcounterall_rep) > 0:
+ # Fix an alleged bug [2] in the keep score computation.
+ # keepscore_recall = keeptmpscore2 / len(keepgramcounterall_rep)
+ keepscore_recall = keeptmpscore2 / sum(keepgramcounterall_rep.values())
+ keepscore = 0
+ if keepscore_precision > 0 or keepscore_recall > 0:
+ keepscore = 2 * keepscore_precision * keepscore_recall / (keepscore_precision + keepscore_recall)
+
+ # DELETION
+ delgramcounter_rep = sgramcounter_rep - cgramcounter_rep
+ delgramcountergood_rep = delgramcounter_rep - rgramcounter
+ delgramcounterall_rep = sgramcounter_rep - rgramcounter
+ deltmpscore1 = 0
+ deltmpscore2 = 0
+ for delgram in delgramcountergood_rep:
+ deltmpscore1 += delgramcountergood_rep[delgram] / delgramcounter_rep[delgram]
+ deltmpscore2 += delgramcountergood_rep[delgram] / delgramcounterall_rep[delgram]
+ # Define 0/0=1 instead of 0 to give higher scores for predictions that match
+ # a target exactly.
+ delscore_precision = 1
+ if len(delgramcounter_rep) > 0:
+ delscore_precision = deltmpscore1 / len(delgramcounter_rep)
+
+ # ADDITION
+ addgramcounter = set(cgramcounter) - set(sgramcounter)
+ addgramcountergood = set(addgramcounter) & set(rgramcounter)
+ addgramcounterall = set(rgramcounter) - set(sgramcounter)
+
+ addtmpscore = 0
+ for addgram in addgramcountergood:
+ addtmpscore += 1
+
+ # Define 0/0=1 instead of 0 to give higher scores for predictions that match
+ # a target exactly.
+ addscore_precision = 1
+ addscore_recall = 1
+ if len(addgramcounter) > 0:
+ addscore_precision = addtmpscore / len(addgramcounter)
+ if len(addgramcounterall) > 0:
+ addscore_recall = addtmpscore / len(addgramcounterall)
+ addscore = 0
+ if addscore_precision > 0 or addscore_recall > 0:
+ addscore = 2 * addscore_precision * addscore_recall / (addscore_precision + addscore_recall)
+
+ return (keepscore, delscore_precision, addscore)
+
+
+def SARIsent(ssent, csent, rsents):
+ numref = len(rsents)
+
+ s1grams = ssent.split(" ")
+ c1grams = csent.split(" ")
+ s2grams = []
+ c2grams = []
+ s3grams = []
+ c3grams = []
+ s4grams = []
+ c4grams = []
+
+ r1gramslist = []
+ r2gramslist = []
+ r3gramslist = []
+ r4gramslist = []
+ for rsent in rsents:
+ r1grams = rsent.split(" ")
+ r2grams = []
+ r3grams = []
+ r4grams = []
+ r1gramslist.append(r1grams)
+ for i in range(0, len(r1grams) - 1):
+ if i < len(r1grams) - 1:
+ r2gram = r1grams[i] + " " + r1grams[i + 1]
+ r2grams.append(r2gram)
+ if i < len(r1grams) - 2:
+ r3gram = r1grams[i] + " " + r1grams[i + 1] + " " + r1grams[i + 2]
+ r3grams.append(r3gram)
+ if i < len(r1grams) - 3:
+ r4gram = r1grams[i] + " " + r1grams[i + 1] + " " + r1grams[i + 2] + " " + r1grams[i + 3]
+ r4grams.append(r4gram)
+ r2gramslist.append(r2grams)
+ r3gramslist.append(r3grams)
+ r4gramslist.append(r4grams)
+
+ for i in range(0, len(s1grams) - 1):
+ if i < len(s1grams) - 1:
+ s2gram = s1grams[i] + " " + s1grams[i + 1]
+ s2grams.append(s2gram)
+ if i < len(s1grams) - 2:
+ s3gram = s1grams[i] + " " + s1grams[i + 1] + " " + s1grams[i + 2]
+ s3grams.append(s3gram)
+ if i < len(s1grams) - 3:
+ s4gram = s1grams[i] + " " + s1grams[i + 1] + " " + s1grams[i + 2] + " " + s1grams[i + 3]
+ s4grams.append(s4gram)
+
+ for i in range(0, len(c1grams) - 1):
+ if i < len(c1grams) - 1:
+ c2gram = c1grams[i] + " " + c1grams[i + 1]
+ c2grams.append(c2gram)
+ if i < len(c1grams) - 2:
+ c3gram = c1grams[i] + " " + c1grams[i + 1] + " " + c1grams[i + 2]
+ c3grams.append(c3gram)
+ if i < len(c1grams) - 3:
+ c4gram = c1grams[i] + " " + c1grams[i + 1] + " " + c1grams[i + 2] + " " + c1grams[i + 3]
+ c4grams.append(c4gram)
+
+ (keep1score, del1score, add1score) = SARIngram(s1grams, c1grams, r1gramslist, numref)
+ (keep2score, del2score, add2score) = SARIngram(s2grams, c2grams, r2gramslist, numref)
+ (keep3score, del3score, add3score) = SARIngram(s3grams, c3grams, r3gramslist, numref)
+ (keep4score, del4score, add4score) = SARIngram(s4grams, c4grams, r4gramslist, numref)
+ avgkeepscore = sum([keep1score, keep2score, keep3score, keep4score]) / 4
+ avgdelscore = sum([del1score, del2score, del3score, del4score]) / 4
+ avgaddscore = sum([add1score, add2score, add3score, add4score]) / 4
+ finalscore = (avgkeepscore + avgdelscore + avgaddscore) / 3
+ return finalscore
+
+
+def normalize(sentence, lowercase: bool = True, tokenizer: str = "13a", return_str: bool = True):
+
+ # Normalization is requried for the ASSET dataset (one of the primary
+ # datasets in sentence simplification) to allow using space
+ # to split the sentence. Even though Wiki-Auto and TURK datasets,
+ # do not require normalization, we do it for consistency.
+ # Code adapted from the EASSE library [1] written by the authors of the ASSET dataset.
+ # [1] https://github.com/feralvam/easse/blob/580bba7e1378fc8289c663f864e0487188fe8067/easse/utils/preprocessing.py#L7
+
+ if lowercase:
+ sentence = sentence.lower()
+
+ if tokenizer in ["13a", "intl"]:
+ if version.parse(sacrebleu.__version__).major >= 2:
+ normalized_sent = sacrebleu.metrics.bleu._get_tokenizer(tokenizer)()(sentence)
+ else:
+ normalized_sent = sacrebleu.TOKENIZERS[tokenizer]()(sentence)
+ elif tokenizer == "moses":
+ normalized_sent = sacremoses.MosesTokenizer().tokenize(sentence, return_str=True, escape=False)
+ elif tokenizer == "penn":
+ normalized_sent = sacremoses.MosesTokenizer().penn_tokenize(sentence, return_str=True)
+ else:
+ normalized_sent = sentence
+
+ if not return_str:
+ normalized_sent = normalized_sent.split()
+
+ return normalized_sent
+
+
+@datasets.utils.file_utils.add_start_docstrings(_DESCRIPTION, _KWARGS_DESCRIPTION)
+class Sari(datasets.Metric):
+ def _info(self):
+ return datasets.MetricInfo(
+ description=_DESCRIPTION,
+ citation=_CITATION,
+ inputs_description=_KWARGS_DESCRIPTION,
+ features=datasets.Features(
+ {
+ "sources": datasets.Value("string", id="sequence"),
+ "predictions": datasets.Value("string", id="sequence"),
+ "references": datasets.Sequence(datasets.Value("string", id="sequence"), id="references"),
+ }
+ ),
+ codebase_urls=[
+ "https://github.com/cocoxu/simplification/blob/master/SARI.py",
+ "https://github.com/tensorflow/tensor2tensor/blob/master/tensor2tensor/utils/sari_hook.py",
+ ],
+ reference_urls=["https://www.aclweb.org/anthology/Q16-1029.pdf"],
+ )
+
+ def _compute(self, sources, predictions, references):
+
+ if not (len(sources) == len(predictions) == len(references)):
+ raise ValueError("Sources length must match predictions and references lengths.")
+ sari_score = 0
+ for src, pred, refs in zip(sources, predictions, references):
+ sari_score += SARIsent(normalize(src), normalize(pred), [normalize(sent) for sent in refs])
+ sari_score = sari_score / len(predictions)
+ return {"sari": 100 * sari_score}
diff --git a/metrics/seqeval/README.md b/metrics/seqeval/README.md
new file mode 100644
index 0000000..0002682
--- /dev/null
+++ b/metrics/seqeval/README.md
@@ -0,0 +1,139 @@
+# Metric Card for seqeval
+
+## Metric description
+
+seqeval is a Python framework for sequence labeling evaluation. seqeval can evaluate the performance of chunking tasks such as named-entity recognition, part-of-speech tagging, semantic role labeling and so on.
+
+
+## How to use
+
+Seqeval produces labelling scores along with its sufficient statistics from a source against one or more references.
+
+It takes two mandatory arguments:
+
+`predictions`: a list of lists of predicted labels, i.e. estimated targets as returned by a tagger.
+
+`references`: a list of lists of reference labels, i.e. the ground truth/target values.
+
+It can also take several optional arguments:
+
+`suffix` (boolean): `True` if the IOB tag is a suffix (after type) instead of a prefix (before type), `False` otherwise. The default value is `False`, i.e. the IOB tag is a prefix (before type).
+
+`scheme`: the target tagging scheme, which can be one of [`IOB1`, `IOB2`, `IOE1`, `IOE2`, `IOBES`, `BILOU`]. The default value is `None`.
+
+`mode`: whether to count correct entity labels with incorrect I/B tags as true positives or not. If you want to only count exact matches, pass `mode="strict"` and a specific `scheme` value. The default is `None`.
+
+`sample_weight`: An array-like of shape (n_samples,) that provides weights for individual samples. The default is `None`.
+
+`zero_division`: Which value to substitute as a metric value when encountering zero division. Should be one of [`0`,`1`,`"warn"`]. `"warn"` acts as `0`, but the warning is raised.
+
+
+```python
+>>> from datasets import load_metric
+>>> seqeval = load_metric('seqeval')
+>>> predictions = [['O', 'O', 'B-MISC', 'I-MISC', 'I-MISC', 'I-MISC', 'O'], ['B-PER', 'I-PER', 'O']]
+>>> references = [['O', 'O', 'O', 'B-MISC', 'I-MISC', 'I-MISC', 'O'], ['B-PER', 'I-PER', 'O']]
+>>> results = seqeval.compute(predictions=predictions, references=references)
+```
+
+## Output values
+
+This metric returns a dictionary with a summary of scores for overall and per type:
+
+Overall:
+
+`accuracy`: the average [accuracy](https://huggingface.co/metrics/accuracy), on a scale between 0.0 and 1.0.
+
+`precision`: the average [precision](https://huggingface.co/metrics/precision), on a scale between 0.0 and 1.0.
+
+`recall`: the average [recall](https://huggingface.co/metrics/recall), on a scale between 0.0 and 1.0.
+
+`f1`: the average [F1 score](https://huggingface.co/metrics/f1), which is the harmonic mean of the precision and recall. It also has a scale of 0.0 to 1.0.
+
+Per type (e.g. `MISC`, `PER`, `LOC`,...):
+
+`precision`: the average [precision](https://huggingface.co/metrics/precision), on a scale between 0.0 and 1.0.
+
+`recall`: the average [recall](https://huggingface.co/metrics/recall), on a scale between 0.0 and 1.0.
+
+`f1`: the average [F1 score](https://huggingface.co/metrics/f1), on a scale between 0.0 and 1.0.
+
+
+### Values from popular papers
+The 1995 "Text Chunking using Transformation-Based Learning" [paper](https://aclanthology.org/W95-0107) reported a baseline recall of 81.9% and a precision of 78.2% using non Deep Learning-based methods.
+
+More recently, seqeval continues being used for reporting performance on tasks such as [named entity detection](https://www.mdpi.com/2306-5729/6/8/84/htm) and [information extraction](https://ieeexplore.ieee.org/abstract/document/9697942/).
+
+
+## Examples
+
+Maximal values (full match) :
+
+```python
+>>> from datasets import load_metric
+>>> seqeval = load_metric('seqeval')
+>>> predictions = [['O', 'O', 'B-MISC', 'I-MISC', 'I-MISC', 'I-MISC', 'O'], ['B-PER', 'I-PER', 'O']]
+>>> references = [['O', 'O', 'B-MISC', 'I-MISC', 'I-MISC', 'I-MISC', 'O'], ['B-PER', 'I-PER', 'O']]
+>>> results = seqeval.compute(predictions=predictions, references=references)
+>>> print(results)
+{'MISC': {'precision': 1.0, 'recall': 1.0, 'f1': 1.0, 'number': 1}, 'PER': {'precision': 1.0, 'recall': 1.0, 'f1': 1.0, 'number': 1}, 'overall_precision': 1.0, 'overall_recall': 1.0, 'overall_f1': 1.0, 'overall_accuracy': 1.0}
+
+```
+
+Minimal values (no match):
+
+```python
+>>> from datasets import load_metric
+>>> seqeval = load_metric('seqeval')
+>>> predictions = [['O', 'B-MISC', 'I-MISC'], ['B-PER', 'I-PER', 'O']]
+>>> references = [['B-MISC', 'O', 'O'], ['I-PER', '0', 'I-PER']]
+>>> results = seqeval.compute(predictions=predictions, references=references)
+>>> print(results)
+{'MISC': {'precision': 0.0, 'recall': 0.0, 'f1': 0.0, 'number': 1}, 'PER': {'precision': 0.0, 'recall': 0.0, 'f1': 0.0, 'number': 2}, '_': {'precision': 0.0, 'recall': 0.0, 'f1': 0.0, 'number': 1}, 'overall_precision': 0.0, 'overall_recall': 0.0, 'overall_f1': 0.0, 'overall_accuracy': 0.0}
+```
+
+Partial match:
+
+```python
+>>> from datasets import load_metric
+>>> seqeval = load_metric('seqeval')
+>>> predictions = [['O', 'O', 'B-MISC', 'I-MISC', 'I-MISC', 'I-MISC', 'O'], ['B-PER', 'I-PER', 'O']]
+>>> references = [['O', 'O', 'O', 'B-MISC', 'I-MISC', 'I-MISC', 'O'], ['B-PER', 'I-PER', 'O']]
+>>> results = seqeval.compute(predictions=predictions, references=references)
+>>> print(results)
+{'MISC': {'precision': 0.0, 'recall': 0.0, 'f1': 0.0, 'number': 1}, 'PER': {'precision': 1.0, 'recall': 1.0, 'f1': 1.0, 'number': 1}, 'overall_precision': 0.5, 'overall_recall': 0.5, 'overall_f1': 0.5, 'overall_accuracy': 0.8}
+```
+
+## Limitations and bias
+
+seqeval supports following IOB formats (short for inside, outside, beginning) : `IOB1`, `IOB2`, `IOE1`, `IOE2`, `IOBES`, `IOBES` (only in strict mode) and `BILOU` (only in strict mode).
+
+For more information about IOB formats, refer to the [Wikipedia page](https://en.wikipedia.org/wiki/Inside%E2%80%93outside%E2%80%93beginning_(tagging)) and the description of the [CoNLL-2000 shared task](https://aclanthology.org/W02-2024).
+
+
+## Citation
+
+```bibtex
+@inproceedings{ramshaw-marcus-1995-text,
+ title = "Text Chunking using Transformation-Based Learning",
+ author = "Ramshaw, Lance and
+ Marcus, Mitch",
+ booktitle = "Third Workshop on Very Large Corpora",
+ year = "1995",
+ url = "https://www.aclweb.org/anthology/W95-0107",
+}
+```
+
+```bibtex
+@misc{seqeval,
+ title={{seqeval}: A Python framework for sequence labeling evaluation},
+ url={https://github.com/chakki-works/seqeval},
+ note={Software available from https://github.com/chakki-works/seqeval},
+ author={Hiroki Nakayama},
+ year={2018},
+}
+```
+
+## Further References
+- [README for seqeval at GitHub](https://github.com/chakki-works/seqeval)
+- [CoNLL-2000 shared task](https://www.clips.uantwerpen.be/conll2002/ner/bin/conlleval.txt)
diff --git a/metrics/seqeval/seqeval.py b/metrics/seqeval/seqeval.py
new file mode 100644
index 0000000..d572b08
--- /dev/null
+++ b/metrics/seqeval/seqeval.py
@@ -0,0 +1,163 @@
+# Copyright 2020 The HuggingFace Datasets Authors.
+#
+# Licensed under the Apache License, Version 2.0 (the "License");
+# you may not use this file except in compliance with the License.
+# You may obtain a copy of the License at
+#
+# http://www.apache.org/licenses/LICENSE-2.0
+#
+# Unless required by applicable law or agreed to in writing, software
+# distributed under the License is distributed on an "AS IS" BASIS,
+# WITHOUT WARRANTIES OR CONDITIONS OF ANY KIND, either express or implied.
+# See the License for the specific language governing permissions and
+# limitations under the License.
+""" seqeval metric. """
+
+import importlib
+from typing import List, Optional, Union
+
+from seqeval.metrics import accuracy_score, classification_report
+
+import datasets
+
+
+_CITATION = """\
+@inproceedings{ramshaw-marcus-1995-text,
+ title = "Text Chunking using Transformation-Based Learning",
+ author = "Ramshaw, Lance and
+ Marcus, Mitch",
+ booktitle = "Third Workshop on Very Large Corpora",
+ year = "1995",
+ url = "https://www.aclweb.org/anthology/W95-0107",
+}
+@misc{seqeval,
+ title={{seqeval}: A Python framework for sequence labeling evaluation},
+ url={https://github.com/chakki-works/seqeval},
+ note={Software available from https://github.com/chakki-works/seqeval},
+ author={Hiroki Nakayama},
+ year={2018},
+}
+"""
+
+_DESCRIPTION = """\
+seqeval is a Python framework for sequence labeling evaluation.
+seqeval can evaluate the performance of chunking tasks such as named-entity recognition, part-of-speech tagging, semantic role labeling and so on.
+
+This is well-tested by using the Perl script conlleval, which can be used for
+measuring the performance of a system that has processed the CoNLL-2000 shared task data.
+
+seqeval supports following formats:
+IOB1
+IOB2
+IOE1
+IOE2
+IOBES
+
+See the [README.md] file at https://github.com/chakki-works/seqeval for more information.
+"""
+
+_KWARGS_DESCRIPTION = """
+Produces labelling scores along with its sufficient statistics
+from a source against one or more references.
+
+Args:
+ predictions: List of List of predicted labels (Estimated targets as returned by a tagger)
+ references: List of List of reference labels (Ground truth (correct) target values)
+ suffix: True if the IOB prefix is after type, False otherwise. default: False
+ scheme: Specify target tagging scheme. Should be one of ["IOB1", "IOB2", "IOE1", "IOE2", "IOBES", "BILOU"].
+ default: None
+ mode: Whether to count correct entity labels with incorrect I/B tags as true positives or not.
+ If you want to only count exact matches, pass mode="strict". default: None.
+ sample_weight: Array-like of shape (n_samples,), weights for individual samples. default: None
+ zero_division: Which value to substitute as a metric value when encountering zero division. Should be on of 0, 1,
+ "warn". "warn" acts as 0, but the warning is raised.
+
+Returns:
+ 'scores': dict. Summary of the scores for overall and per type
+ Overall:
+ 'accuracy': accuracy,
+ 'precision': precision,
+ 'recall': recall,
+ 'f1': F1 score, also known as balanced F-score or F-measure,
+ Per type:
+ 'precision': precision,
+ 'recall': recall,
+ 'f1': F1 score, also known as balanced F-score or F-measure
+Examples:
+
+ >>> predictions = [['O', 'O', 'B-MISC', 'I-MISC', 'I-MISC', 'I-MISC', 'O'], ['B-PER', 'I-PER', 'O']]
+ >>> references = [['O', 'O', 'O', 'B-MISC', 'I-MISC', 'I-MISC', 'O'], ['B-PER', 'I-PER', 'O']]
+ >>> seqeval = datasets.load_metric("seqeval")
+ >>> results = seqeval.compute(predictions=predictions, references=references)
+ >>> print(list(results.keys()))
+ ['MISC', 'PER', 'overall_precision', 'overall_recall', 'overall_f1', 'overall_accuracy']
+ >>> print(results["overall_f1"])
+ 0.5
+ >>> print(results["PER"]["f1"])
+ 1.0
+"""
+
+
+@datasets.utils.file_utils.add_start_docstrings(_DESCRIPTION, _KWARGS_DESCRIPTION)
+class Seqeval(datasets.Metric):
+ def _info(self):
+ return datasets.MetricInfo(
+ description=_DESCRIPTION,
+ citation=_CITATION,
+ homepage="https://github.com/chakki-works/seqeval",
+ inputs_description=_KWARGS_DESCRIPTION,
+ features=datasets.Features(
+ {
+ "predictions": datasets.Sequence(datasets.Value("string", id="label"), id="sequence"),
+ "references": datasets.Sequence(datasets.Value("string", id="label"), id="sequence"),
+ }
+ ),
+ codebase_urls=["https://github.com/chakki-works/seqeval"],
+ reference_urls=["https://github.com/chakki-works/seqeval"],
+ )
+
+ def _compute(
+ self,
+ predictions,
+ references,
+ suffix: bool = False,
+ scheme: Optional[str] = None,
+ mode: Optional[str] = None,
+ sample_weight: Optional[List[int]] = None,
+ zero_division: Union[str, int] = "warn",
+ ):
+ if scheme is not None:
+ try:
+ scheme_module = importlib.import_module("seqeval.scheme")
+ scheme = getattr(scheme_module, scheme)
+ except AttributeError:
+ raise ValueError(f"Scheme should be one of [IOB1, IOB2, IOE1, IOE2, IOBES, BILOU], got {scheme}")
+ report = classification_report(
+ y_true=references,
+ y_pred=predictions,
+ suffix=suffix,
+ output_dict=True,
+ scheme=scheme,
+ mode=mode,
+ sample_weight=sample_weight,
+ zero_division=zero_division,
+ )
+ report.pop("macro avg")
+ report.pop("weighted avg")
+ overall_score = report.pop("micro avg")
+
+ scores = {
+ type_name: {
+ "precision": score["precision"],
+ "recall": score["recall"],
+ "f1": score["f1-score"],
+ "number": score["support"],
+ }
+ for type_name, score in report.items()
+ }
+ scores["overall_precision"] = overall_score["precision"]
+ scores["overall_recall"] = overall_score["recall"]
+ scores["overall_f1"] = overall_score["f1-score"]
+ scores["overall_accuracy"] = accuracy_score(y_true=references, y_pred=predictions)
+
+ return scores
diff --git a/metrics/spearmanr/README.md b/metrics/spearmanr/README.md
new file mode 100644
index 0000000..007fb5d
--- /dev/null
+++ b/metrics/spearmanr/README.md
@@ -0,0 +1,117 @@
+# Metric Card for Spearman Correlation Coefficient Metric (spearmanr)
+
+
+## Metric Description
+The Spearman rank-order correlation coefficient is a measure of the
+relationship between two datasets. Like other correlation coefficients,
+this one varies between -1 and +1 with 0 implying no correlation.
+Positive correlations imply that as data in dataset x increases, so
+does data in dataset y. Negative correlations imply that as x increases,
+y decreases. Correlations of -1 or +1 imply an exact monotonic relationship.
+
+Unlike the Pearson correlation, the Spearman correlation does not
+assume that both datasets are normally distributed.
+
+The p-value roughly indicates the probability of an uncorrelated system
+producing datasets that have a Spearman correlation at least as extreme
+as the one computed from these datasets. The p-values are not entirely
+reliable but are probably reasonable for datasets larger than 500 or so.
+
+
+## How to Use
+At minimum, this metric only requires a `list` of predictions and a `list` of references:
+
+```python
+>>> spearmanr_metric = datasets.load_metric("spearmanr")
+>>> results = spearmanr_metric.compute(references=[1, 2, 3, 4, 5], predictions=[10, 9, 2.5, 6, 4])
+>>> print(results)
+{'spearmanr': -0.7}
+```
+
+### Inputs
+- **`predictions`** (`list` of `float`): Predicted labels, as returned by a model.
+- **`references`** (`list` of `float`): Ground truth labels.
+- **`return_pvalue`** (`bool`): If `True`, returns the p-value. If `False`, returns
+ only the spearmanr score. Defaults to `False`.
+
+### Output Values
+- **`spearmanr`** (`float`): Spearman correlation coefficient.
+- **`p-value`** (`float`): p-value. **Note**: is only returned
+ if `return_pvalue=True` is input.
+
+If `return_pvalue=False`, the output is a `dict` with one value, as below:
+```python
+{'spearmanr': -0.7}
+```
+
+Otherwise, if `return_pvalue=True`, the output is a `dict` containing a the `spearmanr` value as well as the corresponding `pvalue`:
+```python
+{'spearmanr': -0.7, 'spearmanr_pvalue': 0.1881204043741873}
+```
+
+Spearman rank-order correlations can take on any value from `-1` to `1`, inclusive.
+
+The p-values can take on any value from `0` to `1`, inclusive.
+
+#### Values from Popular Papers
+
+
+### Examples
+A basic example:
+```python
+>>> spearmanr_metric = datasets.load_metric("spearmanr")
+>>> results = spearmanr_metric.compute(references=[1, 2, 3, 4, 5], predictions=[10, 9, 2.5, 6, 4])
+>>> print(results)
+{'spearmanr': -0.7}
+```
+
+The same example, but that also returns the pvalue:
+```python
+>>> spearmanr_metric = datasets.load_metric("spearmanr")
+>>> results = spearmanr_metric.compute(references=[1, 2, 3, 4, 5], predictions=[10, 9, 2.5, 6, 4], return_pvalue=True)
+>>> print(results)
+{'spearmanr': -0.7, 'spearmanr_pvalue': 0.1881204043741873
+>>> print(results['spearmanr'])
+-0.7
+>>> print(results['spearmanr_pvalue'])
+0.1881204043741873
+```
+
+## Limitations and Bias
+
+
+## Citation
+```bibtex
+@book{kokoska2000crc,
+ title={CRC standard probability and statistics tables and formulae},
+ author={Kokoska, Stephen and Zwillinger, Daniel},
+ year={2000},
+ publisher={Crc Press}
+}
+@article{2020SciPy-NMeth,
+ author = {Virtanen, Pauli and Gommers, Ralf and Oliphant, Travis E. and
+ Haberland, Matt and Reddy, Tyler and Cournapeau, David and
+ Burovski, Evgeni and Peterson, Pearu and Weckesser, Warren and
+ Bright, Jonathan and {van der Walt}, St{\'e}fan J. and
+ Brett, Matthew and Wilson, Joshua and Millman, K. Jarrod and
+ Mayorov, Nikolay and Nelson, Andrew R. J. and Jones, Eric and
+ Kern, Robert and Larson, Eric and Carey, C J and
+ Polat, {\.I}lhan and Feng, Yu and Moore, Eric W. and
+ {VanderPlas}, Jake and Laxalde, Denis and Perktold, Josef and
+ Cimrman, Robert and Henriksen, Ian and Quintero, E. A. and
+ Harris, Charles R. and Archibald, Anne M. and
+ Ribeiro, Ant{\^o}nio H. and Pedregosa, Fabian and
+ {van Mulbregt}, Paul and {SciPy 1.0 Contributors}},
+ title = {{{SciPy} 1.0: Fundamental Algorithms for Scientific
+ Computing in Python}},
+ journal = {Nature Methods},
+ year = {2020},
+ volume = {17},
+ pages = {261--272},
+ adsurl = {https://rdcu.be/b08Wh},
+ doi = {10.1038/s41592-019-0686-2},
+}
+```
+
+## Further References
+*Add any useful further references.*
diff --git a/metrics/spearmanr/spearmanr.py b/metrics/spearmanr/spearmanr.py
new file mode 100644
index 0000000..bc5d415
--- /dev/null
+++ b/metrics/spearmanr/spearmanr.py
@@ -0,0 +1,119 @@
+# Copyright 2021 The HuggingFace Datasets Authors and the current dataset script contributor.
+#
+# Licensed under the Apache License, Version 2.0 (the "License");
+# you may not use this file except in compliance with the License.
+# You may obtain a copy of the License at
+#
+# http://www.apache.org/licenses/LICENSE-2.0
+#
+# Unless required by applicable law or agreed to in writing, software
+# distributed under the License is distributed on an "AS IS" BASIS,
+# WITHOUT WARRANTIES OR CONDITIONS OF ANY KIND, either express or implied.
+# See the License for the specific language governing permissions and
+# limitations under the License.
+"""Spearman correlation coefficient metric."""
+
+from scipy.stats import spearmanr
+
+import datasets
+
+
+_DESCRIPTION = """
+The Spearman rank-order correlation coefficient is a measure of the
+relationship between two datasets. Like other correlation coefficients,
+this one varies between -1 and +1 with 0 implying no correlation.
+Positive correlations imply that as data in dataset x increases, so
+does data in dataset y. Negative correlations imply that as x increases,
+y decreases. Correlations of -1 or +1 imply an exact monotonic relationship.
+
+Unlike the Pearson correlation, the Spearman correlation does not
+assume that both datasets are normally distributed.
+
+The p-value roughly indicates the probability of an uncorrelated system
+producing datasets that have a Spearman correlation at least as extreme
+as the one computed from these datasets. The p-values are not entirely
+reliable but are probably reasonable for datasets larger than 500 or so.
+"""
+
+_KWARGS_DESCRIPTION = """
+Args:
+ predictions (`List[float]`): Predicted labels, as returned by a model.
+ references (`List[float]`): Ground truth labels.
+ return_pvalue (`bool`): If `True`, returns the p-value. If `False`, returns
+ only the spearmanr score. Defaults to `False`.
+Returns:
+ spearmanr (`float`): Spearman correlation coefficient.
+ p-value (`float`): p-value. **Note**: is only returned if `return_pvalue=True` is input.
+Examples:
+ Example 1:
+ >>> spearmanr_metric = datasets.load_metric("spearmanr")
+ >>> results = spearmanr_metric.compute(references=[1, 2, 3, 4, 5], predictions=[10, 9, 2.5, 6, 4])
+ >>> print(results)
+ {'spearmanr': -0.7}
+
+ Example 2:
+ >>> spearmanr_metric = datasets.load_metric("spearmanr")
+ >>> results = spearmanr_metric.compute(references=[1, 2, 3, 4, 5],
+ ... predictions=[10, 9, 2.5, 6, 4],
+ ... return_pvalue=True)
+ >>> print(results['spearmanr'])
+ -0.7
+ >>> print(round(results['spearmanr_pvalue'], 2))
+ 0.19
+"""
+
+_CITATION = r"""\
+@book{kokoska2000crc,
+ title={CRC standard probability and statistics tables and formulae},
+ author={Kokoska, Stephen and Zwillinger, Daniel},
+ year={2000},
+ publisher={Crc Press}
+}
+@article{2020SciPy-NMeth,
+ author = {Virtanen, Pauli and Gommers, Ralf and Oliphant, Travis E. and
+ Haberland, Matt and Reddy, Tyler and Cournapeau, David and
+ Burovski, Evgeni and Peterson, Pearu and Weckesser, Warren and
+ Bright, Jonathan and {van der Walt}, St{\'e}fan J. and
+ Brett, Matthew and Wilson, Joshua and Millman, K. Jarrod and
+ Mayorov, Nikolay and Nelson, Andrew R. J. and Jones, Eric and
+ Kern, Robert and Larson, Eric and Carey, C J and
+ Polat, {\.I}lhan and Feng, Yu and Moore, Eric W. and
+ {VanderPlas}, Jake and Laxalde, Denis and Perktold, Josef and
+ Cimrman, Robert and Henriksen, Ian and Quintero, E. A. and
+ Harris, Charles R. and Archibald, Anne M. and
+ Ribeiro, Ant{\^o}nio H. and Pedregosa, Fabian and
+ {van Mulbregt}, Paul and {SciPy 1.0 Contributors}},
+ title = {{{SciPy} 1.0: Fundamental Algorithms for Scientific
+ Computing in Python}},
+ journal = {Nature Methods},
+ year = {2020},
+ volume = {17},
+ pages = {261--272},
+ adsurl = {https://rdcu.be/b08Wh},
+ doi = {10.1038/s41592-019-0686-2},
+}
+"""
+
+
+@datasets.utils.file_utils.add_start_docstrings(_DESCRIPTION, _KWARGS_DESCRIPTION)
+class Spearmanr(datasets.Metric):
+ def _info(self):
+ return datasets.MetricInfo(
+ description=_DESCRIPTION,
+ citation=_CITATION,
+ inputs_description=_KWARGS_DESCRIPTION,
+ features=datasets.Features(
+ {
+ "predictions": datasets.Value("float"),
+ "references": datasets.Value("float"),
+ }
+ ),
+ reference_urls=["https://docs.scipy.org/doc/scipy/reference/generated/scipy.stats.spearmanr.html"],
+ )
+
+ def _compute(self, predictions, references, return_pvalue=False):
+ results = spearmanr(references, predictions)
+ if return_pvalue:
+ return {"spearmanr": results[0], "spearmanr_pvalue": results[1]}
+ else:
+ return {"spearmanr": results[0]}
diff --git a/metrics/squad/README.md b/metrics/squad/README.md
new file mode 100644
index 0000000..2d5a5d7
--- /dev/null
+++ b/metrics/squad/README.md
@@ -0,0 +1,90 @@
+# Metric Card for SQuAD
+
+## Metric description
+This metric wraps the official scoring script for version 1 of the [Stanford Question Answering Dataset (SQuAD)](https://huggingface.co/datasets/squad).
+
+SQuAD is a reading comprehension dataset, consisting of questions posed by crowdworkers on a set of Wikipedia articles, where the answer to every question is a segment of text, or span, from the corresponding reading passage, or the question might be unanswerable.
+
+## How to use
+
+The metric takes two files or two lists of question-answers dictionaries as inputs : one with the predictions of the model and the other with the references to be compared to:
+
+```python
+from datasets import load_metric
+squad_metric = load_metric("squad")
+results = squad_metric.compute(predictions=predictions, references=references)
+```
+## Output values
+
+This metric outputs a dictionary with two values: the average exact match score and the average [F1 score](https://huggingface.co/metrics/f1).
+
+```
+{'exact_match': 100.0, 'f1': 100.0}
+```
+
+The range of `exact_match` is 0-100, where 0.0 means no answers were matched and 100.0 means all answers were matched.
+
+The range of `f1` is 0-1 -- its lowest possible value is 0, if either the precision or the recall is 0, and its highest possible value is 1.0, which means perfect precision and recall.
+
+### Values from popular papers
+The [original SQuAD paper](https://nlp.stanford.edu/pubs/rajpurkar2016squad.pdf) reported an F1 score of 51.0% and an Exact Match score of 40.0%. They also report that human performance on the dataset represents an F1 score of 90.5% and an Exact Match score of 80.3%.
+
+For more recent model performance, see the [dataset leaderboard](https://paperswithcode.com/dataset/squad).
+
+## Examples
+
+Maximal values for both exact match and F1 (perfect match):
+
+```python
+from datasets import load_metric
+squad_metric = load_metric("squad")
+predictions = [{'prediction_text': '1976', 'id': '56e10a3be3433e1400422b22'}]
+references = [{'answers': {'answer_start': [97], 'text': ['1976']}, 'id': '56e10a3be3433e1400422b22'}]
+results = squad_metric.compute(predictions=predictions, references=references)
+results
+{'exact_match': 100.0, 'f1': 100.0}
+```
+
+Minimal values for both exact match and F1 (no match):
+
+```python
+from datasets import load_metric
+squad_metric = load_metric("squad")
+predictions = [{'prediction_text': '1999', 'id': '56e10a3be3433e1400422b22'}]
+references = [{'answers': {'answer_start': [97], 'text': ['1976']}, 'id': '56e10a3be3433e1400422b22'}]
+results = squad_metric.compute(predictions=predictions, references=references)
+results
+{'exact_match': 0.0, 'f1': 0.0}
+```
+
+Partial match (2 out of 3 answers correct) :
+
+```python
+from datasets import load_metric
+squad_metric = load_metric("squad")
+predictions = [{'prediction_text': '1976', 'id': '56e10a3be3433e1400422b22'}, {'prediction_text': 'Beyonce', 'id': '56d2051ce7d4791d0090260b'}, {'prediction_text': 'climate change', 'id': '5733b5344776f419006610e1'}]
+references = [{'answers': {'answer_start': [97], 'text': ['1976']}, 'id': '56e10a3be3433e1400422b22'}, {'answers': {'answer_start': [233], 'text': ['Beyoncé and Bruno Mars']}, 'id': '56d2051ce7d4791d0090260b'}, {'answers': {'answer_start': [891], 'text': ['climate change']}, 'id': '5733b5344776f419006610e1'}]
+results = squad_metric.compute(predictions=predictions, references=references)
+results
+{'exact_match': 66.66666666666667, 'f1': 66.66666666666667}
+```
+
+## Limitations and bias
+This metric works only with datasets that have the same format as [SQuAD v.1 dataset](https://huggingface.co/datasets/squad).
+
+The SQuAD dataset does contain a certain amount of noise, such as duplicate questions as well as missing answers, but these represent a minority of the 100,000 question-answer pairs. Also, neither exact match nor F1 score reflect whether models do better on certain types of questions (e.g. who questions) or those that cover a certain gender or geographical area -- carrying out more in-depth error analysis can complement these numbers.
+
+
+## Citation
+
+ @inproceedings{Rajpurkar2016SQuAD10,
+ title={SQuAD: 100, 000+ Questions for Machine Comprehension of Text},
+ author={Pranav Rajpurkar and Jian Zhang and Konstantin Lopyrev and Percy Liang},
+ booktitle={EMNLP},
+ year={2016}
+ }
+
+## Further References
+
+- [The Stanford Question Answering Dataset: Background, Challenges, Progress (blog post)](https://rajpurkar.github.io/mlx/qa-and-squad/)
+- [Hugging Face Course -- Question Answering](https://huggingface.co/course/chapter7/7)
diff --git a/metrics/squad/evaluate.py b/metrics/squad/evaluate.py
new file mode 100644
index 0000000..41effa7
--- /dev/null
+++ b/metrics/squad/evaluate.py
@@ -0,0 +1,92 @@
+""" Official evaluation script for v1.1 of the SQuAD dataset. """
+
+import argparse
+import json
+import re
+import string
+import sys
+from collections import Counter
+
+
+def normalize_answer(s):
+ """Lower text and remove punctuation, articles and extra whitespace."""
+
+ def remove_articles(text):
+ return re.sub(r"\b(a|an|the)\b", " ", text)
+
+ def white_space_fix(text):
+ return " ".join(text.split())
+
+ def remove_punc(text):
+ exclude = set(string.punctuation)
+ return "".join(ch for ch in text if ch not in exclude)
+
+ def lower(text):
+ return text.lower()
+
+ return white_space_fix(remove_articles(remove_punc(lower(s))))
+
+
+def f1_score(prediction, ground_truth):
+ prediction_tokens = normalize_answer(prediction).split()
+ ground_truth_tokens = normalize_answer(ground_truth).split()
+ common = Counter(prediction_tokens) & Counter(ground_truth_tokens)
+ num_same = sum(common.values())
+ if num_same == 0:
+ return 0
+ precision = 1.0 * num_same / len(prediction_tokens)
+ recall = 1.0 * num_same / len(ground_truth_tokens)
+ f1 = (2 * precision * recall) / (precision + recall)
+ return f1
+
+
+def exact_match_score(prediction, ground_truth):
+ return normalize_answer(prediction) == normalize_answer(ground_truth)
+
+
+def metric_max_over_ground_truths(metric_fn, prediction, ground_truths):
+ scores_for_ground_truths = []
+ for ground_truth in ground_truths:
+ score = metric_fn(prediction, ground_truth)
+ scores_for_ground_truths.append(score)
+ return max(scores_for_ground_truths)
+
+
+def evaluate(dataset, predictions):
+ f1 = exact_match = total = 0
+ for article in dataset:
+ for paragraph in article["paragraphs"]:
+ for qa in paragraph["qas"]:
+ total += 1
+ if qa["id"] not in predictions:
+ message = "Unanswered question " + qa["id"] + " will receive score 0."
+ print(message, file=sys.stderr)
+ continue
+ ground_truths = list(map(lambda x: x["text"], qa["answers"]))
+ prediction = predictions[qa["id"]]
+ exact_match += metric_max_over_ground_truths(exact_match_score, prediction, ground_truths)
+ f1 += metric_max_over_ground_truths(f1_score, prediction, ground_truths)
+
+ exact_match = 100.0 * exact_match / total
+ f1 = 100.0 * f1 / total
+
+ return {"exact_match": exact_match, "f1": f1}
+
+
+if __name__ == "__main__":
+ expected_version = "1.1"
+ parser = argparse.ArgumentParser(description="Evaluation for SQuAD " + expected_version)
+ parser.add_argument("dataset_file", help="Dataset file")
+ parser.add_argument("prediction_file", help="Prediction File")
+ args = parser.parse_args()
+ with open(args.dataset_file) as dataset_file:
+ dataset_json = json.load(dataset_file)
+ if dataset_json["version"] != expected_version:
+ print(
+ "Evaluation expects v-" + expected_version + ", but got dataset with v-" + dataset_json["version"],
+ file=sys.stderr,
+ )
+ dataset = dataset_json["data"]
+ with open(args.prediction_file) as prediction_file:
+ predictions = json.load(prediction_file)
+ print(json.dumps(evaluate(dataset, predictions)))
diff --git a/metrics/squad/squad.py b/metrics/squad/squad.py
new file mode 100644
index 0000000..1456f63
--- /dev/null
+++ b/metrics/squad/squad.py
@@ -0,0 +1,109 @@
+# Copyright 2020 The HuggingFace Datasets Authors.
+#
+# Licensed under the Apache License, Version 2.0 (the "License");
+# you may not use this file except in compliance with the License.
+# You may obtain a copy of the License at
+#
+# http://www.apache.org/licenses/LICENSE-2.0
+#
+# Unless required by applicable law or agreed to in writing, software
+# distributed under the License is distributed on an "AS IS" BASIS,
+# WITHOUT WARRANTIES OR CONDITIONS OF ANY KIND, either express or implied.
+# See the License for the specific language governing permissions and
+# limitations under the License.
+""" SQuAD metric. """
+
+import datasets
+
+from .evaluate import evaluate
+
+
+_CITATION = """\
+@inproceedings{Rajpurkar2016SQuAD10,
+ title={SQuAD: 100, 000+ Questions for Machine Comprehension of Text},
+ author={Pranav Rajpurkar and Jian Zhang and Konstantin Lopyrev and Percy Liang},
+ booktitle={EMNLP},
+ year={2016}
+}
+"""
+
+_DESCRIPTION = """
+This metric wrap the official scoring script for version 1 of the Stanford Question Answering Dataset (SQuAD).
+
+Stanford Question Answering Dataset (SQuAD) is a reading comprehension dataset, consisting of questions posed by
+crowdworkers on a set of Wikipedia articles, where the answer to every question is a segment of text, or span,
+from the corresponding reading passage, or the question might be unanswerable.
+"""
+
+_KWARGS_DESCRIPTION = """
+Computes SQuAD scores (F1 and EM).
+Args:
+ predictions: List of question-answers dictionaries with the following key-values:
+ - 'id': id of the question-answer pair as given in the references (see below)
+ - 'prediction_text': the text of the answer
+ references: List of question-answers dictionaries with the following key-values:
+ - 'id': id of the question-answer pair (see above),
+ - 'answers': a Dict in the SQuAD dataset format
+ {
+ 'text': list of possible texts for the answer, as a list of strings
+ 'answer_start': list of start positions for the answer, as a list of ints
+ }
+ Note that answer_start values are not taken into account to compute the metric.
+Returns:
+ 'exact_match': Exact match (the normalized answer exactly match the gold answer)
+ 'f1': The F-score of predicted tokens versus the gold answer
+Examples:
+
+ >>> predictions = [{'prediction_text': '1976', 'id': '56e10a3be3433e1400422b22'}]
+ >>> references = [{'answers': {'answer_start': [97], 'text': ['1976']}, 'id': '56e10a3be3433e1400422b22'}]
+ >>> squad_metric = datasets.load_metric("squad")
+ >>> results = squad_metric.compute(predictions=predictions, references=references)
+ >>> print(results)
+ {'exact_match': 100.0, 'f1': 100.0}
+"""
+
+
+@datasets.utils.file_utils.add_start_docstrings(_DESCRIPTION, _KWARGS_DESCRIPTION)
+class Squad(datasets.Metric):
+ def _info(self):
+ return datasets.MetricInfo(
+ description=_DESCRIPTION,
+ citation=_CITATION,
+ inputs_description=_KWARGS_DESCRIPTION,
+ features=datasets.Features(
+ {
+ "predictions": {"id": datasets.Value("string"), "prediction_text": datasets.Value("string")},
+ "references": {
+ "id": datasets.Value("string"),
+ "answers": datasets.features.Sequence(
+ {
+ "text": datasets.Value("string"),
+ "answer_start": datasets.Value("int32"),
+ }
+ ),
+ },
+ }
+ ),
+ codebase_urls=["https://rajpurkar.github.io/SQuAD-explorer/"],
+ reference_urls=["https://rajpurkar.github.io/SQuAD-explorer/"],
+ )
+
+ def _compute(self, predictions, references):
+ pred_dict = {prediction["id"]: prediction["prediction_text"] for prediction in predictions}
+ dataset = [
+ {
+ "paragraphs": [
+ {
+ "qas": [
+ {
+ "answers": [{"text": answer_text} for answer_text in ref["answers"]["text"]],
+ "id": ref["id"],
+ }
+ for ref in references
+ ]
+ }
+ ]
+ }
+ ]
+ score = evaluate(dataset=dataset, predictions=pred_dict)
+ return score
diff --git a/metrics/squad_v2/README.md b/metrics/squad_v2/README.md
new file mode 100644
index 0000000..fdd97e3
--- /dev/null
+++ b/metrics/squad_v2/README.md
@@ -0,0 +1,117 @@
+# Metric Card for SQuAD v2
+
+## Metric description
+This metric wraps the official scoring script for version 2 of the [Stanford Question Answering Dataset (SQuAD)](https://huggingface.co/datasets/squad_v2).
+
+SQuAD is a reading comprehension dataset, consisting of questions posed by crowdworkers on a set of Wikipedia articles, where the answer to every question is a segment of text, or span, from the corresponding reading passage, or the question might be unanswerable.
+
+SQuAD 2.0 combines the 100,000 questions in SQuAD 1.1 with over 50,000 unanswerable questions written adversarially by crowdworkers to look similar to answerable ones. To do well on SQuAD2.0, systems must not only answer questions when possible, but also determine when no answer is supported by the paragraph and abstain from answering.
+
+## How to use
+
+The metric takes two files or two lists - one representing model predictions and the other the references to compare them to.
+
+*Predictions* : List of triple for question-answers to score with the following key-value pairs:
+* `'id'`: the question-answer identification field of the question and answer pair
+* `'prediction_text'` : the text of the answer
+* `'no_answer_probability'` : the probability that the question has no answer
+
+*References*: List of question-answers dictionaries with the following key-value pairs:
+* `'id'`: id of the question-answer pair (see above),
+* `'answers'`: a list of Dict {'text': text of the answer as a string}
+* `'no_answer_threshold'`: the probability threshold to decide that a question has no answer.
+
+```python
+from datasets import load_metric
+squad_metric = load_metric("squad_v2")
+results = squad_metric.compute(predictions=predictions, references=references)
+```
+## Output values
+
+This metric outputs a dictionary with 13 values:
+* `'exact'`: Exact match (the normalized answer exactly match the gold answer) (see the `exact_match` metric (forthcoming))
+* `'f1'`: The average F1-score of predicted tokens versus the gold answer (see the [F1 score](https://huggingface.co/metrics/f1) metric)
+* `'total'`: Number of scores considered
+* `'HasAns_exact'`: Exact match (the normalized answer exactly match the gold answer)
+* `'HasAns_f1'`: The F-score of predicted tokens versus the gold answer
+* `'HasAns_total'`: How many of the questions have answers
+* `'NoAns_exact'`: Exact match (the normalized answer exactly match the gold answer)
+* `'NoAns_f1'`: The F-score of predicted tokens versus the gold answer
+* `'NoAns_total'`: How many of the questions have no answers
+* `'best_exact'` : Best exact match (with varying threshold)
+* `'best_exact_thresh'`: No-answer probability threshold associated to the best exact match
+* `'best_f1'`: Best F1 score (with varying threshold)
+* `'best_f1_thresh'`: No-answer probability threshold associated to the best F1
+
+
+The range of `exact_match` is 0-100, where 0.0 means no answers were matched and 100.0 means all answers were matched.
+
+The range of `f1` is 0-1 -- its lowest possible value is 0, if either the precision or the recall is 0, and its highest possible value is 1.0, which means perfect precision and recall.
+
+The range of `total` depends on the length of predictions/references: its minimal value is 0, and maximal value is the total number of questions in the predictions and references.
+
+### Values from popular papers
+The [SQuAD v2 paper](https://arxiv.org/pdf/1806.03822.pdf) reported an F1 score of 66.3% and an Exact Match score of 63.4%.
+They also report that human performance on the dataset represents an F1 score of 89.5% and an Exact Match score of 86.9%.
+
+For more recent model performance, see the [dataset leaderboard](https://paperswithcode.com/dataset/squad).
+
+## Examples
+
+Maximal values for both exact match and F1 (perfect match):
+
+```python
+from datasets import load_metric
+squad_v2_ metric = load_metric("squad_v2")
+predictions = [{'prediction_text': '1976', 'id': '56e10a3be3433e1400422b22', 'no_answer_probability': 0.}]
+references = [{'answers': {'answer_start': [97], 'text': ['1976']}, 'id': '56e10a3be3433e1400422b22'}]
+results = squad_v2_metric.compute(predictions=predictions, references=references)
+results
+{'exact': 100.0, 'f1': 100.0, 'total': 1, 'HasAns_exact': 100.0, 'HasAns_f1': 100.0, 'HasAns_total': 1, 'best_exact': 100.0, 'best_exact_thresh': 0.0, 'best_f1': 100.0, 'best_f1_thresh': 0.0}
+```
+
+Minimal values for both exact match and F1 (no match):
+
+```python
+from datasets import load_metric
+squad_metric = load_metric("squad_v2")
+predictions = [{'prediction_text': '1999', 'id': '56e10a3be3433e1400422b22', 'no_answer_probability': 0.}]
+references = [{'answers': {'answer_start': [97], 'text': ['1976']}, 'id': '56e10a3be3433e1400422b22'}]
+results = squad_v2_metric.compute(predictions=predictions, references=references)
+results
+{'exact': 0.0, 'f1': 0.0, 'total': 1, 'HasAns_exact': 0.0, 'HasAns_f1': 0.0, 'HasAns_total': 1, 'best_exact': 0.0, 'best_exact_thresh': 0.0, 'best_f1': 0.0, 'best_f1_thresh': 0.0}
+```
+
+Partial match (2 out of 3 answers correct) :
+
+```python
+from datasets import load_metric
+squad_metric = load_metric("squad_v2")
+predictions = [{'prediction_text': '1976', 'id': '56e10a3be3433e1400422b22', 'no_answer_probability': 0.}, {'prediction_text': 'Beyonce', 'id': '56d2051ce7d4791d0090260b', 'no_answer_probability': 0.}, {'prediction_text': 'climate change', 'id': '5733b5344776f419006610e1', 'no_answer_probability': 0.}]
+references = [{'answers': {'answer_start': [97], 'text': ['1976']}, 'id': '56e10a3be3433e1400422b22'}, {'answers': {'answer_start': [233], 'text': ['Beyoncé and Bruno Mars']}, 'id': '56d2051ce7d4791d0090260b'}, {'answers': {'answer_start': [891], 'text': ['climate change']}, 'id': '5733b5344776f419006610e1'}]
+results = squad_v2_metric.compute(predictions=predictions, references=references)
+results
+{'exact': 66.66666666666667, 'f1': 66.66666666666667, 'total': 3, 'HasAns_exact': 66.66666666666667, 'HasAns_f1': 66.66666666666667, 'HasAns_total': 3, 'best_exact': 66.66666666666667, 'best_exact_thresh': 0.0, 'best_f1': 66.66666666666667, 'best_f1_thresh': 0.0}
+```
+
+## Limitations and bias
+This metric works only with the datasets in the same format as the [SQuAD v.2 dataset](https://huggingface.co/datasets/squad_v2).
+
+The SQuAD datasets do contain a certain amount of noise, such as duplicate questions as well as missing answers, but these represent a minority of the 100,000 question-answer pairs. Also, neither exact match nor F1 score reflect whether models do better on certain types of questions (e.g. who questions) or those that cover a certain gender or geographical area -- carrying out more in-depth error analysis can complement these numbers.
+
+
+## Citation
+
+```bibtex
+@inproceedings{Rajpurkar2018SQuAD2,
+title={Know What You Don't Know: Unanswerable Questions for SQuAD},
+author={Pranav Rajpurkar and Jian Zhang and Percy Liang},
+booktitle={ACL 2018},
+year={2018}
+}
+```
+
+## Further References
+
+- [The Stanford Question Answering Dataset: Background, Challenges, Progress (blog post)](https://rajpurkar.github.io/mlx/qa-and-squad/)
+- [Hugging Face Course -- Question Answering](https://huggingface.co/course/chapter7/7)
diff --git a/metrics/squad_v2/evaluate.py b/metrics/squad_v2/evaluate.py
new file mode 100644
index 0000000..3b512ae
--- /dev/null
+++ b/metrics/squad_v2/evaluate.py
@@ -0,0 +1,323 @@
+"""Official evaluation script for SQuAD version 2.0.
+
+In addition to basic functionality, we also compute additional statistics and
+plot precision-recall curves if an additional na_prob.json file is provided.
+This file is expected to map question ID's to the model's predicted probability
+that a question is unanswerable.
+"""
+import argparse
+import collections
+import json
+import os
+import re
+import string
+import sys
+
+import numpy as np
+
+
+ARTICLES_REGEX = re.compile(r"\b(a|an|the)\b", re.UNICODE)
+
+OPTS = None
+
+
+def parse_args():
+ parser = argparse.ArgumentParser("Official evaluation script for SQuAD version 2.0.")
+ parser.add_argument("data_file", metavar="data.json", help="Input data JSON file.")
+ parser.add_argument("pred_file", metavar="pred.json", help="Model predictions.")
+ parser.add_argument(
+ "--out-file", "-o", metavar="eval.json", help="Write accuracy metrics to file (default is stdout)."
+ )
+ parser.add_argument(
+ "--na-prob-file", "-n", metavar="na_prob.json", help="Model estimates of probability of no answer."
+ )
+ parser.add_argument(
+ "--na-prob-thresh",
+ "-t",
+ type=float,
+ default=1.0,
+ help='Predict "" if no-answer probability exceeds this (default = 1.0).',
+ )
+ parser.add_argument(
+ "--out-image-dir", "-p", metavar="out_images", default=None, help="Save precision-recall curves to directory."
+ )
+ parser.add_argument("--verbose", "-v", action="store_true")
+ if len(sys.argv) == 1:
+ parser.print_help()
+ sys.exit(1)
+ return parser.parse_args()
+
+
+def make_qid_to_has_ans(dataset):
+ qid_to_has_ans = {}
+ for article in dataset:
+ for p in article["paragraphs"]:
+ for qa in p["qas"]:
+ qid_to_has_ans[qa["id"]] = bool(qa["answers"]["text"])
+ return qid_to_has_ans
+
+
+def normalize_answer(s):
+ """Lower text and remove punctuation, articles and extra whitespace."""
+
+ def remove_articles(text):
+ return ARTICLES_REGEX.sub(" ", text)
+
+ def white_space_fix(text):
+ return " ".join(text.split())
+
+ def remove_punc(text):
+ exclude = set(string.punctuation)
+ return "".join(ch for ch in text if ch not in exclude)
+
+ def lower(text):
+ return text.lower()
+
+ return white_space_fix(remove_articles(remove_punc(lower(s))))
+
+
+def get_tokens(s):
+ if not s:
+ return []
+ return normalize_answer(s).split()
+
+
+def compute_exact(a_gold, a_pred):
+ return int(normalize_answer(a_gold) == normalize_answer(a_pred))
+
+
+def compute_f1(a_gold, a_pred):
+ gold_toks = get_tokens(a_gold)
+ pred_toks = get_tokens(a_pred)
+ common = collections.Counter(gold_toks) & collections.Counter(pred_toks)
+ num_same = sum(common.values())
+ if len(gold_toks) == 0 or len(pred_toks) == 0:
+ # If either is no-answer, then F1 is 1 if they agree, 0 otherwise
+ return int(gold_toks == pred_toks)
+ if num_same == 0:
+ return 0
+ precision = 1.0 * num_same / len(pred_toks)
+ recall = 1.0 * num_same / len(gold_toks)
+ f1 = (2 * precision * recall) / (precision + recall)
+ return f1
+
+
+def get_raw_scores(dataset, preds):
+ exact_scores = {}
+ f1_scores = {}
+ for article in dataset:
+ for p in article["paragraphs"]:
+ for qa in p["qas"]:
+ qid = qa["id"]
+ gold_answers = [t for t in qa["answers"]["text"] if normalize_answer(t)]
+ if not gold_answers:
+ # For unanswerable questions, only correct answer is empty string
+ gold_answers = [""]
+ if qid not in preds:
+ print(f"Missing prediction for {qid}")
+ continue
+ a_pred = preds[qid]
+ # Take max over all gold answers
+ exact_scores[qid] = max(compute_exact(a, a_pred) for a in gold_answers)
+ f1_scores[qid] = max(compute_f1(a, a_pred) for a in gold_answers)
+ return exact_scores, f1_scores
+
+
+def apply_no_ans_threshold(scores, na_probs, qid_to_has_ans, na_prob_thresh):
+ new_scores = {}
+ for qid, s in scores.items():
+ pred_na = na_probs[qid] > na_prob_thresh
+ if pred_na:
+ new_scores[qid] = float(not qid_to_has_ans[qid])
+ else:
+ new_scores[qid] = s
+ return new_scores
+
+
+def make_eval_dict(exact_scores, f1_scores, qid_list=None):
+ if not qid_list:
+ total = len(exact_scores)
+ return collections.OrderedDict(
+ [
+ ("exact", 100.0 * sum(exact_scores.values()) / total),
+ ("f1", 100.0 * sum(f1_scores.values()) / total),
+ ("total", total),
+ ]
+ )
+ else:
+ total = len(qid_list)
+ return collections.OrderedDict(
+ [
+ ("exact", 100.0 * sum(exact_scores[k] for k in qid_list) / total),
+ ("f1", 100.0 * sum(f1_scores[k] for k in qid_list) / total),
+ ("total", total),
+ ]
+ )
+
+
+def merge_eval(main_eval, new_eval, prefix):
+ for k in new_eval:
+ main_eval[f"{prefix}_{k}"] = new_eval[k]
+
+
+def plot_pr_curve(precisions, recalls, out_image, title):
+ plt.step(recalls, precisions, color="b", alpha=0.2, where="post")
+ plt.fill_between(recalls, precisions, step="post", alpha=0.2, color="b")
+ plt.xlabel("Recall")
+ plt.ylabel("Precision")
+ plt.xlim([0.0, 1.05])
+ plt.ylim([0.0, 1.05])
+ plt.title(title)
+ plt.savefig(out_image)
+ plt.clf()
+
+
+def make_precision_recall_eval(scores, na_probs, num_true_pos, qid_to_has_ans, out_image=None, title=None):
+ qid_list = sorted(na_probs, key=lambda k: na_probs[k])
+ true_pos = 0.0
+ cur_p = 1.0
+ cur_r = 0.0
+ precisions = [1.0]
+ recalls = [0.0]
+ avg_prec = 0.0
+ for i, qid in enumerate(qid_list):
+ if qid_to_has_ans[qid]:
+ true_pos += scores[qid]
+ cur_p = true_pos / float(i + 1)
+ cur_r = true_pos / float(num_true_pos)
+ if i == len(qid_list) - 1 or na_probs[qid] != na_probs[qid_list[i + 1]]:
+ # i.e., if we can put a threshold after this point
+ avg_prec += cur_p * (cur_r - recalls[-1])
+ precisions.append(cur_p)
+ recalls.append(cur_r)
+ if out_image:
+ plot_pr_curve(precisions, recalls, out_image, title)
+ return {"ap": 100.0 * avg_prec}
+
+
+def run_precision_recall_analysis(main_eval, exact_raw, f1_raw, na_probs, qid_to_has_ans, out_image_dir):
+ if out_image_dir and not os.path.exists(out_image_dir):
+ os.makedirs(out_image_dir)
+ num_true_pos = sum(1 for v in qid_to_has_ans.values() if v)
+ if num_true_pos == 0:
+ return
+ pr_exact = make_precision_recall_eval(
+ exact_raw,
+ na_probs,
+ num_true_pos,
+ qid_to_has_ans,
+ out_image=os.path.join(out_image_dir, "pr_exact.png"),
+ title="Precision-Recall curve for Exact Match score",
+ )
+ pr_f1 = make_precision_recall_eval(
+ f1_raw,
+ na_probs,
+ num_true_pos,
+ qid_to_has_ans,
+ out_image=os.path.join(out_image_dir, "pr_f1.png"),
+ title="Precision-Recall curve for F1 score",
+ )
+ oracle_scores = {k: float(v) for k, v in qid_to_has_ans.items()}
+ pr_oracle = make_precision_recall_eval(
+ oracle_scores,
+ na_probs,
+ num_true_pos,
+ qid_to_has_ans,
+ out_image=os.path.join(out_image_dir, "pr_oracle.png"),
+ title="Oracle Precision-Recall curve (binary task of HasAns vs. NoAns)",
+ )
+ merge_eval(main_eval, pr_exact, "pr_exact")
+ merge_eval(main_eval, pr_f1, "pr_f1")
+ merge_eval(main_eval, pr_oracle, "pr_oracle")
+
+
+def histogram_na_prob(na_probs, qid_list, image_dir, name):
+ if not qid_list:
+ return
+ x = [na_probs[k] for k in qid_list]
+ weights = np.ones_like(x) / float(len(x))
+ plt.hist(x, weights=weights, bins=20, range=(0.0, 1.0))
+ plt.xlabel("Model probability of no-answer")
+ plt.ylabel("Proportion of dataset")
+ plt.title(f"Histogram of no-answer probability: {name}")
+ plt.savefig(os.path.join(image_dir, f"na_prob_hist_{name}.png"))
+ plt.clf()
+
+
+def find_best_thresh(preds, scores, na_probs, qid_to_has_ans):
+ num_no_ans = sum(1 for k in qid_to_has_ans if not qid_to_has_ans[k])
+ cur_score = num_no_ans
+ best_score = cur_score
+ best_thresh = 0.0
+ qid_list = sorted(na_probs, key=lambda k: na_probs[k])
+ for i, qid in enumerate(qid_list):
+ if qid not in scores:
+ continue
+ if qid_to_has_ans[qid]:
+ diff = scores[qid]
+ else:
+ if preds[qid]:
+ diff = -1
+ else:
+ diff = 0
+ cur_score += diff
+ if cur_score > best_score:
+ best_score = cur_score
+ best_thresh = na_probs[qid]
+ return 100.0 * best_score / len(scores), best_thresh
+
+
+def find_all_best_thresh(main_eval, preds, exact_raw, f1_raw, na_probs, qid_to_has_ans):
+ best_exact, exact_thresh = find_best_thresh(preds, exact_raw, na_probs, qid_to_has_ans)
+ best_f1, f1_thresh = find_best_thresh(preds, f1_raw, na_probs, qid_to_has_ans)
+ main_eval["best_exact"] = best_exact
+ main_eval["best_exact_thresh"] = exact_thresh
+ main_eval["best_f1"] = best_f1
+ main_eval["best_f1_thresh"] = f1_thresh
+
+
+def main():
+ with open(OPTS.data_file) as f:
+ dataset_json = json.load(f)
+ dataset = dataset_json["data"]
+ with open(OPTS.pred_file) as f:
+ preds = json.load(f)
+ if OPTS.na_prob_file:
+ with open(OPTS.na_prob_file) as f:
+ na_probs = json.load(f)
+ else:
+ na_probs = {k: 0.0 for k in preds}
+ qid_to_has_ans = make_qid_to_has_ans(dataset) # maps qid to True/False
+ has_ans_qids = [k for k, v in qid_to_has_ans.items() if v]
+ no_ans_qids = [k for k, v in qid_to_has_ans.items() if not v]
+ exact_raw, f1_raw = get_raw_scores(dataset, preds)
+ exact_thresh = apply_no_ans_threshold(exact_raw, na_probs, qid_to_has_ans, OPTS.na_prob_thresh)
+ f1_thresh = apply_no_ans_threshold(f1_raw, na_probs, qid_to_has_ans, OPTS.na_prob_thresh)
+ out_eval = make_eval_dict(exact_thresh, f1_thresh)
+ if has_ans_qids:
+ has_ans_eval = make_eval_dict(exact_thresh, f1_thresh, qid_list=has_ans_qids)
+ merge_eval(out_eval, has_ans_eval, "HasAns")
+ if no_ans_qids:
+ no_ans_eval = make_eval_dict(exact_thresh, f1_thresh, qid_list=no_ans_qids)
+ merge_eval(out_eval, no_ans_eval, "NoAns")
+ if OPTS.na_prob_file:
+ find_all_best_thresh(out_eval, preds, exact_raw, f1_raw, na_probs, qid_to_has_ans)
+ if OPTS.na_prob_file and OPTS.out_image_dir:
+ run_precision_recall_analysis(out_eval, exact_raw, f1_raw, na_probs, qid_to_has_ans, OPTS.out_image_dir)
+ histogram_na_prob(na_probs, has_ans_qids, OPTS.out_image_dir, "hasAns")
+ histogram_na_prob(na_probs, no_ans_qids, OPTS.out_image_dir, "noAns")
+ if OPTS.out_file:
+ with open(OPTS.out_file, "w") as f:
+ json.dump(out_eval, f)
+ else:
+ print(json.dumps(out_eval, indent=2))
+
+
+if __name__ == "__main__":
+ OPTS = parse_args()
+ if OPTS.out_image_dir:
+ import matplotlib
+
+ matplotlib.use("Agg")
+ import matplotlib.pyplot as plt
+ main()
diff --git a/metrics/squad_v2/squad_v2.py b/metrics/squad_v2/squad_v2.py
new file mode 100644
index 0000000..b94db86
--- /dev/null
+++ b/metrics/squad_v2/squad_v2.py
@@ -0,0 +1,135 @@
+# Copyright 2020 The HuggingFace Datasets Authors.
+#
+# Licensed under the Apache License, Version 2.0 (the "License");
+# you may not use this file except in compliance with the License.
+# You may obtain a copy of the License at
+#
+# http://www.apache.org/licenses/LICENSE-2.0
+#
+# Unless required by applicable law or agreed to in writing, software
+# distributed under the License is distributed on an "AS IS" BASIS,
+# WITHOUT WARRANTIES OR CONDITIONS OF ANY KIND, either express or implied.
+# See the License for the specific language governing permissions and
+# limitations under the License.
+""" SQuAD v2 metric. """
+
+import datasets
+
+from .evaluate import (
+ apply_no_ans_threshold,
+ find_all_best_thresh,
+ get_raw_scores,
+ make_eval_dict,
+ make_qid_to_has_ans,
+ merge_eval,
+)
+
+
+_CITATION = """\
+@inproceedings{Rajpurkar2016SQuAD10,
+ title={SQuAD: 100, 000+ Questions for Machine Comprehension of Text},
+ author={Pranav Rajpurkar and Jian Zhang and Konstantin Lopyrev and Percy Liang},
+ booktitle={EMNLP},
+ year={2016}
+}
+"""
+
+_DESCRIPTION = """
+This metric wrap the official scoring script for version 2 of the Stanford Question
+Answering Dataset (SQuAD).
+
+Stanford Question Answering Dataset (SQuAD) is a reading comprehension dataset, consisting of questions posed by
+crowdworkers on a set of Wikipedia articles, where the answer to every question is a segment of text, or span,
+from the corresponding reading passage, or the question might be unanswerable.
+
+SQuAD2.0 combines the 100,000 questions in SQuAD1.1 with over 50,000 unanswerable questions
+written adversarially by crowdworkers to look similar to answerable ones.
+To do well on SQuAD2.0, systems must not only answer questions when possible, but also
+determine when no answer is supported by the paragraph and abstain from answering.
+"""
+
+_KWARGS_DESCRIPTION = """
+Computes SQuAD v2 scores (F1 and EM).
+Args:
+ predictions: List of triple for question-answers to score with the following elements:
+ - the question-answer 'id' field as given in the references (see below)
+ - the text of the answer
+ - the probability that the question has no answer
+ references: List of question-answers dictionaries with the following key-values:
+ - 'id': id of the question-answer pair (see above),
+ - 'answers': a list of Dict {'text': text of the answer as a string}
+ no_answer_threshold: float
+ Probability threshold to decide that a question has no answer.
+Returns:
+ 'exact': Exact match (the normalized answer exactly match the gold answer)
+ 'f1': The F-score of predicted tokens versus the gold answer
+ 'total': Number of score considered
+ 'HasAns_exact': Exact match (the normalized answer exactly match the gold answer)
+ 'HasAns_f1': The F-score of predicted tokens versus the gold answer
+ 'HasAns_total': Number of score considered
+ 'NoAns_exact': Exact match (the normalized answer exactly match the gold answer)
+ 'NoAns_f1': The F-score of predicted tokens versus the gold answer
+ 'NoAns_total': Number of score considered
+ 'best_exact': Best exact match (with varying threshold)
+ 'best_exact_thresh': No-answer probability threshold associated to the best exact match
+ 'best_f1': Best F1 (with varying threshold)
+ 'best_f1_thresh': No-answer probability threshold associated to the best F1
+Examples:
+
+ >>> predictions = [{'prediction_text': '1976', 'id': '56e10a3be3433e1400422b22', 'no_answer_probability': 0.}]
+ >>> references = [{'answers': {'answer_start': [97], 'text': ['1976']}, 'id': '56e10a3be3433e1400422b22'}]
+ >>> squad_v2_metric = datasets.load_metric("squad_v2")
+ >>> results = squad_v2_metric.compute(predictions=predictions, references=references)
+ >>> print(results)
+ {'exact': 100.0, 'f1': 100.0, 'total': 1, 'HasAns_exact': 100.0, 'HasAns_f1': 100.0, 'HasAns_total': 1, 'best_exact': 100.0, 'best_exact_thresh': 0.0, 'best_f1': 100.0, 'best_f1_thresh': 0.0}
+"""
+
+
+@datasets.utils.file_utils.add_start_docstrings(_DESCRIPTION, _KWARGS_DESCRIPTION)
+class SquadV2(datasets.Metric):
+ def _info(self):
+ return datasets.MetricInfo(
+ description=_DESCRIPTION,
+ citation=_CITATION,
+ inputs_description=_KWARGS_DESCRIPTION,
+ features=datasets.Features(
+ {
+ "predictions": {
+ "id": datasets.Value("string"),
+ "prediction_text": datasets.Value("string"),
+ "no_answer_probability": datasets.Value("float32"),
+ },
+ "references": {
+ "id": datasets.Value("string"),
+ "answers": datasets.features.Sequence(
+ {"text": datasets.Value("string"), "answer_start": datasets.Value("int32")}
+ ),
+ },
+ }
+ ),
+ codebase_urls=["https://rajpurkar.github.io/SQuAD-explorer/"],
+ reference_urls=["https://rajpurkar.github.io/SQuAD-explorer/"],
+ )
+
+ def _compute(self, predictions, references, no_answer_threshold=1.0):
+ no_answer_probabilities = {p["id"]: p["no_answer_probability"] for p in predictions}
+ dataset = [{"paragraphs": [{"qas": references}]}]
+ predictions = {p["id"]: p["prediction_text"] for p in predictions}
+
+ qid_to_has_ans = make_qid_to_has_ans(dataset) # maps qid to True/False
+ has_ans_qids = [k for k, v in qid_to_has_ans.items() if v]
+ no_ans_qids = [k for k, v in qid_to_has_ans.items() if not v]
+
+ exact_raw, f1_raw = get_raw_scores(dataset, predictions)
+ exact_thresh = apply_no_ans_threshold(exact_raw, no_answer_probabilities, qid_to_has_ans, no_answer_threshold)
+ f1_thresh = apply_no_ans_threshold(f1_raw, no_answer_probabilities, qid_to_has_ans, no_answer_threshold)
+ out_eval = make_eval_dict(exact_thresh, f1_thresh)
+
+ if has_ans_qids:
+ has_ans_eval = make_eval_dict(exact_thresh, f1_thresh, qid_list=has_ans_qids)
+ merge_eval(out_eval, has_ans_eval, "HasAns")
+ if no_ans_qids:
+ no_ans_eval = make_eval_dict(exact_thresh, f1_thresh, qid_list=no_ans_qids)
+ merge_eval(out_eval, no_ans_eval, "NoAns")
+ find_all_best_thresh(out_eval, predictions, exact_raw, f1_raw, no_answer_probabilities, qid_to_has_ans)
+ return dict(out_eval)
diff --git a/metrics/super_glue/README.md b/metrics/super_glue/README.md
new file mode 100644
index 0000000..d1e2b5b
--- /dev/null
+++ b/metrics/super_glue/README.md
@@ -0,0 +1,112 @@
+# Metric Card for SuperGLUE
+
+## Metric description
+This metric is used to compute the SuperGLUE evaluation metric associated to each of the subsets of the [SuperGLUE dataset](https://huggingface.co/datasets/super_glue).
+
+SuperGLUE is a new benchmark styled after GLUE with a new set of more difficult language understanding tasks, improved resources, and a new public leaderboard.
+
+
+## How to use
+
+There are two steps: (1) loading the SuperGLUE metric relevant to the subset of the dataset being used for evaluation; and (2) calculating the metric.
+
+1. **Loading the relevant SuperGLUE metric** : the subsets of SuperGLUE are the following: `boolq`, `cb`, `copa`, `multirc`, `record`, `rte`, `wic`, `wsc`, `wsc.fixed`, `axb`, `axg`.
+
+More information about the different subsets of the SuperGLUE dataset can be found on the [SuperGLUE dataset page](https://huggingface.co/datasets/super_glue) and on the [official dataset website](https://super.gluebenchmark.com/).
+
+2. **Calculating the metric**: the metric takes two inputs : one list with the predictions of the model to score and one list of reference labels. The structure of both inputs depends on the SuperGlUE subset being used:
+
+Format of `predictions`:
+- for `record`: list of question-answer dictionaries with the following keys:
+ - `idx`: index of the question as specified by the dataset
+ - `prediction_text`: the predicted answer text
+- for `multirc`: list of question-answer dictionaries with the following keys:
+ - `idx`: index of the question-answer pair as specified by the dataset
+ - `prediction`: the predicted answer label
+- otherwise: list of predicted labels
+
+Format of `references`:
+- for `record`: list of question-answers dictionaries with the following keys:
+ - `idx`: index of the question as specified by the dataset
+ - `answers`: list of possible answers
+- otherwise: list of reference labels
+
+```python
+from datasets import load_metric
+super_glue_metric = load_metric('super_glue', 'copa')
+predictions = [0, 1]
+references = [0, 1]
+results = super_glue_metric.compute(predictions=predictions, references=references)
+```
+## Output values
+
+The output of the metric depends on the SuperGLUE subset chosen, consisting of a dictionary that contains one or several of the following metrics:
+
+`exact_match`: A given predicted string's exact match score is 1 if it is the exact same as its reference string, and is 0 otherwise. (See [Exact Match](https://huggingface.co/metrics/exact_match) for more information).
+
+`f1`: the harmonic mean of the precision and recall (see [F1 score](https://huggingface.co/metrics/f1) for more information). Its range is 0-1 -- its lowest possible value is 0, if either the precision or the recall is 0, and its highest possible value is 1.0, which means perfect precision and recall.
+
+`matthews_correlation`: a measure of the quality of binary and multiclass classifications (see [Matthews Correlation](https://huggingface.co/metrics/matthews_correlation) for more information). Its range of values is between -1 and +1, where a coefficient of +1 represents a perfect prediction, 0 an average random prediction and -1 an inverse prediction.
+
+### Values from popular papers
+The [original SuperGLUE paper](https://arxiv.org/pdf/1905.00537.pdf) reported average scores ranging from 47 to 71.5%, depending on the model used (with all evaluation values scaled by 100 to make computing the average possible).
+
+For more recent model performance, see the [dataset leaderboard](https://super.gluebenchmark.com/leaderboard).
+
+## Examples
+
+Maximal values for the COPA subset (which outputs `accuracy`):
+
+```python
+from datasets import load_metric
+super_glue_metric = load_metric('super_glue', 'copa') # any of ["copa", "rte", "wic", "wsc", "wsc.fixed", "boolq", "axg"]
+predictions = [0, 1]
+references = [0, 1]
+results = super_glue_metric.compute(predictions=predictions, references=references)
+print(results)
+{'accuracy': 1.0}
+```
+
+Minimal values for the MultiRC subset (which outputs `pearson` and `spearmanr`):
+
+```python
+from datasets import load_metric
+super_glue_metric = load_metric('super_glue', 'multirc')
+predictions = [{'idx': {'answer': 0, 'paragraph': 0, 'question': 0}, 'prediction': 0}, {'idx': {'answer': 1, 'paragraph': 2, 'question': 3}, 'prediction': 1}]
+references = [1,0]
+results = super_glue_metric.compute(predictions=predictions, references=references)
+print(results)
+{'exact_match': 0.0, 'f1_m': 0.0, 'f1_a': 0.0}
+```
+
+Partial match for the COLA subset (which outputs `matthews_correlation`)
+
+```python
+from datasets import load_metric
+super_glue_metric = load_metric('super_glue', 'axb')
+references = [0, 1]
+predictions = [1,1]
+results = super_glue_metric.compute(predictions=predictions, references=references)
+print(results)
+{'matthews_correlation': 0.0}
+```
+
+## Limitations and bias
+This metric works only with datasets that have the same format as the [SuperGLUE dataset](https://huggingface.co/datasets/super_glue).
+
+The dataset also includes Winogender, a subset of the dataset that is designed to measure gender bias in coreference resolution systems. However, as noted in the SuperGLUE paper, this subset has its limitations: *"It offers only positive predictive value: A poor bias score is clear evidence that a model exhibits gender bias, but a good score does not mean that the model is unbiased.[...] Also, Winogender does not cover all forms of social bias, or even all forms of gender. For instance, the version of the data used here offers no coverage of gender-neutral they or non-binary pronouns."
+
+## Citation
+
+```bibtex
+@article{wang2019superglue,
+ title={Super{GLUE}: A Stickier Benchmark for General-Purpose Language Understanding Systems},
+ author={Wang, Alex and Pruksachatkun, Yada and Nangia, Nikita and Singh, Amanpreet and Michael, Julian and Hill, Felix and Levy, Omer and Bowman, Samuel R},
+ journal={arXiv preprint arXiv:1905.00537},
+ year={2019}
+}
+```
+
+## Further References
+
+- [SuperGLUE benchmark homepage](https://super.gluebenchmark.com/)
diff --git a/metrics/super_glue/record_evaluation.py b/metrics/super_glue/record_evaluation.py
new file mode 100644
index 0000000..396d7ac
--- /dev/null
+++ b/metrics/super_glue/record_evaluation.py
@@ -0,0 +1,111 @@
+"""
+Official evaluation script for ReCoRD v1.0.
+(Some functions are adopted from the SQuAD evaluation script.)
+"""
+
+
+import argparse
+import json
+import re
+import string
+import sys
+from collections import Counter
+
+
+def normalize_answer(s):
+ """Lower text and remove punctuation, articles and extra whitespace."""
+
+ def remove_articles(text):
+ return re.sub(r"\b(a|an|the)\b", " ", text)
+
+ def white_space_fix(text):
+ return " ".join(text.split())
+
+ def remove_punc(text):
+ exclude = set(string.punctuation)
+ return "".join(ch for ch in text if ch not in exclude)
+
+ def lower(text):
+ return text.lower()
+
+ return white_space_fix(remove_articles(remove_punc(lower(s))))
+
+
+def f1_score(prediction, ground_truth):
+ prediction_tokens = normalize_answer(prediction).split()
+ ground_truth_tokens = normalize_answer(ground_truth).split()
+ common = Counter(prediction_tokens) & Counter(ground_truth_tokens)
+ num_same = sum(common.values())
+ if num_same == 0:
+ return 0
+ precision = 1.0 * num_same / len(prediction_tokens)
+ recall = 1.0 * num_same / len(ground_truth_tokens)
+ f1 = (2 * precision * recall) / (precision + recall)
+ return f1
+
+
+def exact_match_score(prediction, ground_truth):
+ return normalize_answer(prediction) == normalize_answer(ground_truth)
+
+
+def metric_max_over_ground_truths(metric_fn, prediction, ground_truths):
+ scores_for_ground_truths = []
+ for ground_truth in ground_truths:
+ score = metric_fn(prediction, ground_truth)
+ scores_for_ground_truths.append(score)
+ return max(scores_for_ground_truths)
+
+
+def evaluate(dataset, predictions):
+ f1 = exact_match = total = 0
+ correct_ids = []
+ for passage in dataset:
+ for qa in passage["qas"]:
+ total += 1
+ if qa["id"] not in predictions:
+ message = f'Unanswered question {qa["id"]} will receive score 0.'
+ print(message, file=sys.stderr)
+ continue
+
+ ground_truths = list(map(lambda x: x["text"], qa["answers"]))
+ prediction = predictions[qa["id"]]
+
+ _exact_match = metric_max_over_ground_truths(exact_match_score, prediction, ground_truths)
+ if int(_exact_match) == 1:
+ correct_ids.append(qa["id"])
+ exact_match += _exact_match
+
+ f1 += metric_max_over_ground_truths(f1_score, prediction, ground_truths)
+
+ exact_match = exact_match / total
+ f1 = f1 / total
+
+ return {"exact_match": exact_match, "f1": f1}, correct_ids
+
+
+if __name__ == "__main__":
+ expected_version = "1.0"
+ parser = argparse.ArgumentParser("Official evaluation script for ReCoRD v1.0.")
+ parser.add_argument("data_file", help="The dataset file in JSON format.")
+ parser.add_argument("pred_file", help="The model prediction file in JSON format.")
+ parser.add_argument("--output_correct_ids", action="store_true", help="Output the correctly answered query IDs.")
+ args = parser.parse_args()
+
+ with open(args.data_file) as data_file:
+ dataset_json = json.load(data_file)
+ if dataset_json["version"] != expected_version:
+ print(
+ f'Evaluation expects v-{expected_version}, but got dataset with v-{dataset_json["version"]}',
+ file=sys.stderr,
+ )
+ dataset = dataset_json["data"]
+
+ with open(args.pred_file) as pred_file:
+ predictions = json.load(pred_file)
+
+ metrics, correct_ids = evaluate(dataset, predictions)
+
+ if args.output_correct_ids:
+ print(f"Output {len(correct_ids)} correctly answered question IDs.")
+ with open("correct_ids.json", "w") as f:
+ json.dump(correct_ids, f)
diff --git a/metrics/super_glue/super_glue.py b/metrics/super_glue/super_glue.py
new file mode 100644
index 0000000..5be291b
--- /dev/null
+++ b/metrics/super_glue/super_glue.py
@@ -0,0 +1,236 @@
+# Copyright 2020 The HuggingFace Datasets Authors.
+#
+# Licensed under the Apache License, Version 2.0 (the "License");
+# you may not use this file except in compliance with the License.
+# You may obtain a copy of the License at
+#
+# http://www.apache.org/licenses/LICENSE-2.0
+#
+# Unless required by applicable law or agreed to in writing, software
+# distributed under the License is distributed on an "AS IS" BASIS,
+# WITHOUT WARRANTIES OR CONDITIONS OF ANY KIND, either express or implied.
+# See the License for the specific language governing permissions and
+# limitations under the License.
+"""The SuperGLUE benchmark metric."""
+
+from sklearn.metrics import f1_score, matthews_corrcoef
+
+import datasets
+
+from .record_evaluation import evaluate as evaluate_record
+
+
+_CITATION = """\
+@article{wang2019superglue,
+ title={SuperGLUE: A Stickier Benchmark for General-Purpose Language Understanding Systems},
+ author={Wang, Alex and Pruksachatkun, Yada and Nangia, Nikita and Singh, Amanpreet and Michael, Julian and Hill, Felix and Levy, Omer and Bowman, Samuel R},
+ journal={arXiv preprint arXiv:1905.00537},
+ year={2019}
+}
+"""
+
+_DESCRIPTION = """\
+SuperGLUE (https://super.gluebenchmark.com/) is a new benchmark styled after
+GLUE with a new set of more difficult language understanding tasks, improved
+resources, and a new public leaderboard.
+"""
+
+_KWARGS_DESCRIPTION = """
+Compute SuperGLUE evaluation metric associated to each SuperGLUE dataset.
+Args:
+ predictions: list of predictions to score. Depending on the SuperGlUE subset:
+ - for 'record': list of question-answer dictionaries with the following keys:
+ - 'idx': index of the question as specified by the dataset
+ - 'prediction_text': the predicted answer text
+ - for 'multirc': list of question-answer dictionaries with the following keys:
+ - 'idx': index of the question-answer pair as specified by the dataset
+ - 'prediction': the predicted answer label
+ - otherwise: list of predicted labels
+ references: list of reference labels. Depending on the SuperGLUE subset:
+ - for 'record': list of question-answers dictionaries with the following keys:
+ - 'idx': index of the question as specified by the dataset
+ - 'answers': list of possible answers
+ - otherwise: list of reference labels
+Returns: depending on the SuperGLUE subset:
+ - for 'record':
+ - 'exact_match': Exact match between answer and gold answer
+ - 'f1': F1 score
+ - for 'multirc':
+ - 'exact_match': Exact match between answer and gold answer
+ - 'f1_m': Per-question macro-F1 score
+ - 'f1_a': Average F1 score over all answers
+ - for 'axb':
+ 'matthews_correlation': Matthew Correlation
+ - for 'cb':
+ - 'accuracy': Accuracy
+ - 'f1': F1 score
+ - for all others:
+ - 'accuracy': Accuracy
+Examples:
+
+ >>> super_glue_metric = datasets.load_metric('super_glue', 'copa') # any of ["copa", "rte", "wic", "wsc", "wsc.fixed", "boolq", "axg"]
+ >>> predictions = [0, 1]
+ >>> references = [0, 1]
+ >>> results = super_glue_metric.compute(predictions=predictions, references=references)
+ >>> print(results)
+ {'accuracy': 1.0}
+
+ >>> super_glue_metric = datasets.load_metric('super_glue', 'cb')
+ >>> predictions = [0, 1]
+ >>> references = [0, 1]
+ >>> results = super_glue_metric.compute(predictions=predictions, references=references)
+ >>> print(results)
+ {'accuracy': 1.0, 'f1': 1.0}
+
+ >>> super_glue_metric = datasets.load_metric('super_glue', 'record')
+ >>> predictions = [{'idx': {'passage': 0, 'query': 0}, 'prediction_text': 'answer'}]
+ >>> references = [{'idx': {'passage': 0, 'query': 0}, 'answers': ['answer', 'another_answer']}]
+ >>> results = super_glue_metric.compute(predictions=predictions, references=references)
+ >>> print(results)
+ {'exact_match': 1.0, 'f1': 1.0}
+
+ >>> super_glue_metric = datasets.load_metric('super_glue', 'multirc')
+ >>> predictions = [{'idx': {'answer': 0, 'paragraph': 0, 'question': 0}, 'prediction': 0}, {'idx': {'answer': 1, 'paragraph': 2, 'question': 3}, 'prediction': 1}]
+ >>> references = [0, 1]
+ >>> results = super_glue_metric.compute(predictions=predictions, references=references)
+ >>> print(results)
+ {'exact_match': 1.0, 'f1_m': 1.0, 'f1_a': 1.0}
+
+ >>> super_glue_metric = datasets.load_metric('super_glue', 'axb')
+ >>> references = [0, 1]
+ >>> predictions = [0, 1]
+ >>> results = super_glue_metric.compute(predictions=predictions, references=references)
+ >>> print(results)
+ {'matthews_correlation': 1.0}
+"""
+
+
+def simple_accuracy(preds, labels):
+ return float((preds == labels).mean())
+
+
+def acc_and_f1(preds, labels, f1_avg="binary"):
+ acc = simple_accuracy(preds, labels)
+ f1 = float(f1_score(y_true=labels, y_pred=preds, average=f1_avg))
+ return {
+ "accuracy": acc,
+ "f1": f1,
+ }
+
+
+def evaluate_multirc(ids_preds, labels):
+ """
+ Computes F1 score and Exact Match for MultiRC predictions.
+ """
+ question_map = {}
+ for id_pred, label in zip(ids_preds, labels):
+ question_id = f'{id_pred["idx"]["paragraph"]}-{id_pred["idx"]["question"]}'
+ pred = id_pred["prediction"]
+ if question_id in question_map:
+ question_map[question_id].append((pred, label))
+ else:
+ question_map[question_id] = [(pred, label)]
+ f1s, ems = [], []
+ for question, preds_labels in question_map.items():
+ question_preds, question_labels = zip(*preds_labels)
+ f1 = f1_score(y_true=question_labels, y_pred=question_preds, average="macro")
+ f1s.append(f1)
+ em = int(sum(p == l for p, l in preds_labels) == len(preds_labels))
+ ems.append(em)
+ f1_m = float(sum(f1s) / len(f1s))
+ em = sum(ems) / len(ems)
+ f1_a = float(f1_score(y_true=labels, y_pred=[id_pred["prediction"] for id_pred in ids_preds]))
+ return {"exact_match": em, "f1_m": f1_m, "f1_a": f1_a}
+
+
+@datasets.utils.file_utils.add_start_docstrings(_DESCRIPTION, _KWARGS_DESCRIPTION)
+class SuperGlue(datasets.Metric):
+ def _info(self):
+ if self.config_name not in [
+ "boolq",
+ "cb",
+ "copa",
+ "multirc",
+ "record",
+ "rte",
+ "wic",
+ "wsc",
+ "wsc.fixed",
+ "axb",
+ "axg",
+ ]:
+ raise KeyError(
+ "You should supply a configuration name selected in "
+ '["boolq", "cb", "copa", "multirc", "record", "rte", "wic", "wsc", "wsc.fixed", "axb", "axg",]'
+ )
+ return datasets.MetricInfo(
+ description=_DESCRIPTION,
+ citation=_CITATION,
+ inputs_description=_KWARGS_DESCRIPTION,
+ features=datasets.Features(self._get_feature_types()),
+ codebase_urls=[],
+ reference_urls=[],
+ format="numpy" if not self.config_name == "record" and not self.config_name == "multirc" else None,
+ )
+
+ def _get_feature_types(self):
+ if self.config_name == "record":
+ return {
+ "predictions": {
+ "idx": {
+ "passage": datasets.Value("int64"),
+ "query": datasets.Value("int64"),
+ },
+ "prediction_text": datasets.Value("string"),
+ },
+ "references": {
+ "idx": {
+ "passage": datasets.Value("int64"),
+ "query": datasets.Value("int64"),
+ },
+ "answers": datasets.Sequence(datasets.Value("string")),
+ },
+ }
+ elif self.config_name == "multirc":
+ return {
+ "predictions": {
+ "idx": {
+ "answer": datasets.Value("int64"),
+ "paragraph": datasets.Value("int64"),
+ "question": datasets.Value("int64"),
+ },
+ "prediction": datasets.Value("int64"),
+ },
+ "references": datasets.Value("int64"),
+ }
+ else:
+ return {
+ "predictions": datasets.Value("int64"),
+ "references": datasets.Value("int64"),
+ }
+
+ def _compute(self, predictions, references):
+ if self.config_name == "axb":
+ return {"matthews_correlation": matthews_corrcoef(references, predictions)}
+ elif self.config_name == "cb":
+ return acc_and_f1(predictions, references, f1_avg="macro")
+ elif self.config_name == "record":
+ dataset = [
+ {
+ "qas": [
+ {"id": ref["idx"]["query"], "answers": [{"text": ans} for ans in ref["answers"]]}
+ for ref in references
+ ]
+ }
+ ]
+ predictions = {pred["idx"]["query"]: pred["prediction_text"] for pred in predictions}
+ return evaluate_record(dataset, predictions)[0]
+ elif self.config_name == "multirc":
+ return evaluate_multirc(predictions, references)
+ elif self.config_name in ["copa", "rte", "wic", "wsc", "wsc.fixed", "boolq", "axg"]:
+ return {"accuracy": simple_accuracy(predictions, references)}
+ else:
+ raise KeyError(
+ "You should supply a configuration name selected in "
+ '["boolq", "cb", "copa", "multirc", "record", "rte", "wic", "wsc", "wsc.fixed", "axb", "axg",]'
+ )
diff --git a/metrics/ter/README.md b/metrics/ter/README.md
new file mode 100644
index 0000000..ae44bbc
--- /dev/null
+++ b/metrics/ter/README.md
@@ -0,0 +1,150 @@
+# Metric Card for TER
+
+## Metric Description
+TER (Translation Edit Rate, also called Translation Error Rate) is a metric to quantify the edit operations that a hypothesis requires to match a reference translation. We use the implementation that is already present in [sacrebleu](https://github.com/mjpost/sacreBLEU#ter), which in turn is inspired by the [TERCOM implementation](https://github.com/jhclark/tercom).
+
+The implementation here is slightly different from sacrebleu in terms of the required input format. The length of the references and hypotheses lists need to be the same, so you may need to transpose your references compared to sacrebleu's required input format. See [this github issue](https://github.com/huggingface/datasets/issues/3154#issuecomment-950746534).
+
+See the README.md file at https://github.com/mjpost/sacreBLEU#ter for more information.
+
+
+## How to Use
+This metric takes, at minimum, predicted sentences and reference sentences:
+```python
+>>> predictions = ["does this sentence match??",
+... "what about this sentence?",
+... "What did the TER metric user say to the developer?"]
+>>> references = [["does this sentence match", "does this sentence match!?!"],
+... ["wHaT aBoUt ThIs SeNtEnCe?", "wHaT aBoUt ThIs SeNtEnCe?"],
+... ["Your jokes are...", "...TERrible"]]
+>>> ter = datasets.load_metric("ter")
+>>> results = ter.compute(predictions=predictions,
+... references=references,
+... case_sensitive=True)
+>>> print(results)
+{'score': 150.0, 'num_edits': 15, 'ref_length': 10.0}
+```
+
+### Inputs
+This metric takes the following as input:
+- **`predictions`** (`list` of `str`): The system stream (a sequence of segments).
+- **`references`** (`list` of `list` of `str`): A list of one or more reference streams (each a sequence of segments).
+- **`normalized`** (`boolean`): If `True`, applies basic tokenization and normalization to sentences. Defaults to `False`.
+- **`ignore_punct`** (`boolean`): If `True`, applies basic tokenization and normalization to sentences. Defaults to `False`.
+- **`support_zh_ja_chars`** (`boolean`): If `True`, tokenization/normalization supports processing of Chinese characters, as well as Japanese Kanji, Hiragana, Katakana, and Phonetic Extensions of Katakana. Only applies if `normalized = True`. Defaults to `False`.
+- **`case_sensitive`** (`boolean`): If `False`, makes all predictions and references lowercase to ignore differences in case. Defaults to `False`.
+
+### Output Values
+This metric returns the following:
+- **`score`** (`float`): TER score (num_edits / sum_ref_lengths * 100)
+- **`num_edits`** (`int`): The cumulative number of edits
+- **`ref_length`** (`float`): The cumulative average reference length
+
+The output takes the following form:
+```python
+{'score': ter_score, 'num_edits': num_edits, 'ref_length': ref_length}
+```
+
+The metric can take on any value `0` and above. `0` is a perfect score, meaning the predictions exactly match the references and no edits were necessary. Higher scores are worse. Scores above 100 mean that the cumulative number of edits, `num_edits`, is higher than the cumulative length of the references, `ref_length`.
+
+#### Values from Popular Papers
+
+
+### Examples
+Basic example with only predictions and references as inputs:
+```python
+>>> predictions = ["does this sentence match??",
+... "what about this sentence?"]
+>>> references = [["does this sentence match", "does this sentence match!?!"],
+... ["wHaT aBoUt ThIs SeNtEnCe?", "wHaT aBoUt ThIs SeNtEnCe?"]]
+>>> ter = datasets.load_metric("ter")
+>>> results = ter.compute(predictions=predictions,
+... references=references,
+... case_sensitive=True)
+>>> print(results)
+{'score': 62.5, 'num_edits': 5, 'ref_length': 8.0}
+```
+
+Example with `normalization = True`:
+```python
+>>> predictions = ["does this sentence match??",
+... "what about this sentence?"]
+>>> references = [["does this sentence match", "does this sentence match!?!"],
+... ["wHaT aBoUt ThIs SeNtEnCe?", "wHaT aBoUt ThIs SeNtEnCe?"]]
+>>> ter = datasets.load_metric("ter")
+>>> results = ter.compute(predictions=predictions,
+... references=references,
+... normalized=True,
+... case_sensitive=True)
+>>> print(results)
+{'score': 57.14285714285714, 'num_edits': 6, 'ref_length': 10.5}
+```
+
+Example ignoring punctuation and capitalization, and everything matches:
+```python
+>>> predictions = ["does this sentence match??",
+... "what about this sentence?"]
+>>> references = [["does this sentence match", "does this sentence match!?!"],
+... ["wHaT aBoUt ThIs SeNtEnCe?", "wHaT aBoUt ThIs SeNtEnCe?"]]
+>>> ter = datasets.load_metric("ter")
+>>> results = ter.compute(predictions=predictions,
+... references=references,
+... ignore_punct=True,
+... case_sensitive=False)
+>>> print(results)
+{'score': 0.0, 'num_edits': 0, 'ref_length': 8.0}
+```
+
+Example ignoring punctuation and capitalization, but with an extra (incorrect) sample:
+```python
+>>> predictions = ["does this sentence match??",
+... "what about this sentence?",
+... "What did the TER metric user say to the developer?"]
+>>> references = [["does this sentence match", "does this sentence match!?!"],
+... ["wHaT aBoUt ThIs SeNtEnCe?", "wHaT aBoUt ThIs SeNtEnCe?"],
+... ["Your jokes are...", "...TERrible"]]
+>>> ter = datasets.load_metric("ter")
+>>> results = ter.compute(predictions=predictions,
+... references=references,
+... ignore_punct=True,
+... case_sensitive=False)
+>>> print(results)
+{'score': 100.0, 'num_edits': 10, 'ref_length': 10.0}
+```
+
+
+## Limitations and Bias
+
+
+## Citation
+```bibtex
+@inproceedings{snover-etal-2006-study,
+ title = "A Study of Translation Edit Rate with Targeted Human Annotation",
+ author = "Snover, Matthew and
+ Dorr, Bonnie and
+ Schwartz, Rich and
+ Micciulla, Linnea and
+ Makhoul, John",
+ booktitle = "Proceedings of the 7th Conference of the Association for Machine Translation in the Americas: Technical Papers",
+ month = aug # " 8-12",
+ year = "2006",
+ address = "Cambridge, Massachusetts, USA",
+ publisher = "Association for Machine Translation in the Americas",
+ url = "https://aclanthology.org/2006.amta-papers.25",
+ pages = "223--231",
+}
+@inproceedings{post-2018-call,
+ title = "A Call for Clarity in Reporting {BLEU} Scores",
+ author = "Post, Matt",
+ booktitle = "Proceedings of the Third Conference on Machine Translation: Research Papers",
+ month = oct,
+ year = "2018",
+ address = "Belgium, Brussels",
+ publisher = "Association for Computational Linguistics",
+ url = "https://www.aclweb.org/anthology/W18-6319",
+ pages = "186--191",
+}
+```
+
+## Further References
+- See [the sacreBLEU github repo](https://github.com/mjpost/sacreBLEU#ter) for more information.
diff --git a/metrics/ter/ter.py b/metrics/ter/ter.py
new file mode 100644
index 0000000..f369366
--- /dev/null
+++ b/metrics/ter/ter.py
@@ -0,0 +1,199 @@
+# Copyright 2021 The HuggingFace Datasets Authors.
+#
+# Licensed under the Apache License, Version 2.0 (the "License");
+# you may not use this file except in compliance with the License.
+# You may obtain a copy of the License at
+#
+# http://www.apache.org/licenses/LICENSE-2.0
+#
+# Unless required by applicable law or agreed to in writing, software
+# distributed under the License is distributed on an "AS IS" BASIS,
+# WITHOUT WARRANTIES OR CONDITIONS OF ANY KIND, either express or implied.
+# See the License for the specific language governing permissions and
+# limitations under the License.
+""" TER metric as available in sacrebleu. """
+import sacrebleu as scb
+from packaging import version
+from sacrebleu import TER
+
+import datasets
+
+
+_CITATION = """\
+@inproceedings{snover-etal-2006-study,
+ title = "A Study of Translation Edit Rate with Targeted Human Annotation",
+ author = "Snover, Matthew and
+ Dorr, Bonnie and
+ Schwartz, Rich and
+ Micciulla, Linnea and
+ Makhoul, John",
+ booktitle = "Proceedings of the 7th Conference of the Association for Machine Translation in the Americas: Technical Papers",
+ month = aug # " 8-12",
+ year = "2006",
+ address = "Cambridge, Massachusetts, USA",
+ publisher = "Association for Machine Translation in the Americas",
+ url = "https://aclanthology.org/2006.amta-papers.25",
+ pages = "223--231",
+}
+@inproceedings{post-2018-call,
+ title = "A Call for Clarity in Reporting {BLEU} Scores",
+ author = "Post, Matt",
+ booktitle = "Proceedings of the Third Conference on Machine Translation: Research Papers",
+ month = oct,
+ year = "2018",
+ address = "Belgium, Brussels",
+ publisher = "Association for Computational Linguistics",
+ url = "https://www.aclweb.org/anthology/W18-6319",
+ pages = "186--191",
+}
+"""
+
+_DESCRIPTION = """\
+TER (Translation Edit Rate, also called Translation Error Rate) is a metric to quantify the edit operations that a
+hypothesis requires to match a reference translation. We use the implementation that is already present in sacrebleu
+(https://github.com/mjpost/sacreBLEU#ter), which in turn is inspired by the TERCOM implementation, which can be found
+here: https://github.com/jhclark/tercom.
+
+The implementation here is slightly different from sacrebleu in terms of the required input format. The length of
+the references and hypotheses lists need to be the same, so you may need to transpose your references compared to
+sacrebleu's required input format. See https://github.com/huggingface/datasets/issues/3154#issuecomment-950746534
+
+See the README.md file at https://github.com/mjpost/sacreBLEU#ter for more information.
+"""
+
+_KWARGS_DESCRIPTION = """
+Produces TER scores alongside the number of edits and reference length.
+
+Args:
+ predictions (list of str): The system stream (a sequence of segments).
+ references (list of list of str): A list of one or more reference streams (each a sequence of segments).
+ normalized (boolean): If `True`, applies basic tokenization and normalization to sentences. Defaults to `False`.
+ ignore_punct (boolean): If `True`, applies basic tokenization and normalization to sentences. Defaults to `False`.
+ support_zh_ja_chars (boolean): If `True`, tokenization/normalization supports processing of Chinese characters,
+ as well as Japanese Kanji, Hiragana, Katakana, and Phonetic Extensions of Katakana.
+ Only applies if `normalized = True`. Defaults to `False`.
+ case_sensitive (boolean): If `False`, makes all predictions and references lowercase to ignore differences in case. Defaults to `False`.
+
+Returns:
+ 'score' (float): TER score (num_edits / sum_ref_lengths * 100)
+ 'num_edits' (int): The cumulative number of edits
+ 'ref_length' (float): The cumulative average reference length
+
+Examples:
+ Example 1:
+ >>> predictions = ["does this sentence match??",
+ ... "what about this sentence?",
+ ... "What did the TER metric user say to the developer?"]
+ >>> references = [["does this sentence match", "does this sentence match!?!"],
+ ... ["wHaT aBoUt ThIs SeNtEnCe?", "wHaT aBoUt ThIs SeNtEnCe?"],
+ ... ["Your jokes are...", "...TERrible"]]
+ >>> ter = datasets.load_metric("ter")
+ >>> results = ter.compute(predictions=predictions,
+ ... references=references,
+ ... case_sensitive=True)
+ >>> print(results)
+ {'score': 150.0, 'num_edits': 15, 'ref_length': 10.0}
+
+ Example 2:
+ >>> predictions = ["does this sentence match??",
+ ... "what about this sentence?"]
+ >>> references = [["does this sentence match", "does this sentence match!?!"],
+ ... ["wHaT aBoUt ThIs SeNtEnCe?", "wHaT aBoUt ThIs SeNtEnCe?"]]
+ >>> ter = datasets.load_metric("ter")
+ >>> results = ter.compute(predictions=predictions,
+ ... references=references,
+ ... case_sensitive=True)
+ >>> print(results)
+ {'score': 62.5, 'num_edits': 5, 'ref_length': 8.0}
+
+ Example 3:
+ >>> predictions = ["does this sentence match??",
+ ... "what about this sentence?"]
+ >>> references = [["does this sentence match", "does this sentence match!?!"],
+ ... ["wHaT aBoUt ThIs SeNtEnCe?", "wHaT aBoUt ThIs SeNtEnCe?"]]
+ >>> ter = datasets.load_metric("ter")
+ >>> results = ter.compute(predictions=predictions,
+ ... references=references,
+ ... normalized=True,
+ ... case_sensitive=True)
+ >>> print(results)
+ {'score': 57.14285714285714, 'num_edits': 6, 'ref_length': 10.5}
+
+ Example 4:
+ >>> predictions = ["does this sentence match??",
+ ... "what about this sentence?"]
+ >>> references = [["does this sentence match", "does this sentence match!?!"],
+ ... ["wHaT aBoUt ThIs SeNtEnCe?", "wHaT aBoUt ThIs SeNtEnCe?"]]
+ >>> ter = datasets.load_metric("ter")
+ >>> results = ter.compute(predictions=predictions,
+ ... references=references,
+ ... ignore_punct=True,
+ ... case_sensitive=False)
+ >>> print(results)
+ {'score': 0.0, 'num_edits': 0, 'ref_length': 8.0}
+
+ Example 5:
+ >>> predictions = ["does this sentence match??",
+ ... "what about this sentence?",
+ ... "What did the TER metric user say to the developer?"]
+ >>> references = [["does this sentence match", "does this sentence match!?!"],
+ ... ["wHaT aBoUt ThIs SeNtEnCe?", "wHaT aBoUt ThIs SeNtEnCe?"],
+ ... ["Your jokes are...", "...TERrible"]]
+ >>> ter = datasets.load_metric("ter")
+ >>> results = ter.compute(predictions=predictions,
+ ... references=references,
+ ... ignore_punct=True,
+ ... case_sensitive=False)
+ >>> print(results)
+ {'score': 100.0, 'num_edits': 10, 'ref_length': 10.0}
+"""
+
+
+@datasets.utils.file_utils.add_start_docstrings(_DESCRIPTION, _KWARGS_DESCRIPTION)
+class Ter(datasets.Metric):
+ def _info(self):
+ if version.parse(scb.__version__) < version.parse("1.4.12"):
+ raise ImportWarning(
+ "To use `sacrebleu`, the module `sacrebleu>=1.4.12` is required, and the current version of `sacrebleu` doesn't match this condition.\n"
+ 'You can install it with `pip install "sacrebleu>=1.4.12"`.'
+ )
+ return datasets.MetricInfo(
+ description=_DESCRIPTION,
+ citation=_CITATION,
+ homepage="http://www.cs.umd.edu/~snover/tercom/",
+ inputs_description=_KWARGS_DESCRIPTION,
+ features=datasets.Features(
+ {
+ "predictions": datasets.Value("string", id="sequence"),
+ "references": datasets.Sequence(datasets.Value("string", id="sequence"), id="references"),
+ }
+ ),
+ codebase_urls=["https://github.com/mjpost/sacreBLEU#ter"],
+ reference_urls=[
+ "https://github.com/jhclark/tercom",
+ ],
+ )
+
+ def _compute(
+ self,
+ predictions,
+ references,
+ normalized: bool = False,
+ ignore_punct: bool = False,
+ support_zh_ja_chars: bool = False,
+ case_sensitive: bool = False,
+ ):
+ references_per_prediction = len(references[0])
+ if any(len(refs) != references_per_prediction for refs in references):
+ raise ValueError("Sacrebleu requires the same number of references for each prediction")
+ transformed_references = [[refs[i] for refs in references] for i in range(references_per_prediction)]
+
+ sb_ter = TER(
+ normalized=normalized,
+ no_punct=ignore_punct,
+ asian_support=support_zh_ja_chars,
+ case_sensitive=case_sensitive,
+ )
+ output = sb_ter.corpus_score(predictions, transformed_references)
+
+ return {"score": output.score, "num_edits": output.num_edits, "ref_length": output.ref_length}
diff --git a/metrics/wer/README.md b/metrics/wer/README.md
new file mode 100644
index 0000000..ae71505
--- /dev/null
+++ b/metrics/wer/README.md
@@ -0,0 +1,123 @@
+# Metric Card for WER
+
+## Metric description
+Word error rate (WER) is a common metric of the performance of an automatic speech recognition (ASR) system.
+
+The general difficulty of measuring the performance of ASR systems lies in the fact that the recognized word sequence can have a different length from the reference word sequence (supposedly the correct one). The WER is derived from the [Levenshtein distance](https://en.wikipedia.org/wiki/Levenshtein_distance), working at the word level.
+
+This problem is solved by first aligning the recognized word sequence with the reference (spoken) word sequence using dynamic string alignment. Examination of this issue is seen through a theory called the power law that states the correlation between [perplexity](https://huggingface.co/metrics/perplexity) and word error rate (see [this article](https://www.cs.cmu.edu/~roni/papers/eval-metrics-bntuw-9802.pdf) for further information).
+
+Word error rate can then be computed as:
+
+`WER = (S + D + I) / N = (S + D + I) / (S + D + C)`
+
+where
+
+`S` is the number of substitutions,
+
+`D` is the number of deletions,
+
+`I` is the number of insertions,
+
+`C` is the number of correct words,
+
+`N` is the number of words in the reference (`N=S+D+C`).
+
+
+## How to use
+
+The metric takes two inputs: references (a list of references for each speech input) and predictions (a list of transcriptions to score).
+
+
+```python
+from datasets import load_metric
+wer = load_metric("wer")
+wer_score = wer.compute(predictions=predictions, references=references)
+```
+## Output values
+
+This metric outputs a float representing the word error rate.
+
+```
+print(wer_score)
+0.5
+```
+
+This value indicates the average number of errors per reference word.
+
+The **lower** the value, the **better** the performance of the ASR system, with a WER of 0 being a perfect score.
+
+### Values from popular papers
+
+This metric is highly dependent on the content and quality of the dataset, and therefore users can expect very different values for the same model but on different datasets.
+
+For example, datasets such as [LibriSpeech](https://huggingface.co/datasets/librispeech_asr) report a WER in the 1.8-3.3 range, whereas ASR models evaluated on [Timit](https://huggingface.co/datasets/timit_asr) report a WER in the 8.3-20.4 range.
+See the leaderboards for [LibriSpeech](https://paperswithcode.com/sota/speech-recognition-on-librispeech-test-clean) and [Timit](https://paperswithcode.com/sota/speech-recognition-on-timit) for the most recent values.
+
+## Examples
+
+Perfect match between prediction and reference:
+
+```python
+from datasets import load_metric
+wer = load_metric("wer")
+predictions = ["hello world", "good night moon"]
+references = ["hello world", "good night moon"]
+wer_score = wer.compute(predictions=predictions, references=references)
+print(wer_score)
+0.0
+```
+
+Partial match between prediction and reference:
+
+```python
+from datasets import load_metric
+wer = load_metric("wer")
+predictions = ["this is the prediction", "there is an other sample"]
+references = ["this is the reference", "there is another one"]
+wer_score = wer.compute(predictions=predictions, references=references)
+print(wer_score)
+0.5
+```
+
+No match between prediction and reference:
+
+```python
+from datasets import load_metric
+wer = load_metric("wer")
+predictions = ["hello world", "good night moon"]
+references = ["hi everyone", "have a great day"]
+wer_score = wer.compute(predictions=predictions, references=references)
+print(wer_score)
+1.0
+```
+
+## Limitations and bias
+
+WER is a valuable tool for comparing different systems as well as for evaluating improvements within one system. This kind of measurement, however, provides no details on the nature of translation errors and further work is therefore required to identify the main source(s) of error and to focus any research effort.
+
+## Citation
+
+```bibtex
+@inproceedings{woodard1982,
+author = {Woodard, J.P. and Nelson, J.T.,
+year = {1982},
+journal = Ẅorkshop on standardisation for speech I/O technology, Naval Air Development Center, Warminster, PA},
+title = {An information theoretic measure of speech recognition performance}
+}
+```
+
+```bibtex
+@inproceedings{morris2004,
+author = {Morris, Andrew and Maier, Viktoria and Green, Phil},
+year = {2004},
+month = {01},
+pages = {},
+title = {From WER and RIL to MER and WIL: improved evaluation measures for connected speech recognition.}
+}
+```
+
+## Further References
+
+- [Word Error Rate -- Wikipedia](https://en.wikipedia.org/wiki/Word_error_rate)
+- [Hugging Face Tasks -- Automatic Speech Recognition](https://huggingface.co/tasks/automatic-speech-recognition)
diff --git a/metrics/wer/wer.py b/metrics/wer/wer.py
new file mode 100644
index 0000000..e846110
--- /dev/null
+++ b/metrics/wer/wer.py
@@ -0,0 +1,105 @@
+# Copyright 2021 The HuggingFace Datasets Authors.
+#
+# Licensed under the Apache License, Version 2.0 (the "License");
+# you may not use this file except in compliance with the License.
+# You may obtain a copy of the License at
+#
+# http://www.apache.org/licenses/LICENSE-2.0
+#
+# Unless required by applicable law or agreed to in writing, software
+# distributed under the License is distributed on an "AS IS" BASIS,
+# WITHOUT WARRANTIES OR CONDITIONS OF ANY KIND, either express or implied.
+# See the License for the specific language governing permissions and
+# limitations under the License.
+""" Word Error Ratio (WER) metric. """
+
+from jiwer import compute_measures
+
+import datasets
+
+
+_CITATION = """\
+@inproceedings{inproceedings,
+ author = {Morris, Andrew and Maier, Viktoria and Green, Phil},
+ year = {2004},
+ month = {01},
+ pages = {},
+ title = {From WER and RIL to MER and WIL: improved evaluation measures for connected speech recognition.}
+}
+"""
+
+_DESCRIPTION = """\
+Word error rate (WER) is a common metric of the performance of an automatic speech recognition system.
+
+The general difficulty of measuring performance lies in the fact that the recognized word sequence can have a different length from the reference word sequence (supposedly the correct one). The WER is derived from the Levenshtein distance, working at the word level instead of the phoneme level. The WER is a valuable tool for comparing different systems as well as for evaluating improvements within one system. This kind of measurement, however, provides no details on the nature of translation errors and further work is therefore required to identify the main source(s) of error and to focus any research effort.
+
+This problem is solved by first aligning the recognized word sequence with the reference (spoken) word sequence using dynamic string alignment. Examination of this issue is seen through a theory called the power law that states the correlation between perplexity and word error rate.
+
+Word error rate can then be computed as:
+
+WER = (S + D + I) / N = (S + D + I) / (S + D + C)
+
+where
+
+S is the number of substitutions,
+D is the number of deletions,
+I is the number of insertions,
+C is the number of correct words,
+N is the number of words in the reference (N=S+D+C).
+
+This value indicates the average number of errors per reference word. The lower the value, the better the
+performance of the ASR system with a WER of 0 being a perfect score.
+"""
+
+_KWARGS_DESCRIPTION = """
+Compute WER score of transcribed segments against references.
+
+Args:
+ references: List of references for each speech input.
+ predictions: List of transcriptions to score.
+ concatenate_texts (bool, default=False): Whether to concatenate all input texts or compute WER iteratively.
+
+Returns:
+ (float): the word error rate
+
+Examples:
+
+ >>> predictions = ["this is the prediction", "there is an other sample"]
+ >>> references = ["this is the reference", "there is another one"]
+ >>> wer = datasets.load_metric("wer")
+ >>> wer_score = wer.compute(predictions=predictions, references=references)
+ >>> print(wer_score)
+ 0.5
+"""
+
+
+@datasets.utils.file_utils.add_start_docstrings(_DESCRIPTION, _KWARGS_DESCRIPTION)
+class WER(datasets.Metric):
+ def _info(self):
+ return datasets.MetricInfo(
+ description=_DESCRIPTION,
+ citation=_CITATION,
+ inputs_description=_KWARGS_DESCRIPTION,
+ features=datasets.Features(
+ {
+ "predictions": datasets.Value("string", id="sequence"),
+ "references": datasets.Value("string", id="sequence"),
+ }
+ ),
+ codebase_urls=["https://github.com/jitsi/jiwer/"],
+ reference_urls=[
+ "https://en.wikipedia.org/wiki/Word_error_rate",
+ ],
+ )
+
+ def _compute(self, predictions=None, references=None, concatenate_texts=False):
+ if concatenate_texts:
+ return compute_measures(references, predictions)["wer"]
+ else:
+ incorrect = 0
+ total = 0
+ for prediction, reference in zip(predictions, references):
+ measures = compute_measures(reference, prediction)
+ incorrect += measures["substitutions"] + measures["deletions"] + measures["insertions"]
+ total += measures["substitutions"] + measures["deletions"] + measures["hits"]
+ return incorrect / total
diff --git a/metrics/wiki_split/README.md b/metrics/wiki_split/README.md
new file mode 100644
index 0000000..1a9c934
--- /dev/null
+++ b/metrics/wiki_split/README.md
@@ -0,0 +1,107 @@
+# Metric Card for WikiSplit
+
+## Metric description
+
+WikiSplit is the combination of three metrics: [SARI](https://huggingface.co/metrics/sari), [exact match](https://huggingface.co/metrics/exact_match) and [SacreBLEU](https://huggingface.co/metrics/sacrebleu).
+
+It can be used to evaluate the quality of sentence splitting approaches, which require rewriting a long sentence into two or more coherent short sentences, e.g. based on the [WikiSplit dataset](https://huggingface.co/datasets/wiki_split).
+
+## How to use
+
+The WIKI_SPLIT metric takes three inputs:
+
+`sources`: a list of source sentences, where each sentence should be a string.
+
+`predictions`: a list of predicted sentences, where each sentence should be a string.
+
+`references`: a list of lists of reference sentences, where each sentence should be a string.
+
+```python
+>>> from datasets import load_metric
+>>> wiki_split = load_metric("wiki_split")
+>>> sources = ["About 95 species are currently accepted ."]
+>>> predictions = ["About 95 you now get in ."]
+>>> references= [["About 95 species are currently known ."]]
+>>> results = wiki_split.compute(sources=sources, predictions=predictions, references=references)
+```
+## Output values
+
+This metric outputs a dictionary containing three scores:
+
+`sari`: the [SARI](https://huggingface.co/metrics/sari) score, whose range is between `0.0` and `100.0` -- the higher the value, the better the performance of the model being evaluated, with a SARI of 100 being a perfect score.
+
+`sacrebleu`: the [SacreBLEU](https://huggingface.co/metrics/sacrebleu) score, which can take any value between `0.0` and `100.0`, inclusive.
+
+`exact`: the [exact match](https://huggingface.co/metrics/exact_match) score, which represents the sum of all of the individual exact match scores in the set, divided by the total number of predictions in the set. It ranges from `0.0` to `100`, inclusive. Here, `0.0` means no prediction/reference pairs were matches, while `100.0` means they all were.
+
+```python
+>>> print(results)
+{'sari': 21.805555555555557, 'sacrebleu': 14.535768424205482, 'exact': 0.0}
+```
+
+### Values from popular papers
+
+This metric was initially used by [Rothe et al.(2020)](https://arxiv.org/pdf/1907.12461.pdf) to evaluate the performance of different split-and-rephrase approaches on the [WikiSplit dataset](https://huggingface.co/datasets/wiki_split). They reported a SARI score of 63.5, a SacreBLEU score of 77.2, and an EXACT_MATCH score of 16.3.
+
+## Examples
+
+Perfect match between prediction and reference:
+
+```python
+>>> from datasets import load_metric
+>>> wiki_split = load_metric("wiki_split")
+>>> sources = ["About 95 species are currently accepted ."]
+>>> predictions = ["About 95 species are currently accepted ."]
+>>> references= [["About 95 species are currently accepted ."]]
+>>> results = wiki_split.compute(sources=sources, predictions=predictions, references=references)
+>>> print(results)
+{'sari': 100.0, 'sacrebleu': 100.00000000000004, 'exact': 100.0
+```
+
+Partial match between prediction and reference:
+
+```python
+>>> from datasets import load_metric
+>>> wiki_split = load_metric("wiki_split")
+>>> sources = ["About 95 species are currently accepted ."]
+>>> predictions = ["About 95 you now get in ."]
+>>> references= [["About 95 species are currently known ."]]
+>>> results = wiki_split.compute(sources=sources, predictions=predictions, references=references)
+>>> print(results)
+{'sari': 21.805555555555557, 'sacrebleu': 14.535768424205482, 'exact': 0.0}
+```
+
+No match between prediction and reference:
+
+```python
+>>> from datasets import load_metric
+>>> wiki_split = load_metric("wiki_split")
+>>> sources = ["About 95 species are currently accepted ."]
+>>> predictions = ["Hello world ."]
+>>> references= [["About 95 species are currently known ."]]
+>>> results = wiki_split.compute(sources=sources, predictions=predictions, references=references)
+>>> print(results)
+{'sari': 14.047619047619046, 'sacrebleu': 0.0, 'exact': 0.0}
+```
+## Limitations and bias
+
+This metric is not the official metric to evaluate models on the [WikiSplit dataset](https://huggingface.co/datasets/wiki_split). It was initially proposed by [Rothe et al.(2020)](https://arxiv.org/pdf/1907.12461.pdf), whereas the [original paper introducing the WikiSplit dataset (2018)](https://aclanthology.org/D18-1080.pdf) uses different metrics to evaluate performance, such as corpus-level [BLEU](https://huggingface.co/metrics/bleu) and sentence-level BLEU.
+
+## Citation
+
+```bibtex
+@article{rothe2020leveraging,
+ title={Leveraging pre-trained checkpoints for sequence generation tasks},
+ author={Rothe, Sascha and Narayan, Shashi and Severyn, Aliaksei},
+ journal={Transactions of the Association for Computational Linguistics},
+ volume={8},
+ pages={264--280},
+ year={2020},
+ publisher={MIT Press}
+}
+```
+
+## Further References
+
+- [WikiSplit dataset](https://huggingface.co/datasets/wiki_split)
+- [WikiSplit paper (Botha et al., 2018)](https://aclanthology.org/D18-1080.pdf)
diff --git a/metrics/wiki_split/wiki_split.py b/metrics/wiki_split/wiki_split.py
new file mode 100644
index 0000000..f985d4d
--- /dev/null
+++ b/metrics/wiki_split/wiki_split.py
@@ -0,0 +1,354 @@
+# Copyright 2020 The HuggingFace Datasets Authors and the current dataset script contributor.
+#
+# Licensed under the Apache License, Version 2.0 (the "License");
+# you may not use this file except in compliance with the License.
+# You may obtain a copy of the License at
+#
+# http://www.apache.org/licenses/LICENSE-2.0
+#
+# Unless required by applicable law or agreed to in writing, software
+# distributed under the License is distributed on an "AS IS" BASIS,
+# WITHOUT WARRANTIES OR CONDITIONS OF ANY KIND, either express or implied.
+# See the License for the specific language governing permissions and
+# limitations under the License.
+""" WIKI_SPLIT metric."""
+
+import re
+import string
+from collections import Counter
+
+import sacrebleu
+import sacremoses
+from packaging import version
+
+import datasets
+
+
+_CITATION = """
+@inproceedings{xu-etal-2016-optimizing,
+ title = {Optimizing Statistical Machine Translation for Text Simplification},
+ authors={Xu, Wei and Napoles, Courtney and Pavlick, Ellie and Chen, Quanze and Callison-Burch, Chris},
+ journal = {Transactions of the Association for Computational Linguistics},
+ volume = {4},
+ year={2016},
+ url = {https://www.aclweb.org/anthology/Q16-1029},
+ pages = {401--415
+},
+@inproceedings{post-2018-call,
+ title = "A Call for Clarity in Reporting {BLEU} Scores",
+ author = "Post, Matt",
+ booktitle = "Proceedings of the Third Conference on Machine Translation: Research Papers",
+ month = oct,
+ year = "2018",
+ address = "Belgium, Brussels",
+ publisher = "Association for Computational Linguistics",
+ url = "https://www.aclweb.org/anthology/W18-6319",
+ pages = "186--191",
+}
+"""
+
+_DESCRIPTION = """\
+WIKI_SPLIT is the combination of three metrics SARI, EXACT and SACREBLEU
+It can be used to evaluate the quality of machine-generated texts.
+"""
+
+
+_KWARGS_DESCRIPTION = """
+Calculates sari score (between 0 and 100) given a list of source and predicted
+sentences, and a list of lists of reference sentences. It also computes the BLEU score as well as the exact match score.
+Args:
+ sources: list of source sentences where each sentence should be a string.
+ predictions: list of predicted sentences where each sentence should be a string.
+ references: list of lists of reference sentences where each sentence should be a string.
+Returns:
+ sari: sari score
+ sacrebleu: sacrebleu score
+ exact: exact score
+
+Examples:
+ >>> sources=["About 95 species are currently accepted ."]
+ >>> predictions=["About 95 you now get in ."]
+ >>> references=[["About 95 species are currently known ."]]
+ >>> wiki_split = datasets.load_metric("wiki_split")
+ >>> results = wiki_split.compute(sources=sources, predictions=predictions, references=references)
+ >>> print(results)
+ {'sari': 21.805555555555557, 'sacrebleu': 14.535768424205482, 'exact': 0.0}
+"""
+
+
+def normalize_answer(s):
+ """Lower text and remove punctuation, articles and extra whitespace."""
+
+ def remove_articles(text):
+ regex = re.compile(r"\b(a|an|the)\b", re.UNICODE)
+ return re.sub(regex, " ", text)
+
+ def white_space_fix(text):
+ return " ".join(text.split())
+
+ def remove_punc(text):
+ exclude = set(string.punctuation)
+ return "".join(ch for ch in text if ch not in exclude)
+
+ def lower(text):
+ return text.lower()
+
+ return white_space_fix(remove_articles(remove_punc(lower(s))))
+
+
+def compute_exact(a_gold, a_pred):
+ return int(normalize_answer(a_gold) == normalize_answer(a_pred))
+
+
+def compute_em(predictions, references):
+ scores = [any([compute_exact(ref, pred) for ref in refs]) for pred, refs in zip(predictions, references)]
+ return (sum(scores) / len(scores)) * 100
+
+
+def SARIngram(sgrams, cgrams, rgramslist, numref):
+ rgramsall = [rgram for rgrams in rgramslist for rgram in rgrams]
+ rgramcounter = Counter(rgramsall)
+
+ sgramcounter = Counter(sgrams)
+ sgramcounter_rep = Counter()
+ for sgram, scount in sgramcounter.items():
+ sgramcounter_rep[sgram] = scount * numref
+
+ cgramcounter = Counter(cgrams)
+ cgramcounter_rep = Counter()
+ for cgram, ccount in cgramcounter.items():
+ cgramcounter_rep[cgram] = ccount * numref
+
+ # KEEP
+ keepgramcounter_rep = sgramcounter_rep & cgramcounter_rep
+ keepgramcountergood_rep = keepgramcounter_rep & rgramcounter
+ keepgramcounterall_rep = sgramcounter_rep & rgramcounter
+
+ keeptmpscore1 = 0
+ keeptmpscore2 = 0
+ for keepgram in keepgramcountergood_rep:
+ keeptmpscore1 += keepgramcountergood_rep[keepgram] / keepgramcounter_rep[keepgram]
+ # Fix an alleged bug [2] in the keep score computation.
+ # keeptmpscore2 += keepgramcountergood_rep[keepgram] / keepgramcounterall_rep[keepgram]
+ keeptmpscore2 += keepgramcountergood_rep[keepgram]
+ # Define 0/0=1 instead of 0 to give higher scores for predictions that match
+ # a target exactly.
+ keepscore_precision = 1
+ keepscore_recall = 1
+ if len(keepgramcounter_rep) > 0:
+ keepscore_precision = keeptmpscore1 / len(keepgramcounter_rep)
+ if len(keepgramcounterall_rep) > 0:
+ # Fix an alleged bug [2] in the keep score computation.
+ # keepscore_recall = keeptmpscore2 / len(keepgramcounterall_rep)
+ keepscore_recall = keeptmpscore2 / sum(keepgramcounterall_rep.values())
+ keepscore = 0
+ if keepscore_precision > 0 or keepscore_recall > 0:
+ keepscore = 2 * keepscore_precision * keepscore_recall / (keepscore_precision + keepscore_recall)
+
+ # DELETION
+ delgramcounter_rep = sgramcounter_rep - cgramcounter_rep
+ delgramcountergood_rep = delgramcounter_rep - rgramcounter
+ delgramcounterall_rep = sgramcounter_rep - rgramcounter
+ deltmpscore1 = 0
+ deltmpscore2 = 0
+ for delgram in delgramcountergood_rep:
+ deltmpscore1 += delgramcountergood_rep[delgram] / delgramcounter_rep[delgram]
+ deltmpscore2 += delgramcountergood_rep[delgram] / delgramcounterall_rep[delgram]
+ # Define 0/0=1 instead of 0 to give higher scores for predictions that match
+ # a target exactly.
+ delscore_precision = 1
+ if len(delgramcounter_rep) > 0:
+ delscore_precision = deltmpscore1 / len(delgramcounter_rep)
+
+ # ADDITION
+ addgramcounter = set(cgramcounter) - set(sgramcounter)
+ addgramcountergood = set(addgramcounter) & set(rgramcounter)
+ addgramcounterall = set(rgramcounter) - set(sgramcounter)
+
+ addtmpscore = 0
+ for addgram in addgramcountergood:
+ addtmpscore += 1
+
+ # Define 0/0=1 instead of 0 to give higher scores for predictions that match
+ # a target exactly.
+ addscore_precision = 1
+ addscore_recall = 1
+ if len(addgramcounter) > 0:
+ addscore_precision = addtmpscore / len(addgramcounter)
+ if len(addgramcounterall) > 0:
+ addscore_recall = addtmpscore / len(addgramcounterall)
+ addscore = 0
+ if addscore_precision > 0 or addscore_recall > 0:
+ addscore = 2 * addscore_precision * addscore_recall / (addscore_precision + addscore_recall)
+
+ return (keepscore, delscore_precision, addscore)
+
+
+def SARIsent(ssent, csent, rsents):
+ numref = len(rsents)
+
+ s1grams = ssent.split(" ")
+ c1grams = csent.split(" ")
+ s2grams = []
+ c2grams = []
+ s3grams = []
+ c3grams = []
+ s4grams = []
+ c4grams = []
+
+ r1gramslist = []
+ r2gramslist = []
+ r3gramslist = []
+ r4gramslist = []
+ for rsent in rsents:
+ r1grams = rsent.split(" ")
+ r2grams = []
+ r3grams = []
+ r4grams = []
+ r1gramslist.append(r1grams)
+ for i in range(0, len(r1grams) - 1):
+ if i < len(r1grams) - 1:
+ r2gram = r1grams[i] + " " + r1grams[i + 1]
+ r2grams.append(r2gram)
+ if i < len(r1grams) - 2:
+ r3gram = r1grams[i] + " " + r1grams[i + 1] + " " + r1grams[i + 2]
+ r3grams.append(r3gram)
+ if i < len(r1grams) - 3:
+ r4gram = r1grams[i] + " " + r1grams[i + 1] + " " + r1grams[i + 2] + " " + r1grams[i + 3]
+ r4grams.append(r4gram)
+ r2gramslist.append(r2grams)
+ r3gramslist.append(r3grams)
+ r4gramslist.append(r4grams)
+
+ for i in range(0, len(s1grams) - 1):
+ if i < len(s1grams) - 1:
+ s2gram = s1grams[i] + " " + s1grams[i + 1]
+ s2grams.append(s2gram)
+ if i < len(s1grams) - 2:
+ s3gram = s1grams[i] + " " + s1grams[i + 1] + " " + s1grams[i + 2]
+ s3grams.append(s3gram)
+ if i < len(s1grams) - 3:
+ s4gram = s1grams[i] + " " + s1grams[i + 1] + " " + s1grams[i + 2] + " " + s1grams[i + 3]
+ s4grams.append(s4gram)
+
+ for i in range(0, len(c1grams) - 1):
+ if i < len(c1grams) - 1:
+ c2gram = c1grams[i] + " " + c1grams[i + 1]
+ c2grams.append(c2gram)
+ if i < len(c1grams) - 2:
+ c3gram = c1grams[i] + " " + c1grams[i + 1] + " " + c1grams[i + 2]
+ c3grams.append(c3gram)
+ if i < len(c1grams) - 3:
+ c4gram = c1grams[i] + " " + c1grams[i + 1] + " " + c1grams[i + 2] + " " + c1grams[i + 3]
+ c4grams.append(c4gram)
+
+ (keep1score, del1score, add1score) = SARIngram(s1grams, c1grams, r1gramslist, numref)
+ (keep2score, del2score, add2score) = SARIngram(s2grams, c2grams, r2gramslist, numref)
+ (keep3score, del3score, add3score) = SARIngram(s3grams, c3grams, r3gramslist, numref)
+ (keep4score, del4score, add4score) = SARIngram(s4grams, c4grams, r4gramslist, numref)
+ avgkeepscore = sum([keep1score, keep2score, keep3score, keep4score]) / 4
+ avgdelscore = sum([del1score, del2score, del3score, del4score]) / 4
+ avgaddscore = sum([add1score, add2score, add3score, add4score]) / 4
+ finalscore = (avgkeepscore + avgdelscore + avgaddscore) / 3
+ return finalscore
+
+
+def normalize(sentence, lowercase: bool = True, tokenizer: str = "13a", return_str: bool = True):
+
+ # Normalization is requried for the ASSET dataset (one of the primary
+ # datasets in sentence simplification) to allow using space
+ # to split the sentence. Even though Wiki-Auto and TURK datasets,
+ # do not require normalization, we do it for consistency.
+ # Code adapted from the EASSE library [1] written by the authors of the ASSET dataset.
+ # [1] https://github.com/feralvam/easse/blob/580bba7e1378fc8289c663f864e0487188fe8067/easse/utils/preprocessing.py#L7
+
+ if lowercase:
+ sentence = sentence.lower()
+
+ if tokenizer in ["13a", "intl"]:
+ if version.parse(sacrebleu.__version__).major >= 2:
+ normalized_sent = sacrebleu.metrics.bleu._get_tokenizer(tokenizer)()(sentence)
+ else:
+ normalized_sent = sacrebleu.TOKENIZERS[tokenizer]()(sentence)
+ elif tokenizer == "moses":
+ normalized_sent = sacremoses.MosesTokenizer().tokenize(sentence, return_str=True, escape=False)
+ elif tokenizer == "penn":
+ normalized_sent = sacremoses.MosesTokenizer().penn_tokenize(sentence, return_str=True)
+ else:
+ normalized_sent = sentence
+
+ if not return_str:
+ normalized_sent = normalized_sent.split()
+
+ return normalized_sent
+
+
+def compute_sari(sources, predictions, references):
+
+ if not (len(sources) == len(predictions) == len(references)):
+ raise ValueError("Sources length must match predictions and references lengths.")
+ sari_score = 0
+ for src, pred, refs in zip(sources, predictions, references):
+ sari_score += SARIsent(normalize(src), normalize(pred), [normalize(sent) for sent in refs])
+ sari_score = sari_score / len(predictions)
+ return 100 * sari_score
+
+
+def compute_sacrebleu(
+ predictions,
+ references,
+ smooth_method="exp",
+ smooth_value=None,
+ force=False,
+ lowercase=False,
+ use_effective_order=False,
+):
+ references_per_prediction = len(references[0])
+ if any(len(refs) != references_per_prediction for refs in references):
+ raise ValueError("Sacrebleu requires the same number of references for each prediction")
+ transformed_references = [[refs[i] for refs in references] for i in range(references_per_prediction)]
+ output = sacrebleu.corpus_bleu(
+ predictions,
+ transformed_references,
+ smooth_method=smooth_method,
+ smooth_value=smooth_value,
+ force=force,
+ lowercase=lowercase,
+ use_effective_order=use_effective_order,
+ )
+ return output.score
+
+
+@datasets.utils.file_utils.add_start_docstrings(_DESCRIPTION, _KWARGS_DESCRIPTION)
+class WikiSplit(datasets.Metric):
+ def _info(self):
+ return datasets.MetricInfo(
+ description=_DESCRIPTION,
+ citation=_CITATION,
+ inputs_description=_KWARGS_DESCRIPTION,
+ features=datasets.Features(
+ {
+ "predictions": datasets.Value("string", id="sequence"),
+ "references": datasets.Sequence(datasets.Value("string", id="sequence"), id="references"),
+ }
+ ),
+ codebase_urls=[
+ "https://github.com/huggingface/transformers/blob/master/src/transformers/data/metrics/squad_metrics.py",
+ "https://github.com/cocoxu/simplification/blob/master/SARI.py",
+ "https://github.com/tensorflow/tensor2tensor/blob/master/tensor2tensor/utils/sari_hook.py",
+ "https://github.com/mjpost/sacreBLEU",
+ ],
+ reference_urls=[
+ "https://www.aclweb.org/anthology/Q16-1029.pdf",
+ "https://github.com/mjpost/sacreBLEU",
+ "https://en.wikipedia.org/wiki/BLEU",
+ "https://towardsdatascience.com/evaluating-text-output-in-nlp-bleu-at-your-own-risk-e8609665a213",
+ ],
+ )
+
+ def _compute(self, sources, predictions, references):
+ result = {}
+ result.update({"sari": compute_sari(sources=sources, predictions=predictions, references=references)})
+ result.update({"sacrebleu": compute_sacrebleu(predictions=predictions, references=references)})
+ result.update({"exact": compute_em(predictions=predictions, references=references)})
+ return result
diff --git a/metrics/xnli/README.md b/metrics/xnli/README.md
new file mode 100644
index 0000000..889804f
--- /dev/null
+++ b/metrics/xnli/README.md
@@ -0,0 +1,99 @@
+# Metric Card for XNLI
+
+## Metric description
+
+The XNLI metric allows to evaluate a model's score on the [XNLI dataset](https://huggingface.co/datasets/xnli), which is a subset of a few thousand examples from the [MNLI dataset](https://huggingface.co/datasets/glue/viewer/mnli) that have been translated into a 14 different languages, some of which are relatively low resource such as Swahili and Urdu.
+
+As with MNLI, the task is to predict textual entailment (does sentence A imply/contradict/neither sentence B) and is a classification task (given two sentences, predict one of three labels).
+
+## How to use
+
+The XNLI metric is computed based on the `predictions` (a list of predicted labels) and the `references` (a list of ground truth labels).
+
+```python
+from datasets import load_metric
+xnli_metric = load_metric("xnli")
+predictions = [0, 1]
+references = [0, 1]
+results = xnli_metric.compute(predictions=predictions, references=references)
+```
+
+## Output values
+
+The output of the XNLI metric is simply the `accuracy`, i.e. the proportion of correct predictions among the total number of cases processed, with a range between 0 and 1 (see [accuracy](https://huggingface.co/metrics/accuracy) for more information).
+
+### Values from popular papers
+The [original XNLI paper](https://arxiv.org/pdf/1809.05053.pdf) reported accuracies ranging from 59.3 (for `ur`) to 73.7 (for `en`) for the BiLSTM-max model.
+
+For more recent model performance, see the [dataset leaderboard](https://paperswithcode.com/dataset/xnli).
+
+## Examples
+
+Maximal values:
+
+```python
+>>> from datasets import load_metric
+>>> xnli_metric = load_metric("xnli")
+>>> predictions = [0, 1]
+>>> references = [0, 1]
+>>> results = xnli_metric.compute(predictions=predictions, references=references)
+>>> print(results)
+{'accuracy': 1.0}
+```
+
+Minimal values:
+
+```python
+>>> from datasets import load_metric
+>>> xnli_metric = load_metric("xnli")
+>>> predictions = [1, 0]
+>>> references = [0, 1]
+>>> results = xnli_metric.compute(predictions=predictions, references=references)
+>>> print(results)
+{'accuracy': 0.0}
+```
+
+Partial match:
+
+```python
+>>> from datasets import load_metric
+>>> xnli_metric = load_metric("xnli")
+>>> predictions = [1, 0, 1]
+>>> references = [1, 0, 0]
+>>> results = xnli_metric.compute(predictions=predictions, references=references)
+>>> print(results)
+{'accuracy': 0.6666666666666666}
+```
+
+## Limitations and bias
+
+While accuracy alone does give a certain indication of performance, it can be supplemented by error analysis and a better understanding of the model's mistakes on each of the categories represented in the dataset, especially if they are unbalanced.
+
+While the XNLI dataset is multilingual and represents a diversity of languages, in reality, cross-lingual sentence understanding goes beyond translation, given that there are many cultural differences that have an impact on human sentiment annotations. Since the XNLI dataset was obtained by translation based on English sentences, it does not capture these cultural differences.
+
+
+
+## Citation
+
+```bibtex
+@InProceedings{conneau2018xnli,
+ author = "Conneau, Alexis
+ and Rinott, Ruty
+ and Lample, Guillaume
+ and Williams, Adina
+ and Bowman, Samuel R.
+ and Schwenk, Holger
+ and Stoyanov, Veselin",
+ title = "XNLI: Evaluating Cross-lingual Sentence Representations",
+ booktitle = "Proceedings of the 2018 Conference on Empirical Methods
+ in Natural Language Processing",
+ year = "2018",
+ publisher = "Association for Computational Linguistics",
+ location = "Brussels, Belgium",
+}
+```
+
+## Further References
+
+- [XNI Dataset GitHub](https://github.com/facebookresearch/XNLI)
+- [HuggingFace Tasks -- Text Classification](https://huggingface.co/tasks/text-classification)
diff --git a/metrics/xnli/xnli.py b/metrics/xnli/xnli.py
new file mode 100644
index 0000000..4c8d337
--- /dev/null
+++ b/metrics/xnli/xnli.py
@@ -0,0 +1,86 @@
+# Copyright 2020 The HuggingFace Datasets Authors.
+#
+# Licensed under the Apache License, Version 2.0 (the "License");
+# you may not use this file except in compliance with the License.
+# You may obtain a copy of the License at
+#
+# http://www.apache.org/licenses/LICENSE-2.0
+#
+# Unless required by applicable law or agreed to in writing, software
+# distributed under the License is distributed on an "AS IS" BASIS,
+# WITHOUT WARRANTIES OR CONDITIONS OF ANY KIND, either express or implied.
+# See the License for the specific language governing permissions and
+# limitations under the License.
+""" XNLI benchmark metric. """
+
+import datasets
+
+
+_CITATION = """\
+@InProceedings{conneau2018xnli,
+ author = "Conneau, Alexis
+ and Rinott, Ruty
+ and Lample, Guillaume
+ and Williams, Adina
+ and Bowman, Samuel R.
+ and Schwenk, Holger
+ and Stoyanov, Veselin",
+ title = "XNLI: Evaluating Cross-lingual Sentence Representations",
+ booktitle = "Proceedings of the 2018 Conference on Empirical Methods
+ in Natural Language Processing",
+ year = "2018",
+ publisher = "Association for Computational Linguistics",
+ location = "Brussels, Belgium",
+}
+"""
+
+_DESCRIPTION = """\
+XNLI is a subset of a few thousand examples from MNLI which has been translated
+into a 14 different languages (some low-ish resource). As with MNLI, the goal is
+to predict textual entailment (does sentence A imply/contradict/neither sentence
+B) and is a classification task (given two sentences, predict one of three
+labels).
+"""
+
+_KWARGS_DESCRIPTION = """
+Computes XNLI score which is just simple accuracy.
+Args:
+ predictions: Predicted labels.
+ references: Ground truth labels.
+Returns:
+ 'accuracy': accuracy
+Examples:
+
+ >>> predictions = [0, 1]
+ >>> references = [0, 1]
+ >>> xnli_metric = datasets.load_metric("xnli")
+ >>> results = xnli_metric.compute(predictions=predictions, references=references)
+ >>> print(results)
+ {'accuracy': 1.0}
+"""
+
+
+def simple_accuracy(preds, labels):
+ return (preds == labels).mean()
+
+
+@datasets.utils.file_utils.add_start_docstrings(_DESCRIPTION, _KWARGS_DESCRIPTION)
+class Xnli(datasets.Metric):
+ def _info(self):
+ return datasets.MetricInfo(
+ description=_DESCRIPTION,
+ citation=_CITATION,
+ inputs_description=_KWARGS_DESCRIPTION,
+ features=datasets.Features(
+ {
+ "predictions": datasets.Value("int64" if self.config_name != "sts-b" else "float32"),
+ "references": datasets.Value("int64" if self.config_name != "sts-b" else "float32"),
+ }
+ ),
+ codebase_urls=[],
+ reference_urls=[],
+ format="numpy",
+ )
+
+ def _compute(self, predictions, references):
+ return {"accuracy": simple_accuracy(predictions, references)}
diff --git a/metrics/xtreme_s/README.md b/metrics/xtreme_s/README.md
new file mode 100644
index 0000000..80c5239
--- /dev/null
+++ b/metrics/xtreme_s/README.md
@@ -0,0 +1,129 @@
+# Metric Card for XTREME-S
+
+
+## Metric Description
+
+The XTREME-S metric aims to evaluate model performance on the Cross-lingual TRansfer Evaluation of Multilingual Encoders for Speech (XTREME-S) benchmark.
+
+This benchmark was designed to evaluate speech representations across languages, tasks, domains and data regimes. It covers 102 languages from 10+ language families, 3 different domains and 4 task families: speech recognition, translation, classification and retrieval.
+
+## How to Use
+
+There are two steps: (1) loading the XTREME-S metric relevant to the subset of the benchmark being used for evaluation; and (2) calculating the metric.
+
+1. **Loading the relevant XTREME-S metric** : the subsets of XTREME-S are the following: `mls`, `voxpopuli`, `covost2`, `fleurs-asr`, `fleurs-lang_id`, `minds14` and `babel`. More information about the different subsets can be found on the [XTREME-S benchmark page](https://huggingface.co/datasets/google/xtreme_s).
+
+
+```python
+>>> from datasets import load_metric
+>>> xtreme_s_metric = datasets.load_metric('xtreme_s', 'mls')
+```
+
+2. **Calculating the metric**: the metric takes two inputs :
+
+- `predictions`: a list of predictions to score, with each prediction a `str`.
+
+- `references`: a list of lists of references for each translation, with each reference a `str`.
+
+```python
+>>> references = ["it is sunny here", "paper and pen are essentials"]
+>>> predictions = ["it's sunny", "paper pen are essential"]
+>>> results = xtreme_s_metric.compute(predictions=predictions, references=references)
+```
+
+It also has two optional arguments:
+
+- `bleu_kwargs`: a `dict` of keywords to be passed when computing the `bleu` metric for the `covost2` subset. Keywords can be one of `smooth_method`, `smooth_value`, `force`, `lowercase`, `tokenize`, `use_effective_order`.
+
+- `wer_kwargs`: optional dict of keywords to be passed when computing `wer` and `cer`, which are computed for the `mls`, `fleurs-asr`, `voxpopuli`, and `babel` subsets. Keywords are `concatenate_texts`.
+
+## Output values
+
+The output of the metric depends on the XTREME-S subset chosen, consisting of a dictionary that contains one or several of the following metrics:
+
+- `accuracy`: the proportion of correct predictions among the total number of cases processed, with a range between 0 and 1 (see [accuracy](https://huggingface.co/metrics/accuracy) for more information). This is returned for the `fleurs-lang_id` and `minds14` subsets.
+
+- `f1`: the harmonic mean of the precision and recall (see [F1 score](https://huggingface.co/metrics/f1) for more information). Its range is 0-1 -- its lowest possible value is 0, if either the precision or the recall is 0, and its highest possible value is 1.0, which means perfect precision and recall. It is returned for the `minds14` subset.
+
+- `wer`: Word error rate (WER) is a common metric of the performance of an automatic speech recognition system. The lower the value, the better the performance of the ASR system, with a WER of 0 being a perfect score (see [WER score](https://huggingface.co/metrics/wer) for more information). It is returned for the `mls`, `fleurs-asr`, `voxpopuli` and `babel` subsets of the benchmark.
+
+- `cer`: Character error rate (CER) is similar to WER, but operates on character instead of word. The lower the CER value, the better the performance of the ASR system, with a CER of 0 being a perfect score (see [CER score](https://huggingface.co/metrics/cer) for more information). It is returned for the `mls`, `fleurs-asr`, `voxpopuli` and `babel` subsets of the benchmark.
+
+- `bleu`: the BLEU score, calculated according to the SacreBLEU metric approach. It can take any value between 0.0 and 100.0, inclusive, with higher values being better (see [SacreBLEU](https://huggingface.co/metrics/sacrebleu) for more details). This is returned for the `covost2` subset.
+
+
+### Values from popular papers
+The [original XTREME-S paper](https://arxiv.org/pdf/2203.10752.pdf) reported average WERs ranging from 9.2 to 14.6, a BLEU score of 20.6, an accuracy of 73.3 and F1 score of 86.9, depending on the subsets of the dataset tested on.
+
+## Examples
+
+For the `mls` subset (which outputs `wer` and `cer`):
+
+```python
+>>> from datasets import load_metric
+>>> xtreme_s_metric = datasets.load_metric('xtreme_s', 'mls')
+>>> references = ["it is sunny here", "paper and pen are essentials"]
+>>> predictions = ["it's sunny", "paper pen are essential"]
+>>> results = xtreme_s_metric.compute(predictions=predictions, references=references)
+>>> print({k: round(v, 2) for k, v in results.items()})
+{'wer': 0.56, 'cer': 0.27}
+```
+
+For the `covost2` subset (which outputs `bleu`):
+
+```python
+>>> from datasets import load_metric
+>>> xtreme_s_metric = datasets.load_metric('xtreme_s', 'covost2')
+>>> references = ["bonjour paris", "il est necessaire de faire du sport de temps en temp"]
+>>> predictions = ["bonjour paris", "il est important de faire du sport souvent"]
+>>> results = xtreme_s_metric.compute(predictions=predictions, references=references)
+>>> print({k: round(v, 2) for k, v in results.items()})
+{'bleu': 31.65}
+```
+
+For the `fleurs-lang_id` subset (which outputs `accuracy`):
+
+```python
+>>> from datasets import load_metric
+>>> xtreme_s_metric = datasets.load_metric('xtreme_s', 'fleurs-lang_id')
+>>> references = [0, 1, 0, 0, 1]
+>>> predictions = [0, 1, 1, 0, 0]
+>>> results = xtreme_s_metric.compute(predictions=predictions, references=references)
+>>> print({k: round(v, 2) for k, v in results.items()})
+{'accuracy': 0.6}
+ ```
+
+For the `minds14` subset (which outputs `f1` and `accuracy`):
+
+```python
+>>> from datasets import load_metric
+>>> xtreme_s_metric = datasets.load_metric('xtreme_s', 'minds14')
+>>> references = [0, 1, 0, 0, 1]
+>>> predictions = [0, 1, 1, 0, 0]
+>>> results = xtreme_s_metric.compute(predictions=predictions, references=references)
+>>> print({k: round(v, 2) for k, v in results.items()})
+{'f1': 0.58, 'accuracy': 0.6}
+```
+
+## Limitations and bias
+This metric works only with datasets that have the same format as the [XTREME-S dataset](https://huggingface.co/datasets/google/xtreme_s).
+
+While the XTREME-S dataset is meant to represent a variety of languages and tasks, it has inherent biases: it is missing many languages that are important and under-represented in NLP datasets.
+
+It also has a particular focus on read-speech because common evaluation benchmarks like CoVoST-2 or LibriSpeech evaluate on this type of speech, which results in a mismatch between performance obtained in a read-speech setting and a more noisy setting (in production or live deployment, for instance).
+
+## Citation
+
+```bibtex
+@article{conneau2022xtreme,
+ title={XTREME-S: Evaluating Cross-lingual Speech Representations},
+ author={Conneau, Alexis and Bapna, Ankur and Zhang, Yu and Ma, Min and von Platen, Patrick and Lozhkov, Anton and Cherry, Colin and Jia, Ye and Rivera, Clara and Kale, Mihir and others},
+ journal={arXiv preprint arXiv:2203.10752},
+ year={2022}
+}
+```
+
+## Further References
+
+- [XTREME-S dataset](https://huggingface.co/datasets/google/xtreme_s)
+- [XTREME-S github repository](https://github.com/google-research/xtreme)
diff --git a/metrics/xtreme_s/xtreme_s.py b/metrics/xtreme_s/xtreme_s.py
new file mode 100644
index 0000000..74083b1
--- /dev/null
+++ b/metrics/xtreme_s/xtreme_s.py
@@ -0,0 +1,270 @@
+# Copyright 2022 The HuggingFace Datasets Authors.
+#
+# Licensed under the Apache License, Version 2.0 (the "License");
+# you may not use this file except in compliance with the License.
+# You may obtain a copy of the License at
+#
+# http://www.apache.org/licenses/LICENSE-2.0
+#
+# Unless required by applicable law or agreed to in writing, software
+# distributed under the License is distributed on an "AS IS" BASIS,
+# WITHOUT WARRANTIES OR CONDITIONS OF ANY KIND, either express or implied.
+# See the License for the specific language governing permissions and
+# limitations under the License.
+""" XTREME-S benchmark metric. """
+
+from typing import List
+
+from packaging import version
+from sklearn.metrics import f1_score
+
+import datasets
+from datasets.config import PY_VERSION
+
+
+if PY_VERSION < version.parse("3.8"):
+ import importlib_metadata
+else:
+ import importlib.metadata as importlib_metadata
+
+
+# TODO(Patrick/Anton)
+_CITATION = """\
+"""
+
+_DESCRIPTION = """\
+ XTREME-S is a benchmark to evaluate universal cross-lingual speech representations in many languages.
+ XTREME-S covers four task families: speech recognition, classification, speech-to-text translation and retrieval.
+"""
+
+_KWARGS_DESCRIPTION = """
+Compute XTREME-S evaluation metric associated to each XTREME-S dataset.
+Args:
+ predictions: list of predictions to score.
+ Each translation should be tokenized into a list of tokens.
+ references: list of lists of references for each translation.
+ Each reference should be tokenized into a list of tokens.
+ bleu_kwargs: optional dict of keywords to be passed when computing 'bleu'.
+ Keywords include Dict can be one of 'smooth_method', 'smooth_value', 'force', 'lowercase',
+ 'tokenize', 'use_effective_order'.
+ wer_kwargs: optional dict of keywords to be passed when computing 'wer' and 'cer'.
+ Keywords include 'concatenate_texts'.
+Returns: depending on the XTREME-S task, one or several of:
+ "accuracy": Accuracy - for 'fleurs-lang_id', 'minds14'
+ "f1": F1 score - for 'minds14'
+ "wer": Word error rate - for 'mls', 'fleurs-asr', 'voxpopuli', 'babel'
+ "cer": Character error rate - for 'mls', 'fleurs-asr', 'voxpopuli', 'babel'
+ "bleu": BLEU score according to the `sacrebleu` metric - for 'covost2'
+Examples:
+
+ >>> xtreme_s_metric = datasets.load_metric('xtreme_s', 'mls') # 'mls', 'voxpopuli', 'fleurs-asr' or 'babel'
+ >>> references = ["it is sunny here", "paper and pen are essentials"]
+ >>> predictions = ["it's sunny", "paper pen are essential"]
+ >>> results = xtreme_s_metric.compute(predictions=predictions, references=references)
+ >>> print({k: round(v, 2) for k, v in results.items()})
+ {'wer': 0.56, 'cer': 0.27}
+
+ >>> xtreme_s_metric = datasets.load_metric('xtreme_s', 'covost2')
+ >>> references = ["bonjour paris", "il est necessaire de faire du sport de temps en temp"]
+ >>> predictions = ["bonjour paris", "il est important de faire du sport souvent"]
+ >>> results = xtreme_s_metric.compute(predictions=predictions, references=references)
+ >>> print({k: round(v, 2) for k, v in results.items()})
+ {'bleu': 31.65}
+
+ >>> xtreme_s_metric = datasets.load_metric('xtreme_s', 'fleurs-lang_id')
+ >>> references = [0, 1, 0, 0, 1]
+ >>> predictions = [0, 1, 1, 0, 0]
+ >>> results = xtreme_s_metric.compute(predictions=predictions, references=references)
+ >>> print({k: round(v, 2) for k, v in results.items()})
+ {'accuracy': 0.6}
+
+ >>> xtreme_s_metric = datasets.load_metric('xtreme_s', 'minds14')
+ >>> references = [0, 1, 0, 0, 1]
+ >>> predictions = [0, 1, 1, 0, 0]
+ >>> results = xtreme_s_metric.compute(predictions=predictions, references=references)
+ >>> print({k: round(v, 2) for k, v in results.items()})
+ {'f1': 0.58, 'accuracy': 0.6}
+"""
+
+_CONFIG_NAMES = ["fleurs-asr", "mls", "voxpopuli", "babel", "covost2", "fleurs-lang_id", "minds14"]
+SENTENCE_DELIMITER = ""
+
+try:
+ from jiwer import transforms as tr
+
+ _jiwer_available = True
+except ImportError:
+ _jiwer_available = False
+
+if _jiwer_available and version.parse(importlib_metadata.version("jiwer")) < version.parse("2.3.0"):
+
+ class SentencesToListOfCharacters(tr.AbstractTransform):
+ def __init__(self, sentence_delimiter: str = " "):
+ self.sentence_delimiter = sentence_delimiter
+
+ def process_string(self, s: str):
+ return list(s)
+
+ def process_list(self, inp: List[str]):
+ chars = []
+ for sent_idx, sentence in enumerate(inp):
+ chars.extend(self.process_string(sentence))
+ if self.sentence_delimiter is not None and self.sentence_delimiter != "" and sent_idx < len(inp) - 1:
+ chars.append(self.sentence_delimiter)
+ return chars
+
+ cer_transform = tr.Compose(
+ [tr.RemoveMultipleSpaces(), tr.Strip(), SentencesToListOfCharacters(SENTENCE_DELIMITER)]
+ )
+elif _jiwer_available:
+ cer_transform = tr.Compose(
+ [
+ tr.RemoveMultipleSpaces(),
+ tr.Strip(),
+ tr.ReduceToSingleSentence(SENTENCE_DELIMITER),
+ tr.ReduceToListOfListOfChars(),
+ ]
+ )
+else:
+ cer_transform = None
+
+
+def simple_accuracy(preds, labels):
+ return float((preds == labels).mean())
+
+
+def f1_and_simple_accuracy(preds, labels):
+ return {
+ "f1": float(f1_score(y_true=labels, y_pred=preds, average="macro")),
+ "accuracy": simple_accuracy(preds, labels),
+ }
+
+
+def bleu(
+ preds,
+ labels,
+ smooth_method="exp",
+ smooth_value=None,
+ force=False,
+ lowercase=False,
+ tokenize=None,
+ use_effective_order=False,
+):
+ # xtreme-s can only have one label
+ labels = [[label] for label in labels]
+ preds = list(preds)
+ try:
+ import sacrebleu as scb
+ except ImportError:
+ raise ValueError(
+ "sacrebleu has to be installed in order to apply the bleu metric for covost2."
+ "You can install it via `pip install sacrebleu`."
+ )
+
+ if version.parse(scb.__version__) < version.parse("1.4.12"):
+ raise ImportWarning(
+ "To use `sacrebleu`, the module `sacrebleu>=1.4.12` is required, and the current version of `sacrebleu` doesn't match this condition.\n"
+ 'You can install it with `pip install "sacrebleu>=1.4.12"`.'
+ )
+
+ references_per_prediction = len(labels[0])
+ if any(len(refs) != references_per_prediction for refs in labels):
+ raise ValueError("Sacrebleu requires the same number of references for each prediction")
+ transformed_references = [[refs[i] for refs in labels] for i in range(references_per_prediction)]
+ output = scb.corpus_bleu(
+ preds,
+ transformed_references,
+ smooth_method=smooth_method,
+ smooth_value=smooth_value,
+ force=force,
+ lowercase=lowercase,
+ use_effective_order=use_effective_order,
+ **(dict(tokenize=tokenize) if tokenize else {}),
+ )
+ return {"bleu": output.score}
+
+
+def wer_and_cer(preds, labels, concatenate_texts, config_name):
+ try:
+ from jiwer import compute_measures
+ except ImportError:
+ raise ValueError(
+ f"jiwer has to be installed in order to apply the wer metric for {config_name}."
+ "You can install it via `pip install jiwer`."
+ )
+
+ if concatenate_texts:
+ wer = compute_measures(labels, preds)["wer"]
+
+ cer = compute_measures(labels, preds, truth_transform=cer_transform, hypothesis_transform=cer_transform)["wer"]
+ return {"wer": wer, "cer": cer}
+ else:
+
+ def compute_score(preds, labels, score_type="wer"):
+ incorrect = 0
+ total = 0
+ for prediction, reference in zip(preds, labels):
+ if score_type == "wer":
+ measures = compute_measures(reference, prediction)
+ elif score_type == "cer":
+ measures = compute_measures(
+ reference, prediction, truth_transform=cer_transform, hypothesis_transform=cer_transform
+ )
+ incorrect += measures["substitutions"] + measures["deletions"] + measures["insertions"]
+ total += measures["substitutions"] + measures["deletions"] + measures["hits"]
+ return incorrect / total
+
+ return {"wer": compute_score(preds, labels, "wer"), "cer": compute_score(preds, labels, "cer")}
+
+
+@datasets.utils.file_utils.add_start_docstrings(_DESCRIPTION, _KWARGS_DESCRIPTION)
+class XtremeS(datasets.Metric):
+ def _info(self):
+ if self.config_name not in _CONFIG_NAMES:
+ raise KeyError(f"You should supply a configuration name selected in {_CONFIG_NAMES}")
+
+ pred_type = "int64" if self.config_name in ["fleurs-lang_id", "minds14"] else "string"
+
+ return datasets.MetricInfo(
+ description=_DESCRIPTION,
+ citation=_CITATION,
+ inputs_description=_KWARGS_DESCRIPTION,
+ features=datasets.Features(
+ {"predictions": datasets.Value(pred_type), "references": datasets.Value(pred_type)}
+ ),
+ codebase_urls=[],
+ reference_urls=[],
+ format="numpy",
+ )
+
+ def _compute(self, predictions, references, bleu_kwargs=None, wer_kwargs=None):
+
+ bleu_kwargs = bleu_kwargs if bleu_kwargs is not None else {}
+ wer_kwargs = wer_kwargs if wer_kwargs is not None else {}
+
+ if self.config_name == "fleurs-lang_id":
+ return {"accuracy": simple_accuracy(predictions, references)}
+ elif self.config_name == "minds14":
+ return f1_and_simple_accuracy(predictions, references)
+ elif self.config_name == "covost2":
+ smooth_method = bleu_kwargs.pop("smooth_method", "exp")
+ smooth_value = bleu_kwargs.pop("smooth_value", None)
+ force = bleu_kwargs.pop("force", False)
+ lowercase = bleu_kwargs.pop("lowercase", False)
+ tokenize = bleu_kwargs.pop("tokenize", None)
+ use_effective_order = bleu_kwargs.pop("use_effective_order", False)
+ return bleu(
+ preds=predictions,
+ labels=references,
+ smooth_method=smooth_method,
+ smooth_value=smooth_value,
+ force=force,
+ lowercase=lowercase,
+ tokenize=tokenize,
+ use_effective_order=use_effective_order,
+ )
+ elif self.config_name in ["fleurs-asr", "mls", "voxpopuli", "babel"]:
+ concatenate_texts = wer_kwargs.pop("concatenate_texts", False)
+ return wer_and_cer(predictions, references, concatenate_texts, self.config_name)
+ else:
+ raise KeyError(f"You should supply a configuration name selected in {_CONFIG_NAMES}")
diff --git a/model/__pycache__/model_adaptation.cpython-39.pyc b/model/__pycache__/model_adaptation.cpython-39.pyc
new file mode 100644
index 0000000..a9a3d15
Binary files /dev/null and b/model/__pycache__/model_adaptation.cpython-39.pyc differ
diff --git a/model/deberta.py b/model/deberta.py
new file mode 100644
index 0000000..576229f
--- /dev/null
+++ b/model/deberta.py
@@ -0,0 +1,1434 @@
+# coding=utf-8
+# Copyright 2020 Microsoft and the Hugging Face Inc. team.
+#
+# Licensed under the Apache License, Version 2.0 (the "License");
+# you may not use this file except in compliance with the License.
+# You may obtain a copy of the License at
+#
+# http://www.apache.org/licenses/LICENSE-2.0
+#
+# Unless required by applicable law or agreed to in writing, software
+# distributed under the License is distributed on an "AS IS" BASIS,
+# WITHOUT WARRANTIES OR CONDITIONS OF ANY KIND, either express or implied.
+# See the License for the specific language governing permissions and
+# limitations under the License.
+""" PyTorch DeBERTa model. """
+
+import math
+from collections.abc import Sequence
+
+import torch
+from torch import _softmax_backward_data, nn
+from torch.nn import CrossEntropyLoss
+
+from transformers.activations import ACT2FN
+from transformers.file_utils import add_code_sample_docstrings, add_start_docstrings, add_start_docstrings_to_model_forward
+from transformers.modeling_outputs import (
+ BaseModelOutput,
+ MaskedLMOutput,
+ QuestionAnsweringModelOutput,
+ SequenceClassifierOutput,
+ TokenClassifierOutput,
+)
+from transformers.modeling_utils import PreTrainedModel
+from transformers.utils import logging
+from transformers.models.deberta.configuration_deberta import DebertaConfig
+
+
+logger = logging.get_logger(__name__)
+
+_CONFIG_FOR_DOC = "DebertaConfig"
+_TOKENIZER_FOR_DOC = "DebertaTokenizer"
+_CHECKPOINT_FOR_DOC = "microsoft/deberta-base"
+
+DEBERTA_PRETRAINED_MODEL_ARCHIVE_LIST = [
+ "microsoft/deberta-base",
+ "microsoft/deberta-large",
+ "microsoft/deberta-xlarge",
+ "microsoft/deberta-base-mnli",
+ "microsoft/deberta-large-mnli",
+ "microsoft/deberta-xlarge-mnli",
+]
+
+
+class ContextPooler(nn.Module):
+ def __init__(self, config):
+ super().__init__()
+ self.dense = nn.Linear(config.pooler_hidden_size, config.pooler_hidden_size)
+ self.dropout = StableDropout(config.pooler_dropout)
+ self.config = config
+
+ def forward(self, hidden_states):
+ # We "pool" the model by simply taking the hidden state corresponding
+ # to the first token.
+
+ context_token = hidden_states[:, 0]
+ context_token = self.dropout(context_token)
+ pooled_output = self.dense(context_token)
+ pooled_output = ACT2FN[self.config.pooler_hidden_act](pooled_output)
+ return pooled_output
+
+ @property
+ def output_dim(self):
+ return self.config.hidden_size
+
+
+class XSoftmax(torch.autograd.Function):
+ """
+ Masked Softmax which is optimized for saving memory
+
+ Args:
+ input (:obj:`torch.tensor`): The input tensor that will apply softmax.
+ mask (:obj:`torch.IntTensor`): The mask matrix where 0 indicate that element will be ignored in the softmax calculation.
+ dim (int): The dimension that will apply softmax
+
+ Example::
+
+ >>> import torch
+ >>> from transformers.models.deberta.modeling_deberta import XSoftmax
+
+ >>> # Make a tensor
+ >>> x = torch.randn([4,20,100])
+
+ >>> # Create a mask
+ >>> mask = (x>0).int()
+
+ >>> y = XSoftmax.apply(x, mask, dim=-1)
+ """
+
+ @staticmethod
+ def forward(self, input, mask, dim):
+ self.dim = dim
+ rmask = ~(mask.bool())
+
+ output = input.masked_fill(rmask, float("-inf"))
+ output = torch.softmax(output, self.dim)
+ output.masked_fill_(rmask, 0)
+ self.save_for_backward(output)
+ return output
+
+ @staticmethod
+ def backward(self, grad_output):
+ (output,) = self.saved_tensors
+ inputGrad = _softmax_backward_data(grad_output, output, self.dim, output)
+ return inputGrad, None, None
+
+
+class DropoutContext(object):
+ def __init__(self):
+ self.dropout = 0
+ self.mask = None
+ self.scale = 1
+ self.reuse_mask = True
+
+
+def get_mask(input, local_context):
+ if not isinstance(local_context, DropoutContext):
+ dropout = local_context
+ mask = None
+ else:
+ dropout = local_context.dropout
+ dropout *= local_context.scale
+ mask = local_context.mask if local_context.reuse_mask else None
+
+ if dropout > 0 and mask is None:
+ mask = (1 - torch.empty_like(input).bernoulli_(1 - dropout)).bool()
+
+ if isinstance(local_context, DropoutContext):
+ if local_context.mask is None:
+ local_context.mask = mask
+
+ return mask, dropout
+
+
+class XDropout(torch.autograd.Function):
+ """Optimized dropout function to save computation and memory by using mask operation instead of multiplication."""
+
+ @staticmethod
+ def forward(ctx, input, local_ctx):
+ mask, dropout = get_mask(input, local_ctx)
+ ctx.scale = 1.0 / (1 - dropout)
+ if dropout > 0:
+ ctx.save_for_backward(mask)
+ return input.masked_fill(mask, 0) * ctx.scale
+ else:
+ return input
+
+ @staticmethod
+ def backward(ctx, grad_output):
+ if ctx.scale > 1:
+ (mask,) = ctx.saved_tensors
+ return grad_output.masked_fill(mask, 0) * ctx.scale, None
+ else:
+ return grad_output, None
+
+
+class StableDropout(nn.Module):
+ """
+ Optimized dropout module for stabilizing the training
+
+ Args:
+ drop_prob (float): the dropout probabilities
+ """
+
+ def __init__(self, drop_prob):
+ super().__init__()
+ self.drop_prob = drop_prob
+ self.count = 0
+ self.context_stack = None
+
+ def forward(self, x):
+ """
+ Call the module
+
+ Args:
+ x (:obj:`torch.tensor`): The input tensor to apply dropout
+ """
+ if self.training and self.drop_prob > 0:
+ return XDropout.apply(x, self.get_context())
+ return x
+
+ def clear_context(self):
+ self.count = 0
+ self.context_stack = None
+
+ def init_context(self, reuse_mask=True, scale=1):
+ if self.context_stack is None:
+ self.context_stack = []
+ self.count = 0
+ for c in self.context_stack:
+ c.reuse_mask = reuse_mask
+ c.scale = scale
+
+ def get_context(self):
+ if self.context_stack is not None:
+ if self.count >= len(self.context_stack):
+ self.context_stack.append(DropoutContext())
+ ctx = self.context_stack[self.count]
+ ctx.dropout = self.drop_prob
+ self.count += 1
+ return ctx
+ else:
+ return self.drop_prob
+
+
+class DebertaLayerNorm(nn.Module):
+ """LayerNorm module in the TF style (epsilon inside the square root)."""
+
+ def __init__(self, size, eps=1e-12):
+ super().__init__()
+ self.weight = nn.Parameter(torch.ones(size))
+ self.bias = nn.Parameter(torch.zeros(size))
+ self.variance_epsilon = eps
+
+ def forward(self, hidden_states):
+ input_type = hidden_states.dtype
+ hidden_states = hidden_states.float()
+ mean = hidden_states.mean(-1, keepdim=True)
+ variance = (hidden_states - mean).pow(2).mean(-1, keepdim=True)
+ hidden_states = (hidden_states - mean) / torch.sqrt(variance + self.variance_epsilon)
+ hidden_states = hidden_states.to(input_type)
+ y = self.weight * hidden_states + self.bias
+ return y
+
+
+class DebertaSelfOutput(nn.Module):
+ def __init__(self, config):
+ super().__init__()
+ self.dense = nn.Linear(config.hidden_size, config.hidden_size)
+ self.LayerNorm = DebertaLayerNorm(config.hidden_size, config.layer_norm_eps)
+ self.dropout = StableDropout(config.hidden_dropout_prob)
+
+ def forward(self, hidden_states, input_tensor):
+ hidden_states = self.dense(hidden_states)
+ hidden_states = self.dropout(hidden_states)
+ hidden_states = self.LayerNorm(hidden_states + input_tensor)
+ return hidden_states
+
+
+class DebertaAttention(nn.Module):
+ def __init__(self, config):
+ super().__init__()
+ self.self = DisentangledSelfAttention(config)
+ self.output = DebertaSelfOutput(config)
+ self.config = config
+
+ def forward(
+ self,
+ hidden_states,
+ attention_mask,
+ return_att=False,
+ query_states=None,
+ relative_pos=None,
+ rel_embeddings=None,
+ past_key_value=None,
+ ):
+ self_output = self.self(
+ hidden_states,
+ attention_mask,
+ return_att,
+ query_states=query_states,
+ relative_pos=relative_pos,
+ rel_embeddings=rel_embeddings,
+ past_key_value=past_key_value,
+ )
+ if return_att:
+ self_output, att_matrix = self_output
+ if query_states is None:
+ query_states = hidden_states
+ attention_output = self.output(self_output, query_states)
+
+ if return_att:
+ return (attention_output, att_matrix)
+ else:
+ return attention_output
+
+
+# Copied from transformers.models.bert.modeling_bert.BertIntermediate with Bert->Deberta
+class DebertaIntermediate(nn.Module):
+ def __init__(self, config):
+ super().__init__()
+ self.dense = nn.Linear(config.hidden_size, config.intermediate_size)
+ if isinstance(config.hidden_act, str):
+ self.intermediate_act_fn = ACT2FN[config.hidden_act]
+ else:
+ self.intermediate_act_fn = config.hidden_act
+
+ def forward(self, hidden_states):
+ hidden_states = self.dense(hidden_states)
+ hidden_states = self.intermediate_act_fn(hidden_states)
+ return hidden_states
+
+
+class DebertaOutput(nn.Module):
+ def __init__(self, config):
+ super().__init__()
+ self.dense = nn.Linear(config.intermediate_size, config.hidden_size)
+ self.LayerNorm = DebertaLayerNorm(config.hidden_size, config.layer_norm_eps)
+ self.dropout = StableDropout(config.hidden_dropout_prob)
+ self.config = config
+
+ def forward(self, hidden_states, input_tensor):
+ hidden_states = self.dense(hidden_states)
+ hidden_states = self.dropout(hidden_states)
+ hidden_states = self.LayerNorm(hidden_states + input_tensor)
+ return hidden_states
+
+
+class DebertaLayer(nn.Module):
+ def __init__(self, config):
+ super().__init__()
+ self.attention = DebertaAttention(config)
+ self.intermediate = DebertaIntermediate(config)
+ self.output = DebertaOutput(config)
+
+ def forward(
+ self,
+ hidden_states,
+ attention_mask,
+ return_att=False,
+ query_states=None,
+ relative_pos=None,
+ rel_embeddings=None,
+ past_key_value=None,
+ ):
+ attention_output = self.attention(
+ hidden_states,
+ attention_mask,
+ return_att=return_att,
+ query_states=query_states,
+ relative_pos=relative_pos,
+ rel_embeddings=rel_embeddings,
+ past_key_value=past_key_value,
+ )
+ if return_att:
+ attention_output, att_matrix = attention_output
+ intermediate_output = self.intermediate(attention_output)
+ layer_output = self.output(intermediate_output, attention_output)
+ if return_att:
+ return (layer_output, att_matrix)
+ else:
+ return layer_output
+
+
+class DebertaEncoder(nn.Module):
+ """Modified BertEncoder with relative position bias support"""
+
+ def __init__(self, config):
+ super().__init__()
+ self.layer = nn.ModuleList([DebertaLayer(config) for _ in range(config.num_hidden_layers)])
+ self.relative_attention = getattr(config, "relative_attention", False)
+ if self.relative_attention:
+ self.max_relative_positions = getattr(config, "max_relative_positions", -1)
+ if self.max_relative_positions < 1:
+ self.max_relative_positions = config.max_position_embeddings
+ self.rel_embeddings = nn.Embedding(self.max_relative_positions * 2, config.hidden_size)
+
+ def get_rel_embedding(self):
+ rel_embeddings = self.rel_embeddings.weight if self.relative_attention else None
+ return rel_embeddings
+
+ def get_attention_mask(self, attention_mask):
+ if attention_mask.dim() <= 2:
+ extended_attention_mask = attention_mask.unsqueeze(1).unsqueeze(2)
+ attention_mask = extended_attention_mask * extended_attention_mask.squeeze(-2).unsqueeze(-1)
+ attention_mask = attention_mask.byte()
+ elif attention_mask.dim() == 3:
+ attention_mask = attention_mask.unsqueeze(1)
+
+ return attention_mask
+
+ def get_rel_pos(self, hidden_states, query_states=None, relative_pos=None):
+ if self.relative_attention and relative_pos is None:
+ q = query_states.size(-2) if query_states is not None else hidden_states.size(-2)
+ relative_pos = build_relative_position(q, hidden_states.size(-2), hidden_states.device)
+ return relative_pos
+
+ def forward(
+ self,
+ hidden_states,
+ attention_mask,
+ output_hidden_states=True,
+ output_attentions=False,
+ query_states=None,
+ relative_pos=None,
+ return_dict=True,
+ past_key_values=None,
+ ):
+ attention_mask = self.get_attention_mask(attention_mask)
+ relative_pos = self.get_rel_pos(hidden_states, query_states, relative_pos)
+
+ all_hidden_states = () if output_hidden_states else None
+ all_attentions = () if output_attentions else None
+
+ if isinstance(hidden_states, Sequence):
+ next_kv = hidden_states[0]
+ else:
+ next_kv = hidden_states
+ rel_embeddings = self.get_rel_embedding()
+ for i, layer_module in enumerate(self.layer):
+
+ if output_hidden_states:
+ all_hidden_states = all_hidden_states + (hidden_states,)
+
+ past_key_value = past_key_values[i] if past_key_values is not None else None
+
+ hidden_states = layer_module(
+ next_kv,
+ attention_mask,
+ output_attentions,
+ query_states=query_states,
+ relative_pos=relative_pos,
+ rel_embeddings=rel_embeddings,
+ past_key_value=past_key_value,
+ )
+ if output_attentions:
+ hidden_states, att_m = hidden_states
+
+ if query_states is not None:
+ query_states = hidden_states
+ if isinstance(hidden_states, Sequence):
+ next_kv = hidden_states[i + 1] if i + 1 < len(self.layer) else None
+ else:
+ next_kv = hidden_states
+
+ if output_attentions:
+ all_attentions = all_attentions + (att_m,)
+
+ if output_hidden_states:
+ all_hidden_states = all_hidden_states + (hidden_states,)
+
+ if not return_dict:
+ return tuple(v for v in [hidden_states, all_hidden_states, all_attentions] if v is not None)
+ return BaseModelOutput(
+ last_hidden_state=hidden_states, hidden_states=all_hidden_states, attentions=all_attentions
+ )
+
+
+def build_relative_position(query_size, key_size, device):
+ """
+ Build relative position according to the query and key
+
+ We assume the absolute position of query :math:`P_q` is range from (0, query_size) and the absolute position of key
+ :math:`P_k` is range from (0, key_size), The relative positions from query to key is :math:`R_{q \\rightarrow k} =
+ P_q - P_k`
+
+ Args:
+ query_size (int): the length of query
+ key_size (int): the length of key
+
+ Return:
+ :obj:`torch.LongTensor`: A tensor with shape [1, query_size, key_size]
+
+ """
+
+ q_ids = torch.arange(query_size, dtype=torch.long, device=device)
+ k_ids = torch.arange(key_size, dtype=torch.long, device=device)
+ rel_pos_ids = q_ids[:, None] - k_ids.view(1, -1).repeat(query_size, 1)
+ rel_pos_ids = rel_pos_ids[:query_size, :]
+ rel_pos_ids = rel_pos_ids.unsqueeze(0)
+ return rel_pos_ids
+
+
+@torch.jit.script
+def c2p_dynamic_expand(c2p_pos, query_layer, relative_pos):
+ return c2p_pos.expand([query_layer.size(0), query_layer.size(1), query_layer.size(2), relative_pos.size(-1)])
+
+
+@torch.jit.script
+def p2c_dynamic_expand(c2p_pos, query_layer, key_layer):
+ return c2p_pos.expand([query_layer.size(0), query_layer.size(1), key_layer.size(-2), key_layer.size(-2)])
+
+
+@torch.jit.script
+def pos_dynamic_expand(pos_index, p2c_att, key_layer):
+ return pos_index.expand(p2c_att.size()[:2] + (pos_index.size(-2), key_layer.size(-2)))
+
+
+class DisentangledSelfAttention(nn.Module):
+ """
+ Disentangled self-attention module
+
+ Parameters:
+ config (:obj:`str`):
+ A model config class instance with the configuration to build a new model. The schema is similar to
+ `BertConfig`, for more details, please refer :class:`~transformers.DebertaConfig`
+
+ """
+
+ def __init__(self, config):
+ super().__init__()
+ if config.hidden_size % config.num_attention_heads != 0:
+ raise ValueError(
+ f"The hidden size ({config.hidden_size}) is not a multiple of the number of attention "
+ f"heads ({config.num_attention_heads})"
+ )
+ self.num_attention_heads = config.num_attention_heads
+ self.attention_head_size = int(config.hidden_size / config.num_attention_heads)
+ self.all_head_size = self.num_attention_heads * self.attention_head_size
+ self.in_proj = nn.Linear(config.hidden_size, self.all_head_size * 3, bias=False)
+ self.q_bias = nn.Parameter(torch.zeros((self.all_head_size), dtype=torch.float))
+ self.v_bias = nn.Parameter(torch.zeros((self.all_head_size), dtype=torch.float))
+ self.pos_att_type = config.pos_att_type if config.pos_att_type is not None else []
+
+ self.relative_attention = getattr(config, "relative_attention", False)
+ self.talking_head = getattr(config, "talking_head", False)
+
+ if self.talking_head:
+ self.head_logits_proj = nn.Linear(config.num_attention_heads, config.num_attention_heads, bias=False)
+ self.head_weights_proj = nn.Linear(config.num_attention_heads, config.num_attention_heads, bias=False)
+
+ if self.relative_attention:
+ self.max_relative_positions = getattr(config, "max_relative_positions", -1)
+ if self.max_relative_positions < 1:
+ self.max_relative_positions = config.max_position_embeddings
+ self.pos_dropout = StableDropout(config.hidden_dropout_prob)
+
+ if "c2p" in self.pos_att_type or "p2p" in self.pos_att_type:
+ self.pos_proj = nn.Linear(config.hidden_size, self.all_head_size, bias=False)
+ if "p2c" in self.pos_att_type or "p2p" in self.pos_att_type:
+ self.pos_q_proj = nn.Linear(config.hidden_size, self.all_head_size)
+
+ self.dropout = StableDropout(config.attention_probs_dropout_prob)
+
+ def transpose_for_scores(self, x):
+ new_x_shape = x.size()[:-1] + (self.num_attention_heads, -1)
+ x = x.view(*new_x_shape)
+ return x.permute(0, 2, 1, 3)
+
+ def forward(
+ self,
+ hidden_states,
+ attention_mask,
+ return_att=False,
+ query_states=None,
+ relative_pos=None,
+ rel_embeddings=None,
+ past_key_value=None,
+ ):
+ """
+ Call the module
+
+ Args:
+ hidden_states (:obj:`torch.FloatTensor`):
+ Input states to the module usually the output from previous layer, it will be the Q,K and V in
+ `Attention(Q,K,V)`
+
+ attention_mask (:obj:`torch.ByteTensor`):
+ An attention mask matrix of shape [`B`, `N`, `N`] where `B` is the batch size, `N` is the maximum
+ sequence length in which element [i,j] = `1` means the `i` th token in the input can attend to the `j`
+ th token.
+
+ return_att (:obj:`bool`, optional):
+ Whether return the attention matrix.
+
+ query_states (:obj:`torch.FloatTensor`, optional):
+ The `Q` state in `Attention(Q,K,V)`.
+
+ relative_pos (:obj:`torch.LongTensor`):
+ The relative position encoding between the tokens in the sequence. It's of shape [`B`, `N`, `N`] with
+ values ranging in [`-max_relative_positions`, `max_relative_positions`].
+
+ rel_embeddings (:obj:`torch.FloatTensor`):
+ The embedding of relative distances. It's a tensor of shape [:math:`2 \\times
+ \\text{max_relative_positions}`, `hidden_size`].
+
+
+ """
+ if query_states is None:
+ qp = self.in_proj(hidden_states) # .split(self.all_head_size, dim=-1)
+ query_layer, key_layer, value_layer = self.transpose_for_scores(qp).chunk(3, dim=-1)
+ else:
+
+ def linear(w, b, x):
+ if b is not None:
+ return torch.matmul(x, w.t()) + b.t()
+ else:
+ return torch.matmul(x, w.t()) # + b.t()
+
+ ws = self.in_proj.weight.chunk(self.num_attention_heads * 3, dim=0)
+ qkvw = [torch.cat([ws[i * 3 + k] for i in range(self.num_attention_heads)], dim=0) for k in range(3)]
+ qkvb = [None] * 3
+
+ q = linear(qkvw[0], qkvb[0], query_states)
+ k, v = [linear(qkvw[i], qkvb[i], hidden_states) for i in range(1, 3)]
+ query_layer, key_layer, value_layer = [self.transpose_for_scores(x) for x in [q, k, v]]
+
+ query_layer = query_layer + self.transpose_for_scores(self.q_bias[None, None, :])
+ value_layer = value_layer + self.transpose_for_scores(self.v_bias[None, None, :])
+
+ rel_att = None
+ # Take the dot product between "query" and "key" to get the raw attention scores.
+ scale_factor = 1 + len(self.pos_att_type)
+ scale = math.sqrt(query_layer.size(-1) * scale_factor)
+
+ past_key_value_length = past_key_value.shape[3] if past_key_value is not None else 0
+ if past_key_value is not None:
+ key_layer_prefix = torch.cat([past_key_value[0], key_layer], dim=2)
+ value_layer = torch.cat([past_key_value[1], value_layer], dim=2)
+ else:
+ key_layer_prefix = key_layer
+
+ query_layer = query_layer / scale
+ attention_scores = torch.matmul(query_layer, key_layer_prefix.transpose(-1, -2))
+ if self.relative_attention:
+ rel_embeddings = self.pos_dropout(rel_embeddings)
+ rel_att = self.disentangled_att_bias(query_layer, key_layer, relative_pos, rel_embeddings, scale_factor)
+
+ if rel_att is not None:
+ if past_key_value is not None:
+ # print(attention_scores.shape)
+ # print(rel_att.shape)
+ # exit()
+ att_shape = rel_att.shape[:-1] + (past_key_value_length,)
+ prefix_att = torch.zeros(*att_shape).to(rel_att.device)
+ attention_scores = attention_scores + torch.cat([prefix_att, rel_att], dim=-1)
+ else:
+ attention_scores = attention_scores + rel_att
+
+ # bxhxlxd
+ if self.talking_head:
+ attention_scores = self.head_logits_proj(attention_scores.permute(0, 2, 3, 1)).permute(0, 3, 1, 2)
+
+ softmax_mask = attention_mask[:,:, past_key_value_length:,:]
+
+ attention_probs = XSoftmax.apply(attention_scores, softmax_mask, -1)
+ attention_probs = self.dropout(attention_probs)
+ if self.talking_head:
+ attention_probs = self.head_weights_proj(attention_probs.permute(0, 2, 3, 1)).permute(0, 3, 1, 2)
+
+ context_layer = torch.matmul(attention_probs, value_layer)
+ context_layer = context_layer.permute(0, 2, 1, 3).contiguous()
+ new_context_layer_shape = context_layer.size()[:-2] + (-1,)
+ context_layer = context_layer.view(*new_context_layer_shape)
+ if return_att:
+ return (context_layer, attention_probs)
+ else:
+ return context_layer
+
+ def disentangled_att_bias(self, query_layer, key_layer, relative_pos, rel_embeddings, scale_factor):
+ if relative_pos is None:
+ q = query_layer.size(-2)
+ relative_pos = build_relative_position(q, key_layer.size(-2), query_layer.device)
+ if relative_pos.dim() == 2:
+ relative_pos = relative_pos.unsqueeze(0).unsqueeze(0)
+ elif relative_pos.dim() == 3:
+ relative_pos = relative_pos.unsqueeze(1)
+ # bxhxqxk
+ elif relative_pos.dim() != 4:
+ raise ValueError(f"Relative position ids must be of dim 2 or 3 or 4. {relative_pos.dim()}")
+
+ att_span = min(max(query_layer.size(-2), key_layer.size(-2)), self.max_relative_positions)
+ relative_pos = relative_pos.long().to(query_layer.device)
+ rel_embeddings = rel_embeddings[
+ self.max_relative_positions - att_span : self.max_relative_positions + att_span, :
+ ].unsqueeze(0)
+ if "c2p" in self.pos_att_type or "p2p" in self.pos_att_type:
+ pos_key_layer = self.pos_proj(rel_embeddings)
+ pos_key_layer = self.transpose_for_scores(pos_key_layer)
+
+ if "p2c" in self.pos_att_type or "p2p" in self.pos_att_type:
+ pos_query_layer = self.pos_q_proj(rel_embeddings)
+ pos_query_layer = self.transpose_for_scores(pos_query_layer)
+
+ score = 0
+ # content->position
+ if "c2p" in self.pos_att_type:
+ c2p_att = torch.matmul(query_layer, pos_key_layer.transpose(-1, -2))
+ c2p_pos = torch.clamp(relative_pos + att_span, 0, att_span * 2 - 1)
+ c2p_att = torch.gather(c2p_att, dim=-1, index=c2p_dynamic_expand(c2p_pos, query_layer, relative_pos))
+ score += c2p_att
+
+ # position->content
+ if "p2c" in self.pos_att_type or "p2p" in self.pos_att_type:
+ pos_query_layer /= math.sqrt(pos_query_layer.size(-1) * scale_factor)
+ if query_layer.size(-2) != key_layer.size(-2):
+ r_pos = build_relative_position(key_layer.size(-2), key_layer.size(-2), query_layer.device)
+ else:
+ r_pos = relative_pos
+ p2c_pos = torch.clamp(-r_pos + att_span, 0, att_span * 2 - 1)
+ if query_layer.size(-2) != key_layer.size(-2):
+ pos_index = relative_pos[:, :, :, 0].unsqueeze(-1)
+
+ if "p2c" in self.pos_att_type:
+ p2c_att = torch.matmul(key_layer, pos_query_layer.transpose(-1, -2))
+ p2c_att = torch.gather(
+ p2c_att, dim=-1, index=p2c_dynamic_expand(p2c_pos, query_layer, key_layer)
+ ).transpose(-1, -2)
+ if query_layer.size(-2) != key_layer.size(-2):
+ p2c_att = torch.gather(p2c_att, dim=-2, index=pos_dynamic_expand(pos_index, p2c_att, key_layer))
+ score += p2c_att
+
+ return score
+
+
+class DebertaEmbeddings(nn.Module):
+ """Construct the embeddings from word, position and token_type embeddings."""
+
+ def __init__(self, config):
+ super().__init__()
+ pad_token_id = getattr(config, "pad_token_id", 0)
+ self.embedding_size = getattr(config, "embedding_size", config.hidden_size)
+ self.word_embeddings = nn.Embedding(config.vocab_size, self.embedding_size, padding_idx=pad_token_id)
+
+ self.position_biased_input = getattr(config, "position_biased_input", True)
+ if not self.position_biased_input:
+ self.position_embeddings = None
+ else:
+ self.position_embeddings = nn.Embedding(config.max_position_embeddings, self.embedding_size)
+
+ if config.type_vocab_size > 0:
+ self.token_type_embeddings = nn.Embedding(config.type_vocab_size, self.embedding_size)
+
+ if self.embedding_size != config.hidden_size:
+ self.embed_proj = nn.Linear(self.embedding_size, config.hidden_size, bias=False)
+ self.LayerNorm = DebertaLayerNorm(config.hidden_size, config.layer_norm_eps)
+ self.dropout = StableDropout(config.hidden_dropout_prob)
+ self.config = config
+
+ # position_ids (1, len position emb) is contiguous in memory and exported when serialized
+ self.register_buffer("position_ids", torch.arange(config.max_position_embeddings).expand((1, -1)))
+
+ def forward(self, input_ids=None, token_type_ids=None, position_ids=None, mask=None, inputs_embeds=None, past_key_values_length=0):
+ if input_ids is not None:
+ input_shape = input_ids.size()
+ else:
+ input_shape = inputs_embeds.size()[:-1]
+
+ seq_length = input_shape[1]
+
+ if position_ids is None:
+ position_ids = self.position_ids[:, past_key_values_length : seq_length + past_key_values_length]
+
+ if token_type_ids is None:
+ token_type_ids = torch.zeros(input_shape, dtype=torch.long, device=self.position_ids.device)
+
+ if inputs_embeds is None:
+ inputs_embeds = self.word_embeddings(input_ids)
+
+ if self.position_embeddings is not None:
+ position_embeddings = self.position_embeddings(position_ids.long())
+ else:
+ position_embeddings = torch.zeros_like(inputs_embeds)
+
+ embeddings = inputs_embeds
+ if self.position_biased_input:
+ embeddings += position_embeddings
+ if self.config.type_vocab_size > 0:
+ token_type_embeddings = self.token_type_embeddings(token_type_ids)
+ embeddings += token_type_embeddings
+
+ if self.embedding_size != self.config.hidden_size:
+ embeddings = self.embed_proj(embeddings)
+
+ embeddings = self.LayerNorm(embeddings)
+
+ if mask is not None:
+ if mask.dim() != embeddings.dim():
+ if mask.dim() == 4:
+ mask = mask.squeeze(1).squeeze(1)
+ mask = mask.unsqueeze(2)
+ mask = mask.to(embeddings.dtype)
+
+ embeddings = embeddings * mask
+
+ embeddings = self.dropout(embeddings)
+ return embeddings
+
+
+class DebertaPreTrainedModel(PreTrainedModel):
+ """
+ An abstract class to handle weights initialization and a simple interface for downloading and loading pretrained
+ models.
+ """
+
+ config_class = DebertaConfig
+ base_model_prefix = "deberta"
+ _keys_to_ignore_on_load_missing = ["position_ids"]
+ _keys_to_ignore_on_load_unexpected = ["position_embeddings"]
+
+ def __init__(self, config):
+ super().__init__(config)
+ self._register_load_state_dict_pre_hook(self._pre_load_hook)
+
+ def _init_weights(self, module):
+ """Initialize the weights."""
+ if isinstance(module, nn.Linear):
+ # Slightly different from the TF version which uses truncated_normal for initialization
+ # cf https://github.com/pytorch/pytorch/pull/5617
+ module.weight.data.normal_(mean=0.0, std=self.config.initializer_range)
+ if module.bias is not None:
+ module.bias.data.zero_()
+ elif isinstance(module, nn.Embedding):
+ module.weight.data.normal_(mean=0.0, std=self.config.initializer_range)
+ if module.padding_idx is not None:
+ module.weight.data[module.padding_idx].zero_()
+
+ def _pre_load_hook(self, state_dict, prefix, local_metadata, strict, missing_keys, unexpected_keys, error_msgs):
+ """
+ Removes the classifier if it doesn't have the correct number of labels.
+ """
+ self_state = self.state_dict()
+ if (
+ ("classifier.weight" in self_state)
+ and ("classifier.weight" in state_dict)
+ and self_state["classifier.weight"].size() != state_dict["classifier.weight"].size()
+ ):
+ logger.warning(
+ f"The checkpoint classifier head has a shape {state_dict['classifier.weight'].size()} and this model "
+ f"classifier head has a shape {self_state['classifier.weight'].size()}. Ignoring the checkpoint "
+ f"weights. You should train your model on new data."
+ )
+ del state_dict["classifier.weight"]
+ if "classifier.bias" in state_dict:
+ del state_dict["classifier.bias"]
+
+
+DEBERTA_START_DOCSTRING = r"""
+ The DeBERTa model was proposed in `DeBERTa: Decoding-enhanced BERT with Disentangled Attention
+ `_ by Pengcheng He, Xiaodong Liu, Jianfeng Gao, Weizhu Chen. It's build on top of
+ BERT/RoBERTa with two improvements, i.e. disentangled attention and enhanced mask decoder. With those two
+ improvements, it out perform BERT/RoBERTa on a majority of tasks with 80GB pretraining data.
+
+ This model is also a PyTorch `torch.nn.Module `__
+ subclass. Use it as a regular PyTorch Module and refer to the PyTorch documentation for all matter related to
+ general usage and behavior.```
+
+
+ Parameters:
+ config (:class:`~transformers.DebertaConfig`): Model configuration class with all the parameters of the model.
+ Initializing with a config file does not load the weights associated with the model, only the
+ configuration. Check out the :meth:`~transformers.PreTrainedModel.from_pretrained` method to load the model
+ weights.
+"""
+
+DEBERTA_INPUTS_DOCSTRING = r"""
+ Args:
+ input_ids (:obj:`torch.LongTensor` of shape :obj:`{0}`):
+ Indices of input sequence tokens in the vocabulary.
+
+ Indices can be obtained using :class:`transformers.DebertaTokenizer`. See
+ :func:`transformers.PreTrainedTokenizer.encode` and :func:`transformers.PreTrainedTokenizer.__call__` for
+ details.
+
+ `What are input IDs? <../glossary.html#input-ids>`__
+ attention_mask (:obj:`torch.FloatTensor` of shape :obj:`{0}`, `optional`):
+ Mask to avoid performing attention on padding token indices. Mask values selected in ``[0, 1]``:
+
+ - 1 for tokens that are **not masked**,
+ - 0 for tokens that are **masked**.
+
+ `What are attention masks? <../glossary.html#attention-mask>`__
+ token_type_ids (:obj:`torch.LongTensor` of shape :obj:`{0}`, `optional`):
+ Segment token indices to indicate first and second portions of the inputs. Indices are selected in ``[0,
+ 1]``:
+
+ - 0 corresponds to a `sentence A` token,
+ - 1 corresponds to a `sentence B` token.
+
+ `What are token type IDs? <../glossary.html#token-type-ids>`_
+ position_ids (:obj:`torch.LongTensor` of shape :obj:`{0}`, `optional`):
+ Indices of positions of each input sequence tokens in the position embeddings. Selected in the range ``[0,
+ config.max_position_embeddings - 1]``.
+
+ `What are position IDs? <../glossary.html#position-ids>`_
+ inputs_embeds (:obj:`torch.FloatTensor` of shape :obj:`(batch_size, sequence_length, hidden_size)`, `optional`):
+ Optionally, instead of passing :obj:`input_ids` you can choose to directly pass an embedded representation.
+ This is useful if you want more control over how to convert `input_ids` indices into associated vectors
+ than the model's internal embedding lookup matrix.
+ output_attentions (:obj:`bool`, `optional`):
+ Whether or not to return the attentions tensors of all attention layers. See ``attentions`` under returned
+ tensors for more detail.
+ output_hidden_states (:obj:`bool`, `optional`):
+ Whether or not to return the hidden states of all layers. See ``hidden_states`` under returned tensors for
+ more detail.
+ return_dict (:obj:`bool`, `optional`):
+ Whether or not to return a :class:`~transformers.file_utils.ModelOutput` instead of a plain tuple.
+"""
+
+
+@add_start_docstrings(
+ "The bare DeBERTa Model transformer outputting raw hidden-states without any specific head on top.",
+ DEBERTA_START_DOCSTRING,
+)
+class DebertaModel(DebertaPreTrainedModel):
+ def __init__(self, config):
+ super().__init__(config)
+
+ self.embeddings = DebertaEmbeddings(config)
+ self.encoder = DebertaEncoder(config)
+ self.z_steps = 0
+ self.config = config
+ self.init_weights()
+
+ def get_input_embeddings(self):
+ return self.embeddings.word_embeddings
+
+ def set_input_embeddings(self, new_embeddings):
+ self.embeddings.word_embeddings = new_embeddings
+
+ def _prune_heads(self, heads_to_prune):
+ """
+ Prunes heads of the model. heads_to_prune: dict of {layer_num: list of heads to prune in this layer} See base
+ class PreTrainedModel
+ """
+ raise NotImplementedError("The prune function is not implemented in DeBERTa model.")
+
+ @add_start_docstrings_to_model_forward(DEBERTA_INPUTS_DOCSTRING.format("batch_size, sequence_length"))
+ @add_code_sample_docstrings(
+ # tokenizer_class=_TOKENIZER_FOR_DOC,
+ checkpoint=_CHECKPOINT_FOR_DOC,
+ output_type=SequenceClassifierOutput,
+ config_class=_CONFIG_FOR_DOC,
+ )
+ def forward(
+ self,
+ input_ids=None,
+ attention_mask=None,
+ token_type_ids=None,
+ position_ids=None,
+ inputs_embeds=None,
+ output_attentions=None,
+ output_hidden_states=None,
+ return_dict=None,
+ past_key_values=None,
+ ):
+ output_attentions = output_attentions if output_attentions is not None else self.config.output_attentions
+ output_hidden_states = (
+ output_hidden_states if output_hidden_states is not None else self.config.output_hidden_states
+ )
+ return_dict = return_dict if return_dict is not None else self.config.use_return_dict
+
+ if input_ids is not None and inputs_embeds is not None:
+ raise ValueError("You cannot specify both input_ids and inputs_embeds at the same time")
+ elif input_ids is not None:
+ input_shape = input_ids.size()
+ batch_size, seq_length = input_shape
+ elif inputs_embeds is not None:
+ input_shape = inputs_embeds.size()[:-1]
+ batch_size, seq_length = input_shape
+ else:
+ raise ValueError("You have to specify either input_ids or inputs_embeds")
+
+ device = input_ids.device if input_ids is not None else inputs_embeds.device
+
+ past_key_values_length = past_key_values[0][0].shape[2] if past_key_values is not None else 0
+
+ embedding_mask = attention_mask[:, past_key_values_length:].contiguous()
+ if attention_mask is None:
+ # attention_mask = torch.ones(input_shape, device=device)
+ attention_mask = torch.ones(((batch_size, seq_length + past_key_values_length)), device=device)
+ if token_type_ids is None:
+ token_type_ids = torch.zeros(input_shape, dtype=torch.long, device=device)
+
+ embedding_output = self.embeddings(
+ input_ids=input_ids,
+ token_type_ids=token_type_ids,
+ position_ids=position_ids,
+ mask=embedding_mask,
+ inputs_embeds=inputs_embeds,
+ past_key_values_length=past_key_values_length,
+ )
+
+ encoder_outputs = self.encoder(
+ embedding_output,
+ attention_mask,
+ output_hidden_states=True,
+ output_attentions=output_attentions,
+ return_dict=return_dict,
+ past_key_values=past_key_values,
+ )
+ encoded_layers = encoder_outputs[1]
+
+ if self.z_steps > 1:
+ hidden_states = encoded_layers[-2]
+ layers = [self.encoder.layer[-1] for _ in range(self.z_steps)]
+ query_states = encoded_layers[-1]
+ rel_embeddings = self.encoder.get_rel_embedding()
+ attention_mask = self.encoder.get_attention_mask(attention_mask)
+ rel_pos = self.encoder.get_rel_pos(embedding_output)
+ for layer in layers[1:]:
+ query_states = layer(
+ hidden_states,
+ attention_mask,
+ return_att=False,
+ query_states=query_states,
+ relative_pos=rel_pos,
+ rel_embeddings=rel_embeddings,
+ )
+ encoded_layers.append(query_states)
+
+ sequence_output = encoded_layers[-1]
+
+ if not return_dict:
+ return (sequence_output,) + encoder_outputs[(1 if output_hidden_states else 2) :]
+
+ return BaseModelOutput(
+ last_hidden_state=sequence_output,
+ hidden_states=encoder_outputs.hidden_states if output_hidden_states else None,
+ attentions=encoder_outputs.attentions,
+ )
+
+
+@add_start_docstrings("""DeBERTa Model with a `language modeling` head on top. """, DEBERTA_START_DOCSTRING)
+class DebertaForMaskedLM(DebertaPreTrainedModel):
+ _keys_to_ignore_on_load_unexpected = [r"pooler"]
+ _keys_to_ignore_on_load_missing = [r"position_ids", r"predictions.decoder.bias"]
+
+ def __init__(self, config):
+ super().__init__(config)
+
+ self.deberta = DebertaModel(config)
+ self.cls = DebertaOnlyMLMHead(config)
+
+ self.init_weights()
+
+ def get_output_embeddings(self):
+ return self.cls.predictions.decoder
+
+ def set_output_embeddings(self, new_embeddings):
+ self.cls.predictions.decoder = new_embeddings
+
+ @add_start_docstrings_to_model_forward(DEBERTA_INPUTS_DOCSTRING.format("batch_size, sequence_length"))
+ @add_code_sample_docstrings(
+ # tokenizer_class=_TOKENIZER_FOR_DOC,
+ checkpoint=_CHECKPOINT_FOR_DOC,
+ output_type=MaskedLMOutput,
+ config_class=_CONFIG_FOR_DOC,
+ )
+ def forward(
+ self,
+ input_ids=None,
+ attention_mask=None,
+ token_type_ids=None,
+ position_ids=None,
+ inputs_embeds=None,
+ labels=None,
+ output_attentions=None,
+ output_hidden_states=None,
+ return_dict=None,
+ ):
+ r"""
+ labels (:obj:`torch.LongTensor` of shape :obj:`(batch_size, sequence_length)`, `optional`):
+ Labels for computing the masked language modeling loss. Indices should be in ``[-100, 0, ...,
+ config.vocab_size]`` (see ``input_ids`` docstring) Tokens with indices set to ``-100`` are ignored
+ (masked), the loss is only computed for the tokens with labels in ``[0, ..., config.vocab_size]``
+ """
+
+ return_dict = return_dict if return_dict is not None else self.config.use_return_dict
+
+ outputs = self.deberta(
+ input_ids,
+ attention_mask=attention_mask,
+ token_type_ids=token_type_ids,
+ position_ids=position_ids,
+ inputs_embeds=inputs_embeds,
+ output_attentions=output_attentions,
+ output_hidden_states=output_hidden_states,
+ return_dict=return_dict,
+ )
+
+ sequence_output = outputs[0]
+ prediction_scores = self.cls(sequence_output)
+
+ masked_lm_loss = None
+ if labels is not None:
+ loss_fct = CrossEntropyLoss() # -100 index = padding token
+ masked_lm_loss = loss_fct(prediction_scores.view(-1, self.config.vocab_size), labels.view(-1))
+
+ if not return_dict:
+ output = (prediction_scores,) + outputs[1:]
+ return ((masked_lm_loss,) + output) if masked_lm_loss is not None else output
+
+ return MaskedLMOutput(
+ loss=masked_lm_loss,
+ logits=prediction_scores,
+ hidden_states=outputs.hidden_states,
+ attentions=outputs.attentions,
+ )
+
+
+# copied from transformers.models.bert.BertPredictionHeadTransform with bert -> deberta
+class DebertaPredictionHeadTransform(nn.Module):
+ def __init__(self, config):
+ super().__init__()
+ self.dense = nn.Linear(config.hidden_size, config.hidden_size)
+ if isinstance(config.hidden_act, str):
+ self.transform_act_fn = ACT2FN[config.hidden_act]
+ else:
+ self.transform_act_fn = config.hidden_act
+ self.LayerNorm = nn.LayerNorm(config.hidden_size, eps=config.layer_norm_eps)
+
+ def forward(self, hidden_states):
+ hidden_states = self.dense(hidden_states)
+ hidden_states = self.transform_act_fn(hidden_states)
+ hidden_states = self.LayerNorm(hidden_states)
+ return hidden_states
+
+
+# copied from transformers.models.bert.BertLMPredictionHead with bert -> deberta
+class DebertaLMPredictionHead(nn.Module):
+ def __init__(self, config):
+ super().__init__()
+ self.transform = DebertaPredictionHeadTransform(config)
+
+ # The output weights are the same as the input embeddings, but there is
+ # an output-only bias for each token.
+ self.decoder = nn.Linear(config.hidden_size, config.vocab_size, bias=False)
+
+ self.bias = nn.Parameter(torch.zeros(config.vocab_size))
+
+ # Need a link between the two variables so that the bias is correctly resized with `resize_token_embeddings`
+ self.decoder.bias = self.bias
+
+ def forward(self, hidden_states):
+ hidden_states = self.transform(hidden_states)
+ hidden_states = self.decoder(hidden_states)
+ return hidden_states
+
+
+# copied from transformers.models.bert.BertOnlyMLMHead with bert -> deberta
+class DebertaOnlyMLMHead(nn.Module):
+ def __init__(self, config):
+ super().__init__()
+ self.predictions = DebertaLMPredictionHead(config)
+
+ def forward(self, sequence_output):
+ prediction_scores = self.predictions(sequence_output)
+ return prediction_scores
+
+
+@add_start_docstrings(
+ """
+ DeBERTa Model transformer with a sequence classification/regression head on top (a linear layer on top of the
+ pooled output) e.g. for GLUE tasks.
+ """,
+ DEBERTA_START_DOCSTRING,
+)
+class DebertaForSequenceClassification(DebertaPreTrainedModel):
+ def __init__(self, config):
+ super().__init__(config)
+
+ num_labels = getattr(config, "num_labels", 2)
+ self.num_labels = num_labels
+
+ self.deberta = DebertaModel(config)
+ self.pooler = ContextPooler(config)
+ output_dim = self.pooler.output_dim
+
+ self.classifier = nn.Linear(output_dim, num_labels)
+ drop_out = getattr(config, "cls_dropout", None)
+ drop_out = self.config.hidden_dropout_prob if drop_out is None else drop_out
+ self.dropout = StableDropout(drop_out)
+
+ self.init_weights()
+
+ def get_input_embeddings(self):
+ return self.deberta.get_input_embeddings()
+
+ def set_input_embeddings(self, new_embeddings):
+ self.deberta.set_input_embeddings(new_embeddings)
+
+ @add_start_docstrings_to_model_forward(DEBERTA_INPUTS_DOCSTRING.format("batch_size, sequence_length"))
+ @add_code_sample_docstrings(
+ # tokenizer_class=_TOKENIZER_FOR_DOC,
+ checkpoint=_CHECKPOINT_FOR_DOC,
+ output_type=SequenceClassifierOutput,
+ config_class=_CONFIG_FOR_DOC,
+ )
+ def forward(
+ self,
+ input_ids=None,
+ attention_mask=None,
+ token_type_ids=None,
+ position_ids=None,
+ inputs_embeds=None,
+ labels=None,
+ output_attentions=None,
+ output_hidden_states=None,
+ return_dict=None,
+ ):
+ r"""
+ labels (:obj:`torch.LongTensor` of shape :obj:`(batch_size,)`, `optional`):
+ Labels for computing the sequence classification/regression loss. Indices should be in :obj:`[0, ...,
+ config.num_labels - 1]`. If :obj:`config.num_labels == 1` a regression loss is computed (Mean-Square loss),
+ If :obj:`config.num_labels > 1` a classification loss is computed (Cross-Entropy).
+ """
+ return_dict = return_dict if return_dict is not None else self.config.use_return_dict
+
+ outputs = self.deberta(
+ input_ids,
+ token_type_ids=token_type_ids,
+ attention_mask=attention_mask,
+ position_ids=position_ids,
+ inputs_embeds=inputs_embeds,
+ output_attentions=output_attentions,
+ output_hidden_states=output_hidden_states,
+ return_dict=return_dict,
+ )
+
+ encoder_layer = outputs[0]
+ pooled_output = self.pooler(encoder_layer)
+ pooled_output = self.dropout(pooled_output)
+ logits = self.classifier(pooled_output)
+
+ loss = None
+ if labels is not None:
+ if self.num_labels == 1:
+ # regression task
+ loss_fn = nn.MSELoss()
+ logits = logits.view(-1).to(labels.dtype)
+ loss = loss_fn(logits, labels.view(-1))
+ elif labels.dim() == 1 or labels.size(-1) == 1:
+ label_index = (labels >= 0).nonzero()
+ labels = labels.long()
+ if label_index.size(0) > 0:
+ labeled_logits = torch.gather(logits, 0, label_index.expand(label_index.size(0), logits.size(1)))
+ labels = torch.gather(labels, 0, label_index.view(-1))
+ loss_fct = CrossEntropyLoss()
+ loss = loss_fct(labeled_logits.view(-1, self.num_labels).float(), labels.view(-1))
+ else:
+ loss = torch.tensor(0).to(logits)
+ else:
+ log_softmax = nn.LogSoftmax(-1)
+ loss = -((log_softmax(logits) * labels).sum(-1)).mean()
+ if not return_dict:
+ output = (logits,) + outputs[1:]
+ return ((loss,) + output) if loss is not None else output
+ else:
+ return SequenceClassifierOutput(
+ loss=loss,
+ logits=logits,
+ hidden_states=outputs.hidden_states,
+ attentions=outputs.attentions,
+ )
+
+
+@add_start_docstrings(
+ """
+ DeBERTa Model with a token classification head on top (a linear layer on top of the hidden-states output) e.g. for
+ Named-Entity-Recognition (NER) tasks.
+ """,
+ DEBERTA_START_DOCSTRING,
+)
+class DebertaForTokenClassification(DebertaPreTrainedModel):
+ _keys_to_ignore_on_load_unexpected = [r"pooler"]
+
+ def __init__(self, config):
+ super().__init__(config)
+ self.num_labels = config.num_labels
+
+ self.deberta = DebertaModel(config)
+ self.dropout = nn.Dropout(config.hidden_dropout_prob)
+ self.classifier = nn.Linear(config.hidden_size, config.num_labels)
+
+ for param in self.deberta.parameters():
+ param.requires_grad = False
+
+ self.init_weights()
+
+ @add_start_docstrings_to_model_forward(DEBERTA_INPUTS_DOCSTRING.format("batch_size, sequence_length"))
+ @add_code_sample_docstrings(
+ # tokenizer_class=_TOKENIZER_FOR_DOC,
+ checkpoint=_CHECKPOINT_FOR_DOC,
+ output_type=TokenClassifierOutput,
+ config_class=_CONFIG_FOR_DOC,
+ )
+ def forward(
+ self,
+ input_ids=None,
+ attention_mask=None,
+ token_type_ids=None,
+ position_ids=None,
+ inputs_embeds=None,
+ labels=None,
+ output_attentions=None,
+ output_hidden_states=None,
+ return_dict=None,
+ ):
+ r"""
+ labels (:obj:`torch.LongTensor` of shape :obj:`(batch_size, sequence_length)`, `optional`):
+ Labels for computing the token classification loss. Indices should be in ``[0, ..., config.num_labels -
+ 1]``.
+ """
+ return_dict = return_dict if return_dict is not None else self.config.use_return_dict
+
+ outputs = self.deberta(
+ input_ids,
+ attention_mask=attention_mask,
+ token_type_ids=token_type_ids,
+ position_ids=position_ids,
+ inputs_embeds=inputs_embeds,
+ output_attentions=output_attentions,
+ output_hidden_states=output_hidden_states,
+ return_dict=return_dict,
+ )
+
+ sequence_output = outputs[0]
+
+ sequence_output = self.dropout(sequence_output)
+ logits = self.classifier(sequence_output)
+
+ loss = None
+ if labels is not None:
+ loss_fct = CrossEntropyLoss()
+ # Only keep active parts of the loss
+ if attention_mask is not None:
+ active_loss = attention_mask.view(-1) == 1
+ active_logits = logits.view(-1, self.num_labels)
+ active_labels = torch.where(
+ active_loss, labels.view(-1), torch.tensor(loss_fct.ignore_index).type_as(labels)
+ )
+ loss = loss_fct(active_logits, active_labels)
+ else:
+ loss = loss_fct(logits.view(-1, self.num_labels), labels.view(-1))
+
+ if not return_dict:
+ output = (logits,) + outputs[1:]
+ return ((loss,) + output) if loss is not None else output
+
+ return TokenClassifierOutput(
+ loss=loss,
+ logits=logits,
+ hidden_states=outputs.hidden_states,
+ attentions=outputs.attentions,
+ )
+
+
+@add_start_docstrings(
+ """
+ DeBERTa Model with a span classification head on top for extractive question-answering tasks like SQuAD (a linear
+ layers on top of the hidden-states output to compute `span start logits` and `span end logits`).
+ """,
+ DEBERTA_START_DOCSTRING,
+)
+class DebertaForQuestionAnswering(DebertaPreTrainedModel):
+ _keys_to_ignore_on_load_unexpected = [r"pooler"]
+
+ def __init__(self, config):
+ super().__init__(config)
+ self.num_labels = config.num_labels
+
+ self.deberta = DebertaModel(config)
+ self.qa_outputs = nn.Linear(config.hidden_size, config.num_labels)
+
+ self.init_weights()
+
+ @add_start_docstrings_to_model_forward(DEBERTA_INPUTS_DOCSTRING.format("batch_size, sequence_length"))
+ @add_code_sample_docstrings(
+ # tokenizer_class=_TOKENIZER_FOR_DOC,
+ checkpoint=_CHECKPOINT_FOR_DOC,
+ output_type=QuestionAnsweringModelOutput,
+ config_class=_CONFIG_FOR_DOC,
+ )
+ def forward(
+ self,
+ input_ids=None,
+ attention_mask=None,
+ token_type_ids=None,
+ position_ids=None,
+ inputs_embeds=None,
+ start_positions=None,
+ end_positions=None,
+ output_attentions=None,
+ output_hidden_states=None,
+ return_dict=None,
+ ):
+ r"""
+ start_positions (:obj:`torch.LongTensor` of shape :obj:`(batch_size,)`, `optional`):
+ Labels for position (index) of the start of the labelled span for computing the token classification loss.
+ Positions are clamped to the length of the sequence (:obj:`sequence_length`). Position outside of the
+ sequence are not taken into account for computing the loss.
+ end_positions (:obj:`torch.LongTensor` of shape :obj:`(batch_size,)`, `optional`):
+ Labels for position (index) of the end of the labelled span for computing the token classification loss.
+ Positions are clamped to the length of the sequence (:obj:`sequence_length`). Position outside of the
+ sequence are not taken into account for computing the loss.
+ """
+ return_dict = return_dict if return_dict is not None else self.config.use_return_dict
+
+ outputs = self.deberta(
+ input_ids,
+ attention_mask=attention_mask,
+ token_type_ids=token_type_ids,
+ position_ids=position_ids,
+ inputs_embeds=inputs_embeds,
+ output_attentions=output_attentions,
+ output_hidden_states=output_hidden_states,
+ return_dict=return_dict,
+ )
+
+ sequence_output = outputs[0]
+
+ logits = self.qa_outputs(sequence_output)
+ start_logits, end_logits = logits.split(1, dim=-1)
+ start_logits = start_logits.squeeze(-1).contiguous()
+ end_logits = end_logits.squeeze(-1).contiguous()
+
+ total_loss = None
+ if start_positions is not None and end_positions is not None:
+ # If we are on multi-GPU, split add a dimension
+ if len(start_positions.size()) > 1:
+ start_positions = start_positions.squeeze(-1)
+ if len(end_positions.size()) > 1:
+ end_positions = end_positions.squeeze(-1)
+ # sometimes the start/end positions are outside our model inputs, we ignore these terms
+ ignored_index = start_logits.size(1)
+ start_positions = start_positions.clamp(0, ignored_index)
+ end_positions = end_positions.clamp(0, ignored_index)
+
+ loss_fct = CrossEntropyLoss(ignore_index=ignored_index)
+ start_loss = loss_fct(start_logits, start_positions)
+ end_loss = loss_fct(end_logits, end_positions)
+ total_loss = (start_loss + end_loss) / 2
+
+ if not return_dict:
+ output = (start_logits, end_logits) + outputs[1:]
+ return ((total_loss,) + output) if total_loss is not None else output
+
+ return QuestionAnsweringModelOutput(
+ loss=total_loss,
+ start_logits=start_logits,
+ end_logits=end_logits,
+ hidden_states=outputs.hidden_states,
+ attentions=outputs.attentions,
+ )
\ No newline at end of file
diff --git a/model/debertaV2.py b/model/debertaV2.py
new file mode 100644
index 0000000..6d9a8ce
--- /dev/null
+++ b/model/debertaV2.py
@@ -0,0 +1,1539 @@
+# coding=utf-8
+# Copyright 2020 Microsoft and the Hugging Face Inc. team.
+#
+# Licensed under the Apache License, Version 2.0 (the "License");
+# you may not use this file except in compliance with the License.
+# You may obtain a copy of the License at
+#
+# http://www.apache.org/licenses/LICENSE-2.0
+#
+# Unless required by applicable law or agreed to in writing, software
+# distributed under the License is distributed on an "AS IS" BASIS,
+# WITHOUT WARRANTIES OR CONDITIONS OF ANY KIND, either express or implied.
+# See the License for the specific language governing permissions and
+# limitations under the License.
+""" PyTorch DeBERTa-v2 model. """
+
+import math
+from collections.abc import Sequence
+
+import numpy as np
+import torch
+from torch import _softmax_backward_data, nn
+from torch.nn import CrossEntropyLoss, LayerNorm
+
+
+from transformers.activations import ACT2FN
+from transformers.file_utils import add_code_sample_docstrings, add_start_docstrings, add_start_docstrings_to_model_forward
+from transformers.modeling_outputs import (
+ BaseModelOutput,
+ MaskedLMOutput,
+ QuestionAnsweringModelOutput,
+ SequenceClassifierOutput,
+ TokenClassifierOutput,
+)
+from transformers.modeling_utils import PreTrainedModel
+from transformers.utils import logging
+from transformers.models.deberta_v2.configuration_deberta_v2 import DebertaV2Config
+
+
+logger = logging.get_logger(__name__)
+
+_CONFIG_FOR_DOC = "DebertaV2Config"
+_TOKENIZER_FOR_DOC = "DebertaV2Tokenizer"
+_CHECKPOINT_FOR_DOC = "microsoft/deberta-v2-xlarge"
+
+DEBERTA_V2_PRETRAINED_MODEL_ARCHIVE_LIST = [
+ "microsoft/deberta-v2-xlarge",
+ "microsoft/deberta-v2-xxlarge",
+ "microsoft/deberta-v2-xlarge-mnli",
+ "microsoft/deberta-v2-xxlarge-mnli",
+]
+
+
+# Copied from transformers.models.deberta.modeling_deberta.ContextPooler
+class ContextPooler(nn.Module):
+ def __init__(self, config):
+ super().__init__()
+ self.dense = nn.Linear(config.pooler_hidden_size, config.pooler_hidden_size)
+ self.dropout = StableDropout(config.pooler_dropout)
+ self.config = config
+
+ def forward(self, hidden_states):
+ # We "pool" the model by simply taking the hidden state corresponding
+ # to the first token.
+
+ context_token = hidden_states[:, 0]
+ context_token = self.dropout(context_token)
+ pooled_output = self.dense(context_token)
+ pooled_output = ACT2FN[self.config.pooler_hidden_act](pooled_output)
+ return pooled_output
+
+ @property
+ def output_dim(self):
+ return self.config.hidden_size
+
+
+# Copied from transformers.models.deberta.modeling_deberta.XSoftmax with deberta->deberta_v2
+class XSoftmax(torch.autograd.Function):
+ """
+ Masked Softmax which is optimized for saving memory
+ Args:
+ input (:obj:`torch.tensor`): The input tensor that will apply softmax.
+ mask (:obj:`torch.IntTensor`): The mask matrix where 0 indicate that element will be ignored in the softmax calculation.
+ dim (int): The dimension that will apply softmax
+ Example::
+ >>> import torch
+ >>> from transformers.models.deberta_v2.modeling_deberta_v2 import XSoftmax
+ >>> # Make a tensor
+ >>> x = torch.randn([4,20,100])
+ >>> # Create a mask
+ >>> mask = (x>0).int()
+ >>> y = XSoftmax.apply(x, mask, dim=-1)
+ """
+
+ @staticmethod
+ def forward(self, input, mask, dim):
+ self.dim = dim
+ rmask = ~(mask.bool())
+
+ output = input.masked_fill(rmask, float("-inf"))
+ output = torch.softmax(output, self.dim)
+ output.masked_fill_(rmask, 0)
+ self.save_for_backward(output)
+ return output
+
+ @staticmethod
+ def backward(self, grad_output):
+ (output,) = self.saved_tensors
+ inputGrad = _softmax_backward_data(grad_output, output, self.dim, output)
+ return inputGrad, None, None
+
+
+# Copied from transformers.models.deberta.modeling_deberta.DropoutContext
+class DropoutContext(object):
+ def __init__(self):
+ self.dropout = 0
+ self.mask = None
+ self.scale = 1
+ self.reuse_mask = True
+
+
+# Copied from transformers.models.deberta.modeling_deberta.get_mask
+def get_mask(input, local_context):
+ if not isinstance(local_context, DropoutContext):
+ dropout = local_context
+ mask = None
+ else:
+ dropout = local_context.dropout
+ dropout *= local_context.scale
+ mask = local_context.mask if local_context.reuse_mask else None
+
+ if dropout > 0 and mask is None:
+ mask = (1 - torch.empty_like(input).bernoulli_(1 - dropout)).bool()
+
+ if isinstance(local_context, DropoutContext):
+ if local_context.mask is None:
+ local_context.mask = mask
+
+ return mask, dropout
+
+
+# Copied from transformers.models.deberta.modeling_deberta.XDropout
+class XDropout(torch.autograd.Function):
+ """Optimized dropout function to save computation and memory by using mask operation instead of multiplication."""
+
+ @staticmethod
+ def forward(ctx, input, local_ctx):
+ mask, dropout = get_mask(input, local_ctx)
+ ctx.scale = 1.0 / (1 - dropout)
+ if dropout > 0:
+ ctx.save_for_backward(mask)
+ return input.masked_fill(mask, 0) * ctx.scale
+ else:
+ return input
+
+ @staticmethod
+ def backward(ctx, grad_output):
+ if ctx.scale > 1:
+ (mask,) = ctx.saved_tensors
+ return grad_output.masked_fill(mask, 0) * ctx.scale, None
+ else:
+ return grad_output, None
+
+
+# Copied from transformers.models.deberta.modeling_deberta.StableDropout
+class StableDropout(nn.Module):
+ """
+ Optimized dropout module for stabilizing the training
+ Args:
+ drop_prob (float): the dropout probabilities
+ """
+
+ def __init__(self, drop_prob):
+ super().__init__()
+ self.drop_prob = drop_prob
+ self.count = 0
+ self.context_stack = None
+
+ def forward(self, x):
+ """
+ Call the module
+ Args:
+ x (:obj:`torch.tensor`): The input tensor to apply dropout
+ """
+ if self.training and self.drop_prob > 0:
+ return XDropout.apply(x, self.get_context())
+ return x
+
+ def clear_context(self):
+ self.count = 0
+ self.context_stack = None
+
+ def init_context(self, reuse_mask=True, scale=1):
+ if self.context_stack is None:
+ self.context_stack = []
+ self.count = 0
+ for c in self.context_stack:
+ c.reuse_mask = reuse_mask
+ c.scale = scale
+
+ def get_context(self):
+ if self.context_stack is not None:
+ if self.count >= len(self.context_stack):
+ self.context_stack.append(DropoutContext())
+ ctx = self.context_stack[self.count]
+ ctx.dropout = self.drop_prob
+ self.count += 1
+ return ctx
+ else:
+ return self.drop_prob
+
+
+# Copied from transformers.models.deberta.modeling_deberta.DebertaSelfOutput with DebertaLayerNorm->LayerNorm
+class DebertaV2SelfOutput(nn.Module):
+ def __init__(self, config):
+ super().__init__()
+ self.dense = nn.Linear(config.hidden_size, config.hidden_size)
+ self.LayerNorm = LayerNorm(config.hidden_size, config.layer_norm_eps)
+ self.dropout = StableDropout(config.hidden_dropout_prob)
+
+ def forward(self, hidden_states, input_tensor):
+ hidden_states = self.dense(hidden_states)
+ hidden_states = self.dropout(hidden_states)
+ hidden_states = self.LayerNorm(hidden_states + input_tensor)
+ return hidden_states
+
+
+# Copied from transformers.models.deberta.modeling_deberta.DebertaAttention with Deberta->DebertaV2
+class DebertaV2Attention(nn.Module):
+ def __init__(self, config):
+ super().__init__()
+ self.self = DisentangledSelfAttention(config)
+ self.output = DebertaV2SelfOutput(config)
+ self.config = config
+
+ def forward(
+ self,
+ hidden_states,
+ attention_mask,
+ return_att=False,
+ query_states=None,
+ relative_pos=None,
+ rel_embeddings=None,
+ past_key_value=None,
+ ):
+ self_output = self.self(
+ hidden_states,
+ attention_mask,
+ return_att,
+ query_states=query_states,
+ relative_pos=relative_pos,
+ rel_embeddings=rel_embeddings,
+ past_key_value=past_key_value,
+ )
+ if return_att:
+ self_output, att_matrix = self_output
+ if query_states is None:
+ query_states = hidden_states
+ attention_output = self.output(self_output, query_states)
+
+ if return_att:
+ return (attention_output, att_matrix)
+ else:
+ return attention_output
+
+
+# Copied from transformers.models.bert.modeling_bert.BertIntermediate with Bert->DebertaV2
+class DebertaV2Intermediate(nn.Module):
+ def __init__(self, config):
+ super().__init__()
+ self.dense = nn.Linear(config.hidden_size, config.intermediate_size)
+ if isinstance(config.hidden_act, str):
+ self.intermediate_act_fn = ACT2FN[config.hidden_act]
+ else:
+ self.intermediate_act_fn = config.hidden_act
+
+ def forward(self, hidden_states):
+ hidden_states = self.dense(hidden_states)
+ hidden_states = self.intermediate_act_fn(hidden_states)
+ return hidden_states
+
+
+# Copied from transformers.models.deberta.modeling_deberta.DebertaOutput with DebertaLayerNorm->LayerNorm
+class DebertaV2Output(nn.Module):
+ def __init__(self, config):
+ super().__init__()
+ self.dense = nn.Linear(config.intermediate_size, config.hidden_size)
+ self.LayerNorm = LayerNorm(config.hidden_size, config.layer_norm_eps)
+ self.dropout = StableDropout(config.hidden_dropout_prob)
+ self.config = config
+
+ def forward(self, hidden_states, input_tensor):
+ hidden_states = self.dense(hidden_states)
+ hidden_states = self.dropout(hidden_states)
+ hidden_states = self.LayerNorm(hidden_states + input_tensor)
+ return hidden_states
+
+
+# Copied from transformers.models.deberta.modeling_deberta.DebertaLayer with Deberta->DebertaV2
+class DebertaV2Layer(nn.Module):
+ def __init__(self, config):
+ super().__init__()
+ self.attention = DebertaV2Attention(config)
+ self.intermediate = DebertaV2Intermediate(config)
+ self.output = DebertaV2Output(config)
+
+ def forward(
+ self,
+ hidden_states,
+ attention_mask,
+ return_att=False,
+ query_states=None,
+ relative_pos=None,
+ rel_embeddings=None,
+ past_key_value=None,
+ ):
+ self_attn_past_key_value = past_key_value[:2] if past_key_value is not None else None
+ attention_output = self.attention(
+ hidden_states,
+ attention_mask,
+ return_att=return_att,
+ query_states=query_states,
+ relative_pos=relative_pos,
+ rel_embeddings=rel_embeddings,
+ past_key_value=self_attn_past_key_value,
+ )
+ if return_att:
+ attention_output, att_matrix = attention_output
+ intermediate_output = self.intermediate(attention_output)
+ layer_output = self.output(intermediate_output, attention_output)
+ if return_att:
+ return (layer_output, att_matrix)
+ else:
+ return layer_output
+
+
+class ConvLayer(nn.Module):
+ def __init__(self, config):
+ super().__init__()
+ kernel_size = getattr(config, "conv_kernel_size", 3)
+ groups = getattr(config, "conv_groups", 1)
+ self.conv_act = getattr(config, "conv_act", "tanh")
+ self.conv = nn.Conv1d(
+ config.hidden_size, config.hidden_size, kernel_size, padding=(kernel_size - 1) // 2, groups=groups
+ )
+ self.LayerNorm = LayerNorm(config.hidden_size, config.layer_norm_eps)
+ self.dropout = StableDropout(config.hidden_dropout_prob)
+ self.config = config
+
+ def forward(self, hidden_states, residual_states, input_mask):
+ out = self.conv(hidden_states.permute(0, 2, 1).contiguous()).permute(0, 2, 1).contiguous()
+ rmask = (1 - input_mask).bool()
+ out.masked_fill_(rmask.unsqueeze(-1).expand(out.size()), 0)
+ out = ACT2FN[self.conv_act](self.dropout(out))
+
+ layer_norm_input = residual_states + out
+ output = self.LayerNorm(layer_norm_input).to(layer_norm_input)
+
+ if input_mask is None:
+ output_states = output
+ else:
+ if input_mask.dim() != layer_norm_input.dim():
+ if input_mask.dim() == 4:
+ input_mask = input_mask.squeeze(1).squeeze(1)
+ input_mask = input_mask.unsqueeze(2)
+
+ input_mask = input_mask.to(output.dtype)
+ output_states = output * input_mask
+
+ return output_states
+
+
+class DebertaV2Encoder(nn.Module):
+ """Modified BertEncoder with relative position bias support"""
+
+ def __init__(self, config):
+ super().__init__()
+
+ self.layer = nn.ModuleList([DebertaV2Layer(config) for _ in range(config.num_hidden_layers)])
+ self.relative_attention = getattr(config, "relative_attention", False)
+
+ if self.relative_attention:
+ self.max_relative_positions = getattr(config, "max_relative_positions", -1)
+ if self.max_relative_positions < 1:
+ self.max_relative_positions = config.max_position_embeddings
+
+ self.position_buckets = getattr(config, "position_buckets", -1)
+ pos_ebd_size = self.max_relative_positions * 2
+
+ if self.position_buckets > 0:
+ pos_ebd_size = self.position_buckets * 2
+
+ self.rel_embeddings = nn.Embedding(pos_ebd_size, config.hidden_size)
+
+ self.norm_rel_ebd = [x.strip() for x in getattr(config, "norm_rel_ebd", "none").lower().split("|")]
+
+ if "layer_norm" in self.norm_rel_ebd:
+ self.LayerNorm = LayerNorm(config.hidden_size, config.layer_norm_eps, elementwise_affine=True)
+
+ self.conv = ConvLayer(config) if getattr(config, "conv_kernel_size", 0) > 0 else None
+
+ def get_rel_embedding(self):
+ rel_embeddings = self.rel_embeddings.weight if self.relative_attention else None
+ if rel_embeddings is not None and ("layer_norm" in self.norm_rel_ebd):
+ rel_embeddings = self.LayerNorm(rel_embeddings)
+ return rel_embeddings
+
+ def get_attention_mask(self, attention_mask):
+ if attention_mask.dim() <= 2:
+ extended_attention_mask = attention_mask.unsqueeze(1).unsqueeze(2)
+ attention_mask = extended_attention_mask * extended_attention_mask.squeeze(-2).unsqueeze(-1)
+ attention_mask = attention_mask.byte()
+ elif attention_mask.dim() == 3:
+ attention_mask = attention_mask.unsqueeze(1)
+
+ return attention_mask
+
+ def get_rel_pos(self, hidden_states, query_states=None, relative_pos=None):
+ if self.relative_attention and relative_pos is None:
+ q = query_states.size(-2) if query_states is not None else hidden_states.size(-2)
+ relative_pos = build_relative_position(
+ q, hidden_states.size(-2), bucket_size=self.position_buckets, max_position=self.max_relative_positions
+ )
+ return relative_pos
+
+ def forward(
+ self,
+ hidden_states,
+ attention_mask,
+ output_hidden_states=True,
+ output_attentions=False,
+ query_states=None,
+ relative_pos=None,
+ return_dict=True,
+ past_key_values=None,
+ ):
+ if attention_mask.dim() <= 2:
+ input_mask = attention_mask
+ else:
+ input_mask = (attention_mask.sum(-2) > 0).byte()
+ attention_mask = self.get_attention_mask(attention_mask)
+ relative_pos = self.get_rel_pos(hidden_states, query_states, relative_pos)
+
+ all_hidden_states = () if output_hidden_states else None
+ all_attentions = () if output_attentions else None
+
+ if isinstance(hidden_states, Sequence): # False
+ next_kv = hidden_states[0]
+ else:
+ next_kv = hidden_states
+ rel_embeddings = self.get_rel_embedding()
+ output_states = next_kv
+ for i, layer_module in enumerate(self.layer):
+
+ if output_hidden_states:
+ all_hidden_states = all_hidden_states + (output_states,)
+
+ past_key_value = past_key_values[i] if past_key_values is not None else None
+
+ output_states = layer_module(
+ next_kv,
+ attention_mask,
+ output_attentions,
+ query_states=query_states,
+ relative_pos=relative_pos,
+ rel_embeddings=rel_embeddings,
+ past_key_value=past_key_value,
+ )
+ if output_attentions:
+ output_states, att_m = output_states
+
+ if i == 0 and self.conv is not None:
+ if past_key_values is not None:
+ past_key_value_length = past_key_values[0][0].shape[2]
+ input_mask = input_mask[:, past_key_value_length:].contiguous()
+ output_states = self.conv(hidden_states, output_states, input_mask)
+
+ if query_states is not None:
+ query_states = output_states
+ if isinstance(hidden_states, Sequence):
+ next_kv = hidden_states[i + 1] if i + 1 < len(self.layer) else None
+ else:
+ next_kv = output_states
+
+ if output_attentions:
+ all_attentions = all_attentions + (att_m,)
+
+ if output_hidden_states:
+ all_hidden_states = all_hidden_states + (output_states,)
+
+ if not return_dict:
+ return tuple(v for v in [output_states, all_hidden_states, all_attentions] if v is not None)
+ return BaseModelOutput(
+ last_hidden_state=output_states, hidden_states=all_hidden_states, attentions=all_attentions
+ )
+
+
+def make_log_bucket_position(relative_pos, bucket_size, max_position):
+ sign = np.sign(relative_pos)
+ mid = bucket_size // 2
+ abs_pos = np.where((relative_pos < mid) & (relative_pos > -mid), mid - 1, np.abs(relative_pos))
+ log_pos = np.ceil(np.log(abs_pos / mid) / np.log((max_position - 1) / mid) * (mid - 1)) + mid
+ bucket_pos = np.where(abs_pos <= mid, relative_pos, log_pos * sign).astype(np.int)
+ return bucket_pos
+
+
+def build_relative_position(query_size, key_size, bucket_size=-1, max_position=-1):
+ """
+ Build relative position according to the query and key
+ We assume the absolute position of query :math:`P_q` is range from (0, query_size) and the absolute position of key
+ :math:`P_k` is range from (0, key_size), The relative positions from query to key is :math:`R_{q \\rightarrow k} =
+ P_q - P_k`
+ Args:
+ query_size (int): the length of query
+ key_size (int): the length of key
+ bucket_size (int): the size of position bucket
+ max_position (int): the maximum allowed absolute position
+ Return:
+ :obj:`torch.LongTensor`: A tensor with shape [1, query_size, key_size]
+ """
+ q_ids = np.arange(0, query_size)
+ k_ids = np.arange(0, key_size)
+ rel_pos_ids = q_ids[:, None] - np.tile(k_ids, (q_ids.shape[0], 1))
+ if bucket_size > 0 and max_position > 0:
+ rel_pos_ids = make_log_bucket_position(rel_pos_ids, bucket_size, max_position)
+ rel_pos_ids = torch.tensor(rel_pos_ids, dtype=torch.long)
+ rel_pos_ids = rel_pos_ids[:query_size, :]
+ rel_pos_ids = rel_pos_ids.unsqueeze(0)
+ return rel_pos_ids
+
+
+@torch.jit.script
+# Copied from transformers.models.deberta.modeling_deberta.c2p_dynamic_expand
+def c2p_dynamic_expand(c2p_pos, query_layer, relative_pos):
+ return c2p_pos.expand([query_layer.size(0), query_layer.size(1), query_layer.size(2), relative_pos.size(-1)])
+
+
+@torch.jit.script
+# Copied from transformers.models.deberta.modeling_deberta.p2c_dynamic_expand
+def p2c_dynamic_expand(c2p_pos, query_layer, key_layer):
+ return c2p_pos.expand([query_layer.size(0), query_layer.size(1), key_layer.size(-2), key_layer.size(-2)])
+
+
+@torch.jit.script
+# Copied from transformers.models.deberta.modeling_deberta.pos_dynamic_expand
+def pos_dynamic_expand(pos_index, p2c_att, key_layer):
+ return pos_index.expand(p2c_att.size()[:2] + (pos_index.size(-2), key_layer.size(-2)))
+
+
+class DisentangledSelfAttention(nn.Module):
+ """
+ Disentangled self-attention module
+ Parameters:
+ config (:obj:`DebertaV2Config`):
+ A model config class instance with the configuration to build a new model. The schema is similar to
+ `BertConfig`, for more details, please refer :class:`~transformers.DebertaV2Config`
+ """
+
+ def __init__(self, config):
+ super().__init__()
+ if config.hidden_size % config.num_attention_heads != 0:
+ raise ValueError(
+ f"The hidden size ({config.hidden_size}) is not a multiple of the number of attention "
+ f"heads ({config.num_attention_heads})"
+ )
+ self.num_attention_heads = config.num_attention_heads
+ _attention_head_size = config.hidden_size // config.num_attention_heads
+ self.attention_head_size = getattr(config, "attention_head_size", _attention_head_size)
+ self.all_head_size = self.num_attention_heads * self.attention_head_size
+ self.query_proj = nn.Linear(config.hidden_size, self.all_head_size, bias=True)
+ self.key_proj = nn.Linear(config.hidden_size, self.all_head_size, bias=True)
+ self.value_proj = nn.Linear(config.hidden_size, self.all_head_size, bias=True)
+
+ self.share_att_key = getattr(config, "share_att_key", False)
+ self.pos_att_type = config.pos_att_type if config.pos_att_type is not None else []
+ self.relative_attention = getattr(config, "relative_attention", False)
+
+ if self.relative_attention:
+ self.position_buckets = getattr(config, "position_buckets", -1)
+ self.max_relative_positions = getattr(config, "max_relative_positions", -1)
+ if self.max_relative_positions < 1:
+ self.max_relative_positions = config.max_position_embeddings
+ self.pos_ebd_size = self.max_relative_positions
+ if self.position_buckets > 0:
+ self.pos_ebd_size = self.position_buckets
+
+ self.pos_dropout = StableDropout(config.hidden_dropout_prob)
+
+ if not self.share_att_key:
+ if "c2p" in self.pos_att_type or "p2p" in self.pos_att_type:
+ self.pos_key_proj = nn.Linear(config.hidden_size, self.all_head_size, bias=True)
+ if "p2c" in self.pos_att_type or "p2p" in self.pos_att_type:
+ self.pos_query_proj = nn.Linear(config.hidden_size, self.all_head_size)
+
+ self.dropout = StableDropout(config.attention_probs_dropout_prob)
+
+ def transpose_for_scores(self, x, attention_heads, past_key_value=None):
+ new_x_shape = x.size()[:-1] + (attention_heads, -1)
+ x = x.view(*new_x_shape)
+ x = x.permute(0, 2, 1, 3)
+ if past_key_value is not None:
+ x = torch.cat([past_key_value, x], dim=2)
+ new_x_shape = x.shape
+ return x.contiguous().view(-1, new_x_shape[2], new_x_shape[-1])
+ # return x.permute(0, 2, 1, 3).contiguous().view(-1, x.size(1), x.size(-1))
+
+ def forward(
+ self,
+ hidden_states,
+ attention_mask,
+ return_att=False,
+ query_states=None,
+ relative_pos=None,
+ rel_embeddings=None,
+ past_key_value=None,
+ ):
+ """
+ Call the module
+ Args:
+ hidden_states (:obj:`torch.FloatTensor`):
+ Input states to the module usually the output from previous layer, it will be the Q,K and V in
+ `Attention(Q,K,V)`
+ attention_mask (:obj:`torch.ByteTensor`):
+ An attention mask matrix of shape [`B`, `N`, `N`] where `B` is the batch size, `N` is the maximum
+ sequence length in which element [i,j] = `1` means the `i` th token in the input can attend to the `j`
+ th token.
+ return_att (:obj:`bool`, optional):
+ Whether return the attention matrix.
+ query_states (:obj:`torch.FloatTensor`, optional):
+ The `Q` state in `Attention(Q,K,V)`.
+ relative_pos (:obj:`torch.LongTensor`):
+ The relative position encoding between the tokens in the sequence. It's of shape [`B`, `N`, `N`] with
+ values ranging in [`-max_relative_positions`, `max_relative_positions`].
+ rel_embeddings (:obj:`torch.FloatTensor`):
+ The embedding of relative distances. It's a tensor of shape [:math:`2 \\times
+ \\text{max_relative_positions}`, `hidden_size`].
+ """
+ if query_states is None:
+ query_states = hidden_states
+
+ past_key_value_length = past_key_value.shape[3] if past_key_value is not None else 0
+ if past_key_value is not None:
+ key_layer_prefix = self.transpose_for_scores(self.key_proj(hidden_states), self.num_attention_heads, past_key_value=past_key_value[0])
+ # value_layer_prefix = self.transpose_for_scores(self.value_proj(hidden_states), self.num_attention_heads, past_key_value=past_key_value[1])
+
+ query_layer = self.transpose_for_scores(self.query_proj(query_states), self.num_attention_heads)
+ key_layer = self.transpose_for_scores(self.key_proj(hidden_states), self.num_attention_heads)
+ value_layer = self.transpose_for_scores(self.value_proj(hidden_states), self.num_attention_heads, past_key_value=past_key_value[1])
+
+ rel_att = None
+ # Take the dot product between "query" and "key" to get the raw attention scores.
+ scale_factor = 1
+ if "c2p" in self.pos_att_type:
+ scale_factor += 1
+ if "p2c" in self.pos_att_type:
+ scale_factor += 1
+ if "p2p" in self.pos_att_type:
+ scale_factor += 1
+ scale = math.sqrt(query_layer.size(-1) * scale_factor)
+ # attention_scores = torch.bmm(query_layer, key_layer.transpose(-1, -2)) / scale
+ attention_scores = torch.bmm(query_layer, key_layer_prefix.transpose(-1, -2)) / scale
+
+ if self.relative_attention:
+ rel_embeddings = self.pos_dropout(rel_embeddings)
+ rel_att = self.disentangled_attention_bias(
+ query_layer, key_layer, relative_pos, rel_embeddings, scale_factor
+ )
+
+ if rel_att is not None:
+ if past_key_value is not None:
+ att_shape = rel_att.shape[:-1] + (past_key_value_length,)
+ prefix_att = torch.zeros(*att_shape).to(rel_att.device)
+ attention_scores = attention_scores + torch.cat([prefix_att, rel_att], dim=-1)
+ else:
+ attention_scores = attention_scores + rel_att
+ # print(attention_scores.shape)
+ attention_scores = attention_scores
+ attention_scores = attention_scores.view(
+ -1, self.num_attention_heads, attention_scores.size(-2), attention_scores.size(-1)
+ )
+
+ # bsz x height x length x dimension
+ attention_mask = attention_mask[:,:, past_key_value_length:,:]
+
+ attention_probs = XSoftmax.apply(attention_scores, attention_mask, -1)
+ attention_probs = self.dropout(attention_probs)
+
+ context_layer = torch.bmm(
+ attention_probs.view(-1, attention_probs.size(-2), attention_probs.size(-1)), value_layer
+ )
+ context_layer = (
+ context_layer.view(-1, self.num_attention_heads, context_layer.size(-2), context_layer.size(-1))
+ .permute(0, 2, 1, 3)
+ .contiguous()
+ )
+ new_context_layer_shape = context_layer.size()[:-2] + (-1,)
+ context_layer = context_layer.view(*new_context_layer_shape)
+ if return_att:
+ return (context_layer, attention_probs)
+ else:
+ return context_layer
+
+ def disentangled_attention_bias(self, query_layer, key_layer, relative_pos, rel_embeddings, scale_factor):
+ if relative_pos is None:
+ q = query_layer.size(-2)
+ relative_pos = build_relative_position(
+ q, key_layer.size(-2), bucket_size=self.position_buckets, max_position=self.max_relative_positions
+ )
+ if relative_pos.dim() == 2:
+ relative_pos = relative_pos.unsqueeze(0).unsqueeze(0)
+ elif relative_pos.dim() == 3:
+ relative_pos = relative_pos.unsqueeze(1)
+ # bsz x height x query x key
+ elif relative_pos.dim() != 4:
+ raise ValueError(f"Relative position ids must be of dim 2 or 3 or 4. {relative_pos.dim()}")
+
+ att_span = self.pos_ebd_size
+ relative_pos = relative_pos.long().to(query_layer.device)
+
+ rel_embeddings = rel_embeddings[self.pos_ebd_size - att_span : self.pos_ebd_size + att_span, :].unsqueeze(0)
+ if self.share_att_key: # True
+ pos_query_layer = self.transpose_for_scores(
+ self.query_proj(rel_embeddings), self.num_attention_heads
+ ).repeat(query_layer.size(0) // self.num_attention_heads, 1, 1)
+ pos_key_layer = self.transpose_for_scores(self.key_proj(rel_embeddings), self.num_attention_heads).repeat(
+ query_layer.size(0) // self.num_attention_heads, 1, 1
+ )
+ else:
+ if "c2p" in self.pos_att_type or "p2p" in self.pos_att_type:
+ pos_key_layer = self.transpose_for_scores(
+ self.pos_key_proj(rel_embeddings), self.num_attention_heads
+ ).repeat(
+ query_layer.size(0) // self.num_attention_heads, 1, 1
+ ) # .split(self.all_head_size, dim=-1)
+ if "p2c" in self.pos_att_type or "p2p" in self.pos_att_type:
+ pos_query_layer = self.transpose_for_scores(
+ self.pos_query_proj(rel_embeddings), self.num_attention_heads
+ ).repeat(
+ query_layer.size(0) // self.num_attention_heads, 1, 1
+ ) # .split(self.all_head_size, dim=-1)
+
+ score = 0
+ # content->position
+ if "c2p" in self.pos_att_type:
+ scale = math.sqrt(pos_key_layer.size(-1) * scale_factor)
+ c2p_att = torch.bmm(query_layer, pos_key_layer.transpose(-1, -2))
+ c2p_pos = torch.clamp(relative_pos + att_span, 0, att_span * 2 - 1)
+ c2p_att = torch.gather(
+ c2p_att,
+ dim=-1,
+ index=c2p_pos.squeeze(0).expand([query_layer.size(0), query_layer.size(1), relative_pos.size(-1)]),
+ )
+ score += c2p_att / scale
+
+ # position->content
+ if "p2c" in self.pos_att_type or "p2p" in self.pos_att_type:
+ scale = math.sqrt(pos_query_layer.size(-1) * scale_factor)
+ if key_layer.size(-2) != query_layer.size(-2):
+ r_pos = build_relative_position(
+ key_layer.size(-2),
+ key_layer.size(-2),
+ bucket_size=self.position_buckets,
+ max_position=self.max_relative_positions,
+ ).to(query_layer.device)
+ r_pos = r_pos.unsqueeze(0)
+ else:
+ r_pos = relative_pos
+
+ p2c_pos = torch.clamp(-r_pos + att_span, 0, att_span * 2 - 1)
+ if query_layer.size(-2) != key_layer.size(-2):
+ pos_index = relative_pos[:, :, :, 0].unsqueeze(-1)
+
+ if "p2c" in self.pos_att_type:
+ p2c_att = torch.bmm(key_layer, pos_query_layer.transpose(-1, -2))
+ p2c_att = torch.gather(
+ p2c_att,
+ dim=-1,
+ index=p2c_pos.squeeze(0).expand([query_layer.size(0), key_layer.size(-2), key_layer.size(-2)]),
+ ).transpose(-1, -2)
+ if query_layer.size(-2) != key_layer.size(-2):
+ p2c_att = torch.gather(
+ p2c_att,
+ dim=-2,
+ index=pos_index.expand(p2c_att.size()[:2] + (pos_index.size(-2), key_layer.size(-2))),
+ )
+ score += p2c_att / scale
+
+ # position->position
+ if "p2p" in self.pos_att_type:
+ pos_query = pos_query_layer[:, :, att_span:, :]
+ p2p_att = torch.matmul(pos_query, pos_key_layer.transpose(-1, -2))
+ p2p_att = p2p_att.expand(query_layer.size()[:2] + p2p_att.size()[2:])
+ if query_layer.size(-2) != key_layer.size(-2):
+ p2p_att = torch.gather(
+ p2p_att,
+ dim=-2,
+ index=pos_index.expand(query_layer.size()[:2] + (pos_index.size(-2), p2p_att.size(-1))),
+ )
+ p2p_att = torch.gather(
+ p2p_att,
+ dim=-1,
+ index=c2p_pos.expand(
+ [query_layer.size(0), query_layer.size(1), query_layer.size(2), relative_pos.size(-1)]
+ ),
+ )
+ score += p2p_att
+
+ return score
+
+
+# Copied from transformers.models.deberta.modeling_deberta.DebertaEmbeddings with DebertaLayerNorm->LayerNorm
+class DebertaV2Embeddings(nn.Module):
+ """Construct the embeddings from word, position and token_type embeddings."""
+
+ def __init__(self, config):
+ super().__init__()
+ pad_token_id = getattr(config, "pad_token_id", 0)
+ self.embedding_size = getattr(config, "embedding_size", config.hidden_size)
+ self.word_embeddings = nn.Embedding(config.vocab_size, self.embedding_size, padding_idx=pad_token_id)
+
+ self.position_biased_input = getattr(config, "position_biased_input", True)
+ if not self.position_biased_input:
+ self.position_embeddings = None
+ else:
+ self.position_embeddings = nn.Embedding(config.max_position_embeddings, self.embedding_size)
+
+ if config.type_vocab_size > 0:
+ self.token_type_embeddings = nn.Embedding(config.type_vocab_size, self.embedding_size)
+
+ if self.embedding_size != config.hidden_size:
+ self.embed_proj = nn.Linear(self.embedding_size, config.hidden_size, bias=False)
+ self.LayerNorm = LayerNorm(config.hidden_size, config.layer_norm_eps)
+ self.dropout = StableDropout(config.hidden_dropout_prob)
+ self.config = config
+
+ # position_ids (1, len position emb) is contiguous in memory and exported when serialized
+ self.register_buffer("position_ids", torch.arange(config.max_position_embeddings).expand((1, -1)))
+
+ def forward(self, input_ids=None, token_type_ids=None, position_ids=None, mask=None, inputs_embeds=None, past_key_values_length=0,):
+ if input_ids is not None:
+ input_shape = input_ids.size()
+ else:
+ input_shape = inputs_embeds.size()[:-1]
+
+ seq_length = input_shape[1]
+
+ if position_ids is None:
+ # position_ids = self.position_ids[:, :seq_length]
+ position_ids = self.position_ids[:, past_key_values_length : seq_length + past_key_values_length]
+
+ if token_type_ids is None:
+ token_type_ids = torch.zeros(input_shape, dtype=torch.long, device=self.position_ids.device)
+
+ if inputs_embeds is None:
+ inputs_embeds = self.word_embeddings(input_ids)
+
+ if self.position_embeddings is not None:
+ position_embeddings = self.position_embeddings(position_ids.long())
+ else:
+ position_embeddings = torch.zeros_like(inputs_embeds)
+
+ embeddings = inputs_embeds
+ if self.position_biased_input:
+ embeddings += position_embeddings
+ if self.config.type_vocab_size > 0:
+ token_type_embeddings = self.token_type_embeddings(token_type_ids)
+ embeddings += token_type_embeddings
+
+ if self.embedding_size != self.config.hidden_size:
+ embeddings = self.embed_proj(embeddings)
+
+ embeddings = self.LayerNorm(embeddings)
+
+ if mask is not None:
+ if mask.dim() != embeddings.dim():
+ if mask.dim() == 4:
+ mask = mask.squeeze(1).squeeze(1)
+ mask = mask.unsqueeze(2)
+ mask = mask.to(embeddings.dtype)
+
+ embeddings = embeddings * mask
+
+ embeddings = self.dropout(embeddings)
+ return embeddings
+
+
+# Copied from transformers.models.deberta.modeling_deberta.DebertaPreTrainedModel with Deberta->DebertaV2
+class DebertaV2PreTrainedModel(PreTrainedModel):
+ """
+ An abstract class to handle weights initialization and a simple interface for downloading and loading pretrained
+ models.
+ """
+
+ config_class = DebertaV2Config
+ base_model_prefix = "deberta"
+ _keys_to_ignore_on_load_missing = ["position_ids"]
+ _keys_to_ignore_on_load_unexpected = ["position_embeddings"]
+
+ def __init__(self, config):
+ super().__init__(config)
+ self._register_load_state_dict_pre_hook(self._pre_load_hook)
+
+ def _init_weights(self, module):
+ """Initialize the weights."""
+ if isinstance(module, nn.Linear):
+ # Slightly different from the TF version which uses truncated_normal for initialization
+ # cf https://github.com/pytorch/pytorch/pull/5617
+ module.weight.data.normal_(mean=0.0, std=self.config.initializer_range)
+ if module.bias is not None:
+ module.bias.data.zero_()
+ elif isinstance(module, nn.Embedding):
+ module.weight.data.normal_(mean=0.0, std=self.config.initializer_range)
+ if module.padding_idx is not None:
+ module.weight.data[module.padding_idx].zero_()
+
+ def _pre_load_hook(self, state_dict, prefix, local_metadata, strict, missing_keys, unexpected_keys, error_msgs):
+ """
+ Removes the classifier if it doesn't have the correct number of labels.
+ """
+ self_state = self.state_dict()
+ if (
+ ("classifier.weight" in self_state)
+ and ("classifier.weight" in state_dict)
+ and self_state["classifier.weight"].size() != state_dict["classifier.weight"].size()
+ ):
+ logger.warning(
+ f"The checkpoint classifier head has a shape {state_dict['classifier.weight'].size()} and this model "
+ f"classifier head has a shape {self_state['classifier.weight'].size()}. Ignoring the checkpoint "
+ f"weights. You should train your model on new data."
+ )
+ del state_dict["classifier.weight"]
+ if "classifier.bias" in state_dict:
+ del state_dict["classifier.bias"]
+
+
+DEBERTA_START_DOCSTRING = r"""
+ The DeBERTa model was proposed in `DeBERTa: Decoding-enhanced BERT with Disentangled Attention
+ `_ by Pengcheng He, Xiaodong Liu, Jianfeng Gao, Weizhu Chen. It's build on top of
+ BERT/RoBERTa with two improvements, i.e. disentangled attention and enhanced mask decoder. With those two
+ improvements, it out perform BERT/RoBERTa on a majority of tasks with 80GB pretraining data.
+ This model is also a PyTorch `torch.nn.Module `__
+ subclass. Use it as a regular PyTorch Module and refer to the PyTorch documentation for all matter related to
+ general usage and behavior.```
+ Parameters:
+ config (:class:`~transformers.DebertaV2Config`): Model configuration class with all the parameters of the model.
+ Initializing with a config file does not load the weights associated with the model, only the
+ configuration. Check out the :meth:`~transformers.PreTrainedModel.from_pretrained` method to load the model
+ weights.
+"""
+
+DEBERTA_INPUTS_DOCSTRING = r"""
+ Args:
+ input_ids (:obj:`torch.LongTensor` of shape :obj:`{0}`):
+ Indices of input sequence tokens in the vocabulary.
+ Indices can be obtained using :class:`transformers.DebertaV2Tokenizer`. See
+ :func:`transformers.PreTrainedTokenizer.encode` and :func:`transformers.PreTrainedTokenizer.__call__` for
+ details.
+ `What are input IDs? <../glossary.html#input-ids>`__
+ attention_mask (:obj:`torch.FloatTensor` of shape :obj:`{0}`, `optional`):
+ Mask to avoid performing attention on padding token indices. Mask values selected in ``[0, 1]``:
+ - 1 for tokens that are **not masked**,
+ - 0 for tokens that are **masked**.
+ `What are attention masks? <../glossary.html#attention-mask>`__
+ token_type_ids (:obj:`torch.LongTensor` of shape :obj:`{0}`, `optional`):
+ Segment token indices to indicate first and second portions of the inputs. Indices are selected in ``[0,
+ 1]``:
+ - 0 corresponds to a `sentence A` token,
+ - 1 corresponds to a `sentence B` token.
+ `What are token type IDs? <../glossary.html#token-type-ids>`_
+ position_ids (:obj:`torch.LongTensor` of shape :obj:`{0}`, `optional`):
+ Indices of positions of each input sequence tokens in the position embeddings. Selected in the range ``[0,
+ config.max_position_embeddings - 1]``.
+ `What are position IDs? <../glossary.html#position-ids>`_
+ inputs_embeds (:obj:`torch.FloatTensor` of shape :obj:`(batch_size, sequence_length, hidden_size)`, `optional`):
+ Optionally, instead of passing :obj:`input_ids` you can choose to directly pass an embedded representation.
+ This is useful if you want more control over how to convert `input_ids` indices into associated vectors
+ than the model's internal embedding lookup matrix.
+ output_attentions (:obj:`bool`, `optional`):
+ Whether or not to return the attentions tensors of all attention layers. See ``attentions`` under returned
+ tensors for more detail.
+ output_hidden_states (:obj:`bool`, `optional`):
+ Whether or not to return the hidden states of all layers. See ``hidden_states`` under returned tensors for
+ more detail.
+ return_dict (:obj:`bool`, `optional`):
+ Whether or not to return a :class:`~transformers.file_utils.ModelOutput` instead of a plain tuple.
+"""
+
+
+@add_start_docstrings(
+ "The bare DeBERTa Model transformer outputting raw hidden-states without any specific head on top.",
+ DEBERTA_START_DOCSTRING,
+)
+# Copied from transformers.models.deberta.modeling_deberta.DebertaModel with Deberta->DebertaV2
+class DebertaV2Model(DebertaV2PreTrainedModel):
+ def __init__(self, config):
+ super().__init__(config)
+
+ self.embeddings = DebertaV2Embeddings(config)
+ self.encoder = DebertaV2Encoder(config)
+ self.z_steps = 0
+ self.config = config
+ self.init_weights()
+
+ def get_input_embeddings(self):
+ return self.embeddings.word_embeddings
+
+ def set_input_embeddings(self, new_embeddings):
+ self.embeddings.word_embeddings = new_embeddings
+
+ def _prune_heads(self, heads_to_prune):
+ """
+ Prunes heads of the model. heads_to_prune: dict of {layer_num: list of heads to prune in this layer} See base
+ class PreTrainedModel
+ """
+ raise NotImplementedError("The prune function is not implemented in DeBERTa model.")
+
+ @add_start_docstrings_to_model_forward(DEBERTA_INPUTS_DOCSTRING.format("batch_size, sequence_length"))
+ @add_code_sample_docstrings(
+ # tokenizer_class=_TOKENIZER_FOR_DOC,
+ checkpoint=_CHECKPOINT_FOR_DOC,
+ output_type=SequenceClassifierOutput,
+ config_class=_CONFIG_FOR_DOC,
+ )
+ def forward(
+ self,
+ input_ids=None,
+ attention_mask=None,
+ token_type_ids=None,
+ position_ids=None,
+ inputs_embeds=None,
+ output_attentions=None,
+ output_hidden_states=None,
+ return_dict=None,
+ past_key_values=None,
+ ):
+ output_attentions = output_attentions if output_attentions is not None else self.config.output_attentions
+ output_hidden_states = (
+ output_hidden_states if output_hidden_states is not None else self.config.output_hidden_states
+ )
+ return_dict = return_dict if return_dict is not None else self.config.use_return_dict
+
+ if input_ids is not None and inputs_embeds is not None:
+ raise ValueError("You cannot specify both input_ids and inputs_embeds at the same time")
+ elif input_ids is not None:
+ input_shape = input_ids.size()
+ batch_size, seq_length = input_shape
+ elif inputs_embeds is not None:
+ input_shape = inputs_embeds.size()[:-1]
+ batch_size, seq_length = input_shape
+ else:
+ raise ValueError("You have to specify either input_ids or inputs_embeds")
+
+ device = input_ids.device if input_ids is not None else inputs_embeds.device
+
+ past_key_values_length = past_key_values[0][0].shape[2] if past_key_values is not None else 0
+
+ embedding_mask = torch.ones(input_shape, device=device)
+ if attention_mask is None:
+ # attention_mask = torch.ones(input_shape, device=device)
+ attention_mask = torch.ones(((batch_size, seq_length + past_key_values_length)), device=device)
+ if token_type_ids is None:
+ token_type_ids = torch.zeros(input_shape, dtype=torch.long, device=device)
+
+ embedding_output = self.embeddings(
+ input_ids=input_ids,
+ token_type_ids=token_type_ids,
+ position_ids=position_ids,
+ # mask=attention_mask,
+ mask=embedding_mask,
+ inputs_embeds=inputs_embeds,
+ past_key_values_length=past_key_values_length, # Ongoing
+ )
+
+ encoder_outputs = self.encoder(
+ embedding_output,
+ attention_mask,
+ output_hidden_states=True,
+ output_attentions=output_attentions,
+ return_dict=return_dict,
+ past_key_values=past_key_values, # Ongoing
+ )
+ encoded_layers = encoder_outputs[1]
+
+ if self.z_steps > 1:
+ hidden_states = encoded_layers[-2]
+ layers = [self.encoder.layer[-1] for _ in range(self.z_steps)]
+ query_states = encoded_layers[-1]
+ rel_embeddings = self.encoder.get_rel_embedding()
+ attention_mask = self.encoder.get_attention_mask(attention_mask)
+ rel_pos = self.encoder.get_rel_pos(embedding_output)
+ for layer in layers[1:]:
+ query_states = layer(
+ hidden_states,
+ attention_mask,
+ return_att=False,
+ query_states=query_states,
+ relative_pos=rel_pos,
+ rel_embeddings=rel_embeddings,
+ )
+ encoded_layers.append(query_states)
+
+ sequence_output = encoded_layers[-1]
+
+ if not return_dict:
+ return (sequence_output,) + encoder_outputs[(1 if output_hidden_states else 2) :]
+
+ return BaseModelOutput(
+ last_hidden_state=sequence_output,
+ hidden_states=encoder_outputs.hidden_states if output_hidden_states else None,
+ attentions=encoder_outputs.attentions,
+ )
+
+
+@add_start_docstrings("""DeBERTa Model with a `language modeling` head on top. """, DEBERTA_START_DOCSTRING)
+# Copied from transformers.models.deberta.modeling_deberta.DebertaForMaskedLM with Deberta->DebertaV2
+class DebertaV2ForMaskedLM(DebertaV2PreTrainedModel):
+ _keys_to_ignore_on_load_unexpected = [r"pooler"]
+ _keys_to_ignore_on_load_missing = [r"position_ids", r"predictions.decoder.bias"]
+
+ def __init__(self, config):
+ super().__init__(config)
+
+ self.deberta = DebertaV2Model(config)
+ self.cls = DebertaV2OnlyMLMHead(config)
+
+ self.init_weights()
+
+ def get_output_embeddings(self):
+ return self.cls.predictions.decoder
+
+ def set_output_embeddings(self, new_embeddings):
+ self.cls.predictions.decoder = new_embeddings
+
+ @add_start_docstrings_to_model_forward(DEBERTA_INPUTS_DOCSTRING.format("batch_size, sequence_length"))
+ @add_code_sample_docstrings(
+ # tokenizer_class=_TOKENIZER_FOR_DOC,
+ checkpoint=_CHECKPOINT_FOR_DOC,
+ output_type=MaskedLMOutput,
+ config_class=_CONFIG_FOR_DOC,
+ )
+ def forward(
+ self,
+ input_ids=None,
+ attention_mask=None,
+ token_type_ids=None,
+ position_ids=None,
+ inputs_embeds=None,
+ labels=None,
+ output_attentions=None,
+ output_hidden_states=None,
+ return_dict=None,
+ ):
+ r"""
+ labels (:obj:`torch.LongTensor` of shape :obj:`(batch_size, sequence_length)`, `optional`):
+ Labels for computing the masked language modeling loss. Indices should be in ``[-100, 0, ...,
+ config.vocab_size]`` (see ``input_ids`` docstring) Tokens with indices set to ``-100`` are ignored
+ (masked), the loss is only computed for the tokens with labels in ``[0, ..., config.vocab_size]``
+ """
+
+ return_dict = return_dict if return_dict is not None else self.config.use_return_dict
+
+ outputs = self.deberta(
+ input_ids,
+ attention_mask=attention_mask,
+ token_type_ids=token_type_ids,
+ position_ids=position_ids,
+ inputs_embeds=inputs_embeds,
+ output_attentions=output_attentions,
+ output_hidden_states=output_hidden_states,
+ return_dict=return_dict,
+ )
+
+ sequence_output = outputs[0]
+ prediction_scores = self.cls(sequence_output)
+
+ masked_lm_loss = None
+ if labels is not None:
+ loss_fct = CrossEntropyLoss() # -100 index = padding token
+ masked_lm_loss = loss_fct(prediction_scores.view(-1, self.config.vocab_size), labels.view(-1))
+
+ if not return_dict:
+ output = (prediction_scores,) + outputs[1:]
+ return ((masked_lm_loss,) + output) if masked_lm_loss is not None else output
+
+ return MaskedLMOutput(
+ loss=masked_lm_loss,
+ logits=prediction_scores,
+ hidden_states=outputs.hidden_states,
+ attentions=outputs.attentions,
+ )
+
+
+# copied from transformers.models.bert.BertPredictionHeadTransform with bert -> deberta
+class DebertaV2PredictionHeadTransform(nn.Module):
+ def __init__(self, config):
+ super().__init__()
+ self.dense = nn.Linear(config.hidden_size, config.hidden_size)
+ if isinstance(config.hidden_act, str):
+ self.transform_act_fn = ACT2FN[config.hidden_act]
+ else:
+ self.transform_act_fn = config.hidden_act
+ self.LayerNorm = nn.LayerNorm(config.hidden_size, eps=config.layer_norm_eps)
+
+ def forward(self, hidden_states):
+ hidden_states = self.dense(hidden_states)
+ hidden_states = self.transform_act_fn(hidden_states)
+ hidden_states = self.LayerNorm(hidden_states)
+ return hidden_states
+
+
+# copied from transformers.models.bert.BertLMPredictionHead with bert -> deberta
+class DebertaV2LMPredictionHead(nn.Module):
+ def __init__(self, config):
+ super().__init__()
+ self.transform = DebertaV2PredictionHeadTransform(config)
+
+ # The output weights are the same as the input embeddings, but there is
+ # an output-only bias for each token.
+ self.decoder = nn.Linear(config.hidden_size, config.vocab_size, bias=False)
+
+ self.bias = nn.Parameter(torch.zeros(config.vocab_size))
+
+ # Need a link between the two variables so that the bias is correctly resized with `resize_token_embeddings`
+ self.decoder.bias = self.bias
+
+ def forward(self, hidden_states):
+ hidden_states = self.transform(hidden_states)
+ hidden_states = self.decoder(hidden_states)
+ return hidden_states
+
+
+# copied from transformers.models.bert.BertOnlyMLMHead with bert -> deberta
+class DebertaV2OnlyMLMHead(nn.Module):
+ def __init__(self, config):
+ super().__init__()
+ self.predictions = DebertaV2LMPredictionHead(config)
+
+ def forward(self, sequence_output):
+ prediction_scores = self.predictions(sequence_output)
+ return prediction_scores
+
+
+@add_start_docstrings(
+ """
+ DeBERTa Model transformer with a sequence classification/regression head on top (a linear layer on top of the
+ pooled output) e.g. for GLUE tasks.
+ """,
+ DEBERTA_START_DOCSTRING,
+)
+# Copied from transformers.models.deberta.modeling_deberta.DebertaForSequenceClassification with Deberta->DebertaV2
+class DebertaV2ForSequenceClassification(DebertaV2PreTrainedModel):
+ def __init__(self, config):
+ super().__init__(config)
+
+ num_labels = getattr(config, "num_labels", 2)
+ self.num_labels = num_labels
+
+ self.deberta = DebertaV2Model(config)
+ self.pooler = ContextPooler(config)
+ output_dim = self.pooler.output_dim
+
+ self.classifier = nn.Linear(output_dim, num_labels)
+ drop_out = getattr(config, "cls_dropout", None)
+ drop_out = self.config.hidden_dropout_prob if drop_out is None else drop_out
+ self.dropout = StableDropout(drop_out)
+
+ self.init_weights()
+
+ def get_input_embeddings(self):
+ return self.deberta.get_input_embeddings()
+
+ def set_input_embeddings(self, new_embeddings):
+ self.deberta.set_input_embeddings(new_embeddings)
+
+ @add_start_docstrings_to_model_forward(DEBERTA_INPUTS_DOCSTRING.format("batch_size, sequence_length"))
+ @add_code_sample_docstrings(
+ # tokenizer_class=_TOKENIZER_FOR_DOC,
+ checkpoint=_CHECKPOINT_FOR_DOC,
+ output_type=SequenceClassifierOutput,
+ config_class=_CONFIG_FOR_DOC,
+ )
+ def forward(
+ self,
+ input_ids=None,
+ attention_mask=None,
+ token_type_ids=None,
+ position_ids=None,
+ inputs_embeds=None,
+ labels=None,
+ output_attentions=None,
+ output_hidden_states=None,
+ return_dict=None,
+ ):
+ r"""
+ labels (:obj:`torch.LongTensor` of shape :obj:`(batch_size,)`, `optional`):
+ Labels for computing the sequence classification/regression loss. Indices should be in :obj:`[0, ...,
+ config.num_labels - 1]`. If :obj:`config.num_labels == 1` a regression loss is computed (Mean-Square loss),
+ If :obj:`config.num_labels > 1` a classification loss is computed (Cross-Entropy).
+ """
+ return_dict = return_dict if return_dict is not None else self.config.use_return_dict
+
+ outputs = self.deberta(
+ input_ids,
+ token_type_ids=token_type_ids,
+ attention_mask=attention_mask,
+ position_ids=position_ids,
+ inputs_embeds=inputs_embeds,
+ output_attentions=output_attentions,
+ output_hidden_states=output_hidden_states,
+ return_dict=return_dict,
+ )
+
+ encoder_layer = outputs[0]
+ pooled_output = self.pooler(encoder_layer)
+ pooled_output = self.dropout(pooled_output)
+ logits = self.classifier(pooled_output)
+
+ loss = None
+ if labels is not None:
+ if self.num_labels == 1:
+ # regression task
+ loss_fn = nn.MSELoss()
+ logits = logits.view(-1).to(labels.dtype)
+ loss = loss_fn(logits, labels.view(-1))
+ elif labels.dim() == 1 or labels.size(-1) == 1:
+ label_index = (labels >= 0).nonzero()
+ labels = labels.long()
+ if label_index.size(0) > 0:
+ labeled_logits = torch.gather(logits, 0, label_index.expand(label_index.size(0), logits.size(1)))
+ labels = torch.gather(labels, 0, label_index.view(-1))
+ loss_fct = CrossEntropyLoss()
+ loss = loss_fct(labeled_logits.view(-1, self.num_labels).float(), labels.view(-1))
+ else:
+ loss = torch.tensor(0).to(logits)
+ else:
+ log_softmax = nn.LogSoftmax(-1)
+ loss = -((log_softmax(logits) * labels).sum(-1)).mean()
+ if not return_dict:
+ output = (logits,) + outputs[1:]
+ return ((loss,) + output) if loss is not None else output
+ else:
+ return SequenceClassifierOutput(
+ loss=loss,
+ logits=logits,
+ hidden_states=outputs.hidden_states,
+ attentions=outputs.attentions,
+ )
+
+
+@add_start_docstrings(
+ """
+ DeBERTa Model with a token classification head on top (a linear layer on top of the hidden-states output) e.g. for
+ Named-Entity-Recognition (NER) tasks.
+ """,
+ DEBERTA_START_DOCSTRING,
+)
+# Copied from transformers.models.deberta.modeling_deberta.DebertaForTokenClassification with Deberta->DebertaV2
+class DebertaV2ForTokenClassification(DebertaV2PreTrainedModel):
+ _keys_to_ignore_on_load_unexpected = [r"pooler"]
+
+ def __init__(self, config):
+ super().__init__(config)
+ self.num_labels = config.num_labels
+
+ self.deberta = DebertaV2Model(config)
+ self.dropout = nn.Dropout(config.hidden_dropout_prob)
+ self.classifier = nn.Linear(config.hidden_size, config.num_labels)
+
+ self.init_weights()
+ for param in self.deberta.parameters():
+ param.requires_grad = False
+
+ @add_start_docstrings_to_model_forward(DEBERTA_INPUTS_DOCSTRING.format("batch_size, sequence_length"))
+ @add_code_sample_docstrings(
+ # tokenizer_class=_TOKENIZER_FOR_DOC,
+ checkpoint=_CHECKPOINT_FOR_DOC,
+ output_type=TokenClassifierOutput,
+ config_class=_CONFIG_FOR_DOC,
+ )
+ def forward(
+ self,
+ input_ids=None,
+ attention_mask=None,
+ token_type_ids=None,
+ position_ids=None,
+ inputs_embeds=None,
+ labels=None,
+ output_attentions=None,
+ output_hidden_states=None,
+ return_dict=None,
+ past_key_values=None,
+ ):
+ r"""
+ labels (:obj:`torch.LongTensor` of shape :obj:`(batch_size, sequence_length)`, `optional`):
+ Labels for computing the token classification loss. Indices should be in ``[0, ..., config.num_labels -
+ 1]``.
+ """
+ return_dict = return_dict if return_dict is not None else self.config.use_return_dict
+
+ outputs = self.deberta(
+ input_ids,
+ attention_mask=attention_mask,
+ token_type_ids=token_type_ids,
+ position_ids=position_ids,
+ inputs_embeds=inputs_embeds,
+ output_attentions=output_attentions,
+ output_hidden_states=output_hidden_states,
+ return_dict=return_dict,
+ )
+
+ sequence_output = outputs[0]
+
+ sequence_output = self.dropout(sequence_output)
+ logits = self.classifier(sequence_output)
+
+ loss = None
+ if labels is not None:
+ loss_fct = CrossEntropyLoss()
+ # Only keep active parts of the loss
+ if attention_mask is not None:
+ active_loss = attention_mask.view(-1) == 1
+ active_logits = logits.view(-1, self.num_labels)
+ active_labels = torch.where(
+ active_loss, labels.view(-1), torch.tensor(loss_fct.ignore_index).type_as(labels)
+ )
+ loss = loss_fct(active_logits, active_labels)
+ else:
+ loss = loss_fct(logits.view(-1, self.num_labels), labels.view(-1))
+
+ if not return_dict:
+ output = (logits,) + outputs[1:]
+ return ((loss,) + output) if loss is not None else output
+
+ return TokenClassifierOutput(
+ loss=loss,
+ logits=logits,
+ hidden_states=outputs.hidden_states,
+ attentions=outputs.attentions,
+ )
+
+
+@add_start_docstrings(
+ """
+ DeBERTa Model with a span classification head on top for extractive question-answering tasks like SQuAD (a linear
+ layers on top of the hidden-states output to compute `span start logits` and `span end logits`).
+ """,
+ DEBERTA_START_DOCSTRING,
+)
+# Copied from transformers.models.deberta.modeling_deberta.DebertaForQuestionAnswering with Deberta->DebertaV2
+class DebertaV2ForQuestionAnswering(DebertaV2PreTrainedModel):
+ _keys_to_ignore_on_load_unexpected = [r"pooler"]
+
+ def __init__(self, config):
+ super().__init__(config)
+ self.num_labels = config.num_labels
+
+ self.deberta = DebertaV2Model(config)
+ self.qa_outputs = nn.Linear(config.hidden_size, config.num_labels)
+
+ self.init_weights()
+
+ @add_start_docstrings_to_model_forward(DEBERTA_INPUTS_DOCSTRING.format("batch_size, sequence_length"))
+ @add_code_sample_docstrings(
+ # tokenizer_class=_TOKENIZER_FOR_DOC,
+ checkpoint=_CHECKPOINT_FOR_DOC,
+ output_type=QuestionAnsweringModelOutput,
+ config_class=_CONFIG_FOR_DOC,
+ )
+ def forward(
+ self,
+ input_ids=None,
+ attention_mask=None,
+ token_type_ids=None,
+ position_ids=None,
+ inputs_embeds=None,
+ start_positions=None,
+ end_positions=None,
+ output_attentions=None,
+ output_hidden_states=None,
+ return_dict=None,
+ ):
+ r"""
+ start_positions (:obj:`torch.LongTensor` of shape :obj:`(batch_size,)`, `optional`):
+ Labels for position (index) of the start of the labelled span for computing the token classification loss.
+ Positions are clamped to the length of the sequence (:obj:`sequence_length`). Position outside of the
+ sequence are not taken into account for computing the loss.
+ end_positions (:obj:`torch.LongTensor` of shape :obj:`(batch_size,)`, `optional`):
+ Labels for position (index) of the end of the labelled span for computing the token classification loss.
+ Positions are clamped to the length of the sequence (:obj:`sequence_length`). Position outside of the
+ sequence are not taken into account for computing the loss.
+ """
+ return_dict = return_dict if return_dict is not None else self.config.use_return_dict
+
+ outputs = self.deberta(
+ input_ids,
+ attention_mask=attention_mask,
+ token_type_ids=token_type_ids,
+ position_ids=position_ids,
+ inputs_embeds=inputs_embeds,
+ output_attentions=output_attentions,
+ output_hidden_states=output_hidden_states,
+ return_dict=return_dict,
+ )
+
+ sequence_output = outputs[0]
+
+ logits = self.qa_outputs(sequence_output)
+ start_logits, end_logits = logits.split(1, dim=-1)
+ start_logits = start_logits.squeeze(-1).contiguous()
+ end_logits = end_logits.squeeze(-1).contiguous()
+
+ total_loss = None
+ if start_positions is not None and end_positions is not None:
+ # If we are on multi-GPU, split add a dimension
+ if len(start_positions.size()) > 1:
+ start_positions = start_positions.squeeze(-1)
+ if len(end_positions.size()) > 1:
+ end_positions = end_positions.squeeze(-1)
+ # sometimes the start/end positions are outside our model inputs, we ignore these terms
+ ignored_index = start_logits.size(1)
+ start_positions = start_positions.clamp(0, ignored_index)
+ end_positions = end_positions.clamp(0, ignored_index)
+
+ loss_fct = CrossEntropyLoss(ignore_index=ignored_index)
+ start_loss = loss_fct(start_logits, start_positions)
+ end_loss = loss_fct(end_logits, end_positions)
+ total_loss = (start_loss + end_loss) / 2
+
+ if not return_dict:
+ output = (start_logits, end_logits) + outputs[1:]
+ return ((total_loss,) + output) if total_loss is not None else output
+
+ return QuestionAnsweringModelOutput(
+ loss=total_loss,
+ start_logits=start_logits,
+ end_logits=end_logits,
+ hidden_states=outputs.hidden_states,
+ attentions=outputs.attentions,
+ )
\ No newline at end of file
diff --git a/model/head_for_question_answering.py b/model/head_for_question_answering.py
new file mode 100644
index 0000000..bfd78fa
--- /dev/null
+++ b/model/head_for_question_answering.py
@@ -0,0 +1,730 @@
+import torch
+import torch.nn
+from torch.nn import CrossEntropyLoss
+from transformers import BertPreTrainedModel, BertModel, RobertaPreTrainedModel, RobertaModel
+from transformers.modeling_outputs import QuestionAnsweringModelOutput
+
+from model.prefix_encoder import PrefixEncoder
+from model.deberta import DebertaPreTrainedModel, DebertaModel
+from model.model_adaptation import BertAdaModel, RobertaAdaModel
+
+from model.parameter_freeze import ParameterFreeze
+
+freezer = ParameterFreeze()
+
+# ========== BERT ==========
+# Vanilla Fine-tuning for BERT
+class BertForQuestionAnswering(BertPreTrainedModel):
+
+ _keys_to_ignore_on_load_unexpected = [r"pooler"]
+
+ def __init__(self, config):
+ super().__init__(config)
+ self.num_labels = config.num_labels
+
+ self.bert = BertModel(config, add_pooling_layer=False)
+ self.qa_outputs = torch.nn.Linear(config.hidden_size, config.num_labels)
+
+ if self.config.use_pe:
+ self.bert = freezer.freeze_lm(self.bert)
+ # for param in self.bert.parameters():
+ # param.requires_grad = False
+
+ self.init_weights()
+
+ def freeze_backbone(self, use_pe: bool=True):
+ if use_pe:
+ self.bert = freezer.freeze_lm(self.bert)
+ else:
+ self.bert = freezer.unfreeze_lm(self.bert)
+
+ def forward(
+ self,
+ input_ids=None,
+ attention_mask=None,
+ token_type_ids=None,
+ position_ids=None,
+ head_mask=None,
+ inputs_embeds=None,
+ start_positions=None,
+ end_positions=None,
+ output_attentions=None,
+ output_hidden_states=None,
+ return_dict=None,
+ ):
+ r"""
+ start_positions (:obj:`torch.LongTensor` of shape :obj:`(batch_size,)`, `optional`):
+ Labels for position (index) of the start of the labelled span for computing the token classification loss.
+ Positions are clamped to the length of the sequence (:obj:`sequence_length`). Position outside of the
+ sequence are not taken into account for computing the loss.
+ end_positions (:obj:`torch.LongTensor` of shape :obj:`(batch_size,)`, `optional`):
+ Labels for position (index) of the end of the labelled span for computing the token classification loss.
+ Positions are clamped to the length of the sequence (:obj:`sequence_length`). Position outside of the
+ sequence are not taken into account for computing the loss.
+ """
+ return_dict = return_dict if return_dict is not None else self.config.use_return_dict
+
+ outputs = self.bert(
+ input_ids,
+ attention_mask=attention_mask,
+ token_type_ids=token_type_ids,
+ position_ids=position_ids,
+ head_mask=head_mask,
+ inputs_embeds=inputs_embeds,
+ output_attentions=output_attentions,
+ output_hidden_states=output_hidden_states,
+ return_dict=return_dict,
+ )
+
+ sequence_output = outputs[0]
+
+ logits = self.qa_outputs(sequence_output)
+ start_logits, end_logits = logits.split(1, dim=-1)
+ start_logits = start_logits.squeeze(-1).contiguous()
+ end_logits = end_logits.squeeze(-1).contiguous()
+
+ total_loss = None
+ if start_positions is not None and end_positions is not None:
+ # If we are on multi-GPU, split add a dimension
+ if len(start_positions.size()) > 1:
+ start_positions = start_positions.squeeze(-1)
+ if len(end_positions.size()) > 1:
+ end_positions = end_positions.squeeze(-1)
+ # sometimes the start/end positions are outside our model inputs, we ignore these terms
+ ignored_index = start_logits.size(1)
+ start_positions = start_positions.clamp(0, ignored_index)
+ end_positions = end_positions.clamp(0, ignored_index)
+
+ loss_fct = CrossEntropyLoss(ignore_index=ignored_index)
+ start_loss = loss_fct(start_logits, start_positions)
+ end_loss = loss_fct(end_logits, end_positions)
+ total_loss = (start_loss + end_loss) / 2
+
+ if not return_dict:
+ output = (start_logits, end_logits) + outputs[2:]
+ return ((total_loss,) + output) if total_loss is not None else output
+
+ return QuestionAnsweringModelOutput(
+ loss=total_loss,
+ start_logits=start_logits,
+ end_logits=end_logits,
+ hidden_states=outputs.hidden_states,
+ attentions=outputs.attentions,
+ )
+
+# Prefix-tuning for BERT
+class BertPrefixForQuestionAnswering(BertPreTrainedModel):
+ def __init__(self, config):
+ super().__init__(config)
+ self.num_labels = config.num_labels
+
+ self.pre_seq_len = config.pre_seq_len
+ self.n_layer = config.num_hidden_layers
+ self.n_head = config.num_attention_heads
+ self.n_embd = config.hidden_size // config.num_attention_heads
+
+ self.bert = BertModel(config, add_pooling_layer=False)
+ self.qa_outputs = torch.nn.Linear(config.hidden_size, config.num_labels)
+ self.dropout = torch.nn.Dropout(config.hidden_dropout_prob)
+ self.prefix_encoder = PrefixEncoder(config)
+ self.prefix_tokens = torch.arange(self.pre_seq_len).long()
+
+ # for param in self.bert.parameters():
+ # param.requires_grad = False
+
+ if self.config.use_pe:
+ self.bert = freezer.freeze_lm(self.bert)
+
+ self.init_weights()
+
+ def freeze_backbone(self, use_pe: bool=True):
+ if use_pe:
+ self.bert = freezer.freeze_lm(self.bert)
+ else:
+ self.bert = freezer.unfreeze_lm(self.bert)
+
+ def get_prompt(self, batch_size):
+ prefix_tokens = self.prefix_tokens.unsqueeze(0).expand(batch_size, -1).to(self.bert.device)
+ past_key_values = self.prefix_encoder(prefix_tokens)
+ bsz, seqlen, _ = past_key_values.shape
+ past_key_values = past_key_values.view(
+ bsz,
+ seqlen,
+ self.n_layer * 2,
+ self.n_head,
+ self.n_embd
+ )
+ past_key_values = self.dropout(past_key_values)
+ past_key_values = past_key_values.permute([2, 0, 3, 1, 4]).split(2)
+ return past_key_values
+
+ def forward(
+ self,
+ input_ids=None,
+ attention_mask=None,
+ token_type_ids=None,
+ position_ids=None,
+ head_mask=None,
+ inputs_embeds=None,
+ start_positions=None,
+ end_positions=None,
+ output_attentions=None,
+ output_hidden_states=None,
+ return_dict=None,
+ ):
+ r"""
+ start_positions (:obj:`torch.LongTensor` of shape :obj:`(batch_size,)`, `optional`):
+ Labels for position (index) of the start of the labelled span for computing the token classification loss.
+ Positions are clamped to the length of the sequence (:obj:`sequence_length`). Position outside of the
+ sequence are not taken into account for computing the loss.
+ end_positions (:obj:`torch.LongTensor` of shape :obj:`(batch_size,)`, `optional`):
+ Labels for position (index) of the end of the labelled span for computing the token classification loss.
+ Positions are clamped to the length of the sequence (:obj:`sequence_length`). Position outside of the
+ sequence are not taken into account for computing the loss.
+ """
+ return_dict = return_dict if return_dict is not None else self.config.use_return_dict
+
+ batch_size = input_ids.shape[0]
+ past_key_values = self.get_prompt(batch_size=batch_size)
+ prefix_attention_mask = torch.ones(batch_size, self.pre_seq_len).to(self.bert.device)
+ attention_mask = torch.cat((prefix_attention_mask, attention_mask), dim=1)
+
+ outputs = self.bert(
+ input_ids,
+ attention_mask=attention_mask,
+ token_type_ids=token_type_ids,
+ position_ids=position_ids,
+ head_mask=head_mask,
+ inputs_embeds=inputs_embeds,
+ output_attentions=output_attentions,
+ output_hidden_states=output_hidden_states,
+ return_dict=return_dict,
+ past_key_values=past_key_values,
+ )
+
+ sequence_output = outputs[0]
+
+ logits = self.qa_outputs(sequence_output)
+ start_logits, end_logits = logits.split(1, dim=-1)
+ start_logits = start_logits.squeeze(-1).contiguous()
+ end_logits = end_logits.squeeze(-1).contiguous()
+
+ total_loss = None
+ if start_positions is not None and end_positions is not None:
+ # If we are on multi-GPU, split add a dimension
+ if len(start_positions.size()) > 1:
+ start_positions = start_positions.squeeze(-1)
+ if len(end_positions.size()) > 1:
+ end_positions = end_positions.squeeze(-1)
+ # sometimes the start/end positions are outside our model inputs, we ignore these terms
+ ignored_index = start_logits.size(1)
+ start_positions = start_positions.clamp(0, ignored_index)
+ end_positions = end_positions.clamp(0, ignored_index)
+
+ loss_fct = CrossEntropyLoss(ignore_index=ignored_index)
+ start_loss = loss_fct(start_logits, start_positions)
+ end_loss = loss_fct(end_logits, end_positions)
+ total_loss = (start_loss + end_loss) / 2
+
+ if not return_dict:
+ output = (start_logits, end_logits) + outputs[2:]
+ return ((total_loss,) + output) if total_loss is not None else output
+
+ return QuestionAnsweringModelOutput(
+ loss=total_loss,
+ start_logits=start_logits,
+ end_logits=end_logits,
+ hidden_states=outputs.hidden_states,
+ attentions=outputs.attentions,
+ )
+
+# Adapter-tuning for BERT
+class BertAdapterForQuestionAnswering(BertPreTrainedModel):
+ def __init__(self, config):
+ super().__init__(config)
+ self.num_labels = config.num_labels
+
+ self.pre_seq_len = config.pre_seq_len
+ self.n_layer = config.num_hidden_layers
+ self.n_head = config.num_attention_heads
+ self.n_embd = config.hidden_size // config.num_attention_heads
+
+ self.bert = BertAdaModel(config, add_pooling_layer=False)
+ self.embeddings = self.bert.embeddings
+ self.qa_outputs = torch.nn.Linear(config.hidden_size, config.num_labels)
+ self.dropout = torch.nn.Dropout(config.hidden_dropout_prob)
+
+ # for param in self.bert.parameters():
+ # param.requires_grad = False
+ if self.config.use_pe:
+ self.bert = freezer.freeze_lm_component(self.bert, "adapter")
+
+ self.init_weights()
+
+ def freeze_backbone(self, use_pe: bool=True):
+ if use_pe:
+ self.bert = freezer.freeze_lm_component(self.bert, "adapter")
+ else:
+ self.bert = freezer.unfreeze_lm(self.bert)
+
+ def forward(
+ self,
+ input_ids=None,
+ attention_mask=None,
+ token_type_ids=None,
+ position_ids=None,
+ head_mask=None,
+ inputs_embeds=None,
+ start_positions=None,
+ end_positions=None,
+ output_attentions=None,
+ output_hidden_states=None,
+ return_dict=None,
+ ):
+ r"""
+ start_positions (:obj:`torch.LongTensor` of shape :obj:`(batch_size,)`, `optional`):
+ Labels for position (index) of the start of the labelled span for computing the token classification loss.
+ Positions are clamped to the length of the sequence (:obj:`sequence_length`). Position outside of the
+ sequence are not taken into account for computing the loss.
+ end_positions (:obj:`torch.LongTensor` of shape :obj:`(batch_size,)`, `optional`):
+ Labels for position (index) of the end of the labelled span for computing the token classification loss.
+ Positions are clamped to the length of the sequence (:obj:`sequence_length`). Position outside of the
+ sequence are not taken into account for computing the loss.
+ """
+ return_dict = return_dict if return_dict is not None else self.config.use_return_dict
+
+ batch_size = input_ids.shape[0]
+ inputs_embeds = self.embeddings(
+ input_ids=input_ids,
+ position_ids=position_ids,
+ token_type_ids=token_type_ids,
+ )
+ # past_key_values = self.get_prompt(batch_size=batch_size)
+ # prefix_attention_mask = torch.ones(batch_size, self.pre_seq_len).to(self.bert.device)
+ # attention_mask = torch.cat((prefix_attention_mask, attention_mask), dim=1)
+
+ outputs = self.bert(
+ # input_ids,
+ attention_mask=attention_mask,
+ # token_type_ids=token_type_ids,
+ # position_ids=position_ids,
+ head_mask=head_mask,
+ inputs_embeds=inputs_embeds,
+ output_attentions=output_attentions,
+ output_hidden_states=output_hidden_states,
+ return_dict=return_dict,
+ # past_key_values=past_key_values,
+ )
+
+ sequence_output = outputs[0]
+
+ logits = self.qa_outputs(sequence_output)
+ start_logits, end_logits = logits.split(1, dim=-1)
+ start_logits = start_logits.squeeze(-1).contiguous()
+ end_logits = end_logits.squeeze(-1).contiguous()
+
+ total_loss = None
+ if start_positions is not None and end_positions is not None:
+ # If we are on multi-GPU, split add a dimension
+ if len(start_positions.size()) > 1:
+ start_positions = start_positions.squeeze(-1)
+ if len(end_positions.size()) > 1:
+ end_positions = end_positions.squeeze(-1)
+ # sometimes the start/end positions are outside our model inputs, we ignore these terms
+ ignored_index = start_logits.size(1)
+ start_positions = start_positions.clamp(0, ignored_index)
+ end_positions = end_positions.clamp(0, ignored_index)
+
+ loss_fct = CrossEntropyLoss(ignore_index=ignored_index)
+ start_loss = loss_fct(start_logits, start_positions)
+ end_loss = loss_fct(end_logits, end_positions)
+ total_loss = (start_loss + end_loss) / 2
+
+ if not return_dict:
+ output = (start_logits, end_logits) + outputs[2:]
+ return ((total_loss,) + output) if total_loss is not None else output
+
+ return QuestionAnsweringModelOutput(
+ loss=total_loss,
+ start_logits=start_logits,
+ end_logits=end_logits,
+ hidden_states=outputs.hidden_states,
+ attentions=outputs.attentions,
+ )
+
+# ========== RoBERTa ==========
+
+# Prefix-tuning for RoBERTa
+class RobertaPrefixModelForQuestionAnswering(RobertaPreTrainedModel):
+ def __init__(self, config):
+ super().__init__(config)
+ self.num_labels = config.num_labels
+
+ self.pre_seq_len = config.pre_seq_len
+ self.n_layer = config.num_hidden_layers
+ self.n_head = config.num_attention_heads
+ self.n_embd = config.hidden_size // config.num_attention_heads
+
+ self.roberta = RobertaModel(config, add_pooling_layer=False)
+ self.qa_outputs = torch.nn.Linear(config.hidden_size, config.num_labels)
+
+ self.init_weights()
+ self.dropout = torch.nn.Dropout(config.hidden_dropout_prob)
+ self.prefix_encoder = PrefixEncoder(config)
+ self.prefix_tokens = torch.arange(self.pre_seq_len).long()
+
+ # for param in self.roberta.parameters():
+ # param.requires_grad = False
+ if self.config.use_pe:
+ self.roberta = freezer.freeze_lm(self.roberta)
+
+ def freeze_backbone(self, use_pe: bool=True):
+ if use_pe:
+ self.roberta = freezer.freeze_lm(self.roberta)
+ else:
+ self.roberta = freezer.unfreeze_lm(self.roberta)
+
+ def get_prompt(self, batch_size):
+ prefix_tokens = self.prefix_tokens.unsqueeze(0).expand(batch_size, -1).to(self.roberta.device)
+ past_key_values = self.prefix_encoder(prefix_tokens)
+ bsz, seqlen, _ = past_key_values.shape
+ past_key_values = past_key_values.view(
+ bsz,
+ seqlen,
+ self.n_layer * 2,
+ self.n_head,
+ self.n_embd
+ )
+ past_key_values = self.dropout(past_key_values)
+ past_key_values = past_key_values.permute([2, 0, 3, 1, 4]).split(2)
+ return past_key_values
+
+ def forward(
+ self,
+ input_ids=None,
+ attention_mask=None,
+ token_type_ids=None,
+ position_ids=None,
+ head_mask=None,
+ inputs_embeds=None,
+ start_positions=None,
+ end_positions=None,
+ output_attentions=None,
+ output_hidden_states=None,
+ return_dict=None,
+ ):
+ r"""
+ start_positions (:obj:`torch.LongTensor` of shape :obj:`(batch_size,)`, `optional`):
+ Labels for position (index) of the start of the labelled span for computing the token classification loss.
+ Positions are clamped to the length of the sequence (:obj:`sequence_length`). Position outside of the
+ sequence are not taken into account for computing the loss.
+ end_positions (:obj:`torch.LongTensor` of shape :obj:`(batch_size,)`, `optional`):
+ Labels for position (index) of the end of the labelled span for computing the token classification loss.
+ Positions are clamped to the length of the sequence (:obj:`sequence_length`). Position outside of the
+ sequence are not taken into account for computing the loss.
+ """
+ return_dict = return_dict if return_dict is not None else self.config.use_return_dict
+
+ batch_size = input_ids.shape[0]
+ past_key_values = self.get_prompt(batch_size=batch_size)
+ prefix_attention_mask = torch.ones(batch_size, self.pre_seq_len).to(self.roberta.device)
+ attention_mask = torch.cat((prefix_attention_mask, attention_mask), dim=1)
+
+ outputs = self.roberta(
+ input_ids,
+ attention_mask=attention_mask,
+ token_type_ids=token_type_ids,
+ position_ids=position_ids,
+ head_mask=head_mask,
+ inputs_embeds=inputs_embeds,
+ output_attentions=output_attentions,
+ output_hidden_states=output_hidden_states,
+ return_dict=return_dict,
+ past_key_values=past_key_values,
+ )
+
+ sequence_output = outputs[0]
+
+ logits = self.qa_outputs(sequence_output)
+ start_logits, end_logits = logits.split(1, dim=-1)
+ start_logits = start_logits.squeeze(-1).contiguous()
+ end_logits = end_logits.squeeze(-1).contiguous()
+
+ total_loss = None
+ if start_positions is not None and end_positions is not None:
+ # If we are on multi-GPU, split add a dimension
+ if len(start_positions.size()) > 1:
+ start_positions = start_positions.squeeze(-1)
+ if len(end_positions.size()) > 1:
+ end_positions = end_positions.squeeze(-1)
+ # sometimes the start/end positions are outside our model inputs, we ignore these terms
+ ignored_index = start_logits.size(1)
+ start_positions = start_positions.clamp(0, ignored_index)
+ end_positions = end_positions.clamp(0, ignored_index)
+
+ loss_fct = CrossEntropyLoss(ignore_index=ignored_index)
+ start_loss = loss_fct(start_logits, start_positions)
+ end_loss = loss_fct(end_logits, end_positions)
+ total_loss = (start_loss + end_loss) / 2
+
+ if not return_dict:
+ output = (start_logits, end_logits) + outputs[2:]
+ return ((total_loss,) + output) if total_loss is not None else output
+
+ return QuestionAnsweringModelOutput(
+ loss=total_loss,
+ start_logits=start_logits,
+ end_logits=end_logits,
+ hidden_states=outputs.hidden_states,
+ attentions=outputs.attentions,
+ )
+
+# Adapter-tuning for RoBERTa
+class RobertaAdapterForQuestionAnswering(RobertaPreTrainedModel):
+ def __init__(self, config):
+ super().__init__(config)
+ self.num_labels = config.num_labels
+
+ self.pre_seq_len = config.pre_seq_len
+ self.n_layer = config.num_hidden_layers
+ self.n_head = config.num_attention_heads
+ self.n_embd = config.hidden_size // config.num_attention_heads
+
+ self.roberta = RobertaAdaModel(config, add_pooling_layer=False)
+ self.embeddings = self.roberta.embeddings
+ self.qa_outputs = torch.nn.Linear(config.hidden_size, config.num_labels)
+ self.dropout = torch.nn.Dropout(config.hidden_dropout_prob)
+
+ # for param in self.bert.parameters():
+ # param.requires_grad = False
+ if self.config.use_pe:
+ self.roberta = freezer.freeze_lm_component(self.roberta, "adapter")
+
+ self.init_weights()
+
+ def freeze_backbone(self, use_pe: bool=True):
+ if use_pe:
+ self.roberta = freezer.freeze_lm_component(self.roberta, "adapter")
+ else:
+ self.roberta = freezer.unfreeze_lm(self.roberta)
+
+ def forward(
+ self,
+ input_ids=None,
+ attention_mask=None,
+ token_type_ids=None,
+ position_ids=None,
+ head_mask=None,
+ inputs_embeds=None,
+ start_positions=None,
+ end_positions=None,
+ output_attentions=None,
+ output_hidden_states=None,
+ return_dict=None,
+ ):
+ r"""
+ start_positions (:obj:`torch.LongTensor` of shape :obj:`(batch_size,)`, `optional`):
+ Labels for position (index) of the start of the labelled span for computing the token classification loss.
+ Positions are clamped to the length of the sequence (:obj:`sequence_length`). Position outside of the
+ sequence are not taken into account for computing the loss.
+ end_positions (:obj:`torch.LongTensor` of shape :obj:`(batch_size,)`, `optional`):
+ Labels for position (index) of the end of the labelled span for computing the token classification loss.
+ Positions are clamped to the length of the sequence (:obj:`sequence_length`). Position outside of the
+ sequence are not taken into account for computing the loss.
+ """
+ return_dict = return_dict if return_dict is not None else self.config.use_return_dict
+
+ batch_size = input_ids.shape[0]
+ inputs_embeds = self.embeddings(
+ input_ids=input_ids,
+ position_ids=position_ids,
+ token_type_ids=token_type_ids,
+ )
+ # past_key_values = self.get_prompt(batch_size=batch_size)
+ # prefix_attention_mask = torch.ones(batch_size, self.pre_seq_len).to(self.bert.device)
+ # attention_mask = torch.cat((prefix_attention_mask, attention_mask), dim=1)
+
+ outputs = self.roberta(
+ # input_ids,
+ attention_mask=attention_mask,
+ # token_type_ids=token_type_ids,
+ # position_ids=position_ids,
+ head_mask=head_mask,
+ inputs_embeds=inputs_embeds,
+ output_attentions=output_attentions,
+ output_hidden_states=output_hidden_states,
+ return_dict=return_dict,
+ # past_key_values=past_key_values,
+ )
+
+ sequence_output = outputs[0]
+
+ logits = self.qa_outputs(sequence_output)
+ start_logits, end_logits = logits.split(1, dim=-1)
+ start_logits = start_logits.squeeze(-1).contiguous()
+ end_logits = end_logits.squeeze(-1).contiguous()
+
+ total_loss = None
+ if start_positions is not None and end_positions is not None:
+ # If we are on multi-GPU, split add a dimension
+ if len(start_positions.size()) > 1:
+ start_positions = start_positions.squeeze(-1)
+ if len(end_positions.size()) > 1:
+ end_positions = end_positions.squeeze(-1)
+ # sometimes the start/end positions are outside our model inputs, we ignore these terms
+ ignored_index = start_logits.size(1)
+ start_positions = start_positions.clamp(0, ignored_index)
+ end_positions = end_positions.clamp(0, ignored_index)
+
+ loss_fct = CrossEntropyLoss(ignore_index=ignored_index)
+ start_loss = loss_fct(start_logits, start_positions)
+ end_loss = loss_fct(end_logits, end_positions)
+ total_loss = (start_loss + end_loss) / 2
+
+ if not return_dict:
+ output = (start_logits, end_logits) + outputs[2:]
+ return ((total_loss,) + output) if total_loss is not None else output
+
+ return QuestionAnsweringModelOutput(
+ loss=total_loss,
+ start_logits=start_logits,
+ end_logits=end_logits,
+ hidden_states=outputs.hidden_states,
+ attentions=outputs.attentions,
+ )
+
+
+# ========== DeBERTa ==========
+
+# Prefix-tuning for DeBERTa
+class DebertaPrefixModelForQuestionAnswering(DebertaPreTrainedModel):
+ def __init__(self, config):
+ super().__init__(config)
+ self.num_labels = config.num_labels
+ self.deberta = DebertaModel(config)
+ self.dropout = torch.nn.Dropout(config.hidden_dropout_prob)
+ self.qa_outputs = torch.nn.Linear(config.hidden_size, config.num_labels)
+ self.init_weights()
+
+ # for param in self.deberta.parameters():
+ # param.requires_grad = False
+ if self.config.use_pe:
+ self.deberta = freezer.freeze_lm(self.deberta)
+
+ self.pre_seq_len = config.pre_seq_len
+ self.n_layer = config.num_hidden_layers
+ self.n_head = config.num_attention_heads
+ self.n_embd = config.hidden_size // config.num_attention_heads
+
+ # Use a two layered MLP to encode the prefix
+ self.prefix_tokens = torch.arange(self.pre_seq_len).long()
+ self.prefix_encoder = PrefixEncoder(config)
+
+ deberta_param = 0
+ for name, param in self.deberta.named_parameters():
+ deberta_param += param.numel()
+ all_param = 0
+ for name, param in self.named_parameters():
+ all_param += param.numel()
+ total_param = all_param - deberta_param
+ print('total param is {}'.format(total_param)) # 9860105
+
+ def freeze_backbone(self, use_pe: bool=True):
+ if use_pe:
+ self.deberta = freezer.freeze_lm(self.deberta)
+ else:
+ self.deberta = freezer.unfreeze_lm(self.deberta)
+
+ def get_prompt(self, batch_size):
+ prefix_tokens = self.prefix_tokens.unsqueeze(0).expand(batch_size, -1).to(self.deberta.device)
+ past_key_values = self.prefix_encoder(prefix_tokens)
+ # bsz, seqlen, _ = past_key_values.shape
+ past_key_values = past_key_values.view(
+ batch_size,
+ self.pre_seq_len,
+ self.n_layer * 2,
+ self.n_head,
+ self.n_embd
+ )
+ past_key_values = self.dropout(past_key_values)
+ past_key_values = past_key_values.permute([2, 0, 3, 1, 4]).split(2)
+ return past_key_values
+
+ def forward(
+ self,
+ input_ids=None,
+ attention_mask=None,
+ token_type_ids=None,
+ position_ids=None,
+ # head_mask=None,
+ inputs_embeds=None,
+ start_positions=None,
+ end_positions=None,
+ output_attentions=None,
+ output_hidden_states=None,
+ return_dict=None,
+ ):
+ r"""
+ start_positions (:obj:`torch.LongTensor` of shape :obj:`(batch_size,)`, `optional`):
+ Labels for position (index) of the start of the labelled span for computing the token classification loss.
+ Positions are clamped to the length of the sequence (:obj:`sequence_length`). Position outside of the
+ sequence are not taken into account for computing the loss.
+ end_positions (:obj:`torch.LongTensor` of shape :obj:`(batch_size,)`, `optional`):
+ Labels for position (index) of the end of the labelled span for computing the token classification loss.
+ Positions are clamped to the length of the sequence (:obj:`sequence_length`). Position outside of the
+ sequence are not taken into account for computing the loss.
+ """
+ return_dict = return_dict if return_dict is not None else self.config.use_return_dict
+
+ batch_size = input_ids.shape[0]
+ past_key_values = self.get_prompt(batch_size=batch_size)
+ prefix_attention_mask = torch.ones(batch_size, self.pre_seq_len).to(self.deberta.device)
+ attention_mask = torch.cat((prefix_attention_mask, attention_mask), dim=1)
+
+ outputs = self.deberta(
+ input_ids,
+ attention_mask=attention_mask,
+ token_type_ids=token_type_ids,
+ position_ids=position_ids,
+ inputs_embeds=inputs_embeds,
+ output_attentions=output_attentions,
+ output_hidden_states=output_hidden_states,
+ return_dict=return_dict,
+ past_key_values=past_key_values,
+ )
+
+ sequence_output = outputs[0]
+
+ logits = self.qa_outputs(sequence_output)
+ start_logits, end_logits = logits.split(1, dim=-1)
+ start_logits = start_logits.squeeze(-1).contiguous()
+ end_logits = end_logits.squeeze(-1).contiguous()
+
+ total_loss = None
+ if start_positions is not None and end_positions is not None:
+ # If we are on multi-GPU, split add a dimension
+ if len(start_positions.size()) > 1:
+ start_positions = start_positions.squeeze(-1)
+ if len(end_positions.size()) > 1:
+ end_positions = end_positions.squeeze(-1)
+ # sometimes the start/end positions are outside our model inputs, we ignore these terms
+ ignored_index = start_logits.size(1)
+ start_positions = start_positions.clamp(0, ignored_index)
+ end_positions = end_positions.clamp(0, ignored_index)
+
+ loss_fct = CrossEntropyLoss(ignore_index=ignored_index)
+ start_loss = loss_fct(start_logits, start_positions)
+ end_loss = loss_fct(end_logits, end_positions)
+ total_loss = (start_loss + end_loss) / 2
+
+ if not return_dict:
+ output = (start_logits, end_logits) + outputs[2:]
+ return ((total_loss,) + output) if total_loss is not None else output
+
+ return QuestionAnsweringModelOutput(
+ loss=total_loss,
+ start_logits=start_logits,
+ end_logits=end_logits,
+ hidden_states=outputs.hidden_states,
+ attentions=outputs.attentions,
+ )
\ No newline at end of file
diff --git a/model/head_for_sequence_classification.py b/model/head_for_sequence_classification.py
new file mode 100644
index 0000000..44f2106
--- /dev/null
+++ b/model/head_for_sequence_classification.py
@@ -0,0 +1,1038 @@
+'''
+Head Tuning with Prefix / Adapter
+'''
+import torch
+from torch._C import NoopLogger
+import torch.nn
+import torch.nn.functional as F
+from torch import Tensor
+from torch.nn import CrossEntropyLoss, MSELoss, BCEWithLogitsLoss
+
+from transformers import BertModel, BertPreTrainedModel
+from transformers import RobertaModel, RobertaPreTrainedModel
+from transformers.modeling_outputs import SequenceClassifierOutput, BaseModelOutput, Seq2SeqLMOutput
+
+from model.prefix_encoder import PrefixEncoder
+from model.deberta import DebertaModel, DebertaPreTrainedModel, ContextPooler, StableDropout
+
+from model.model_adaptation import BertAdaModel, RobertaAdaModel, init_adapter
+from model.parameter_freeze import ParameterFreeze # add by wjn
+import copy
+
+freezer = ParameterFreeze()
+
+## ======== BERT ========
+
+# Vanilla Fine-tuning For BERT
+class BertForSequenceClassification(BertPreTrainedModel):
+ def __init__(self, config):
+ super().__init__(config)
+ self.num_labels = config.num_labels
+ self.config = config
+
+ self.bert = BertModel(config)
+ if self.config.use_pe:
+ self.bert = freezer.freeze_lm(self.bert)
+
+ self.dropout = torch.nn.Dropout(config.hidden_dropout_prob)
+ self.classifier = torch.nn.Linear(config.hidden_size, config.num_labels)
+
+ self.init_weights()
+
+ def freeze_backbone(self, use_pe: bool=True):
+ if use_pe:
+ self.bert = freezer.freeze_lm(self.bert)
+ else:
+ self.bert = freezer.unfreeze_lm(self.bert)
+
+ def forward(
+ self,
+ input_ids=None,
+ attention_mask=None,
+ token_type_ids=None,
+ position_ids=None,
+ head_mask=None,
+ inputs_embeds=None,
+ labels=None,
+ output_attentions=None,
+ output_hidden_states=None,
+ return_dict=None,
+ ):
+ r"""
+ labels (:obj:`torch.LongTensor` of shape :obj:`(batch_size,)`, `optional`):
+ Labels for computing the sequence classification/regression loss. Indices should be in :obj:`[0, ...,
+ config.num_labels - 1]`. If :obj:`config.num_labels == 1` a regression loss is computed (Mean-Square loss),
+ If :obj:`config.num_labels > 1` a classification loss is computed (Cross-Entropy).
+ """
+ return_dict = return_dict if return_dict is not None else self.config.use_return_dict
+
+ outputs = self.bert(
+ input_ids,
+ attention_mask=attention_mask,
+ token_type_ids=token_type_ids,
+ position_ids=position_ids,
+ head_mask=head_mask,
+ inputs_embeds=inputs_embeds,
+ output_attentions=output_attentions,
+ output_hidden_states=output_hidden_states,
+ return_dict=return_dict,
+ )
+
+ pooled_output = outputs[1]
+
+ pooled_output = self.dropout(pooled_output)
+ logits = self.classifier(pooled_output)
+
+ loss = None
+ if labels is not None:
+ if self.config.problem_type is None:
+ if self.num_labels == 1:
+ self.config.problem_type = "regression"
+ elif self.num_labels > 1 and (labels.dtype == torch.long or labels.dtype == torch.int):
+ self.config.problem_type = "single_label_classification"
+ else:
+ self.config.problem_type = "multi_label_classification"
+
+ if self.config.problem_type == "regression":
+ loss_fct = MSELoss()
+ if self.num_labels == 1:
+ loss = loss_fct(logits.squeeze(), labels.squeeze())
+ else:
+ loss = loss_fct(logits, labels)
+ elif self.config.problem_type == "single_label_classification":
+ loss_fct = CrossEntropyLoss()
+ loss = loss_fct(logits.view(-1, self.num_labels), labels.view(-1))
+ elif self.config.problem_type == "multi_label_classification":
+ loss_fct = BCEWithLogitsLoss()
+ loss = loss_fct(logits, labels)
+ if not return_dict:
+ output = (logits,) + outputs[2:]
+ return ((loss,) + output) if loss is not None else output
+
+ return SequenceClassifierOutput(
+ loss=loss,
+ logits=logits,
+ hidden_states=outputs.hidden_states,
+ attentions=outputs.attentions,
+ )
+
+# Prefix-tuning For BERT
+class BertPrefixForSequenceClassification(BertPreTrainedModel):
+ def __init__(self, config):
+ super().__init__(config)
+ self.num_labels = config.num_labels
+ self.config = config
+ self.bert = BertModel(config)
+ self.dropout = torch.nn.Dropout(config.hidden_dropout_prob)
+ self.classifier = torch.nn.Linear(config.hidden_size, config.num_labels)
+
+ # for param in self.bert.parameters():
+ # param.requires_grad = False
+
+ if self.config.use_pe:
+ self.bert = freezer.freeze_lm(self.bert)
+
+ self.pre_seq_len = config.pre_seq_len
+ self.n_layer = config.num_hidden_layers
+ self.n_head = config.num_attention_heads
+ self.n_embd = config.hidden_size // config.num_attention_heads
+
+ self.prefix_tokens = torch.arange(self.pre_seq_len).long()
+
+ self.prefix_encoder = PrefixEncoder(config)
+
+ bert_param = 0
+ for name, param in self.bert.named_parameters():
+ bert_param += param.numel()
+ all_param = 0
+ for name, param in self.named_parameters():
+ all_param += param.numel()
+ total_param = all_param - bert_param
+ print('total param is {}'.format(total_param)) # 9860105
+
+ def freeze_backbone(self, use_pe: bool=True):
+ if use_pe:
+ self.bert = freezer.freeze_lm(self.bert)
+ else:
+ self.bert = freezer.unfreeze_lm(self.bert)
+
+ def get_prompt(self, batch_size):
+ prefix_tokens = self.prefix_tokens.unsqueeze(0).expand(batch_size, -1).to(self.bert.device)
+ past_key_values = self.prefix_encoder(prefix_tokens)
+ # bsz, seqlen, _ = past_key_values.shape
+ past_key_values = past_key_values.view(
+ batch_size,
+ self.pre_seq_len,
+ self.n_layer * 2,
+ self.n_head,
+ self.n_embd
+ )
+ past_key_values = self.dropout(past_key_values)
+ past_key_values = past_key_values.permute([2, 0, 3, 1, 4]).split(2)
+ return past_key_values
+
+ def forward(
+ self,
+ input_ids=None,
+ attention_mask=None,
+ token_type_ids=None,
+ position_ids=None,
+ head_mask=None,
+ inputs_embeds=None,
+ labels=None,
+ output_attentions=None,
+ output_hidden_states=None,
+ return_dict=None,
+ ):
+ return_dict = return_dict if return_dict is not None else self.config.use_return_dict
+
+ batch_size = input_ids.shape[0]
+ past_key_values = self.get_prompt(batch_size=batch_size)
+ prefix_attention_mask = torch.ones(batch_size, self.pre_seq_len).to(self.bert.device)
+ attention_mask = torch.cat((prefix_attention_mask, attention_mask), dim=1)
+
+ outputs = self.bert(
+ input_ids,
+ attention_mask=attention_mask,
+ token_type_ids=token_type_ids,
+ position_ids=position_ids,
+ head_mask=head_mask,
+ inputs_embeds=inputs_embeds,
+ output_attentions=output_attentions,
+ output_hidden_states=output_hidden_states,
+ return_dict=return_dict,
+ past_key_values=past_key_values,
+ )
+
+ pooled_output = outputs[1]
+
+ pooled_output = self.dropout(pooled_output)
+ logits = self.classifier(pooled_output)
+
+ loss = None
+ if labels is not None:
+ if self.config.problem_type is None:
+ if self.num_labels == 1:
+ self.config.problem_type = "regression"
+ elif self.num_labels > 1 and (labels.dtype == torch.long or labels.dtype == torch.int):
+ self.config.problem_type = "single_label_classification"
+ else:
+ self.config.problem_type = "multi_label_classification"
+
+ if self.config.problem_type == "regression":
+ loss_fct = MSELoss()
+ if self.num_labels == 1:
+ loss = loss_fct(logits.squeeze(), labels.squeeze())
+ else:
+ loss = loss_fct(logits, labels)
+ elif self.config.problem_type == "single_label_classification":
+ loss_fct = CrossEntropyLoss()
+ loss = loss_fct(logits.view(-1, self.num_labels), labels.view(-1))
+ elif self.config.problem_type == "multi_label_classification":
+ loss_fct = BCEWithLogitsLoss()
+ loss = loss_fct(logits, labels)
+ if not return_dict:
+ output = (logits,) + outputs[2:]
+ return ((loss,) + output) if loss is not None else output
+
+ return SequenceClassifierOutput(
+ loss=loss,
+ logits=logits,
+ hidden_states=outputs.hidden_states,
+ attentions=outputs.attentions,
+ )
+
+# Prompt-tuning For BERT
+class BertPtuningForSequenceClassification(BertPreTrainedModel):
+ def __init__(self, config):
+ super().__init__(config)
+ self.num_labels = config.num_labels
+ self.bert = BertModel(config)
+ self.embeddings = self.bert.embeddings
+ self.dropout = torch.nn.Dropout(config.hidden_dropout_prob)
+ self.classifier = torch.nn.Linear(config.hidden_size, config.num_labels)
+
+ # for param in self.bert.parameters():
+ # param.requires_grad = False
+
+ if self.config.use_pe:
+ self.bert = freezer.freeze_lm(self.bert)
+
+ self.pre_seq_len = config.pre_seq_len
+ self.n_layer = config.num_hidden_layers
+ self.n_head = config.num_attention_heads
+ self.n_embd = config.hidden_size // config.num_attention_heads
+
+ self.prefix_tokens = torch.arange(self.pre_seq_len).long()
+ self.prefix_encoder = torch.nn.Embedding(self.pre_seq_len, config.hidden_size)
+
+ def freeze_backbone(self, use_pe: bool=True):
+ if use_pe:
+ self.bert = freezer.freeze_lm(self.bert)
+ else:
+ self.bert = freezer.unfreeze_lm(self.bert)
+
+ def get_prompt(self, batch_size):
+ prefix_tokens = self.prefix_tokens.unsqueeze(0).expand(batch_size, -1).to(self.bert.device)
+ prompts = self.prefix_encoder(prefix_tokens)
+ return prompts
+
+ def forward(
+ self,
+ input_ids=None,
+ attention_mask=None,
+ token_type_ids=None,
+ position_ids=None,
+ head_mask=None,
+ inputs_embeds=None,
+ labels=None,
+ output_attentions=None,
+ output_hidden_states=None,
+ return_dict=None,
+ ):
+ return_dict = return_dict if return_dict is not None else self.config.use_return_dict
+
+ batch_size = input_ids.shape[0]
+ raw_embedding = self.embeddings(
+ input_ids=input_ids,
+ position_ids=position_ids,
+ token_type_ids=token_type_ids,
+ )
+ prompts = self.get_prompt(batch_size=batch_size)
+ inputs_embeds = torch.cat((prompts, raw_embedding), dim=1)
+ prefix_attention_mask = torch.ones(batch_size, self.pre_seq_len).to(self.bert.device)
+ attention_mask = torch.cat((prefix_attention_mask, attention_mask), dim=1)
+
+ outputs = self.bert(
+ # input_ids,
+ attention_mask=attention_mask,
+ # token_type_ids=token_type_ids,
+ # position_ids=position_ids,
+ head_mask=head_mask,
+ inputs_embeds=inputs_embeds,
+ output_attentions=output_attentions,
+ output_hidden_states=output_hidden_states,
+ return_dict=return_dict,
+ # past_key_values=past_key_values,
+ )
+
+ # pooled_output = outputs[1]
+ sequence_output = outputs[0]
+ sequence_output = sequence_output[:, self.pre_seq_len:, :].contiguous()
+ first_token_tensor = sequence_output[:, 0]
+ pooled_output = self.bert.pooler.dense(first_token_tensor)
+ pooled_output = self.bert.pooler.activation(pooled_output)
+
+ pooled_output = self.dropout(pooled_output)
+ logits = self.classifier(pooled_output)
+
+ loss = None
+ if labels is not None:
+ if self.config.problem_type is None:
+ if self.num_labels == 1:
+ self.config.problem_type = "regression"
+ elif self.num_labels > 1 and (labels.dtype == torch.long or labels.dtype == torch.int):
+ self.config.problem_type = "single_label_classification"
+ else:
+ self.config.problem_type = "multi_label_classification"
+
+ if self.config.problem_type == "regression":
+ loss_fct = MSELoss()
+ if self.num_labels == 1:
+ loss = loss_fct(logits.squeeze(), labels.squeeze())
+ else:
+ loss = loss_fct(logits, labels)
+ elif self.config.problem_type == "single_label_classification":
+ loss_fct = CrossEntropyLoss()
+ loss = loss_fct(logits.view(-1, self.num_labels), labels.view(-1))
+ elif self.config.problem_type == "multi_label_classification":
+ loss_fct = BCEWithLogitsLoss()
+ loss = loss_fct(logits, labels)
+ if not return_dict:
+ output = (logits,) + outputs[2:]
+ return ((loss,) + output) if loss is not None else output
+
+ return SequenceClassifierOutput(
+ loss=loss,
+ logits=logits,
+ hidden_states=outputs.hidden_states,
+ attentions=outputs.attentions,
+ )
+
+# Adapter-tuning For BERT
+class BertAdapterForSequenceClassification(BertPreTrainedModel):
+ def __init__(self, config):
+ super().__init__(config)
+ self.num_labels = config.num_labels
+ self.bert = BertAdaModel(config)
+ self.embeddings = self.bert.embeddings
+ self.dropout = torch.nn.Dropout(config.hidden_dropout_prob)
+ self.classifier = torch.nn.Linear(config.hidden_size, config.num_labels)
+
+ # for param in self.bert.parameters():
+ # param.requires_grad = False
+ if self.config.use_pe:
+ self.bert = freezer.freeze_lm_component(self.bert, "adapter")
+
+ def freeze_backbone(self, use_pe: bool=True):
+ if use_pe:
+ self.bert = freezer.freeze_lm_component(self.bert, "adapter")
+ else:
+ self.bert = freezer.unfreeze_lm(self.bert)
+
+
+ def forward(
+ self,
+ input_ids=None,
+ attention_mask=None,
+ token_type_ids=None,
+ position_ids=None,
+ head_mask=None,
+ inputs_embeds=None,
+ labels=None,
+ output_attentions=None,
+ output_hidden_states=None,
+ return_dict=None,
+ ):
+ return_dict = return_dict if return_dict is not None else self.config.use_return_dict
+
+ batch_size = input_ids.shape[0]
+ inputs_embeds = self.embeddings(
+ input_ids=input_ids,
+ position_ids=position_ids,
+ token_type_ids=token_type_ids,
+ )
+ outputs = self.bert(
+ # input_ids,
+ attention_mask=attention_mask,
+ # token_type_ids=token_type_ids,
+ # position_ids=position_ids,
+ head_mask=head_mask,
+ inputs_embeds=inputs_embeds,
+ output_attentions=output_attentions,
+ output_hidden_states=output_hidden_states,
+ return_dict=return_dict,
+ # past_key_values=past_key_values,
+ )
+
+ # pooled_output = outputs[1]
+ sequence_output = outputs[0]
+ # sequence_output = sequence_output[:, self.pre_seq_len:, :].contiguous()
+ first_token_tensor = sequence_output[:, 0]
+ pooled_output = self.bert.pooler.dense(first_token_tensor)
+ pooled_output = self.bert.pooler.activation(pooled_output)
+
+ pooled_output = self.dropout(pooled_output)
+ logits = self.classifier(pooled_output)
+
+ loss = None
+ if labels is not None:
+ if self.config.problem_type is None:
+ if self.num_labels == 1:
+ self.config.problem_type = "regression"
+ elif self.num_labels > 1 and (labels.dtype == torch.long or labels.dtype == torch.int):
+ self.config.problem_type = "single_label_classification"
+ else:
+ self.config.problem_type = "multi_label_classification"
+
+ if self.config.problem_type == "regression":
+ loss_fct = MSELoss()
+ if self.num_labels == 1:
+ loss = loss_fct(logits.squeeze(), labels.squeeze())
+ else:
+ loss = loss_fct(logits, labels)
+ elif self.config.problem_type == "single_label_classification":
+ loss_fct = CrossEntropyLoss()
+ loss = loss_fct(logits.view(-1, self.num_labels), labels.view(-1))
+ elif self.config.problem_type == "multi_label_classification":
+ loss_fct = BCEWithLogitsLoss()
+ loss = loss_fct(logits, labels)
+ if not return_dict:
+ output = (logits,) + outputs[2:]
+ return ((loss,) + output) if loss is not None else output
+
+ return SequenceClassifierOutput(
+ loss=loss,
+ logits=logits,
+ hidden_states=outputs.hidden_states,
+ attentions=outputs.attentions,
+ )
+
+
+
+# ========= RoBERTa =========
+
+# Vanilla Fine-tuning For RoBERTa
+class RobertForSequenceClassification(RobertaPreTrainedModel):
+ def __init__(self, config):
+ super().__init__(config)
+ self.num_labels = config.num_labels
+ self.config = config
+ self.roberta = RobertaModel(config)
+ if self.config.use_pe:
+ self.roberta = freezer.freeze_lm(self.roberta)
+ self.dropout = torch.nn.Dropout(config.hidden_dropout_prob)
+ self.classifier = torch.nn.Linear(config.hidden_size, config.num_labels)
+ self.init_weights()
+
+ def freeze_backbone(self, use_pe: bool=True):
+ if use_pe:
+ self.roberta = freezer.freeze_lm(self.roberta)
+ else:
+ self.roberta = freezer.unfreeze_lm(self.roberta)
+
+ def forward(
+ self,
+ input_ids=None,
+ attention_mask=None,
+ token_type_ids=None,
+ position_ids=None,
+ head_mask=None,
+ inputs_embeds=None,
+ labels=None,
+ output_attentions=None,
+ output_hidden_states=None,
+ return_dict=None,
+ ):
+ r"""
+ labels (:obj:`torch.LongTensor` of shape :obj:`(batch_size,)`, `optional`):
+ Labels for computing the sequence classification/regression loss. Indices should be in :obj:`[0, ...,
+ config.num_labels - 1]`. If :obj:`config.num_labels == 1` a regression loss is computed (Mean-Square loss),
+ If :obj:`config.num_labels > 1` a classification loss is computed (Cross-Entropy).
+ """
+ return_dict = return_dict if return_dict is not None else self.config.use_return_dict
+
+ outputs = self.roberta(
+ input_ids,
+ attention_mask=attention_mask,
+ token_type_ids=token_type_ids,
+ position_ids=position_ids,
+ head_mask=head_mask,
+ inputs_embeds=inputs_embeds,
+ output_attentions=output_attentions,
+ output_hidden_states=output_hidden_states,
+ return_dict=return_dict,
+ )
+
+ pooled_output = outputs[1]
+
+ pooled_output = self.dropout(pooled_output)
+ logits = self.classifier(pooled_output)
+
+ loss = None
+ if labels is not None:
+ if self.config.problem_type is None:
+ if self.num_labels == 1:
+ self.config.problem_type = "regression"
+ elif self.num_labels > 1 and (labels.dtype == torch.long or labels.dtype == torch.int):
+ self.config.problem_type = "single_label_classification"
+ else:
+ self.config.problem_type = "multi_label_classification"
+
+ if self.config.problem_type == "regression":
+ loss_fct = MSELoss()
+ if self.num_labels == 1:
+ loss = loss_fct(logits.squeeze(), labels.squeeze())
+ else:
+ loss = loss_fct(logits, labels)
+ elif self.config.problem_type == "single_label_classification":
+ loss_fct = CrossEntropyLoss()
+ loss = loss_fct(logits.view(-1, self.num_labels), labels.view(-1))
+ elif self.config.problem_type == "multi_label_classification":
+ loss_fct = BCEWithLogitsLoss()
+ loss = loss_fct(logits, labels)
+ if not return_dict:
+ output = (logits,) + outputs[2:]
+ return ((loss,) + output) if loss is not None else output
+
+ return SequenceClassifierOutput(
+ loss=loss,
+ logits=logits,
+ hidden_states=outputs.hidden_states,
+ attentions=outputs.attentions,
+ )
+
+# Prefix-tuning For RoBERTa
+class RobertaPrefixForSequenceClassification(RobertaPreTrainedModel):
+ def __init__(self, config):
+ super().__init__(config)
+ self.num_labels = config.num_labels
+ self.config = config
+ self.roberta = RobertaModel(config)
+ self.dropout = torch.nn.Dropout(config.hidden_dropout_prob)
+ self.classifier = torch.nn.Linear(config.hidden_size, config.num_labels)
+ self.init_weights()
+
+ for param in self.roberta.parameters():
+ param.requires_grad = False
+ # if self.config.use_pe:
+ # self.roberta = freezer.freeze_lm(self.roberta)
+
+ self.pre_seq_len = config.pre_seq_len
+ self.n_layer = config.num_hidden_layers
+ self.n_head = config.num_attention_heads
+ self.n_embd = config.hidden_size // config.num_attention_heads
+
+ self.prefix_tokens = torch.arange(self.pre_seq_len).long()
+ self.prefix_encoder = PrefixEncoder(config)
+
+ bert_param = 0
+ for name, param in self.roberta.named_parameters():
+ bert_param += param.numel()
+ all_param = 0
+ for name, param in self.named_parameters():
+ all_param += param.numel()
+ total_param = all_param - bert_param
+ print('total param is {}'.format(total_param)) # 9860105
+
+ def freeze_backbone(self, use_pe: bool=True):
+ if use_pe:
+ self.roberta = freezer.freeze_lm(self.roberta)
+ else:
+ self.roberta = freezer.unfreeze_lm(self.roberta)
+
+
+ def get_prompt(self, batch_size):
+ prefix_tokens = self.prefix_tokens.unsqueeze(0).expand(batch_size, -1).to(self.roberta.device)
+ # print("prefix_tokens.shape=", prefix_tokens.shape)
+ past_key_values = self.prefix_encoder(prefix_tokens)
+ # print("past_key_values[0].shape=", past_key_values[0].shape)
+ past_key_values = past_key_values.view(
+ batch_size,
+ self.pre_seq_len,
+ self.n_layer * 2,
+ self.n_head,
+ self.n_embd
+ )
+ # print("past_key_values[0].shape=", past_key_values[0].shape)
+ past_key_values = self.dropout(past_key_values)
+ past_key_values = past_key_values.permute([2, 0, 3, 1, 4]).split(2)
+ # print("past_key_values[0].shape=", past_key_values[0].shape)
+ return past_key_values
+
+ def forward(
+ self,
+ input_ids=None,
+ attention_mask=None,
+ token_type_ids=None,
+ position_ids=None,
+ head_mask=None,
+ inputs_embeds=None,
+ labels=None,
+ output_attentions=None,
+ output_hidden_states=None,
+ return_dict=None,
+ ):
+ return_dict = return_dict if return_dict is not None else self.config.use_return_dict
+
+ batch_size = input_ids.shape[0]
+ # print("batch_size=", batch_size)
+ past_key_values = self.get_prompt(batch_size=batch_size)
+ prefix_attention_mask = torch.ones(batch_size, self.pre_seq_len).to(self.roberta.device)
+ attention_mask = torch.cat((prefix_attention_mask, attention_mask), dim=1)
+ # print("prefix_attention_mask.shape=", prefix_attention_mask.shape)
+ # print("attention_mask.shape=", attention_mask.shape)
+ outputs = self.roberta(
+ input_ids,
+ attention_mask=attention_mask,
+ token_type_ids=token_type_ids,
+ position_ids=position_ids,
+ head_mask=head_mask,
+ inputs_embeds=inputs_embeds,
+ output_attentions=output_attentions,
+ output_hidden_states=output_hidden_states,
+ return_dict=return_dict,
+ past_key_values=past_key_values,
+ )
+
+ pooled_output = outputs[1]
+
+ pooled_output = self.dropout(pooled_output)
+ logits = self.classifier(pooled_output)
+
+ # print("labels=", labels)
+
+
+ loss = None
+ if labels is not None:
+ labels = (labels < 0).long().to(labels.device) + labels
+
+ if self.config.problem_type is None:
+ if self.num_labels == 1:
+ self.config.problem_type = "regression"
+ elif self.num_labels > 1 and (labels.dtype == torch.long or labels.dtype == torch.int):
+ self.config.problem_type = "single_label_classification"
+ else:
+ self.config.problem_type = "multi_label_classification"
+
+ if self.config.problem_type == "regression":
+ loss_fct = MSELoss()
+ if self.num_labels == 1:
+ loss = loss_fct(logits.squeeze(), labels.squeeze())
+ else:
+ loss = loss_fct(logits, labels)
+ elif self.config.problem_type == "single_label_classification":
+ loss_fct = CrossEntropyLoss()
+ loss = loss_fct(logits.view(-1, self.num_labels), labels.view(-1))
+ elif self.config.problem_type == "multi_label_classification":
+ loss_fct = BCEWithLogitsLoss()
+ loss = loss_fct(logits, labels)
+ if not return_dict:
+ output = (logits,) + outputs[2:]
+ return ((loss,) + output) if loss is not None else output
+
+ return SequenceClassifierOutput(
+ loss=loss,
+ logits=logits,
+ hidden_states=outputs.hidden_states,
+ attentions=outputs.attentions,
+ )
+
+from transformers.models.bert.modeling_bert import BertForSequenceClassification
+
+# Prompt-tuning For RoBERTa
+class RobertaPtuningForSequenceClassification(RobertaPreTrainedModel):
+ def __init__(self, config):
+ super().__init__(config)
+ self.num_labels = config.num_labels
+ self.roberta = RobertaModel(config)
+ self.embeddings = self.roberta.embeddings
+ self.dropout = torch.nn.Dropout(config.hidden_dropout_prob)
+ self.classifier = torch.nn.Linear(config.hidden_size, config.num_labels)
+
+ # for param in self.roberta.parameters():
+ # param.requires_grad = False
+
+ if self.config.use_pe:
+ self.roberta = freezer.freeze_lm(self.roberta)
+
+ self.pre_seq_len = config.pre_seq_len
+ self.n_layer = config.num_hidden_layers
+ self.n_head = config.num_attention_heads
+ self.n_embd = config.hidden_size // config.num_attention_heads
+
+ self.prefix_tokens = torch.arange(self.pre_seq_len).long()
+ self.prefix_encoder = torch.nn.Embedding(self.pre_seq_len, config.hidden_size)
+
+ def freeze_backbone(self, use_pe: bool=True):
+ if use_pe:
+ self.roberta = freezer.freeze_lm(self.roberta)
+ else:
+ self.roberta = freezer.unfreeze_lm(self.roberta)
+
+ def get_prompt(self, batch_size):
+ prefix_tokens = self.prefix_tokens.unsqueeze(0).expand(batch_size, -1).to(self.roberta.device)
+ prompts = self.prefix_encoder(prefix_tokens)
+ return prompts
+
+ def forward(
+ self,
+ input_ids=None,
+ attention_mask=None,
+ token_type_ids=None,
+ position_ids=None,
+ head_mask=None,
+ inputs_embeds=None,
+ labels=None,
+ output_attentions=None,
+ output_hidden_states=None,
+ return_dict=None,
+ ):
+ return_dict = return_dict if return_dict is not None else self.config.use_return_dict
+
+ batch_size = input_ids.shape[0]
+ raw_embedding = self.embeddings(
+ input_ids=input_ids,
+ position_ids=position_ids,
+ token_type_ids=token_type_ids,
+ )
+ prompts = self.get_prompt(batch_size=batch_size)
+ inputs_embeds = torch.cat((prompts, raw_embedding), dim=1)
+ # print(input_embeddings.shape)
+ # exit()
+ prefix_attention_mask = torch.ones(batch_size, self.pre_seq_len).to(self.roberta.device)
+ attention_mask = torch.cat((prefix_attention_mask, attention_mask), dim=1)
+
+ outputs = self.roberta(
+ # input_ids,
+ attention_mask=attention_mask,
+ # token_type_ids=token_type_ids,
+ # position_ids=position_ids,
+ head_mask=head_mask,
+ inputs_embeds=inputs_embeds,
+ output_attentions=output_attentions,
+ output_hidden_states=output_hidden_states,
+ return_dict=return_dict,
+ # past_key_values=past_key_values,
+ )
+
+ # pooled_output = outputs[1]
+ sequence_output = outputs[0]
+ sequence_output = sequence_output[:, self.pre_seq_len:, :].contiguous()
+ first_token_tensor = sequence_output[:, 0]
+ pooled_output = self.roberta.pooler.dense(first_token_tensor)
+ pooled_output = self.roberta.pooler.activation(pooled_output)
+
+ pooled_output = self.dropout(pooled_output)
+ logits = self.classifier(pooled_output)
+
+ loss = None
+ if labels is not None:
+ if self.config.problem_type is None:
+ if self.num_labels == 1:
+ self.config.problem_type = "regression"
+ elif self.num_labels > 1 and (labels.dtype == torch.long or labels.dtype == torch.int):
+ self.config.problem_type = "single_label_classification"
+ else:
+ self.config.problem_type = "multi_label_classification"
+
+ if self.config.problem_type == "regression":
+ loss_fct = MSELoss()
+ if self.num_labels == 1:
+ loss = loss_fct(logits.squeeze(), labels.squeeze())
+ else:
+ loss = loss_fct(logits, labels)
+ elif self.config.problem_type == "single_label_classification":
+ loss_fct = CrossEntropyLoss()
+ loss = loss_fct(logits.view(-1, self.num_labels), labels.view(-1))
+ elif self.config.problem_type == "multi_label_classification":
+ loss_fct = BCEWithLogitsLoss()
+ loss = loss_fct(logits, labels)
+ if not return_dict:
+ output = (logits,) + outputs[2:]
+ return ((loss,) + output) if loss is not None else output
+
+ return SequenceClassifierOutput(
+ loss=loss,
+ logits=logits,
+ hidden_states=outputs.hidden_states,
+ attentions=outputs.attentions,
+ )
+
+# Adapter-tuning For RoBERTa
+class RobertaAdapterForSequenceClassification(RobertaPreTrainedModel):
+ def __init__(self, config):
+ super().__init__(config)
+ self.num_labels = config.num_labels
+ self.roberta = RobertaAdaModel(config)
+ self.embeddings = self.roberta.embeddings
+ self.dropout = torch.nn.Dropout(config.hidden_dropout_prob)
+ self.classifier = torch.nn.Linear(config.hidden_size, config.num_labels)
+ self.init_weights()
+ # for param in self.roberta.parameters():
+ # param.requires_grad = False
+ self.roberta = init_adapter(self.roberta)
+ if self.config.use_pe:
+ self.roberta = freezer.freeze_lm_component(self.roberta, "adapter")
+
+ def freeze_backbone(self, use_pe: bool=True):
+ if use_pe:
+ self.roberta = freezer.freeze_lm_component(self.roberta, "adapter")
+ else:
+ self.roberta = freezer.unfreeze_lm(self.roberta)
+
+ def forward(
+ self,
+ input_ids=None,
+ attention_mask=None,
+ token_type_ids=None,
+ position_ids=None,
+ head_mask=None,
+ inputs_embeds=None,
+ labels=None,
+ output_attentions=None,
+ output_hidden_states=None,
+ return_dict=None,
+ ):
+ return_dict = return_dict if return_dict is not None else self.config.use_return_dict
+
+ batch_size = input_ids.shape[0]
+ inputs_embeds = self.embeddings(
+ input_ids=input_ids,
+ position_ids=position_ids,
+ token_type_ids=token_type_ids,
+ )
+
+ outputs = self.roberta(
+ # input_ids,
+ attention_mask=attention_mask,
+ # token_type_ids=token_type_ids,
+ # position_ids=position_ids,
+ head_mask=head_mask,
+ inputs_embeds=inputs_embeds,
+ output_attentions=output_attentions,
+ output_hidden_states=output_hidden_states,
+ return_dict=return_dict,
+ # past_key_values=past_key_values,
+ )
+
+ # pooled_output = outputs[1]
+ sequence_output = outputs[0]
+ # sequence_output = sequence_output[:, self.pre_seq_len:, :].contiguous()
+ first_token_tensor = sequence_output[:, 0]
+ pooled_output = self.roberta.pooler.dense(first_token_tensor)
+ pooled_output = self.roberta.pooler.activation(pooled_output)
+
+ pooled_output = self.dropout(pooled_output)
+ logits = self.classifier(pooled_output)
+
+ loss = None
+ if labels is not None:
+ if self.config.problem_type is None:
+ if self.num_labels == 1:
+ self.config.problem_type = "regression"
+ elif self.num_labels > 1 and (labels.dtype == torch.long or labels.dtype == torch.int):
+ self.config.problem_type = "single_label_classification"
+ else:
+ self.config.problem_type = "multi_label_classification"
+
+ if self.config.problem_type == "regression":
+ loss_fct = MSELoss()
+ if self.num_labels == 1:
+ loss = loss_fct(logits.squeeze(), labels.squeeze())
+ else:
+ loss = loss_fct(logits, labels)
+ elif self.config.problem_type == "single_label_classification":
+ loss_fct = CrossEntropyLoss()
+ loss = loss_fct(logits.view(-1, self.num_labels), labels.view(-1))
+ elif self.config.problem_type == "multi_label_classification":
+ loss_fct = BCEWithLogitsLoss()
+ loss = loss_fct(logits, labels)
+ if not return_dict:
+ output = (logits,) + outputs[2:]
+ return ((loss,) + output) if loss is not None else output
+
+ return SequenceClassifierOutput(
+ loss=loss,
+ logits=logits,
+ hidden_states=outputs.hidden_states,
+ attentions=outputs.attentions,
+ )
+
+
+# ========= DeBERTa =========
+
+# Prefix-tuning For DeBERTa
+class DebertaPrefixForSequenceClassification(DebertaPreTrainedModel):
+ def __init__(self, config):
+ super().__init__(config)
+ self.num_labels = config.num_labels
+ self.config = config
+ self.deberta = DebertaModel(config)
+ self.pooler = ContextPooler(config)
+ output_dim = self.pooler.output_dim
+ self.classifier = torch.nn.Linear(output_dim, self.num_labels)
+ self.dropout = StableDropout(config.hidden_dropout_prob)
+ self.init_weights()
+
+ # for param in self.deberta.parameters():
+ # param.requires_grad = False
+
+ if self.config.use_pe:
+ self.deberta = freezer.freeze_lm(self.deberta)
+
+ self.pre_seq_len = config.pre_seq_len
+ self.n_layer = config.num_hidden_layers
+ self.n_head = config.num_attention_heads
+ self.n_embd = config.hidden_size // config.num_attention_heads
+
+ self.prefix_tokens = torch.arange(self.pre_seq_len).long()
+ self.prefix_encoder = PrefixEncoder(config)
+
+ deberta_param = 0
+ for name, param in self.deberta.named_parameters():
+ deberta_param += param.numel()
+ all_param = 0
+ for name, param in self.named_parameters():
+ all_param += param.numel()
+ total_param = all_param - deberta_param
+ print('total param is {}'.format(total_param)) # 9860105
+
+ def freeze_backbone(self, use_pe: bool=True):
+ if use_pe:
+ self.deberta = freezer.freeze_lm(self.deberta)
+ else:
+ self.deberta = freezer.unfreeze_lm(self.deberta)
+
+ def get_prompt(self, batch_size):
+ prefix_tokens = self.prefix_tokens.unsqueeze(0).expand(batch_size, -1).to(self.deberta.device)
+ past_key_values = self.prefix_encoder(prefix_tokens)
+ # bsz, seqlen, _ = past_key_values.shape
+ past_key_values = past_key_values.view(
+ batch_size,
+ self.pre_seq_len,
+ self.n_layer * 2,
+ self.n_head,
+ self.n_embd
+ )
+ past_key_values = self.dropout(past_key_values)
+ past_key_values = past_key_values.permute([2, 0, 3, 1, 4]).split(2)
+ return past_key_values
+
+ def forward(
+ self,
+ input_ids=None,
+ attention_mask=None,
+ token_type_ids=None,
+ position_ids=None,
+ head_mask=None,
+ inputs_embeds=None,
+ labels=None,
+ output_attentions=None,
+ output_hidden_states=None,
+ return_dict=None,
+ ):
+ return_dict = return_dict if return_dict is not None else self.config.use_return_dict
+
+ batch_size = input_ids.shape[0]
+ past_key_values = self.get_prompt(batch_size=batch_size)
+ prefix_attention_mask = torch.ones(batch_size, self.pre_seq_len).to(self.deberta.device)
+ attention_mask = torch.cat((prefix_attention_mask, attention_mask), dim=1)
+
+ outputs = self.deberta(
+ input_ids,
+ attention_mask=attention_mask,
+ token_type_ids=token_type_ids,
+ position_ids=position_ids,
+ inputs_embeds=inputs_embeds,
+ output_attentions=output_attentions,
+ output_hidden_states=output_hidden_states,
+ return_dict=return_dict,
+ past_key_values=past_key_values,
+ )
+
+ encoder_layer = outputs[0]
+ pooled_output = self.pooler(encoder_layer)
+ pooled_output = self.dropout(pooled_output)
+ logits = self.classifier(pooled_output)
+
+ loss = None
+ if labels is not None:
+ if self.num_labels == 1:
+ # regression task
+ loss_fn = torch.nn.MSELoss()
+ logits = logits.view(-1).to(labels.dtype)
+ loss = loss_fn(logits, labels.view(-1))
+ elif labels.dim() == 1 or labels.size(-1) == 1:
+ label_index = (labels >= 0).nonzero()
+ labels = labels.long()
+ if label_index.size(0) > 0:
+ labeled_logits = torch.gather(logits, 0, label_index.expand(label_index.size(0), logits.size(1)))
+ labels = torch.gather(labels, 0, label_index.view(-1))
+ loss_fct = CrossEntropyLoss()
+ loss = loss_fct(labeled_logits.view(-1, self.num_labels).float(), labels.view(-1))
+ else:
+ loss = torch.tensor(0).to(logits)
+ else:
+ log_softmax = torch.nn.LogSoftmax(-1)
+ loss = -((log_softmax(logits) * labels).sum(-1)).mean()
+ if not return_dict:
+ output = (logits,) + outputs[1:]
+ return ((loss,) + output) if loss is not None else output
+ else:
+ return SequenceClassifierOutput(
+ loss=loss,
+ logits=logits,
+ hidden_states=outputs.hidden_states,
+ attentions=outputs.attentions,
+ )
diff --git a/model/head_for_token_classification.py b/model/head_for_token_classification.py
new file mode 100644
index 0000000..abe5ab1
--- /dev/null
+++ b/model/head_for_token_classification.py
@@ -0,0 +1,720 @@
+import torch
+import torch.nn
+import torch.nn.functional as F
+from torch import Tensor
+from torch.nn import CrossEntropyLoss
+
+from transformers import BertModel, BertPreTrainedModel
+from transformers import RobertaModel, RobertaPreTrainedModel
+from transformers.modeling_outputs import TokenClassifierOutput
+
+from model.prefix_encoder import PrefixEncoder
+from model.deberta import DebertaModel, DebertaPreTrainedModel
+from model.debertaV2 import DebertaV2Model, DebertaV2PreTrainedModel
+from model.model_adaptation import BertAdaModel, RobertaAdaModel
+
+from transformers.models.t5.modeling_t5 import T5PreTrainedModel
+from model.parameter_freeze import ParameterFreeze
+
+freezer = ParameterFreeze()
+
+## ======== BERT ========
+
+# Vanilla Fine-tuning For BERT
+class BertForTokenClassification(BertPreTrainedModel):
+
+ _keys_to_ignore_on_load_unexpected = [r"pooler"]
+
+ def __init__(self, config):
+ super().__init__(config)
+ self.num_labels = config.num_labels
+
+ self.bert = BertModel(config, add_pooling_layer=False)
+ self.dropout = torch.nn.Dropout(config.hidden_dropout_prob)
+ self.classifier = torch.nn.Linear(config.hidden_size, config.num_labels)
+
+ only_cls_head = True # False in SRL
+ if only_cls_head:
+ for param in self.bert.parameters():
+ param.requires_grad = False
+
+ self.init_weights()
+
+ bert_param = 0
+ for name, param in self.bert.named_parameters():
+ bert_param += param.numel()
+ all_param = 0
+ for name, param in self.named_parameters():
+ all_param += param.numel()
+ total_param = all_param - bert_param
+ print('total param is {}'.format(total_param))
+
+
+ def forward(
+ self,
+ input_ids=None,
+ attention_mask=None,
+ token_type_ids=None,
+ position_ids=None,
+ head_mask=None,
+ inputs_embeds=None,
+ labels=None,
+ output_attentions=None,
+ output_hidden_states=None,
+ return_dict=None,
+ ):
+ r"""
+ labels (:obj:`torch.LongTensor` of shape :obj:`(batch_size, sequence_length)`, `optional`):
+ Labels for computing the token classification loss. Indices should be in ``[0, ..., config.num_labels -
+ 1]``.
+ """
+ return_dict = return_dict if return_dict is not None else self.config.use_return_dict
+
+ outputs = self.bert(
+ input_ids,
+ attention_mask=attention_mask,
+ token_type_ids=token_type_ids,
+ position_ids=position_ids,
+ head_mask=head_mask,
+ inputs_embeds=inputs_embeds,
+ output_attentions=output_attentions,
+ output_hidden_states=output_hidden_states,
+ return_dict=return_dict,
+ )
+
+ sequence_output = outputs[0]
+
+ sequence_output = self.dropout(sequence_output)
+ logits = self.classifier(sequence_output)
+
+ loss = None
+ if labels is not None:
+ loss_fct = CrossEntropyLoss()
+ # Only keep active parts of the loss
+ if attention_mask is not None:
+ active_loss = attention_mask.view(-1) == 1
+ active_logits = logits.view(-1, self.num_labels)
+ active_labels = torch.where(
+ active_loss, labels.view(-1), torch.tensor(loss_fct.ignore_index).type_as(labels)
+ )
+ loss = loss_fct(active_logits, active_labels)
+ else:
+ loss = loss_fct(logits.view(-1, self.num_labels), labels.view(-1))
+
+ if not return_dict:
+ output = (logits,) + outputs[2:]
+ return ((loss,) + output) if loss is not None else output
+
+ return TokenClassifierOutput(
+ loss=loss,
+ logits=logits,
+ hidden_states=outputs.hidden_states,
+ attentions=outputs.attentions,
+ )
+
+
+
+# Prefix-tuning For BERT
+class BertPrefixForTokenClassification(BertPreTrainedModel):
+ def __init__(self, config):
+ super().__init__(config)
+ self.num_labels = config.num_labels
+ self.bert = BertModel(config, add_pooling_layer=False)
+ self.dropout = torch.nn.Dropout(config.hidden_dropout_prob)
+ self.classifier = torch.nn.Linear(config.hidden_size, config.num_labels)
+
+ from_pretrained = False
+ if from_pretrained:
+ self.classifier.load_state_dict(torch.load('model/checkpoint.pkl'))
+
+ # for param in self.bert.parameters():
+ # param.requires_grad = False
+ self.bert = freezer.freeze_lm(self.bert)
+
+ self.pre_seq_len = config.pre_seq_len
+ self.n_layer = config.num_hidden_layers
+ self.n_head = config.num_attention_heads
+ self.n_embd = config.hidden_size // config.num_attention_heads
+
+ self.prefix_tokens = torch.arange(self.pre_seq_len).long()
+ self.prefix_encoder = PrefixEncoder(config)
+
+
+ bert_param = 0
+ for name, param in self.bert.named_parameters():
+ bert_param += param.numel()
+ all_param = 0
+ for name, param in self.named_parameters():
+ all_param += param.numel()
+ total_param = all_param - bert_param
+ print('total param is {}'.format(total_param)) # 9860105
+
+ def get_prompt(self, batch_size):
+ prefix_tokens = self.prefix_tokens.unsqueeze(0).expand(batch_size, -1).to(self.bert.device)
+ past_key_values = self.prefix_encoder(prefix_tokens)
+ # bsz, seqlen, _ = past_key_values.shape
+ past_key_values = past_key_values.view(
+ batch_size,
+ self.pre_seq_len,
+ self.n_layer * 2,
+ self.n_head,
+ self.n_embd
+ )
+ past_key_values = self.dropout(past_key_values)
+ past_key_values = past_key_values.permute([2, 0, 3, 1, 4]).split(2)
+ return past_key_values
+
+ def forward(
+ self,
+ input_ids=None,
+ attention_mask=None,
+ token_type_ids=None,
+ position_ids=None,
+ head_mask=None,
+ inputs_embeds=None,
+ labels=None,
+ output_attentions=None,
+ output_hidden_states=None,
+ return_dict=None,
+ ):
+ return_dict = return_dict if return_dict is not None else self.config.use_return_dict
+
+ batch_size = input_ids.shape[0]
+ past_key_values = self.get_prompt(batch_size=batch_size)
+ prefix_attention_mask = torch.ones(batch_size, self.pre_seq_len).to(self.bert.device)
+ attention_mask = torch.cat((prefix_attention_mask, attention_mask), dim=1)
+
+ outputs = self.bert(
+ input_ids,
+ attention_mask=attention_mask,
+ token_type_ids=token_type_ids,
+ position_ids=position_ids,
+ head_mask=head_mask,
+ inputs_embeds=inputs_embeds,
+ output_attentions=output_attentions,
+ output_hidden_states=output_hidden_states,
+ return_dict=return_dict,
+ past_key_values=past_key_values,
+ )
+
+ sequence_output = outputs[0]
+ sequence_output = self.dropout(sequence_output)
+ logits = self.classifier(sequence_output)
+ attention_mask = attention_mask[:,self.pre_seq_len:].contiguous()
+
+ loss = None
+ if labels is not None:
+ loss_fct = CrossEntropyLoss()
+ # Only keep active parts of the loss
+ if attention_mask is not None:
+ active_loss = attention_mask.view(-1) == 1
+ active_logits = logits.view(-1, self.num_labels)
+ active_labels = torch.where(
+ active_loss, labels.view(-1), torch.tensor(loss_fct.ignore_index).type_as(labels)
+ )
+ loss = loss_fct(active_logits, active_labels)
+ else:
+ loss = loss_fct(logits.view(-1, self.num_labels), labels.view(-1))
+
+ if not return_dict:
+ output = (logits,) + outputs[2:]
+ return ((loss,) + output) if loss is not None else output
+
+ return TokenClassifierOutput(
+ loss=loss,
+ logits=logits,
+ hidden_states=outputs.hidden_states,
+ attentions=outputs.attentions,
+ )
+
+
+# Adapter-tuning For BERT
+class BertAdapterForTokenClassification(BertPreTrainedModel):
+ def __init__(self, config):
+ super().__init__(config)
+ self.num_labels = config.num_labels
+ self.bert = BertAdaModel(config, add_pooling_layer=False)
+ self.embeddings = self.bert.embeddings
+ self.dropout = torch.nn.Dropout(config.hidden_dropout_prob)
+ self.classifier = torch.nn.Linear(config.hidden_size, config.num_labels)
+
+ from_pretrained = False
+ if from_pretrained:
+ self.classifier.load_state_dict(torch.load('model/checkpoint.pkl'))
+
+ # for param in self.bert.parameters():
+ # param.requires_grad = False
+ self.bert = freezer.freeze_lm_component(self.bert, "adapter")
+
+ def forward(
+ self,
+ input_ids=None,
+ attention_mask=None,
+ token_type_ids=None,
+ position_ids=None,
+ head_mask=None,
+ inputs_embeds=None,
+ labels=None,
+ output_attentions=None,
+ output_hidden_states=None,
+ return_dict=None,
+ ):
+ return_dict = return_dict if return_dict is not None else self.config.use_return_dict
+
+ batch_size = input_ids.shape[0]
+ inputs_embeds = self.embeddings(
+ input_ids=input_ids,
+ position_ids=position_ids,
+ token_type_ids=token_type_ids,
+ )
+
+ outputs = self.bert(
+ # input_ids,
+ attention_mask=attention_mask,
+ # token_type_ids=token_type_ids,
+ # position_ids=position_ids,
+ head_mask=head_mask,
+ inputs_embeds=inputs_embeds,
+ output_attentions=output_attentions,
+ output_hidden_states=output_hidden_states,
+ return_dict=return_dict,
+ # past_key_values=past_key_values,
+ )
+
+ sequence_output = outputs[0]
+ sequence_output = self.dropout(sequence_output)
+ logits = self.classifier(sequence_output)
+ # attention_mask = attention_mask[:,self.pre_seq_len:].contiguous()
+
+ loss = None
+ if labels is not None:
+ loss_fct = CrossEntropyLoss()
+ # Only keep active parts of the loss
+ if attention_mask is not None:
+ active_loss = attention_mask.view(-1) == 1
+ active_logits = logits.view(-1, self.num_labels)
+ active_labels = torch.where(
+ active_loss, labels.view(-1), torch.tensor(loss_fct.ignore_index).type_as(labels)
+ )
+ loss = loss_fct(active_logits, active_labels)
+ else:
+ loss = loss_fct(logits.view(-1, self.num_labels), labels.view(-1))
+
+ if not return_dict:
+ output = (logits,) + outputs[2:]
+ return ((loss,) + output) if loss is not None else output
+
+ return TokenClassifierOutput(
+ loss=loss,
+ logits=logits,
+ hidden_states=outputs.hidden_states,
+ attentions=outputs.attentions,
+ )
+
+## ======== RoBERTa ========
+
+# Prefix-tuning For RoBERTa
+class RobertaPrefixForTokenClassification(RobertaPreTrainedModel):
+ def __init__(self, config):
+ super().__init__(config)
+ self.num_labels = config.num_labels
+ self.roberta = RobertaModel(config, add_pooling_layer=False)
+ self.dropout = torch.nn.Dropout(config.hidden_dropout_prob)
+ self.classifier = torch.nn.Linear(config.hidden_size, config.num_labels)
+ self.init_weights()
+
+ # for param in self.roberta.parameters():
+ # param.requires_grad = False
+ self.bert = freezer.freeze_lm(self.bert)
+
+ self.pre_seq_len = config.pre_seq_len # prefix prompt token数量
+ self.n_layer = config.num_hidden_layers
+ self.n_head = config.num_attention_heads
+ self.n_embd = config.hidden_size // config.num_attention_heads
+
+ self.prefix_tokens = torch.arange(self.pre_seq_len).long() # e.g., tensor([0, 1, 2, 3])
+ self.prefix_encoder = PrefixEncoder(config)
+
+ bert_param = 0
+ for name, param in self.roberta.named_parameters():
+ bert_param += param.numel()
+ all_param = 0
+ for name, param in self.named_parameters():
+ all_param += param.numel()
+ total_param = all_param - bert_param
+ print('total param is {}'.format(total_param)) # 9860105
+
+
+ def get_prompt(self, batch_size):
+ prefix_tokens = self.prefix_tokens.unsqueeze(0).expand(batch_size, -1).to(self.roberta.device)
+ past_key_values = self.prefix_encoder(prefix_tokens)
+ past_key_values = past_key_values.view(
+ batch_size,
+ self.pre_seq_len,
+ self.n_layer * 2,
+ self.n_head,
+ self.n_embd
+ )
+ past_key_values = self.dropout(past_key_values)
+ past_key_values = past_key_values.permute([2, 0, 3, 1, 4]).split(2)
+ return past_key_values
+
+ def forward(
+ self,
+ input_ids=None,
+ attention_mask=None,
+ token_type_ids=None,
+ position_ids=None,
+ head_mask=None,
+ inputs_embeds=None,
+ labels=None,
+ output_attentions=None,
+ output_hidden_states=None,
+ return_dict=None,
+ ):
+ return_dict = return_dict if return_dict is not None else self.config.use_return_dict
+
+ batch_size = input_ids.shape[0]
+ past_key_values = self.get_prompt(batch_size=batch_size)
+ prefix_attention_mask = torch.ones(batch_size, self.pre_seq_len).to(self.roberta.device)
+ attention_mask = torch.cat((prefix_attention_mask, attention_mask), dim=1)
+
+ outputs = self.roberta(
+ input_ids,
+ attention_mask=attention_mask,
+ token_type_ids=token_type_ids,
+ position_ids=position_ids,
+ head_mask=head_mask,
+ inputs_embeds=inputs_embeds,
+ output_attentions=output_attentions,
+ output_hidden_states=output_hidden_states,
+ return_dict=return_dict,
+ past_key_values=past_key_values,
+ )
+
+ sequence_output = outputs[0]
+ sequence_output = self.dropout(sequence_output)
+ logits = self.classifier(sequence_output)
+ attention_mask = attention_mask[:,self.pre_seq_len:].contiguous()
+
+ loss = None
+ if labels is not None:
+ loss_fct = CrossEntropyLoss()
+ # Only keep active parts of the loss
+ if attention_mask is not None:
+ active_loss = attention_mask.view(-1) == 1
+ active_logits = logits.view(-1, self.num_labels)
+ active_labels = torch.where(
+ active_loss, labels.view(-1), torch.tensor(loss_fct.ignore_index).type_as(labels)
+ )
+ loss = loss_fct(active_logits, active_labels)
+ else:
+ loss = loss_fct(logits.view(-1, self.num_labels), labels.view(-1))
+
+ if not return_dict:
+ output = (logits,) + outputs[2:]
+ return ((loss,) + output) if loss is not None else output
+
+ return TokenClassifierOutput(
+ loss=loss,
+ logits=logits,
+ hidden_states=outputs.hidden_states,
+ attentions=outputs.attentions,
+ )
+
+# Adapter-tuning For RoBERTa
+class RobertaAdapterForTokenClassification(RobertaPreTrainedModel):
+ def __init__(self, config):
+ super().__init__(config)
+ self.num_labels = config.num_labels
+ self.roberta = RobertaAdaModel(config, add_pooling_layer=False)
+ self.embeddings = self.roberta.embeddings
+ self.dropout = torch.nn.Dropout(config.hidden_dropout_prob)
+ self.classifier = torch.nn.Linear(config.hidden_size, config.num_labels)
+
+ from_pretrained = False
+ if from_pretrained:
+ self.classifier.load_state_dict(torch.load('model/checkpoint.pkl'))
+
+ # for param in self.bert.parameters():
+ # param.requires_grad = False
+ self.roberta = freezer.freeze_lm_component(self.roberta, "adapter")
+
+ def forward(
+ self,
+ input_ids=None,
+ attention_mask=None,
+ token_type_ids=None,
+ position_ids=None,
+ head_mask=None,
+ inputs_embeds=None,
+ labels=None,
+ output_attentions=None,
+ output_hidden_states=None,
+ return_dict=None,
+ ):
+ return_dict = return_dict if return_dict is not None else self.config.use_return_dict
+
+ batch_size = input_ids.shape[0]
+ inputs_embeds = self.embeddings(
+ input_ids=input_ids,
+ position_ids=position_ids,
+ token_type_ids=token_type_ids,
+ )
+
+ outputs = self.roberta(
+ # input_ids,
+ attention_mask=attention_mask,
+ # token_type_ids=token_type_ids,
+ # position_ids=position_ids,
+ head_mask=head_mask,
+ inputs_embeds=inputs_embeds,
+ output_attentions=output_attentions,
+ output_hidden_states=output_hidden_states,
+ return_dict=return_dict,
+ # past_key_values=past_key_values,
+ )
+
+ sequence_output = outputs[0]
+ sequence_output = self.dropout(sequence_output)
+ logits = self.classifier(sequence_output)
+ # attention_mask = attention_mask[:,self.pre_seq_len:].contiguous()
+
+ loss = None
+ if labels is not None:
+ loss_fct = CrossEntropyLoss()
+ # Only keep active parts of the loss
+ if attention_mask is not None:
+ active_loss = attention_mask.view(-1) == 1
+ active_logits = logits.view(-1, self.num_labels)
+ active_labels = torch.where(
+ active_loss, labels.view(-1), torch.tensor(loss_fct.ignore_index).type_as(labels)
+ )
+ loss = loss_fct(active_logits, active_labels)
+ else:
+ loss = loss_fct(logits.view(-1, self.num_labels), labels.view(-1))
+
+ if not return_dict:
+ output = (logits,) + outputs[2:]
+ return ((loss,) + output) if loss is not None else output
+
+ return TokenClassifierOutput(
+ loss=loss,
+ logits=logits,
+ hidden_states=outputs.hidden_states,
+ attentions=outputs.attentions,
+ )
+
+
+class DebertaPrefixForTokenClassification(DebertaPreTrainedModel):
+ def __init__(self, config):
+ super().__init__(config)
+ self.num_labels = config.num_labels
+ self.deberta = DebertaModel(config)
+ self.dropout = torch.nn.Dropout(config.hidden_dropout_prob)
+ self.classifier = torch.nn.Linear(config.hidden_size, config.num_labels)
+ self.init_weights()
+
+ # for param in self.deberta.parameters():
+ # param.requires_grad = False
+ self.deberta = freezer.freeze_lm(self.deberta)
+
+ self.pre_seq_len = config.pre_seq_len
+ self.n_layer = config.num_hidden_layers
+ self.n_head = config.num_attention_heads
+ self.n_embd = config.hidden_size // config.num_attention_heads
+
+ self.prefix_tokens = torch.arange(self.pre_seq_len).long()
+ self.prefix_encoder = PrefixEncoder(config)
+
+ deberta_param = 0
+ for name, param in self.deberta.named_parameters():
+ deberta_param += param.numel()
+ all_param = 0
+ for name, param in self.named_parameters():
+ all_param += param.numel()
+ total_param = all_param - deberta_param
+ print('total param is {}'.format(total_param)) # 9860105
+
+ def get_prompt(self, batch_size):
+ prefix_tokens = self.prefix_tokens.unsqueeze(0).expand(batch_size, -1).to(self.deberta.device)
+ past_key_values = self.prefix_encoder(prefix_tokens)
+ # bsz, seqlen, _ = past_key_values.shape
+ past_key_values = past_key_values.view(
+ batch_size,
+ self.pre_seq_len,
+ self.n_layer * 2,
+ self.n_head,
+ self.n_embd
+ )
+ past_key_values = self.dropout(past_key_values)
+ past_key_values = past_key_values.permute([2, 0, 3, 1, 4]).split(2)
+ return past_key_values
+
+ def forward(
+ self,
+ input_ids=None,
+ attention_mask=None,
+ token_type_ids=None,
+ position_ids=None,
+ head_mask=None,
+ inputs_embeds=None,
+ labels=None,
+ output_attentions=None,
+ output_hidden_states=None,
+ return_dict=None,
+ ):
+ return_dict = return_dict if return_dict is not None else self.config.use_return_dict
+
+ batch_size = input_ids.shape[0]
+ past_key_values = self.get_prompt(batch_size=batch_size)
+ prefix_attention_mask = torch.ones(batch_size, self.pre_seq_len).to(self.deberta.device)
+ attention_mask = torch.cat((prefix_attention_mask, attention_mask), dim=1)
+
+ outputs = self.deberta(
+ input_ids,
+ attention_mask=attention_mask,
+ token_type_ids=token_type_ids,
+ position_ids=position_ids,
+ inputs_embeds=inputs_embeds,
+ output_attentions=output_attentions,
+ output_hidden_states=output_hidden_states,
+ return_dict=return_dict,
+ past_key_values=past_key_values,
+ )
+
+ sequence_output = outputs[0]
+ sequence_output = self.dropout(sequence_output)
+ logits = self.classifier(sequence_output)
+ attention_mask = attention_mask[:,self.pre_seq_len:].contiguous()
+
+ loss = None
+ if labels is not None:
+ loss_fct = CrossEntropyLoss()
+ # Only keep active parts of the loss
+ if attention_mask is not None:
+ active_loss = attention_mask.view(-1) == 1
+ active_logits = logits.view(-1, self.num_labels)
+ active_labels = torch.where(
+ active_loss, labels.view(-1), torch.tensor(loss_fct.ignore_index).type_as(labels)
+ )
+ loss = loss_fct(active_logits, active_labels)
+ else:
+ loss = loss_fct(logits.view(-1, self.num_labels), labels.view(-1))
+
+ if not return_dict:
+ output = (logits,) + outputs[2:]
+ return ((loss,) + output) if loss is not None else output
+
+ return TokenClassifierOutput(
+ loss=loss,
+ logits=logits,
+ hidden_states=outputs.hidden_states,
+ attentions=outputs.attentions,
+ )
+
+
+class DebertaV2PrefixForTokenClassification(DebertaV2PreTrainedModel):
+ def __init__(self, config):
+ super().__init__(config)
+ self.num_labels = config.num_labels
+ self.deberta = DebertaV2Model(config)
+ self.dropout = torch.nn.Dropout(config.hidden_dropout_prob)
+ self.classifier = torch.nn.Linear(config.hidden_size, config.num_labels)
+ self.init_weights()
+
+ for param in self.deberta.parameters():
+ param.requires_grad = False
+
+ self.pre_seq_len = config.pre_seq_len
+ self.n_layer = config.num_hidden_layers
+ self.n_head = config.num_attention_heads
+ self.n_embd = config.hidden_size // config.num_attention_heads
+
+ self.prefix_tokens = torch.arange(self.pre_seq_len).long()
+ self.prefix_encoder = PrefixEncoder(config)
+
+ deberta_param = 0
+ for name, param in self.deberta.named_parameters():
+ deberta_param += param.numel()
+ all_param = 0
+ for name, param in self.named_parameters():
+ all_param += param.numel()
+ total_param = all_param - deberta_param
+ print('total param is {}'.format(total_param)) # 9860105
+
+ def get_prompt(self, batch_size):
+ prefix_tokens = self.prefix_tokens.unsqueeze(0).expand(batch_size, -1).to(self.deberta.device)
+ past_key_values = self.prefix_encoder(prefix_tokens)
+ past_key_values = past_key_values.view(
+ batch_size,
+ self.pre_seq_len,
+ self.n_layer * 2,
+ self.n_head,
+ self.n_embd
+ )
+ past_key_values = self.dropout(past_key_values)
+ past_key_values = past_key_values.permute([2, 0, 3, 1, 4]).split(2)
+ return past_key_values
+
+ def forward(
+ self,
+ input_ids=None,
+ attention_mask=None,
+ token_type_ids=None,
+ position_ids=None,
+ head_mask=None,
+ inputs_embeds=None,
+ labels=None,
+ output_attentions=None,
+ output_hidden_states=None,
+ return_dict=None,
+ ):
+ return_dict = return_dict if return_dict is not None else self.config.use_return_dict
+
+ batch_size = input_ids.shape[0]
+ past_key_values = self.get_prompt(batch_size=batch_size)
+ prefix_attention_mask = torch.ones(batch_size, self.pre_seq_len).to(self.deberta.device)
+ attention_mask = torch.cat((prefix_attention_mask, attention_mask), dim=1)
+
+ outputs = self.deberta(
+ input_ids,
+ attention_mask=attention_mask,
+ token_type_ids=token_type_ids,
+ position_ids=position_ids,
+ inputs_embeds=inputs_embeds,
+ output_attentions=output_attentions,
+ output_hidden_states=output_hidden_states,
+ return_dict=return_dict,
+ past_key_values=past_key_values,
+ )
+
+ sequence_output = outputs[0]
+ sequence_output = self.dropout(sequence_output)
+ logits = self.classifier(sequence_output)
+ attention_mask = attention_mask[:,self.pre_seq_len:].contiguous()
+
+ loss = None
+ if labels is not None:
+ loss_fct = CrossEntropyLoss()
+ # Only keep active parts of the loss
+ if attention_mask is not None:
+ active_loss = attention_mask.view(-1) == 1
+ active_logits = logits.view(-1, self.num_labels)
+ active_labels = torch.where(
+ active_loss, labels.view(-1), torch.tensor(loss_fct.ignore_index).type_as(labels)
+ )
+ loss = loss_fct(active_logits, active_labels)
+ else:
+ loss = loss_fct(logits.view(-1, self.num_labels), labels.view(-1))
+
+ if not return_dict:
+ output = (logits,) + outputs[2:]
+ return ((loss,) + output) if loss is not None else output
+
+ return TokenClassifierOutput(
+ loss=loss,
+ logits=logits,
+ hidden_states=outputs.hidden_states,
+ attentions=outputs.attentions,
+ )
\ No newline at end of file
diff --git a/model/loss.py b/model/loss.py
new file mode 100644
index 0000000..fab9101
--- /dev/null
+++ b/model/loss.py
@@ -0,0 +1,344 @@
+# coding=utf-8
+# Copyright (c) Microsoft. All rights reserved.
+
+import torch
+from torch.nn.modules.loss import _Loss
+import torch.nn.functional as F
+import torch.nn as nn
+from enum import IntEnum
+
+def stable_kl(logit, target, epsilon=1e-6, reduce=True):
+ logit = logit.view(-1, logit.size(-1)).float()
+ target = target.view(-1, target.size(-1)).float()
+ bs = logit.size(0)
+ p = F.log_softmax(logit, 1).exp()
+ y = F.log_softmax(target, 1).exp()
+ rp = -(1.0/(p + epsilon) -1 + epsilon).detach().log()
+ ry = -(1.0/(y + epsilon) -1 + epsilon).detach().log()
+ if reduce:
+ return (p* (rp- ry) * 2).sum() / bs
+ else:
+ return (p* (rp- ry) * 2).sum()
+
+def entropy(logit, epsilon=1e-6, reduce=True):
+ logit = logit.view(-1, logit.size(-1)).float()
+
+ bs = logit.size(0)
+ p = F.log_softmax(logit, 1).exp()
+ log_p = p.log()
+ loss = - p * log_p
+
+ if reduce:
+ return loss.sum() / bs
+ else:
+ return loss.sum()
+
+class Criterion(_Loss):
+ def __init__(self, alpha=1.0, name='criterion'):
+ super().__init__()
+ """Alpha is used to weight each loss term
+ """
+ self.alpha = alpha
+ self.name = name
+
+ def forward(self, input, target, weight=None, ignore_index=-1):
+ """weight: sample weight
+ """
+ return
+
+class CeCriterion(Criterion):
+ def __init__(self, alpha=1.0, name='Cross Entropy Criterion'):
+ super().__init__()
+ self.alpha = alpha
+ self.name = name
+
+ def forward(self, input, target, weight=None, ignore_index=-1):
+ """weight: sample weight
+ """
+ if weight:
+ loss = torch.mean(F.cross_entropy(input, target, reduce=False, ignore_index=ignore_index) * weight)
+ else:
+ loss = F.cross_entropy(input, target, ignore_index=ignore_index)
+ loss = loss * self.alpha
+ return loss
+
+
+class SeqCeCriterion(CeCriterion):
+ def __init__(self, alpha=1.0, name='Seq Cross Entropy Criterion'):
+ super().__init__(alpha, name)
+
+ def forward(self, input, target, weight=None, ignore_index=-1):
+ target = target.view(-1)
+ if weight:
+ loss = torch.mean(F.cross_entropy(input, target, reduce=False, ignore_index=ignore_index) * weight)
+ else:
+ loss = F.cross_entropy(input, target, ignore_index=ignore_index)
+ loss = loss * self.alpha
+ return loss
+
+class MseCriterion(Criterion):
+ def __init__(self, alpha=1.0, name='MSE Regression Criterion'):
+ super().__init__()
+ self.alpha = alpha
+ self.name = name
+
+ def forward(self, input, target, weight=None, ignore_index=-1):
+ """weight: sample weight
+ """
+ if weight:
+ loss = torch.mean(F.mse_loss(input.squeeze(), target, reduce=False) *
+ weight.reshape((target.shape[0], 1)))
+ else:
+ loss = F.mse_loss(input.squeeze(), target)
+ loss = loss * self.alpha
+ return loss
+
+class KlCriterion(Criterion):
+ def __init__(self, alpha=1.0, name='KL Div Criterion'):
+ super().__init__()
+ self.alpha = alpha
+ self.name = name
+
+ def forward(self, input, target, weight=None, ignore_index=-1, target_soft=False):
+ """input/target: logits
+ """
+ input = input.float()
+ target = target.float()
+ if not target_soft:
+ loss = F.kl_div(F.log_softmax(input, dim=-1, dtype=torch.float32), F.softmax(target, dim=-1, dtype=torch.float32), reduction='batchmean')
+ else:
+ loss = F.kl_div(F.log_softmax(input, dim=-1, dtype=torch.float32),
+ target, reduction='batchmean')
+ loss = loss * self.alpha
+ return loss
+
+class NsKlCriterion(Criterion):
+ def __init__(self, alpha=1.0, name='KL Div Criterion'):
+ super().__init__()
+ self.alpha = alpha
+ self.name = name
+
+ def forward(self, input, target, weight=None, ignore_index=-1):
+ """input/target: logits
+ """
+ input = input.float()
+ target = target.float()
+ loss = stable_kl(input, target.detach())
+ loss = loss * self.alpha
+ return loss
+
+
+class SymKlCriterion(Criterion):
+ def __init__(self, alpha=1.0, name='KL Div Criterion'):
+ super().__init__()
+ self.alpha = alpha
+ self.name = name
+
+ def forward(self, input, target, weight=None, ignore_index=-1, reduction='batchmean'):
+ """input/target: logits
+ """
+ input = input.float()
+ target = target.float()
+ loss = F.kl_div(F.log_softmax(input, dim=-1, dtype=torch.float32), F.softmax(target.detach(), dim=-1, dtype=torch.float32), reduction=reduction) + \
+ F.kl_div(F.log_softmax(target, dim=-1, dtype=torch.float32), F.softmax(input.detach(), dim=-1, dtype=torch.float32), reduction=reduction)
+ loss = loss * self.alpha
+ return loss
+
+class NsSymKlCriterion(Criterion):
+ def __init__(self, alpha=1.0, name='KL Div Criterion'):
+ super().__init__()
+ self.alpha = alpha
+ self.name = name
+
+ def forward(self, input, target, weight=None, ignore_index=-1):
+ """input/target: logits
+ """
+ input = input.float()
+ target = target.float()
+ loss = stable_kl(input, target.detach()) + \
+ stable_kl(target, input.detach())
+ loss = loss * self.alpha
+ return loss
+
+class JSCriterion(Criterion):
+ def __init__(self, alpha=1.0, name='JS Div Criterion'):
+ super().__init__()
+ self.alpha = alpha
+ self.name = name
+
+ def forward(self, input, target, weight=None, ignore_index=-1, reduction='batchmean'):
+ """input/target: logits
+ """
+ input = input.float()
+ target = target.float()
+ m = F.softmax(target.detach(), dim=-1, dtype=torch.float32) + \
+ F.softmax(input.detach(), dim=-1, dtype=torch.float32)
+ m = 0.5 * m
+ loss = F.kl_div(F.log_softmax(input, dim=-1, dtype=torch.float32), m, reduction=reduction) + \
+ F.kl_div(F.log_softmax(target, dim=-1, dtype=torch.float32), m, reduction=reduction)
+ loss = loss * self.alpha
+ return loss
+
+class HLCriterion(Criterion):
+ def __init__(self, alpha=1.0, name='Hellinger Criterion'):
+ super().__init__()
+ self.alpha = alpha
+ self.name = name
+
+ def forward(self, input, target, weight=None, ignore_index=-1, reduction='batchmean'):
+ """input/target: logits
+ """
+ input = input.float()
+ target = target.float()
+ si = F.softmax(target.detach(), dim=-1, dtype=torch.float32).sqrt_()
+ st = F.softmax(input.detach(), dim=-1, dtype=torch.float32).sqrt_()
+ loss = F.mse_loss(si, st)
+ loss = loss * self.alpha
+ return loss
+
+
+class RankCeCriterion(Criterion):
+ def __init__(self, alpha=1.0, name='Cross Entropy Criterion'):
+ super().__init__()
+ self.alpha = alpha
+ self.name = name
+
+ def forward(self, input, target, weight=None, ignore_index=-1, pairwise_size=1):
+ input = input.view(-1, pairwise_size)
+ target = target.contiguous().view(-1, pairwise_size)[:, 0]
+ if weight:
+ loss = torch.mean(F.cross_entropy(input, target, reduce=False, ignore_index=ignore_index) * weight)
+ else:
+ loss = F.cross_entropy(input, target, ignore_index=ignore_index)
+ loss = loss * self.alpha
+ return loss
+
+class SpanCeCriterion(Criterion):
+ def __init__(self, alpha=1.0, name='Span Cross Entropy Criterion'):
+ super().__init__()
+ """This is for extractive MRC, e.g., SQuAD, ReCoRD ... etc
+ """
+ self.alpha = alpha
+ self.name = name
+
+ def forward(self, input, target, weight=None, ignore_index=-1):
+ """weight: sample weight
+ """
+ assert len(input) == 2
+ start_input, end_input = input
+ start_target, end_target = target
+ if weight:
+ b = torch.mean(F.cross_entropy(start_input, start_target, reduce=False, ignore_index=ignore_index) * weight)
+ e = torch.mean(F.cross_entropy(end_input, end_target, reduce=False, ignore_index=ignore_index) * weight)
+ else:
+ b = F.cross_entropy(start_input, start_target, ignore_index=ignore_index)
+ e = F.cross_entropy(end_input, end_target, ignore_index=ignore_index)
+ loss = 0.5 * (b + e) * self.alpha
+ return loss
+
+class MlmCriterion(Criterion):
+ def __init__(self, alpha=1.0, name='BERT pre-train Criterion'):
+ super().__init__()
+ self.alpha = alpha
+ self.name = name
+
+ def forward(self, input, target, weight=None, ignore_index=-1):
+ """TODO: support sample weight, xiaodl
+ """
+ mlm_y, y = target
+ mlm_p, nsp_p = input
+ mlm_p = mlm_p.view(-1, mlm_p.size(-1))
+ mlm_y = mlm_y.view(-1)
+ mlm_loss = F.cross_entropy(mlm_p, mlm_y, ignore_index=ignore_index)
+ nsp_loss = F.cross_entropy(nsp_p, y)
+ loss = mlm_loss + nsp_loss
+ loss = loss * self.alpha
+ return loss
+
+class ContrastiveLoss(nn.Module):
+ """
+ Contrastive loss function.
+ Based on:
+ """
+
+ def __init__(self, margin=1.0, metric = 'cos'):
+ super(ContrastiveLoss, self).__init__()
+ self.margin = margin
+ self.metric = metric
+ self.sim = nn.CosineSimilarity(dim=-1)
+ self.loss = torch.nn.BCEWithLogitsLoss()
+ # print('ContrastiveLoss, Metric:', self.metric)
+
+ def check_type_forward(self, in_types):
+ assert len(in_types) == 3
+
+ x0_type, x1_type, y_type = in_types
+ assert x0_type.size() == x1_type.shape
+ assert x1_type.size()[0] == y_type.shape[0]
+ assert x1_type.size()[0] > 0
+ assert x0_type.dim() == 2
+ assert x1_type.dim() == 2
+ assert y_type.dim() == 1
+
+ def get_loss(self, x0, x1, y):
+ # import pdb
+ # pdb.set_trace()
+ #elf.check_type_forward((x0, x1, y))
+
+ # euclidian distance
+ if self.metric == 'l2':
+ diff = x0 - x1
+ dist_sq = torch.sum(torch.pow(diff, 2), 1) / x0.shape[-1]
+ dist = torch.sqrt(dist_sq)
+ elif self.metric == 'cos':
+ sim = self.sim(x0, x1) / 0.1
+ #print(x0, x1, torch.sum(torch.pow(x0-x1, 2), 1) / x0.shape[-1], dist, dist_sq)
+ else:
+ print("Error Loss Metric!!")
+ return 0
+ #dist = torch.sum( - x0 * x1 / np.sqrt(x0.shape[-1]), 1).exp()
+ #dist_sq = dist ** 2
+
+ # mdist = self.margin - dist
+ # dist = torch.clamp(mdist, min=0.0)
+ # loss = y * dist_sq + (1 - y) * torch.pow(dist, 2)
+ # loss = torch.sum(loss) / 2.0 / x0.size()[0]
+ loss = self.loss(sim, y)
+ return loss#, dist_sq, dist
+
+ def forward(self, x0, x1, y):
+ loss1 = self.get_loss(x0, x1.detach(), y)
+ loss2 = self.get_loss(x1.detach(), x0, y)
+ return (loss1 + loss2) /2
+
+
+
+class LossCriterion(IntEnum):
+ CeCriterion = 0
+ MseCriterion = 1
+ RankCeCriterion = 2
+ SpanCeCriterion = 3
+ SeqCeCriterion = 4
+ MlmCriterion = 5
+ KlCriterion = 6
+ SymKlCriterion = 7
+ NsKlCriterion = 8
+ NsSymKlCriterion = 9
+ JSCriterion = 10
+ HLCriterion = 11
+
+
+LOSS_REGISTRY = {
+ LossCriterion.CeCriterion: CeCriterion,
+ LossCriterion.MseCriterion: MseCriterion,
+ LossCriterion.RankCeCriterion: RankCeCriterion,
+ LossCriterion.SpanCeCriterion: SpanCeCriterion,
+ LossCriterion.SeqCeCriterion: SeqCeCriterion,
+ LossCriterion.MlmCriterion: MlmCriterion,
+ LossCriterion.KlCriterion: KlCriterion,
+ LossCriterion.SymKlCriterion: SymKlCriterion,
+ LossCriterion.NsKlCriterion: NsKlCriterion,
+ LossCriterion.NsSymKlCriterion: NsSymKlCriterion,
+ LossCriterion.JSCriterion: JSCriterion,
+ LossCriterion.HLCriterion: HLCriterion,
+}
diff --git a/model/model_adaptation.py b/model/model_adaptation.py
new file mode 100644
index 0000000..37adde3
--- /dev/null
+++ b/model/model_adaptation.py
@@ -0,0 +1,1070 @@
+"""Custom models for few-shot learning specific operations."""
+
+import torch
+import torch.nn as nn
+import transformers
+import torch.nn.functional as F
+from transformers import AutoConfig, AutoModelForSequenceClassification, AutoTokenizer, EvalPrediction
+from transformers.models.bert.modeling_bert import BertPreTrainedModel, BertForSequenceClassification, BertModel, \
+ BertOnlyMLMHead
+from transformers.models.roberta.modeling_roberta import *
+from transformers.models.bert.modeling_bert import *
+from transformers.models.deberta_v2.modeling_deberta_v2 import DebertaV2PreTrainedModel, DebertaV2Model, StableDropout, \
+ ContextPooler, DebertaV2OnlyMLMHead
+from transformers.models.deberta.modeling_deberta import DebertaPreTrainedModel, DebertaModel, StableDropout, \
+ ContextPooler, DebertaOnlyMLMHead
+from transformers.modeling_outputs import SequenceClassifierOutput
+from transformers.modeling_utils import PreTrainedModel
+# from loss import stable_kl, CeCriterion, KlCriterion, entropy, SymKlCriterion, ContrastiveLoss
+# from processors import processors_mapping, num_labels_mapping, output_modes_mapping, compute_metrics_mapping, \
+# bound_mapping
+import logging
+
+logger = logging.getLogger(__name__)
+
+# adapter_choice: LiST, houlsby, lora
+
+# add by wjn
+def init_adapter(model, std=0.0002):
+ with torch.no_grad():
+ for name, param in model.named_parameters():
+ init_value = 0
+ if 'adapter_proj' in name:
+ # if self.model_args.adapter_choice == 'simple':
+ # init_value = torch.eye(param.size(0))
+ if std > 0:
+ init_value += torch.normal(0, std, size=param.size())
+ param.copy_(init_value)
+ return model
+
+# Adapter Layer
+class AdapeterLayer(nn.Module):
+ def __init__(self, n_in, n_out=None, adapter_dim=128, adapter_choice='LiST'):
+ super(AdapeterLayer, self).__init__()
+ if not n_out:
+ n_out = n_in
+
+ self.adapter_choice = adapter_choice
+ self.act_fun = None
+
+ if self.adapter_choice == 'LiST':
+
+ self.adapter_dim = adapter_dim
+ # print("self.adapter_dim=", self.adapter_dim) # 128
+ self.adapter_proj_1 = nn.Linear(n_out, adapter_dim, bias=False)
+ nn.init.normal_(self.adapter_proj_1.weight, std=0.02)
+ self.adapter_proj_2 = nn.Linear(adapter_dim, n_out, bias=False)
+ nn.init.normal_(self.adapter_proj_2.weight, std=0.02)
+ elif self.adapter_choice == 'houlsby':
+
+ self.adapter_dim = adapter_dim
+ self.adapter_proj_1 = nn.Linear(n_out, adapter_dim, bias=False)
+ nn.init.normal_(self.adapter_proj_1.weight, std=0.02)
+ self.act_fun = torch.nn.ReLU()
+ self.adapter_proj_2 = nn.Linear(adapter_dim, n_out, bias=False)
+ nn.init.normal_(self.adapter_proj_2.weight, std=0.02)
+ else:
+
+ self.adapter_dim = adapter_dim
+ self.adapter_proj_1 = nn.Linear(n_out, n_out, bias=False)
+
+
+ def forward(self, x):
+ if self.adapter_choice == 'LiST':
+ result = torch.matmul(x, self.adapter_proj_1.weight.type_as(x).T)
+ result = torch.matmul(result, self.adapter_proj_2.weight.type_as(x).T)
+ return result + x
+ elif self.adapter_choice == 'houlsby':
+ result = torch.matmul(x, self.adapter_proj_1.weight.type_as(x).T)
+ if self.act_fun is not None:
+ result = self.act_fun(result)
+ result = torch.matmul(result, self.adapter_proj_2.weight.type_as(x).T)
+ return result + x
+
+ else:
+ result = torch.matmul(x, self.adapter_proj_1.weight.type_as(x).T)
+ return result
+
+
+## ======== Adapter For RoBERTa ========
+
+class RobertaAdaOutput(nn.Module):
+ def __init__(self, config):
+ super().__init__()
+ self.dense = nn.Linear(config.intermediate_size, config.hidden_size)
+ self.config = config
+ self.adaptation_layer = AdapeterLayer(n_in=config.intermediate_size, n_out=config.hidden_size,
+ adapter_dim=config.adapter_dim, adapter_choice=config.adapter_choice)
+ self.LayerNorm = nn.LayerNorm(config.hidden_size, eps=config.layer_norm_eps)
+ self.dropout = nn.Dropout(config.hidden_dropout_prob)
+
+ def forward(self, hidden_states, input_tensor):
+
+ hidden_states = self.dense(hidden_states)
+ hidden_states = self.adaptation_layer(hidden_states)
+
+
+ hidden_states = self.dropout(hidden_states)
+ hidden_states = self.LayerNorm(hidden_states + input_tensor)
+ # hidden_states = self.adaptation_layer_skip(hidden_states)
+ return hidden_states
+
+
+# Copied from transformers.models.bert.modeling_bert.BertSelfOutput
+class RobertaAdaSelfOutput(nn.Module):
+ def __init__(self, config):
+ super().__init__()
+ self.config = config
+ self.dense = nn.Linear(config.hidden_size, config.hidden_size)
+ self.adaptation_layer = AdapeterLayer(n_in=config.intermediate_size, n_out=config.hidden_size,
+ adapter_dim=config.adapter_dim, adapter_choice=config.adapter_choice)
+ self.LayerNorm = nn.LayerNorm(config.hidden_size, eps=config.layer_norm_eps)
+ self.dropout = nn.Dropout(config.hidden_dropout_prob)
+
+ def forward(self, hidden_states, input_tensor):
+
+ hidden_states = self.dense(hidden_states)
+ hidden_states = self.adaptation_layer(hidden_states)
+ hidden_states = self.dropout(hidden_states)
+ hidden_states = self.LayerNorm(hidden_states + input_tensor)
+ # input_tensor = hidden_states
+ # hidden_states = self.adaptation_layer(hidden_states)
+ # hidden_states = self.LayerNorm(hidden_states + input_tensor)
+ return hidden_states
+
+
+# Copied from transformers.models.bert.modeling_bert.BertAttention with Bert->Roberta
+class RobertaAdaAttention(nn.Module):
+ def __init__(self, config):
+ super().__init__()
+ self.self = RobertaSelfAttention(config)
+ self.output = RobertaAdaSelfOutput(config)
+ self.pruned_heads = set()
+
+ def prune_heads(self, heads):
+ if len(heads) == 0:
+ return
+ heads, index = find_pruneable_heads_and_indices(
+ heads, self.self.num_attention_heads, self.self.attention_head_size, self.pruned_heads
+ )
+
+ # Prune linear layers
+ self.self.query = prune_linear_layer(self.self.query, index)
+ self.self.key = prune_linear_layer(self.self.key, index)
+ self.self.value = prune_linear_layer(self.self.value, index)
+ self.output.dense = prune_linear_layer(self.output.dense, index, dim=1)
+
+ # Update hyper params and store pruned heads
+ self.self.num_attention_heads = self.self.num_attention_heads - len(heads)
+ self.self.all_head_size = self.self.attention_head_size * self.self.num_attention_heads
+ self.pruned_heads = self.pruned_heads.union(heads)
+
+ def forward(
+ self,
+ hidden_states,
+ attention_mask=None,
+ head_mask=None,
+ encoder_hidden_states=None,
+ encoder_attention_mask=None,
+ past_key_value=None,
+ output_attentions=False,
+ ):
+ self_outputs = self.self(
+ hidden_states,
+ attention_mask,
+ head_mask,
+ encoder_hidden_states,
+ encoder_attention_mask,
+ past_key_value,
+ output_attentions,
+ )
+ attention_output = self.output(self_outputs[0], hidden_states)
+ outputs = (attention_output,) + self_outputs[1:] # add attentions if we output them
+ return outputs
+
+
+# Copied from transformers.models.bert.modeling_bert.BertLayer with Bert->Roberta
+class RobertaAdaLayer(nn.Module):
+ def __init__(self, config):
+ super().__init__()
+ self.chunk_size_feed_forward = config.chunk_size_feed_forward
+ self.seq_len_dim = 1
+ self.attention = RobertaAdaAttention(config)
+ self.is_decoder = config.is_decoder
+ self.add_cross_attention = config.add_cross_attention
+ if self.add_cross_attention:
+ assert self.is_decoder, f"{self} should be used as a decoder model if cross attention is added"
+ self.crossattention = RobertaAttention(config)
+ self.intermediate = RobertaIntermediate(config)
+ self.output = RobertaAdaOutput(config)
+
+ def forward(
+ self,
+ hidden_states,
+ attention_mask=None,
+ head_mask=None,
+ encoder_hidden_states=None,
+ encoder_attention_mask=None,
+ past_key_value=None,
+ output_attentions=False,
+ ):
+ # decoder uni-directional self-attention cached key/values tuple is at positions 1,2
+ self_attn_past_key_value = past_key_value[:2] if past_key_value is not None else None
+ self_attention_outputs = self.attention(
+ hidden_states,
+ attention_mask,
+ head_mask,
+ output_attentions=output_attentions,
+ past_key_value=self_attn_past_key_value,
+ )
+ attention_output = self_attention_outputs[0]
+
+ # if decoder, the last output is tuple of self-attn cache
+ if self.is_decoder:
+ outputs = self_attention_outputs[1:-1]
+ present_key_value = self_attention_outputs[-1]
+ else:
+ outputs = self_attention_outputs[1:] # add self attentions if we output attention weights
+
+ cross_attn_present_key_value = None
+ if self.is_decoder and encoder_hidden_states is not None:
+ assert hasattr(
+ self, "crossattention"
+ ), f"If `encoder_hidden_states` are passed, {self} has to be instantiated with cross-attention layers by setting `config.add_cross_attention=True`"
+
+ # cross_attn cached key/values tuple is at positions 3,4 of past_key_value tuple
+ cross_attn_past_key_value = past_key_value[-2:] if past_key_value is not None else None
+ cross_attention_outputs = self.crossattention(
+ attention_output,
+ attention_mask,
+ head_mask,
+ encoder_hidden_states,
+ encoder_attention_mask,
+ cross_attn_past_key_value,
+ output_attentions,
+ )
+ attention_output = cross_attention_outputs[0]
+ outputs = outputs + cross_attention_outputs[1:-1] # add cross attentions if we output attention weights
+
+ # add cross-attn cache to positions 3,4 of present_key_value tuple
+ cross_attn_present_key_value = cross_attention_outputs[-1]
+ present_key_value = present_key_value + cross_attn_present_key_value
+
+ layer_output = apply_chunking_to_forward(
+ self.feed_forward_chunk, self.chunk_size_feed_forward, self.seq_len_dim, attention_output
+ )
+ outputs = (layer_output,) + outputs
+
+ # if decoder, return the attn key/values as the last output
+ if self.is_decoder:
+ outputs = outputs + (present_key_value,)
+
+ return outputs
+
+ def feed_forward_chunk(self, attention_output):
+ intermediate_output = self.intermediate(attention_output)
+ layer_output = self.output(intermediate_output, attention_output)
+ return layer_output
+
+
+# Copied from transformers.models.bert.modeling_bert.BertEncoder with Bert->Roberta
+class RobertaAdaEncoder(nn.Module):
+ def __init__(self, config):
+ super().__init__()
+ self.config = config
+ self.layer = nn.ModuleList([RobertaAdaLayer(config) for _ in range(config.num_hidden_layers)])
+ self.skip = 2
+
+ def learn_init(
+ self,
+ hidden_states,
+ attention_mask=None,
+ head_mask=None,
+ encoder_hidden_states=None,
+ encoder_attention_mask=None,
+ past_key_values=None,
+ use_cache=None,
+ output_attentions=False,
+ output_hidden_states=False,
+ return_dict=True):
+
+
+ all_hidden_states = () if output_hidden_states else None
+ all_self_attentions = () if output_attentions else None
+ all_cross_attentions = () if output_attentions and self.config.add_cross_attention else None
+
+ next_decoder_cache = () if use_cache else None
+ self.skip_list = []
+ for i, layer_module in enumerate(self.layer):
+ # if i+1 % self.skip
+
+ if output_hidden_states:
+ all_hidden_states = all_hidden_states + (hidden_states,)
+
+ layer_head_mask = head_mask[i] if head_mask is not None else None
+ past_key_value = past_key_values[i] if past_key_values is not None else None
+
+ if getattr(self.config, "gradient_checkpointing", False) and self.training:
+
+ if use_cache:
+ logger.warning(
+ "`use_cache=True` is incompatible with `config.gradient_checkpointing=True`. Setting "
+ "`use_cache=False`..."
+ )
+ use_cache = False
+
+ def create_custom_forward(module):
+ def custom_forward(*inputs):
+ return module(*inputs, past_key_value, output_attentions)
+
+ return custom_forward
+
+ layer_outputs = torch.utils.checkpoint.checkpoint(
+ create_custom_forward(layer_module),
+ hidden_states,
+ attention_mask,
+ layer_head_mask,
+ encoder_hidden_states,
+ encoder_attention_mask,
+ )
+ else:
+ layer_outputs = layer_module(
+ hidden_states,
+ attention_mask,
+ layer_head_mask,
+ encoder_hidden_states,
+ encoder_attention_mask,
+ past_key_value,
+ output_attentions,
+ )
+
+ hidden_states = layer_outputs[0]
+ if use_cache:
+ next_decoder_cache += (layer_outputs[-1],)
+ if output_attentions:
+ all_self_attentions = all_self_attentions + (layer_outputs[1],)
+ if self.config.add_cross_attention:
+ all_cross_attentions = all_cross_attentions + (layer_outputs[2],)
+
+ if output_hidden_states:
+ all_hidden_states = all_hidden_states + (hidden_states,)
+
+ if not return_dict:
+ return tuple(
+ v
+ for v in [
+ hidden_states,
+ next_decoder_cache,
+ all_hidden_states,
+ all_self_attentions,
+ all_cross_attentions,
+ ]
+ if v is not None
+ )
+ return BaseModelOutputWithPastAndCrossAttentions(
+ last_hidden_state=hidden_states,
+ past_key_values=next_decoder_cache,
+ hidden_states=all_hidden_states,
+ attentions=all_self_attentions,
+ cross_attentions=all_cross_attentions,
+ )
+
+ def forward(
+ self,
+ hidden_states,
+ attention_mask=None,
+ head_mask=None,
+ encoder_hidden_states=None,
+ encoder_attention_mask=None,
+ past_key_values=None,
+ use_cache=None,
+ output_attentions=False,
+ output_hidden_states=False,
+ return_dict=True,
+ ):
+ all_hidden_states = () if output_hidden_states else None
+ all_self_attentions = () if output_attentions else None
+ all_cross_attentions = () if output_attentions and self.config.add_cross_attention else None
+
+ next_decoder_cache = () if use_cache else None
+ for i, layer_module in enumerate(self.layer):
+ # if (i+1) % 3 == 0:
+ # continue
+ if output_hidden_states:
+ all_hidden_states = all_hidden_states + (hidden_states,)
+
+ layer_head_mask = head_mask[i] if head_mask is not None else None
+ past_key_value = past_key_values[i] if past_key_values is not None else None
+
+ if getattr(self.config, "gradient_checkpointing", False) and self.training:
+
+ if use_cache:
+ logger.warning(
+ "`use_cache=True` is incompatible with `config.gradient_checkpointing=True`. Setting "
+ "`use_cache=False`..."
+ )
+ use_cache = False
+
+ def create_custom_forward(module):
+ def custom_forward(*inputs):
+ return module(*inputs, past_key_value, output_attentions)
+
+ return custom_forward
+
+ layer_outputs = torch.utils.checkpoint.checkpoint(
+ create_custom_forward(layer_module),
+ hidden_states,
+ attention_mask,
+ layer_head_mask,
+ encoder_hidden_states,
+ encoder_attention_mask,
+ )
+ else:
+ layer_outputs = layer_module(
+ hidden_states,
+ attention_mask,
+ layer_head_mask,
+ encoder_hidden_states,
+ encoder_attention_mask,
+ past_key_value,
+ output_attentions,
+ )
+
+ hidden_states = layer_outputs[0]
+ if use_cache:
+ next_decoder_cache += (layer_outputs[-1],)
+ if output_attentions:
+ all_self_attentions = all_self_attentions + (layer_outputs[1],)
+ if self.config.add_cross_attention:
+ all_cross_attentions = all_cross_attentions + (layer_outputs[2],)
+
+ if output_hidden_states:
+ all_hidden_states = all_hidden_states + (hidden_states,)
+
+ if not return_dict:
+ return tuple(
+ v
+ for v in [
+ hidden_states,
+ next_decoder_cache,
+ all_hidden_states,
+ all_self_attentions,
+ all_cross_attentions,
+ ]
+ if v is not None
+ )
+ return BaseModelOutputWithPastAndCrossAttentions(
+ last_hidden_state=hidden_states,
+ past_key_values=next_decoder_cache,
+ hidden_states=all_hidden_states,
+ attentions=all_self_attentions,
+ cross_attentions=all_cross_attentions,
+ )
+
+class RobertaAdaModel(RobertaPreTrainedModel):
+ """
+ The model can behave as an encoder (with only self-attention) as well as a decoder, in which case a layer of
+ cross-attention is added between the self-attention layers, following the architecture described in `Attention is
+ all you need`_ by Ashish Vaswani, Noam Shazeer, Niki Parmar, Jakob Uszkoreit, Llion Jones, Aidan N. Gomez, Lukasz
+ Kaiser and Illia Polosukhin.
+ To behave as an decoder the model needs to be initialized with the :obj:`is_decoder` argument of the configuration
+ set to :obj:`True`. To be used in a Seq2Seq model, the model needs to initialized with both :obj:`is_decoder`
+ argument and :obj:`add_cross_attention` set to :obj:`True`; an :obj:`encoder_hidden_states` is then expected as an
+ input to the forward pass.
+ .. _`Attention is all you need`: https://arxiv.org/abs/1706.03762
+ """
+
+ _keys_to_ignore_on_load_missing = [r"position_ids"]
+
+ # Copied from transformers.models.bert.modeling_bert.BertModel.__init__ with Bert->Roberta
+ def __init__(self, config, add_pooling_layer=True):
+ super().__init__(config)
+ self.config = config
+
+ self.embeddings = RobertaEmbeddings(config)
+ self.encoder = RobertaAdaEncoder(config)
+
+
+ self.pooler = RobertaPooler(config) if add_pooling_layer else None
+
+ #self.init_weights()
+
+ def get_input_embeddings(self):
+ return self.embeddings.word_embeddings
+
+ def set_input_embeddings(self, value):
+ self.embeddings.word_embeddings = value
+
+ def _prune_heads(self, heads_to_prune):
+ """
+ Prunes heads of the model. heads_to_prune: dict of {layer_num: list of heads to prune in this layer} See base
+ class PreTrainedModel
+ """
+ for layer, heads in heads_to_prune.items():
+ self.encoder.layer[layer].attention.prune_heads(heads)
+
+ # Copied from transformers.models.bert.modeling_bert.BertModel.forward
+ def forward(
+ self,
+ input_ids=None,
+ attention_mask=None,
+ token_type_ids=None,
+ position_ids=None,
+ head_mask=None,
+ inputs_embeds=None,
+ encoder_hidden_states=None,
+ encoder_attention_mask=None,
+ past_key_values=None,
+ use_cache=None,
+ output_attentions=None,
+ output_hidden_states=None,
+ return_dict=None,
+ ):
+ r"""
+ encoder_hidden_states (:obj:`torch.FloatTensor` of shape :obj:`(batch_size, sequence_length, hidden_size)`, `optional`):
+ Sequence of hidden-states at the output of the last layer of the encoder. Used in the cross-attention if
+ the model is configured as a decoder.
+ encoder_attention_mask (:obj:`torch.FloatTensor` of shape :obj:`(batch_size, sequence_length)`, `optional`):
+ Mask to avoid performing attention on the padding token indices of the encoder input. This mask is used in
+ the cross-attention if the model is configured as a decoder. Mask values selected in ``[0, 1]``:
+ - 1 for tokens that are **not masked**,
+ - 0 for tokens that are **masked**.
+ past_key_values (:obj:`tuple(tuple(torch.FloatTensor))` of length :obj:`config.n_layers` with each tuple having 4 tensors of shape :obj:`(batch_size, num_heads, sequence_length - 1, embed_size_per_head)`):
+ Contains precomputed key and value hidden states of the attention blocks. Can be used to speed up decoding.
+ If :obj:`past_key_values` are used, the user can optionally input only the last :obj:`decoder_input_ids`
+ (those that don't have their past key value states given to this model) of shape :obj:`(batch_size, 1)`
+ instead of all :obj:`decoder_input_ids` of shape :obj:`(batch_size, sequence_length)`.
+ use_cache (:obj:`bool`, `optional`):
+ If set to :obj:`True`, :obj:`past_key_values` key value states are returned and can be used to speed up
+ decoding (see :obj:`past_key_values`).
+ """
+ output_attentions = output_attentions if output_attentions is not None else self.config.output_attentions
+ output_hidden_states = (
+ output_hidden_states if output_hidden_states is not None else self.config.output_hidden_states
+ )
+ return_dict = return_dict if return_dict is not None else self.config.use_return_dict
+
+ if self.config.is_decoder:
+ use_cache = use_cache if use_cache is not None else self.config.use_cache
+ else:
+ use_cache = False
+
+ if input_ids is not None and inputs_embeds is not None:
+ raise ValueError("You cannot specify both input_ids and inputs_embeds at the same time")
+ elif input_ids is not None:
+ input_shape = input_ids.size()
+ batch_size, seq_length = input_shape
+ elif inputs_embeds is not None:
+ input_shape = inputs_embeds.size()[:-1]
+ batch_size, seq_length = input_shape
+ else:
+ raise ValueError("You have to specify either input_ids or inputs_embeds")
+
+ device = input_ids.device if input_ids is not None else inputs_embeds.device
+
+ # past_key_values_length
+ past_key_values_length = past_key_values[0][0].shape[2] if past_key_values is not None else 0
+
+ if attention_mask is None:
+ attention_mask = torch.ones(((batch_size, seq_length + past_key_values_length)), device=device)
+
+ if token_type_ids is None:
+ if hasattr(self.embeddings, "token_type_ids"):
+ buffered_token_type_ids = self.embeddings.token_type_ids[:, :seq_length]
+ buffered_token_type_ids_expanded = buffered_token_type_ids.expand(batch_size, seq_length)
+ token_type_ids = buffered_token_type_ids_expanded
+ else:
+ token_type_ids = torch.zeros(input_shape, dtype=torch.long, device=device)
+
+ # We can provide a self-attention mask of dimensions [batch_size, from_seq_length, to_seq_length]
+ # ourselves in which case we just need to make it broadcastable to all heads.
+ extended_attention_mask: torch.Tensor = self.get_extended_attention_mask(attention_mask, input_shape, device)
+
+ # If a 2D or 3D attention mask is provided for the cross-attention
+ # we need to make broadcastable to [batch_size, num_heads, seq_length, seq_length]
+ if self.config.is_decoder and encoder_hidden_states is not None:
+ encoder_batch_size, encoder_sequence_length, _ = encoder_hidden_states.size()
+ encoder_hidden_shape = (encoder_batch_size, encoder_sequence_length)
+ if encoder_attention_mask is None:
+ encoder_attention_mask = torch.ones(encoder_hidden_shape, device=device)
+ encoder_extended_attention_mask = self.invert_attention_mask(encoder_attention_mask)
+ else:
+ encoder_extended_attention_mask = None
+
+ # Prepare head mask if needed
+ # 1.0 in head_mask indicate we keep the head
+ # attention_probs has shape bsz x n_heads x N x N
+ # input head_mask has shape [num_heads] or [num_hidden_layers x num_heads]
+ # and head_mask is converted to shape [num_hidden_layers x batch x num_heads x seq_length x seq_length]
+ head_mask = self.get_head_mask(head_mask, self.config.num_hidden_layers)
+
+ embedding_output = self.embeddings(
+ input_ids=input_ids,
+ position_ids=position_ids,
+ token_type_ids=token_type_ids,
+ inputs_embeds=inputs_embeds,
+ past_key_values_length=past_key_values_length,
+ )
+ encoder_outputs = self.encoder(
+ embedding_output,
+ attention_mask=extended_attention_mask,
+ head_mask=head_mask,
+ encoder_hidden_states=encoder_hidden_states,
+ encoder_attention_mask=encoder_extended_attention_mask,
+ past_key_values=past_key_values,
+ use_cache=use_cache,
+ output_attentions=output_attentions,
+ output_hidden_states=output_hidden_states,
+ return_dict=return_dict,
+ )
+ sequence_output = encoder_outputs[0]
+ pooled_output = self.pooler(sequence_output) if self.pooler is not None else None
+
+ if not return_dict:
+ return (sequence_output, pooled_output) + encoder_outputs[1:]
+
+ return BaseModelOutputWithPoolingAndCrossAttentions(
+ last_hidden_state=sequence_output,
+ pooler_output=pooled_output,
+ past_key_values=encoder_outputs.past_key_values,
+ hidden_states=encoder_outputs.hidden_states,
+ attentions=encoder_outputs.attentions,
+ cross_attentions=encoder_outputs.cross_attentions,
+ )
+
+
+## ======== Adapter For BERT ========
+class BertAdaOutput(nn.Module):
+ def __init__(self, config):
+ super().__init__()
+ self.dense = nn.Linear(config.intermediate_size, config.hidden_size)
+ self.config = config
+
+ self.adaptation_layer = AdapeterLayer(n_in=config.intermediate_size, n_out=config.hidden_size,
+ adapter_dim=config.adapter_dim, adapter_choice=config.adapter_choice)
+
+ self.LayerNorm = nn.LayerNorm(config.hidden_size, eps=config.layer_norm_eps)
+ self.dropout = nn.Dropout(config.hidden_dropout_prob)
+
+ def forward(self, hidden_states, input_tensor):
+ if self.config.adapter_choice == 'lora':
+ hidden_states = self.dense(hidden_states) + self.adaptation_layer(hidden_states)
+ else:
+ hidden_states = self.dense(hidden_states)
+ hidden_states = self.adaptation_layer(hidden_states)
+ hidden_states = self.dropout(hidden_states)
+ hidden_states = self.LayerNorm(hidden_states + input_tensor)
+ return hidden_states
+
+class BertAdaSelfOutput(nn.Module):
+ def __init__(self, config):
+ super().__init__()
+ self.config = config
+ self.dense = nn.Linear(config.hidden_size, config.hidden_size)
+ self.adaptation_layer = AdapeterLayer(n_in=config.intermediate_size, n_out=config.hidden_size,
+ adapter_dim=config.adapter_dim, adapter_choice=config.adapter_choice)
+ self.LayerNorm = nn.LayerNorm(config.hidden_size, eps=config.layer_norm_eps)
+ self.dropout = nn.Dropout(config.hidden_dropout_prob)
+
+ def forward(self, hidden_states, input_tensor):
+ if self.config.adapter_choice == 'lora':
+ hidden_states = self.dense(hidden_states) + self.adaptation_layer(hidden_states)
+ else:
+ hidden_states = self.dense(hidden_states)
+ hidden_states = self.adaptation_layer(hidden_states)
+ hidden_states = self.dropout(hidden_states)
+ hidden_states = self.LayerNorm(hidden_states + input_tensor)
+ return hidden_states
+
+
+class BertAdaAttention(nn.Module):
+ def __init__(self, config):
+ super().__init__()
+ self.self = BertSelfAttention(config)
+ self.output = BertAdaSelfOutput(config)
+ self.pruned_heads = set()
+
+ def prune_heads(self, heads):
+ if len(heads) == 0:
+ return
+ heads, index = find_pruneable_heads_and_indices(
+ heads, self.self.num_attention_heads, self.self.attention_head_size, self.pruned_heads
+ )
+
+ # Prune linear layers
+ self.self.query = prune_linear_layer(self.self.query, index)
+ self.self.key = prune_linear_layer(self.self.key, index)
+ self.self.value = prune_linear_layer(self.self.value, index)
+ self.output.dense = prune_linear_layer(self.output.dense, index, dim=1)
+
+ # Update hyper params and store pruned heads
+ self.self.num_attention_heads = self.self.num_attention_heads - len(heads)
+ self.self.all_head_size = self.self.attention_head_size * self.self.num_attention_heads
+ self.pruned_heads = self.pruned_heads.union(heads)
+
+ def forward(
+ self,
+ hidden_states,
+ attention_mask=None,
+ head_mask=None,
+ encoder_hidden_states=None,
+ encoder_attention_mask=None,
+ past_key_value=None,
+ output_attentions=False,
+ ):
+ self_outputs = self.self(
+ hidden_states,
+ attention_mask,
+ head_mask,
+ encoder_hidden_states,
+ encoder_attention_mask,
+ past_key_value,
+ output_attentions,
+ )
+ attention_output = self.output(self_outputs[0], hidden_states)
+ outputs = (attention_output,) + self_outputs[1:] # add attentions if we output them
+ return outputs
+
+class BertAdaLayer(nn.Module):
+ def __init__(self, config):
+ super().__init__()
+ self.chunk_size_feed_forward = config.chunk_size_feed_forward
+ self.seq_len_dim = 1
+ self.attention = BertAdaAttention(config)
+ self.is_decoder = config.is_decoder
+ self.add_cross_attention = config.add_cross_attention
+ if self.add_cross_attention:
+ assert self.is_decoder, f"{self} should be used as a decoder model if cross attention is added"
+ self.crossattention = BertAttention(config)
+ self.intermediate = BertIntermediate(config)
+ self.output = BertAdaOutput(config)
+
+ def forward(
+ self,
+ hidden_states,
+ attention_mask=None,
+ head_mask=None,
+ encoder_hidden_states=None,
+ encoder_attention_mask=None,
+ past_key_value=None,
+ output_attentions=False,
+ ):
+ # decoder uni-directional self-attention cached key/values tuple is at positions 1,2
+ self_attn_past_key_value = past_key_value[:2] if past_key_value is not None else None
+ self_attention_outputs = self.attention(
+ hidden_states,
+ attention_mask,
+ head_mask,
+ output_attentions=output_attentions,
+ past_key_value=self_attn_past_key_value,
+ )
+ attention_output = self_attention_outputs[0]
+
+ # if decoder, the last output is tuple of self-attn cache
+ if self.is_decoder:
+ outputs = self_attention_outputs[1:-1]
+ present_key_value = self_attention_outputs[-1]
+ else:
+ outputs = self_attention_outputs[1:] # add self attentions if we output attention weights
+
+ cross_attn_present_key_value = None
+ if self.is_decoder and encoder_hidden_states is not None:
+ assert hasattr(
+ self, "crossattention"
+ ), f"If `encoder_hidden_states` are passed, {self} has to be instantiated with cross-attention layers by setting `config.add_cross_attention=True`"
+
+ # cross_attn cached key/values tuple is at positions 3,4 of past_key_value tuple
+ cross_attn_past_key_value = past_key_value[-2:] if past_key_value is not None else None
+ cross_attention_outputs = self.crossattention(
+ attention_output,
+ attention_mask,
+ head_mask,
+ encoder_hidden_states,
+ encoder_attention_mask,
+ cross_attn_past_key_value,
+ output_attentions,
+ )
+ attention_output = cross_attention_outputs[0]
+ outputs = outputs + cross_attention_outputs[1:-1] # add cross attentions if we output attention weights
+
+ # add cross-attn cache to positions 3,4 of present_key_value tuple
+ cross_attn_present_key_value = cross_attention_outputs[-1]
+ present_key_value = present_key_value + cross_attn_present_key_value
+
+ layer_output = apply_chunking_to_forward(
+ self.feed_forward_chunk, self.chunk_size_feed_forward, self.seq_len_dim, attention_output
+ )
+ outputs = (layer_output,) + outputs
+
+ # if decoder, return the attn key/values as the last output
+ if self.is_decoder:
+ outputs = outputs + (present_key_value,)
+
+ return outputs
+
+ def feed_forward_chunk(self, attention_output):
+ intermediate_output = self.intermediate(attention_output)
+ layer_output = self.output(intermediate_output, attention_output)
+ return layer_output
+
+class BertAdaEncoder(nn.Module):
+ def __init__(self, config):
+ super().__init__()
+ self.config = config
+ self.layer = nn.ModuleList([BertAdaLayer(config) for _ in range(config.num_hidden_layers)])
+
+ def forward(
+ self,
+ hidden_states,
+ attention_mask=None,
+ head_mask=None,
+ encoder_hidden_states=None,
+ encoder_attention_mask=None,
+ past_key_values=None,
+ use_cache=None,
+ output_attentions=False,
+ output_hidden_states=False,
+ return_dict=True,
+ ):
+ all_hidden_states = () if output_hidden_states else None
+ all_self_attentions = () if output_attentions else None
+ all_cross_attentions = () if output_attentions and self.config.add_cross_attention else None
+
+ next_decoder_cache = () if use_cache else None
+ for i, layer_module in enumerate(self.layer):
+ if output_hidden_states:
+ all_hidden_states = all_hidden_states + (hidden_states,)
+
+ layer_head_mask = head_mask[i] if head_mask is not None else None
+ past_key_value = past_key_values[i] if past_key_values is not None else None
+
+ if getattr(self.config, "gradient_checkpointing", False) and self.training:
+
+ if use_cache:
+ logger.warning(
+ "`use_cache=True` is incompatible with `config.gradient_checkpointing=True`. Setting "
+ "`use_cache=False`..."
+ )
+ use_cache = False
+
+ def create_custom_forward(module):
+ def custom_forward(*inputs):
+ return module(*inputs, past_key_value, output_attentions)
+
+ return custom_forward
+
+ layer_outputs = torch.utils.checkpoint.checkpoint(
+ create_custom_forward(layer_module),
+ hidden_states,
+ attention_mask,
+ layer_head_mask,
+ encoder_hidden_states,
+ encoder_attention_mask,
+ )
+ else:
+ layer_outputs = layer_module(
+ hidden_states,
+ attention_mask,
+ layer_head_mask,
+ encoder_hidden_states,
+ encoder_attention_mask,
+ past_key_value,
+ output_attentions,
+ )
+
+ hidden_states = layer_outputs[0]
+ if use_cache:
+ next_decoder_cache += (layer_outputs[-1],)
+ if output_attentions:
+ all_self_attentions = all_self_attentions + (layer_outputs[1],)
+ if self.config.add_cross_attention:
+ all_cross_attentions = all_cross_attentions + (layer_outputs[2],)
+
+ if output_hidden_states:
+ all_hidden_states = all_hidden_states + (hidden_states,)
+
+ if not return_dict:
+ return tuple(
+ v
+ for v in [
+ hidden_states,
+ next_decoder_cache,
+ all_hidden_states,
+ all_self_attentions,
+ all_cross_attentions,
+ ]
+ if v is not None
+ )
+ return BaseModelOutputWithPastAndCrossAttentions(
+ last_hidden_state=hidden_states,
+ past_key_values=next_decoder_cache,
+ hidden_states=all_hidden_states,
+ attentions=all_self_attentions,
+ cross_attentions=all_cross_attentions,
+ )
+
+
+class BertAdaModel(BertPreTrainedModel):
+ """
+ The model can behave as an encoder (with only self-attention) as well as a decoder, in which case a layer of
+ cross-attention is added between the self-attention layers, following the architecture described in `Attention is
+ all you need `__ by Ashish Vaswani, Noam Shazeer, Niki Parmar, Jakob Uszkoreit,
+ Llion Jones, Aidan N. Gomez, Lukasz Kaiser and Illia Polosukhin.
+ To behave as an decoder the model needs to be initialized with the :obj:`is_decoder` argument of the configuration
+ set to :obj:`True`. To be used in a Seq2Seq model, the model needs to initialized with both :obj:`is_decoder`
+ argument and :obj:`add_cross_attention` set to :obj:`True`; an :obj:`encoder_hidden_states` is then expected as an
+ input to the forward pass.
+ """
+
+ def __init__(self, config, add_pooling_layer=True):
+ super().__init__(config)
+ self.config = config
+
+ self.embeddings = BertEmbeddings(config)
+ self.encoder = BertAdaEncoder(config)
+
+ self.pooler = BertPooler(config) if add_pooling_layer else None
+
+ self.init_weights()
+
+ def get_input_embeddings(self):
+ return self.embeddings.word_embeddings
+
+ def set_input_embeddings(self, value):
+ self.embeddings.word_embeddings = value
+
+ def _prune_heads(self, heads_to_prune):
+ """
+ Prunes heads of the model. heads_to_prune: dict of {layer_num: list of heads to prune in this layer} See base
+ class PreTrainedModel
+ """
+ for layer, heads in heads_to_prune.items():
+ self.encoder.layer[layer].attention.prune_heads(heads)
+
+
+ def forward(
+ self,
+ input_ids=None,
+ attention_mask=None,
+ token_type_ids=None,
+ position_ids=None,
+ head_mask=None,
+ inputs_embeds=None,
+ encoder_hidden_states=None,
+ encoder_attention_mask=None,
+ past_key_values=None,
+ use_cache=None,
+ output_attentions=None,
+ output_hidden_states=None,
+ return_dict=None,
+ ):
+ r"""
+ encoder_hidden_states (:obj:`torch.FloatTensor` of shape :obj:`(batch_size, sequence_length, hidden_size)`, `optional`):
+ Sequence of hidden-states at the output of the last layer of the encoder. Used in the cross-attention if
+ the model is configured as a decoder.
+ encoder_attention_mask (:obj:`torch.FloatTensor` of shape :obj:`(batch_size, sequence_length)`, `optional`):
+ Mask to avoid performing attention on the padding token indices of the encoder input. This mask is used in
+ the cross-attention if the model is configured as a decoder. Mask values selected in ``[0, 1]``:
+ - 1 for tokens that are **not masked**,
+ - 0 for tokens that are **masked**.
+ past_key_values (:obj:`tuple(tuple(torch.FloatTensor))` of length :obj:`config.n_layers` with each tuple having 4 tensors of shape :obj:`(batch_size, num_heads, sequence_length - 1, embed_size_per_head)`):
+ Contains precomputed key and value hidden states of the attention blocks. Can be used to speed up decoding.
+ If :obj:`past_key_values` are used, the user can optionally input only the last :obj:`decoder_input_ids`
+ (those that don't have their past key value states given to this model) of shape :obj:`(batch_size, 1)`
+ instead of all :obj:`decoder_input_ids` of shape :obj:`(batch_size, sequence_length)`.
+ use_cache (:obj:`bool`, `optional`):
+ If set to :obj:`True`, :obj:`past_key_values` key value states are returned and can be used to speed up
+ decoding (see :obj:`past_key_values`).
+ """
+ output_attentions = output_attentions if output_attentions is not None else self.config.output_attentions
+ output_hidden_states = (
+ output_hidden_states if output_hidden_states is not None else self.config.output_hidden_states
+ )
+ return_dict = return_dict if return_dict is not None else self.config.use_return_dict
+
+ if self.config.is_decoder:
+ use_cache = use_cache if use_cache is not None else self.config.use_cache
+ else:
+ use_cache = False
+
+ if input_ids is not None and inputs_embeds is not None:
+ raise ValueError("You cannot specify both input_ids and inputs_embeds at the same time")
+ elif input_ids is not None:
+ input_shape = input_ids.size()
+ elif inputs_embeds is not None:
+ input_shape = inputs_embeds.size()[:-1]
+ else:
+ raise ValueError("You have to specify either input_ids or inputs_embeds")
+
+ batch_size, seq_length = input_shape
+ device = input_ids.device if input_ids is not None else inputs_embeds.device
+
+ # past_key_values_length
+ past_key_values_length = past_key_values[0][0].shape[2] if past_key_values is not None else 0
+
+ if attention_mask is None:
+ attention_mask = torch.ones(((batch_size, seq_length + past_key_values_length)), device=device)
+
+ if token_type_ids is None:
+ if hasattr(self.embeddings, "token_type_ids"):
+ buffered_token_type_ids = self.embeddings.token_type_ids[:, :seq_length]
+ buffered_token_type_ids_expanded = buffered_token_type_ids.expand(batch_size, seq_length)
+ token_type_ids = buffered_token_type_ids_expanded
+ else:
+ token_type_ids = torch.zeros(input_shape, dtype=torch.long, device=device)
+
+ # We can provide a self-attention mask of dimensions [batch_size, from_seq_length, to_seq_length]
+ # ourselves in which case we just need to make it broadcastable to all heads.
+ extended_attention_mask: torch.Tensor = self.get_extended_attention_mask(attention_mask, input_shape, device)
+
+ # If a 2D or 3D attention mask is provided for the cross-attention
+ # we need to make broadcastable to [batch_size, num_heads, seq_length, seq_length]
+ if self.config.is_decoder and encoder_hidden_states is not None:
+ encoder_batch_size, encoder_sequence_length, _ = encoder_hidden_states.size()
+ encoder_hidden_shape = (encoder_batch_size, encoder_sequence_length)
+ if encoder_attention_mask is None:
+ encoder_attention_mask = torch.ones(encoder_hidden_shape, device=device)
+ encoder_extended_attention_mask = self.invert_attention_mask(encoder_attention_mask)
+ else:
+ encoder_extended_attention_mask = None
+
+ # Prepare head mask if needed
+ # 1.0 in head_mask indicate we keep the head
+ # attention_probs has shape bsz x n_heads x N x N
+ # input head_mask has shape [num_heads] or [num_hidden_layers x num_heads]
+ # and head_mask is converted to shape [num_hidden_layers x batch x num_heads x seq_length x seq_length]
+ head_mask = self.get_head_mask(head_mask, self.config.num_hidden_layers)
+
+ embedding_output = self.embeddings(
+ input_ids=input_ids,
+ position_ids=position_ids,
+ token_type_ids=token_type_ids,
+ inputs_embeds=inputs_embeds,
+ past_key_values_length=past_key_values_length,
+ )
+ encoder_outputs = self.encoder(
+ embedding_output,
+ attention_mask=extended_attention_mask,
+ head_mask=head_mask,
+ encoder_hidden_states=encoder_hidden_states,
+ encoder_attention_mask=encoder_extended_attention_mask,
+ past_key_values=past_key_values,
+ use_cache=use_cache,
+ output_attentions=output_attentions,
+ output_hidden_states=output_hidden_states,
+ return_dict=return_dict,
+ )
+ sequence_output = encoder_outputs[0]
+ pooled_output = self.pooler(sequence_output) if self.pooler is not None else None
+
+ if not return_dict:
+ return (sequence_output, pooled_output) + encoder_outputs[1:]
+
+ return BaseModelOutputWithPoolingAndCrossAttentions(
+ last_hidden_state=sequence_output,
+ pooler_output=pooled_output,
+ past_key_values=encoder_outputs.past_key_values,
+ hidden_states=encoder_outputs.hidden_states,
+ attentions=encoder_outputs.attentions,
+ cross_attentions=encoder_outputs.cross_attentions,
+ )
\ No newline at end of file
diff --git a/model/multiple_choice.py b/model/multiple_choice.py
new file mode 100644
index 0000000..dadac3d
--- /dev/null
+++ b/model/multiple_choice.py
@@ -0,0 +1,680 @@
+import torch
+from torch._C import NoopLogger
+import torch.nn
+import torch.nn.functional as F
+from torch import Tensor
+from torch.nn import CrossEntropyLoss, MSELoss, BCEWithLogitsLoss
+
+from transformers import BertModel, BertPreTrainedModel
+from transformers import RobertaModel, RobertaPreTrainedModel
+from transformers.modeling_outputs import MultipleChoiceModelOutput, BaseModelOutput, Seq2SeqLMOutput
+
+from model.prefix_encoder import PrefixEncoder
+from model.deberta import DebertaModel, DebertaPreTrainedModel, ContextPooler, StableDropout
+
+
+
+class BertForMultipleChoice(BertPreTrainedModel):
+ """BERT model for multiple choice tasks.
+ This module is composed of the BERT model with a linear layer on top of
+ the pooled output.
+
+ Params:
+ `config`: a BertConfig class instance with the configuration to build a new model.
+ `num_choices`: the number of classes for the classifier. Default = 2.
+
+ Inputs:
+ `input_ids`: a torch.LongTensor of shape [batch_size, num_choices, sequence_length]
+ with the word token indices in the vocabulary(see the tokens preprocessing logic in the scripts
+ `extract_features.py`, `run_classifier.py` and `run_squad.py`)
+ `token_type_ids`: an optional torch.LongTensor of shape [batch_size, num_choices, sequence_length]
+ with the token types indices selected in [0, 1]. Type 0 corresponds to a `sentence A`
+ and type 1 corresponds to a `sentence B` token (see BERT paper for more details).
+ `attention_mask`: an optional torch.LongTensor of shape [batch_size, num_choices, sequence_length] with indices
+ selected in [0, 1]. It's a mask to be used if the input sequence length is smaller than the max
+ input sequence length in the current batch. It's the mask that we typically use for attention when
+ a batch has varying length sentences.
+ `labels`: labels for the classification output: torch.LongTensor of shape [batch_size]
+ with indices selected in [0, ..., num_choices].
+
+ Outputs:
+ if `labels` is not `None`:
+ Outputs the CrossEntropy classification loss of the output with the labels.
+ if `labels` is `None`:
+ Outputs the classification logits of shape [batch_size, num_labels].
+
+ Example usage:
+ ```python
+ # Already been converted into WordPiece token ids
+ input_ids = torch.LongTensor([[[31, 51, 99], [15, 5, 0]], [[12, 16, 42], [14, 28, 57]]])
+ input_mask = torch.LongTensor([[[1, 1, 1], [1, 1, 0]],[[1,1,0], [1, 0, 0]]])
+ token_type_ids = torch.LongTensor([[[0, 0, 1], [0, 1, 0]],[[0, 1, 1], [0, 0, 1]]])
+ config = BertConfig(vocab_size_or_config_json_file=32000, hidden_size=768,
+ num_hidden_layers=12, num_attention_heads=12, intermediate_size=3072)
+
+ num_choices = 2
+
+ model = BertForMultipleChoice(config, num_choices)
+ logits = model(input_ids, token_type_ids, input_mask)
+ ```
+ """
+
+ def __init__(self, config):
+ super().__init__(config)
+ self.bert = BertModel(config)
+ self.dropout = torch.nn.Dropout(config.hidden_dropout_prob)
+ self.classifier = torch.nn.Linear(config.hidden_size, 1)
+
+ self.init_weights()
+
+ def forward(
+ self,
+ input_ids=None,
+ attention_mask=None,
+ token_type_ids=None,
+ position_ids=None,
+ head_mask=None,
+ inputs_embeds=None,
+ labels=None,
+ output_attentions=None,
+ output_hidden_states=None,
+ return_dict=None,
+ ):
+ return_dict = return_dict if return_dict is not None else self.config.use_return_dict
+ batch_size, num_choices = input_ids.shape[:2]
+
+ input_ids = input_ids.reshape(-1, input_ids.size(-1))
+ token_type_ids = token_type_ids.reshape(-1, token_type_ids.size(-1))
+ attention_mask = attention_mask.reshape(-1, attention_mask.size(-1))
+ position_ids = position_ids.view(-1, position_ids.size(-1)) if position_ids is not None else None
+ inputs_embeds = (
+ inputs_embeds.view(-1, inputs_embeds.size(-2), inputs_embeds.size(-1))
+ if inputs_embeds is not None
+ else None
+ )
+
+ outputs = self.bert(
+ input_ids,
+ attention_mask=attention_mask,
+ token_type_ids=token_type_ids,
+ position_ids=position_ids,
+ head_mask=head_mask,
+ inputs_embeds=inputs_embeds,
+ output_attentions=output_attentions,
+ output_hidden_states=output_hidden_states,
+ return_dict=return_dict,
+ )
+
+ pooled_output = outputs[1]
+
+ pooled_output = self.dropout(pooled_output)
+ logits = self.classifier(pooled_output)
+ reshaped_logits = logits.reshape(-1, num_choices)
+
+ loss = None
+ if labels is not None:
+ loss_fct = CrossEntropyLoss()
+ loss = loss_fct(reshaped_logits, labels)
+
+ if not return_dict:
+ output = (reshaped_logits,) + outputs[2:]
+ return ((loss,) + output) if loss is not None else output
+
+ return MultipleChoiceModelOutput(
+ loss=loss,
+ logits=reshaped_logits,
+ hidden_states=outputs.hidden_states,
+ attentions=outputs.attentions,
+ )
+
+class BertPrefixForMultipleChoice(BertPreTrainedModel):
+ def __init__(self, config):
+ super().__init__(config)
+ self.num_labels = config.num_labels
+ self.config = config
+ self.bert = BertModel(config)
+ self.dropout = torch.nn.Dropout(config.hidden_dropout_prob)
+ self.classifier = torch.nn.Linear(config.hidden_size, 1)
+
+ for param in self.bert.parameters():
+ param.requires_grad = False
+
+ self.pre_seq_len = config.pre_seq_len
+ self.n_layer = config.num_hidden_layers
+ self.n_head = config.num_attention_heads
+ self.n_embd = config.hidden_size // config.num_attention_heads
+
+ self.prefix_tokens = torch.arange(self.pre_seq_len).long()
+ self.prefix_encoder = PrefixEncoder(config)
+
+ bert_param = 0
+ for name, param in self.bert.named_parameters():
+ bert_param += param.numel()
+ all_param = 0
+ for name, param in self.named_parameters():
+ all_param += param.numel()
+ total_param = all_param - bert_param
+ print('total param is {}'.format(total_param)) # 9860105
+
+ def get_prompt(self, batch_size):
+ prefix_tokens = self.prefix_tokens.unsqueeze(0).expand(batch_size, -1).to(self.bert.device)
+ past_key_values = self.prefix_encoder(prefix_tokens)
+ past_key_values = past_key_values.view(
+ batch_size,
+ self.pre_seq_len,
+ self.n_layer * 2,
+ self.n_head,
+ self.n_embd
+ )
+ past_key_values = self.dropout(past_key_values)
+ past_key_values = past_key_values.permute([2, 0, 3, 1, 4]).split(2)
+ return past_key_values
+
+ def forward(
+ self,
+ input_ids=None,
+ attention_mask=None,
+ token_type_ids=None,
+ position_ids=None,
+ head_mask=None,
+ inputs_embeds=None,
+ labels=None,
+ output_attentions=None,
+ output_hidden_states=None,
+ return_dict=None,
+ ):
+ return_dict = return_dict if return_dict is not None else self.config.use_return_dict
+ batch_size, num_choices = input_ids.shape[:2] if input_ids is not None else inputs_embeds[:2]
+
+ input_ids = input_ids.reshape(-1, input_ids.size(-1)) if input_ids is not None else None
+ token_type_ids = token_type_ids.reshape(-1, token_type_ids.size(-1)) if token_type_ids is not None else None
+ attention_mask = attention_mask.reshape(-1, attention_mask.size(-1)) if attention_mask is not None else None
+ position_ids = position_ids.view(-1, position_ids.size(-1)) if position_ids is not None else None
+ inputs_embeds = (
+ inputs_embeds.view(-1, inputs_embeds.size(-2), inputs_embeds.size(-1))
+ if inputs_embeds is not None
+ else None
+ )
+
+ past_key_values = self.get_prompt(batch_size=batch_size * num_choices)
+ prefix_attention_mask = torch.ones(batch_size * num_choices, self.pre_seq_len).to(self.bert.device)
+ attention_mask = torch.cat((prefix_attention_mask, attention_mask), dim=1)
+
+ outputs = self.bert(
+ input_ids,
+ attention_mask=attention_mask,
+ token_type_ids=token_type_ids,
+ position_ids=position_ids,
+ head_mask=head_mask,
+ inputs_embeds=inputs_embeds,
+ output_attentions=output_attentions,
+ output_hidden_states=output_hidden_states,
+ return_dict=return_dict,
+ past_key_values=past_key_values,
+ )
+
+ pooled_output = outputs[1]
+
+ pooled_output = self.dropout(pooled_output)
+ logits = self.classifier(pooled_output)
+ reshaped_logits = logits.reshape(-1, num_choices)
+
+ loss = None
+ if labels is not None:
+ loss_fct = CrossEntropyLoss()
+ loss = loss_fct(reshaped_logits, labels)
+
+ if not return_dict:
+ output = (reshaped_logits,) + outputs[2:]
+ return ((loss,) + output) if loss is not None else output
+
+ return MultipleChoiceModelOutput(
+ loss=loss,
+ logits=reshaped_logits,
+ hidden_states=outputs.hidden_states,
+ attentions=outputs.attentions,
+ )
+
+class RobertaPrefixForMultipleChoice(RobertaPreTrainedModel):
+ _keys_to_ignore_on_load_missing = [r"position_ids"]
+
+ def __init__(self, config):
+ super().__init__(config)
+
+ self.roberta = RobertaModel(config)
+ self.dropout = torch.nn.Dropout(config.hidden_dropout_prob)
+ self.classifier = torch.nn.Linear(config.hidden_size, 1)
+
+ self.init_weights()
+
+
+ for param in self.roberta.parameters():
+ param.requires_grad = False
+
+ self.pre_seq_len = config.pre_seq_len
+ self.n_layer = config.num_hidden_layers
+ self.n_head = config.num_attention_heads
+ self.n_embd = config.hidden_size // config.num_attention_heads
+
+ self.prefix_tokens = torch.arange(self.pre_seq_len).long()
+ self.prefix_encoder = PrefixEncoder(config)
+
+ bert_param = 0
+ for name, param in self.roberta.named_parameters():
+ bert_param += param.numel()
+ all_param = 0
+ for name, param in self.named_parameters():
+ all_param += param.numel()
+ total_param = all_param - bert_param
+ print('total param is {}'.format(total_param))
+
+ def get_prompt(self, batch_size):
+ prefix_tokens = self.prefix_tokens.unsqueeze(0).expand(batch_size, -1).to(self.roberta.device)
+ past_key_values = self.prefix_encoder(prefix_tokens)
+ past_key_values = past_key_values.view(
+ batch_size,
+ self.pre_seq_len,
+ self.n_layer * 2,
+ self.n_head,
+ self.n_embd
+ )
+ past_key_values = self.dropout(past_key_values)
+ past_key_values = past_key_values.permute([2, 0, 3, 1, 4]).split(2)
+ return past_key_values
+
+ def forward(
+ self,
+ input_ids=None,
+ token_type_ids=None,
+ attention_mask=None,
+ labels=None,
+ position_ids=None,
+ head_mask=None,
+ inputs_embeds=None,
+ output_attentions=None,
+ output_hidden_states=None,
+ return_dict=None,
+ ):
+ r"""
+ labels (:obj:`torch.LongTensor` of shape :obj:`(batch_size,)`, `optional`):
+ Labels for computing the multiple choice classification loss. Indices should be in ``[0, ...,
+ num_choices-1]`` where :obj:`num_choices` is the size of the second dimension of the input tensors. (See
+ :obj:`input_ids` above)
+ """
+ return_dict = return_dict if return_dict is not None else self.config.use_return_dict
+ batch_size, num_choices = input_ids.shape[:2] if input_ids is not None else inputs_embeds.shape[:2]
+
+ flat_input_ids = input_ids.view(-1, input_ids.size(-1)) if input_ids is not None else None
+ flat_position_ids = position_ids.view(-1, position_ids.size(-1)) if position_ids is not None else None
+ flat_token_type_ids = token_type_ids.view(-1, token_type_ids.size(-1)) if token_type_ids is not None else None
+ flat_attention_mask = attention_mask.view(-1, attention_mask.size(-1)) if attention_mask is not None else None
+ flat_inputs_embeds = (
+ inputs_embeds.view(-1, inputs_embeds.size(-2), inputs_embeds.size(-1))
+ if inputs_embeds is not None
+ else None
+ )
+
+ past_key_values = self.get_prompt(batch_size=batch_size * num_choices)
+ prefix_attention_mask = torch.ones(batch_size * num_choices, self.pre_seq_len).to(self.roberta.device)
+ flat_attention_mask = torch.cat((prefix_attention_mask, flat_attention_mask), dim=1)
+
+ outputs = self.roberta(
+ flat_input_ids,
+ position_ids=flat_position_ids,
+ token_type_ids=flat_token_type_ids,
+ attention_mask=flat_attention_mask,
+ head_mask=head_mask,
+ inputs_embeds=flat_inputs_embeds,
+ output_attentions=output_attentions,
+ output_hidden_states=output_hidden_states,
+ return_dict=return_dict,
+ past_key_values=past_key_values,
+ )
+ pooled_output = outputs[1]
+
+ pooled_output = self.dropout(pooled_output)
+ logits = self.classifier(pooled_output)
+ reshaped_logits = logits.view(-1, num_choices)
+
+ loss = None
+ if labels is not None:
+ loss_fct = CrossEntropyLoss()
+ loss = loss_fct(reshaped_logits, labels)
+
+ if not return_dict:
+ output = (reshaped_logits,) + outputs[2:]
+ return ((loss,) + output) if loss is not None else output
+
+ return MultipleChoiceModelOutput(
+ loss=loss,
+ logits=reshaped_logits,
+ hidden_states=outputs.hidden_states,
+ attentions=outputs.attentions,
+ )
+
+class DebertaPrefixForMultipleChoice(DebertaPreTrainedModel):
+ def __init__(self, config):
+ super().__init__(config)
+ self.num_labels = config.num_labels
+ self.config = config
+ self.deberta = DebertaModel(config)
+ self.pooler = ContextPooler(config)
+ output_dim = self.pooler.output_dim
+ self.classifier = torch.nn.Linear(output_dim, 1)
+ self.dropout = StableDropout(config.hidden_dropout_prob)
+ self.init_weights()
+
+ for param in self.deberta.parameters():
+ param.requires_grad = False
+
+ self.pre_seq_len = config.pre_seq_len
+ self.n_layer = config.num_hidden_layers
+ self.n_head = config.num_attention_heads
+ self.n_embd = config.hidden_size // config.num_attention_heads
+
+ self.prefix_tokens = torch.arange(self.pre_seq_len).long()
+ self.prefix_encoder = PrefixEncoder(config)
+
+ deberta_param = 0
+ for name, param in self.deberta.named_parameters():
+ deberta_param += param.numel()
+ all_param = 0
+ for name, param in self.named_parameters():
+ all_param += param.numel()
+ total_param = all_param - deberta_param
+ print('total param is {}'.format(total_param))
+
+ def get_prompt(self, batch_size):
+ prefix_tokens = self.prefix_tokens.unsqueeze(0).expand(batch_size, -1).to(self.deberta.device)
+ past_key_values = self.prefix_encoder(prefix_tokens)
+ past_key_values = past_key_values.view(
+ batch_size,
+ self.pre_seq_len,
+ self.n_layer * 2,
+ self.n_head,
+ self.n_embd
+ )
+ past_key_values = self.dropout(past_key_values)
+ past_key_values = past_key_values.permute([2, 0, 3, 1, 4]).split(2)
+ return past_key_values
+
+ def forward(
+ self,
+ input_ids=None,
+ attention_mask=None,
+ token_type_ids=None,
+ position_ids=None,
+ head_mask=None,
+ inputs_embeds=None,
+ labels=None,
+ output_attentions=None,
+ output_hidden_states=None,
+ return_dict=None,
+ ):
+ return_dict = return_dict if return_dict is not None else self.config.use_return_dict
+ batch_size, num_choices = input_ids.shape[:2] if input_ids is not None else inputs_embeds.shape[:2]
+
+ flat_input_ids = input_ids.view(-1, input_ids.size(-1)) if input_ids is not None else None
+ flat_position_ids = position_ids.view(-1, position_ids.size(-1)) if position_ids is not None else None
+ flat_token_type_ids = token_type_ids.view(-1, token_type_ids.size(-1)) if token_type_ids is not None else None
+ flat_attention_mask = attention_mask.view(-1, attention_mask.size(-1)) if attention_mask is not None else None
+ flat_inputs_embeds = (
+ inputs_embeds.view(-1, inputs_embeds.size(-2), inputs_embeds.size(-1))
+ if inputs_embeds is not None
+ else None
+ )
+
+ past_key_values = self.get_prompt(batch_size=batch_size * num_choices)
+ prefix_attention_mask = torch.ones(batch_size * num_choices, self.pre_seq_len).to(self.deberta.device)
+ flat_attention_mask = torch.cat((prefix_attention_mask, flat_attention_mask), dim=1)
+
+ outputs = self.deberta(
+ flat_input_ids,
+ attention_mask=flat_attention_mask,
+ token_type_ids=flat_token_type_ids,
+ position_ids=flat_position_ids,
+ inputs_embeds=flat_inputs_embeds,
+ output_attentions=output_attentions,
+ output_hidden_states=output_hidden_states,
+ return_dict=return_dict,
+ past_key_values=past_key_values,
+ )
+
+ encoder_layer = outputs[0]
+
+ pooled_output = self.pooler(encoder_layer)
+ pooled_output = self.dropout(pooled_output)
+ logits = self.classifier(pooled_output)
+ reshaped_logits = logits.view(-1, num_choices)
+
+ loss = None
+ if labels is not None:
+ loss_fct = CrossEntropyLoss()
+ loss = loss_fct(reshaped_logits, labels)
+
+ if not return_dict:
+ output = (reshaped_logits,) + outputs[2:]
+ return ((loss,) + output) if loss is not None else output
+
+ return MultipleChoiceModelOutput(
+ loss=loss,
+ logits=reshaped_logits,
+ hidden_states=outputs.hidden_states,
+ attentions=outputs.attentions,
+ )
+
+
+class BertPromptForMultipleChoice(BertPreTrainedModel):
+ def __init__(self, config):
+ super().__init__(config)
+ self.num_labels = config.num_labels
+ self.config = config
+ self.bert = BertModel(config)
+ self.embeddings = self.bert.embeddings
+ self.dropout = torch.nn.Dropout(config.hidden_dropout_prob)
+ self.classifier = torch.nn.Linear(config.hidden_size, 1)
+
+ for param in self.bert.parameters():
+ param.requires_grad = False
+
+ self.pre_seq_len = config.pre_seq_len
+ self.n_layer = config.num_hidden_layers
+ self.n_head = config.num_attention_heads
+ self.n_embd = config.hidden_size // config.num_attention_heads
+
+ self.prefix_tokens = torch.arange(self.pre_seq_len).long()
+ self.prefix_encoder = torch.nn.Embedding(self.pre_seq_len, config.hidden_size)
+
+ bert_param = 0
+ for name, param in self.bert.named_parameters():
+ bert_param += param.numel()
+ all_param = 0
+ for name, param in self.named_parameters():
+ all_param += param.numel()
+ total_param = all_param - bert_param
+ print('total param is {}'.format(total_param)) # 9860105
+
+ def get_prompt(self, batch_size):
+ prefix_tokens = self.prefix_tokens.unsqueeze(0).expand(batch_size, -1).to(self.bert.device)
+ prompts = self.prefix_encoder(prefix_tokens)
+ return prompts
+
+ def forward(
+ self,
+ input_ids=None,
+ attention_mask=None,
+ token_type_ids=None,
+ position_ids=None,
+ head_mask=None,
+ inputs_embeds=None,
+ labels=None,
+ output_attentions=None,
+ output_hidden_states=None,
+ return_dict=None,
+ ):
+ return_dict = return_dict if return_dict is not None else self.config.use_return_dict
+ batch_size, num_choices = input_ids.shape[:2] if input_ids is not None else inputs_embeds[:2]
+
+ input_ids = input_ids.reshape(-1, input_ids.size(-1)) if input_ids is not None else None
+ token_type_ids = token_type_ids.reshape(-1, token_type_ids.size(-1)) if token_type_ids is not None else None
+ attention_mask = attention_mask.reshape(-1, attention_mask.size(-1)) if attention_mask is not None else None
+ position_ids = position_ids.view(-1, position_ids.size(-1)) if position_ids is not None else None
+ inputs_embeds = (
+ inputs_embeds.view(-1, inputs_embeds.size(-2), inputs_embeds.size(-1))
+ if inputs_embeds is not None
+ else None
+ )
+
+ raw_embedding = self.embeddings(
+ input_ids=input_ids,
+ position_ids=position_ids,
+ token_type_ids=token_type_ids,
+ )
+ prompts = self.get_prompt(batch_size=batch_size * num_choices)
+ inputs_embeds = torch.cat((prompts, raw_embedding), dim=1)
+
+ prefix_attention_mask = torch.ones(batch_size * num_choices, self.pre_seq_len).to(self.bert.device)
+ attention_mask = torch.cat((prefix_attention_mask, attention_mask), dim=1)
+
+ outputs = self.bert(
+ attention_mask=attention_mask,
+ head_mask=head_mask,
+ inputs_embeds=inputs_embeds,
+ output_attentions=output_attentions,
+ output_hidden_states=output_hidden_states,
+ return_dict=return_dict,
+ )
+
+ pooled_output = outputs[1]
+
+ pooled_output = self.dropout(pooled_output)
+ logits = self.classifier(pooled_output)
+ reshaped_logits = logits.reshape(-1, num_choices)
+
+ loss = None
+ if labels is not None:
+ loss_fct = CrossEntropyLoss()
+ loss = loss_fct(reshaped_logits, labels)
+
+ if not return_dict:
+ output = (reshaped_logits,) + outputs[2:]
+ return ((loss,) + output) if loss is not None else output
+
+ return MultipleChoiceModelOutput(
+ loss=loss,
+ logits=reshaped_logits,
+ hidden_states=outputs.hidden_states,
+ attentions=outputs.attentions,
+ )
+
+
+class RobertaPromptForMultipleChoice(RobertaPreTrainedModel):
+ _keys_to_ignore_on_load_missing = [r"position_ids"]
+
+ def __init__(self, config):
+ super().__init__(config)
+
+ self.roberta = RobertaModel(config)
+ self.embeddings = self.roberta.embeddings
+ self.dropout = torch.nn.Dropout(config.hidden_dropout_prob)
+ self.classifier = torch.nn.Linear(config.hidden_size, 1)
+
+ self.init_weights()
+
+
+ for param in self.roberta.parameters():
+ param.requires_grad = False
+
+ self.pre_seq_len = config.pre_seq_len
+ self.n_layer = config.num_hidden_layers
+ self.n_head = config.num_attention_heads
+ self.n_embd = config.hidden_size // config.num_attention_heads
+
+ self.prefix_tokens = torch.arange(self.pre_seq_len).long()
+ self.prefix_encoder = torch.nn.Embedding(self.pre_seq_len, config.hidden_size)
+
+ bert_param = 0
+ for name, param in self.roberta.named_parameters():
+ bert_param += param.numel()
+ all_param = 0
+ for name, param in self.named_parameters():
+ all_param += param.numel()
+ total_param = all_param - bert_param
+ print('total param is {}'.format(total_param))
+
+ def get_prompt(self, batch_size):
+ prefix_tokens = self.prefix_tokens.unsqueeze(0).expand(batch_size, -1).to(self.roberta.device)
+ prompts = self.prefix_encoder(prefix_tokens)
+ return prompts
+
+ def forward(
+ self,
+ input_ids=None,
+ token_type_ids=None,
+ attention_mask=None,
+ labels=None,
+ position_ids=None,
+ head_mask=None,
+ inputs_embeds=None,
+ output_attentions=None,
+ output_hidden_states=None,
+ return_dict=None,
+ ):
+ r"""
+ labels (:obj:`torch.LongTensor` of shape :obj:`(batch_size,)`, `optional`):
+ Labels for computing the multiple choice classification loss. Indices should be in ``[0, ...,
+ num_choices-1]`` where :obj:`num_choices` is the size of the second dimension of the input tensors. (See
+ :obj:`input_ids` above)
+ """
+ return_dict = return_dict if return_dict is not None else self.config.use_return_dict
+ batch_size, num_choices = input_ids.shape[:2] if input_ids is not None else inputs_embeds.shape[:2]
+
+ input_ids = input_ids.view(-1, input_ids.size(-1)) if input_ids is not None else None
+ position_ids = position_ids.view(-1, position_ids.size(-1)) if position_ids is not None else None
+ token_type_ids = token_type_ids.view(-1, token_type_ids.size(-1)) if token_type_ids is not None else None
+ attention_mask = attention_mask.view(-1, attention_mask.size(-1)) if attention_mask is not None else None
+ inputs_embeds = (
+ inputs_embeds.view(-1, inputs_embeds.size(-2), inputs_embeds.size(-1))
+ if inputs_embeds is not None
+ else None
+ )
+
+ raw_embedding = self.embeddings(
+ input_ids=input_ids,
+ position_ids=position_ids,
+ token_type_ids=token_type_ids,
+ )
+ prompts = self.get_prompt(batch_size=batch_size * num_choices)
+ inputs_embeds = torch.cat((prompts, raw_embedding), dim=1)
+ prefix_attention_mask = torch.ones(batch_size * num_choices, self.pre_seq_len).to(self.roberta.device)
+ attention_mask = torch.cat((prefix_attention_mask, attention_mask), dim=1)
+
+ outputs = self.roberta(
+ attention_mask=attention_mask,
+ head_mask=head_mask,
+ inputs_embeds=inputs_embeds,
+ output_attentions=output_attentions,
+ output_hidden_states=output_hidden_states,
+ return_dict=return_dict,
+ )
+ pooled_output = outputs[1]
+
+ pooled_output = self.dropout(pooled_output)
+ logits = self.classifier(pooled_output)
+ reshaped_logits = logits.view(-1, num_choices)
+
+ loss = None
+ if labels is not None:
+ loss_fct = CrossEntropyLoss()
+ loss = loss_fct(reshaped_logits, labels)
+
+ if not return_dict:
+ output = (reshaped_logits,) + outputs[2:]
+ return ((loss,) + output) if loss is not None else output
+
+ return MultipleChoiceModelOutput(
+ loss=loss,
+ logits=reshaped_logits,
+ hidden_states=outputs.hidden_states,
+ attentions=outputs.attentions,
+ )
\ No newline at end of file
diff --git a/model/parameter_freeze.py b/model/parameter_freeze.py
new file mode 100644
index 0000000..d3e18b6
--- /dev/null
+++ b/model/parameter_freeze.py
@@ -0,0 +1,118 @@
+import torch
+# parameter fixing and unfreezing
+# add by wjn
+class ParameterFreeze():
+ # freeze all parameters
+ def freeze_lm(self, model: torch.nn.Module):
+ for name, param in model.named_parameters():
+ param.requires_grad = False
+ return model
+
+ # freeze all parameters without cls / mlm head
+ def freeze_lm_encoder(self, model: torch.nn.Module):
+ for name, param in model.named_parameters():
+ if 'lm_head' in name or ('cls' in name):
+ print(name)
+ continue
+ param.requires_grad = False
+ return model
+
+ # freeze all parameters without bias
+ def freeze_lm_finetune_bias(self, model: torch.nn.Module):
+ for name, param in model.named_parameters():
+ if "bias" in name:
+ print(name)
+ continue
+ param.requires_grad = False
+ return model
+
+ # freeze the comonent that user defined
+ def freeze_lm_component(self, model: torch.nn.Module, component: str):
+ if 'attention' in component:
+ for name, param in model.named_parameters():
+ if 'attention' in name:
+ if 'output' in component:
+ if 'output' in name:
+ continue
+ else:
+ continue
+ param.requires_grad = False
+ model = self.unfreeze_classification_head(model)
+ elif 'feedforward' in component:
+ for name, param in model.named_parameters():
+ if 'dense' in name and 'attention' not in name:
+ if 'output' in component:
+ if 'output' in name:
+ continue
+ else:
+ if 'intermediate' in component:
+ if 'intermediate' in name:
+ continue
+ param.requires_grad = False
+ model = self.unfreeze_classification_head(model)
+ elif component == 'adapter':
+ for name, param in model.named_parameters():
+ if 'adapter' in name:
+ continue
+
+ param.requires_grad = False
+ model = self.unfreeze_classification_head(model)
+ elif 'embedding' in component:
+ for name, param in model.named_parameters():
+ if 'embedding' in name:
+ continue
+
+ param.requires_grad = False
+ model = self.unfreeze_classification_head(model)
+ elif 'bias' in component:
+ for name, param in model.named_parameters():
+ if 'bias' in name:
+ continue
+ param.requires_grad = False
+ model = self.unfreeze_classification_head(model)
+ elif 'head' in component:
+ for name, param in model.named_parameters():
+ param.requires_grad = False
+ model = self.unfreeze_classification_head(model)
+
+ elif "prompt_emb" in component:
+ for name, param in model.named_parameters():
+ if 'prompt_emb' in name:
+ continue
+ param.requires_grad = False
+ return model
+
+ # unfreeze cls head
+ def unfreeze_classification_head(self, model: torch.nn.Module):
+ for name, param in model.named_parameters():
+ if 'lm_head' in name or ('cls' in name) or ('classifier' in name):
+ param.requires_grad = True
+ return model
+
+ # freeze k layers
+ def freeze_lm_k_layers(self, model: torch.nn.Module, k):
+ keep_layers = []
+ update_parameters = []
+ for i in range(k):
+ keep_layers.append('layer.'+str(23-i))
+
+ for name, param in model.named_parameters():
+ update = False
+ for layer_num in keep_layers:
+ if layer_num in name:
+ if 'dense' in name and 'attention' not in name:
+ if 'output' in name:
+ print(name)
+ update_parameters.append(name)
+ update = True
+
+ if not update:
+ param.requires_grad = False
+ model = self.unfreeze_classification_head(model)
+ return model
+
+
+ def unfreeze_lm(self, model: torch.nn.Module):
+ for param in model.parameters():
+ param.requires_grad = True
+ return model
diff --git a/model/prefix_encoder.py b/model/prefix_encoder.py
new file mode 100644
index 0000000..a0d630e
--- /dev/null
+++ b/model/prefix_encoder.py
@@ -0,0 +1,38 @@
+import torch
+
+# from transformers.models.bart.modeling_bart import BartForConditionalGeneration
+# from transformers.models.bert.modeling_bert import BertForSequenceClassification
+
+# model = BartForConditionalGeneration(None)
+
+
+
+class PrefixEncoder(torch.nn.Module):
+ r'''
+ The torch.nn model to encode the prefix
+
+ Input shape: (batch-size, prefix-length)
+
+ Output shape: (batch-size, prefix-length, 2*layers*hidden)
+ '''
+ def __init__(self, config):
+ super().__init__()
+ self.prefix_projection = config.prefix_projection
+ if self.prefix_projection:
+ # Use a two-layer MLP to encode the prefix
+ self.embedding = torch.nn.Embedding(config.pre_seq_len, config.hidden_size)
+ self.trans = torch.nn.Sequential(
+ torch.nn.Linear(config.hidden_size, config.prefix_hidden_size),
+ torch.nn.Tanh(),
+ torch.nn.Linear(config.prefix_hidden_size, config.num_hidden_layers * 2 * config.hidden_size)
+ )
+ else:
+ self.embedding = torch.nn.Embedding(config.pre_seq_len, config.num_hidden_layers * 2 * config.hidden_size)
+
+ def forward(self, prefix: torch.Tensor):
+ if self.prefix_projection:
+ prefix_tokens = self.embedding(prefix) # [pre_seq_len, hidden_dim]
+ past_key_values = self.trans(prefix_tokens)
+ else:
+ past_key_values = self.embedding(prefix)
+ return past_key_values
\ No newline at end of file
diff --git a/model/prompt_for_sequence_classification.py b/model/prompt_for_sequence_classification.py
new file mode 100644
index 0000000..6e04e14
--- /dev/null
+++ b/model/prompt_for_sequence_classification.py
@@ -0,0 +1,3403 @@
+"""Custom models for few-shot learning specific operations."""
+
+import torch
+import torch.nn as nn
+import transformers
+import torch.nn.functional as F
+from transformers import AutoConfig, AutoModelForSequenceClassification, AutoTokenizer, EvalPrediction
+from transformers.models.t5.modeling_t5 import T5ForConditionalGeneration
+from transformers.models.bert.modeling_bert import BertPreTrainedModel, BertForSequenceClassification, BertModel, BertOnlyMLMHead
+from transformers.models.roberta.modeling_roberta import RobertaForSequenceClassification, RobertaModel, RobertaLMHead, RobertaClassificationHead, RobertaPreTrainedModel
+from transformers.models.deberta_v2.modeling_deberta_v2 import DebertaV2PreTrainedModel, DebertaV2Model, StableDropout, ContextPooler, DebertaV2OnlyMLMHead
+from transformers.models.deberta.modeling_deberta import DebertaPreTrainedModel, DebertaModel, StableDropout, ContextPooler, DebertaOnlyMLMHead
+from transformers.modeling_outputs import SequenceClassifierOutput
+from transformers.modeling_utils import PreTrainedModel
+from model.loss import stable_kl, CeCriterion, KlCriterion, entropy, SymKlCriterion, ContrastiveLoss
+from transformers.models.bert.configuration_bert import BertConfig
+# from processors import processors_mapping, num_labels_mapping, output_modes_mapping, compute_metrics_mapping, bound_mapping
+import logging
+from model.model_adaptation import RobertaAdaModel, BertAdaModel
+import os
+from model.prefix_encoder import PrefixEncoder
+from model.parameter_freeze import ParameterFreeze
+
+freezer = ParameterFreeze()
+
+
+logger = logging.getLogger(__name__)
+
+def generate_noise(embed, mask, epsilon=1e-5):
+ noise = embed.data.new(embed.size()).normal_(0, 1) * epsilon
+ noise.detach()
+ noise.requires_grad_()
+ return noise
+
+def norm_grad(grad, eff_grad=None, sentence_level=False, norm_p='max', epsilon=1e-5):
+ if norm_p == 'l2':
+ if sentence_level:
+ direction = grad / (torch.norm(grad, dim=(-2, -1), keepdim=True) + epsilon)
+ else:
+ direction = grad / (torch.norm(grad, dim=-1, keepdim=True) + epsilon)
+ elif norm_p == 'l1':
+ direction = grad.sign()
+ else:
+ if sentence_level:
+ direction = grad / (grad.abs().max((-2, -1), keepdim=True)[0] + epsilon)
+ else:
+ direction = grad / (grad.abs().max(-1, keepdim=True)[0] + epsilon)
+ eff_direction = eff_grad / (grad.abs().max(-1, keepdim=True)[0] + epsilon)
+ return direction, eff_direction
+
+
+
+def resize_token_type_embeddings(model, new_num_types: int, random_segment: bool):
+ """
+ Resize the segment (token type) embeddings for BERT
+ """
+ if hasattr(model, 'bert'):
+ old_token_type_embeddings = model.bert.embeddings.token_type_embeddings
+ else:
+ raise NotImplementedError
+ new_token_type_embeddings = nn.Embedding(new_num_types, old_token_type_embeddings.weight.size(1))
+ if not random_segment:
+ new_token_type_embeddings.weight.data[:old_token_type_embeddings.weight.size(0)] = old_token_type_embeddings.weight.data
+
+ model.config.type_vocab_size = new_num_types
+ if hasattr(model, 'bert'):
+ model.bert.embeddings.token_type_embeddings = new_token_type_embeddings
+ else:
+ raise NotImplementedError
+
+
+
+
+# Training the model with prompt and verbalizer
+class LMForPromptFinetuning(BertPreTrainedModel):
+ def __init__(self, config, model_args, data_args):
+ super().__init__(config)
+ self.model_args = model_args
+ self.data_args = data_args
+ self.config = config
+ # Create config
+ num_labels = num_labels_mapping[data_args.dataset_name]
+ self.num_labels = num_labels
+ config.adapter_dim = model_args.adapter_dim
+ try:
+ config.adapter_alpha = model_args.adapter_alpha
+ except:
+ config.adapter_alpha = 32
+ config.adapter_choice = model_args.adapter_choice
+ self.pre_seq_len = self.model_args.pre_seq_len
+ config.pre_seq_len = self.pre_seq_len
+ self.config = config
+
+ if config.model_type == 'roberta':
+ if model_args.prompt_prefix:
+ model_fn = RobertPrefixForPromptFinetuning
+ elif model_args.prompt_ptuning:
+ model_fn = RobertaForPromptFinetuning
+ elif model_args.prompt_adapter:
+ model_fn = RobertaAdapterForPromptFinetuning
+ else:
+ model_fn = RobertaForPromptFinetuning
+
+ elif config.model_type == 'bert':
+ if model_args.prompt_prefix:
+ model_fn = BertPrefixForPromptFinetuning
+ elif model_args.prompt_ptuning:
+ model_fn = BertForPromptFinetuning
+ elif model_args.prompt_adapter:
+ model_fn = BertAdapterForPromptFinetuning
+ else:
+ model_fn = BertForPromptFinetuning
+
+ elif config.model_type == 'deberta':
+ if model_args.prompt_prefix:
+ model_fn = DebertPrefixForPromptFinetuning
+ elif model_args.prompt_ptuning:
+ model_fn = DebertaForPromptFinetuning
+ elif model_args.prompt_adapter:
+ pass
+ else:
+ model_fn = DebertaForPromptFinetuning
+
+ elif config.model_type == 'deberta-v2':
+ if model_args.prompt_prefix:
+ pass
+ elif model_args.prompt_ptuning:
+ model_fn = Debertav2ForPromptFinetuning
+ elif model_args.prompt_adapter:
+ pass
+ else:
+ model_fn = Debertav2ForPromptFinetuning
+
+ elif config.model_type == 't5':
+ if model_args.prompt_prefix:
+ pass
+ elif model_args.prompt_ptuning:
+ self.lm_model = T5ForPromptFinetuning(config)
+ elif model_args.prompt_adapter:
+ pass
+ else:
+ self.lm_model = T5ForPromptFinetuning(config)
+
+ else:
+ raise NotImplementedError
+
+
+ if config.model_type == 't5':
+ self.lm_model.T5 = self.lm_model.T5.from_pretrained(
+ model_args.model_name_or_path,
+ from_tf=bool(".ckpt" in model_args.model_name_or_path),
+ config=config,
+ cache_dir=model_args.cache_dir,
+ )
+
+ else:
+ self.lm_model = model_fn.from_pretrained(
+ model_args.model_name_or_path,
+ from_tf=bool(".ckpt" in model_args.model_name_or_path),
+ config=config,
+ cache_dir=model_args.cache_dir,
+ )
+
+ if config.model_type == "roberta":
+ self.embeddings = self.lm_model.roberta.embeddings
+ elif config.model_type == "bert":
+ self.embeddings = self.lm_model.bert.embeddings
+ elif config.model_type in ["deberta", "deberta-v2"]:
+ self.embeddings = self.lm_model.deberta.embeddings
+ elif config.model_type == "t5":
+ self.embeddings = self.lm_model.T5.embeddings
+
+
+ # Pass dataset and argument information to the model
+ if model_args.prompt_prefix or model_args.prompt_ptuning or model_args.prompt_adapter or model_args.prompt_only:
+ self.lm_model.label_word_list = torch.tensor(data_args.label_word_list).long().cuda()
+ else:
+ raise RuntimeError("You must choose prompt_prefix or prompt_ptuning or prompt_adapter or prompt_only.")
+
+ if output_modes_mapping[data_args.dataset_name] == 'regression':
+ # lower / upper bounds
+ self.lm_model.lb, self.lm_model.ub = bound_mapping[data_args.dataset_name]
+
+ self.lm_model.model_args = model_args
+ self.lm_model.data_args = data_args
+ self.hidden_size = config.hidden_size
+
+ # edit by wjn
+ if self.model_args.prompt_ptuning:
+ self.prompt_embeddings = torch.nn.Embedding(self.pre_seq_len, self.hidden_size)
+ else:
+ self.prompt_embeddings = None
+ # self.data_args.continuous_prompt = self.model_args.pre_seq_len
+
+ if self.model_args.prompt_adapter and self.model_args.adapter_choice != 'none':
+ try:
+ std = self.model_args.adapter_init_std
+ except:
+ std = 0.0002
+ self.init_adapter(std=std)
+
+ self.prompt_encoder = None
+ # add by wjn
+ self.prefix_tokens = torch.arange(self.pre_seq_len).long()
+ self.prefix_encoder = torch.nn.Embedding(self.pre_seq_len, config.hidden_size)
+ # if self.model_args.prompt_ptuning or self.model_args.prompt_prefix:
+ # self.init_embedding()
+
+ def init_adapter(self, std):
+ with torch.no_grad():
+ for name, param in self.lm_model.named_parameters():
+ init_value = 0
+ if 'adapter_proj' in name:
+ if self.model_args.adapter_choice == 'simple':
+ init_value = torch.eye(param.size(0))
+ if std > 0:
+ init_value += torch.normal(0, std, size=param.size())
+ param.copy_(init_value)
+
+ def freeze_lm(self):
+ for name, param in self.lm_model.named_parameters():
+ param.requires_grad = False
+
+ def freeze_lm_encoder(self):
+ for name, param in self.lm_model.named_parameters():
+ if 'lm_head' in name or ('cls' in name):
+ print(name)
+ continue
+ param.requires_grad = False
+
+ def freeze_lm_finetune_bias(self):
+ for name, param in self.lm_model.named_parameters():
+ if "bias" in name:
+ print(name)
+ continue
+ param.requires_grad = False
+
+ def freeze_lm_component(self, component):
+
+ if 'attention' in component:
+ for name, param in self.lm_model.named_parameters():
+ if 'attention' in name:
+ if 'output' in component:
+ if 'output' in name:
+ continue
+ else:
+ continue
+ param.requires_grad = False
+ self.unfreeze_classification_head()
+ elif 'feedforward' in component:
+ for name, param in self.lm_model.named_parameters():
+ if 'dense' in name and 'attention' not in name:
+ if 'output' in component:
+ if 'output' in name:
+ continue
+ else:
+ if 'intermediate' in component:
+ if 'intermediate' in name:
+ continue
+ param.requires_grad = False
+ self.unfreeze_classification_head()
+ elif component == 'adapter':
+ for name, param in self.lm_model.named_parameters():
+ if 'adapter' in name:
+ continue
+
+ param.requires_grad = False
+ self.unfreeze_classification_head()
+ elif 'embedding' in component:
+ for name, param in self.lm_model.named_parameters():
+ if 'embedding' in name:
+ continue
+
+ param.requires_grad = False
+ self.unfreeze_classification_head()
+ elif 'bias' in component:
+ for name, param in self.lm_model.named_parameters():
+ if 'bias' in name:
+ continue
+ param.requires_grad = False
+ self.unfreeze_classification_head()
+ elif 'head' in component:
+ for name, param in self.lm_model.named_parameters():
+ param.requires_grad = False
+ self.unfreeze_classification_head()
+
+
+ elif "prompt_emb" in component:
+
+ for name, param in self.lm_model.named_parameters():
+ if 'prompt_emb' in name:
+ continue
+ param.requires_grad = False
+
+
+ def unfreeze_classification_head(self):
+ for name, param in self.lm_model.named_parameters():
+ if 'lm_head' in name or ('cls' in name) or ('classifier' in name):
+ param.requires_grad = True
+
+
+
+ def freeeze_lm_k_layers(self, k):
+
+ keep_layers = []
+ update_parameters = []
+ for i in range(k):
+ keep_layers.append('layer.'+str(23-i))
+
+ for name, param in self.lm_model.named_parameters():
+ update = False
+ for layer_num in keep_layers:
+ if layer_num in name:
+ if 'dense' in name and 'attention' not in name:
+ if 'output' in name:
+ print(name)
+ update_parameters.append(name)
+ update = True
+
+ if not update:
+ param.requires_grad = False
+ self.unfreeze_classification_head()
+
+
+ def unfreeze_lm(self):
+ for param in self.lm_model.parameters():
+ param.requires_grad = True
+
+
+ def init_embedding(self):
+
+ rand_id = torch.randint(100, self.config.vocab_size, (self.model_args.pre_seq_len,)).long()
+ rand_emb = self.lm_model.embed_encode(rand_id)
+ self.prompt_embeddings = self.prompt_embeddings.from_pretrained(rand_emb, freeze=False)
+
+
+
+ def get_adv_loss(self,
+ input_ids=None,
+ attention_mask=None,
+ mask_pos=None,
+ labels=None,
+ inputs_embeds=None):
+
+ logits, sequence_mask_output = self.encode(input_ids, attention_mask, mask_pos, inputs_embeds)
+
+ input_args = [input_ids, attention_mask, mask_pos, labels, None, 1]
+ embed = self.forward(*input_args)
+ noise = generate_noise(embed, attention_mask)
+
+ for step in range(0, self.K):
+ vat_args = [input_ids, attention_mask, mask_pos, labels, embed + noise, 2]
+ adv_logits, _ = self.forward(*vat_args)
+ adv_loss = stable_kl(adv_logits, logits.detach(), reduce=False)
+ try:
+ delta_grad, = torch.autograd.grad(adv_loss, noise, only_inputs=True, retain_graph=False)
+ except:
+ import pdb
+ pdb.set_trace()
+
+ norm = delta_grad.norm()
+ if (torch.isnan(norm) or torch.isinf(norm)):
+ return 0
+ eff_delta_grad = delta_grad * self.step_size
+ delta_grad, eff_noise = norm_grad(delta_grad, eff_grad=eff_delta_grad)
+ noise = noise + delta_grad * self.step_size
+ # noise, eff_noise = self._norm_grad(delta_grad, eff_grad=eff_delta_grad, sentence_level=self.norm_level)
+ noise = noise.detach()
+ noise.requires_grad_()
+
+ vat_args = [input_ids, attention_mask, mask_pos, labels, embed + noise, 2]
+
+ adv_logits, sequence_mask_output = self.forward(*vat_args)
+ # ori_args = model(*ori_args)
+ # aug_args = [input_ids, token_type_ids, attention_mask, premise_mask, hyp_mask, task_id, 2, (embed + native_noise).detach()]
+
+ adv_loss = self.adv_lc(adv_logits, logits, reduction='none')
+ return adv_loss
+
+ def embed_encode(self, input_ids):
+ embedding_output = self.lm_model.embeddings.word_embeddings(input_ids)
+ return embedding_output
+
+ def encode(self, input_ids=None, attention_mask=None, mask_pos=None, inputs_embeds=None, return_full_softmax=False):
+ # import pdb
+ # pdb.set_trace()
+ batch_size = input_ids.size(0)
+
+ if mask_pos is not None:
+ mask_pos = mask_pos.squeeze()
+
+ # Encode everything
+ if inputs_embeds is None:
+ outputs = self.lm_model(
+ input_ids,
+ attention_mask=attention_mask
+ )
+ else:
+ outputs = self.lm_model(
+ None,
+ attention_mask=attention_mask,
+ inputs_embeds=inputs_embeds
+ )
+
+ # Get token representation
+ sequence_output, pooled_output = outputs[:2]
+ sequence_mask_output = sequence_output[torch.arange(sequence_output.size(0)), mask_pos]
+
+ # Logits over vocabulary tokens
+ prediction_mask_scores = self.lm_head(sequence_mask_output)
+
+ # sequence_mask_output = self.lm_head.dense(sequence_mask_output)
+
+ # Exit early and only return mask logits.
+ if return_full_softmax:
+
+ return prediction_mask_scores
+
+ # Return logits for each label
+ logits = []
+ for label_id in range(len(self.label_word_list)):
+ logits.append(prediction_mask_scores[:, self.label_word_list[label_id]].unsqueeze(-1))
+ logits = torch.cat(logits, -1)
+
+ # Regression task
+ if self.config.num_labels == 1:
+ logsoftmax = nn.LogSoftmax(-1)
+ logits = logsoftmax(logits) # Log prob of right polarity
+
+ # if self.model_args.hybrid == 1:
+ # cls_logits = self.classifier(sequence_output)
+ # return (logits, cls_logits), sequence_mask_output
+
+ return logits, sequence_mask_output
+
+ # add by wjn
+ # insert soft prompt in input
+ def get_prompt(self, batch_size):
+ prefix_tokens = self.prefix_tokens.unsqueeze(0).expand(batch_size, -1).to(self.lm_model.device)
+ prompts = self.prefix_encoder(prefix_tokens)
+ return prompts
+
+ # add soft prompt via block_flag
+ def generate_continuous_prompt_inputs(self, input_ids, block_flag):
+
+
+ inputs_embeds = self.lm_model.embed_encode(input_ids)
+ bz = inputs_embeds.shape[0]
+
+ try:
+ replace_embeds = self.prompt_embeddings(
+ torch.LongTensor(list(range(self.model_args.pre_seq_len))).to(inputs_embeds.device))
+ except:
+ import pdb
+ pdb.set_trace()
+ replace_embeds = self.prompt_embeddings(
+ torch.LongTensor(list(range(self.model_args.pre_seq_len))))
+
+ replace_embeds = replace_embeds.unsqueeze(0) # [batch_size, prompt_length, embed_size]
+
+
+ if self.prompt_encoder is not None:
+ replace_embeds = self.prompt_encoder(replace_embeds)
+
+ blocked_indices = (block_flag == 1).nonzero(as_tuple=False).reshape((bz, self.model_args.pre_seq_len, 2))[:, :, 1]
+
+ for bidx in range(bz):
+ for i in range(blocked_indices.shape[1]):
+ inputs_embeds[bidx, blocked_indices[bidx, i], :] = replace_embeds[:, i, :].squeeze()
+
+ return inputs_embeds
+
+ def forward(
+ self,
+ input_ids=None,
+ attention_mask=None,
+ token_type_ids=None,
+ mask_pos=None,
+ labels=None,
+ inputs_embeds=None,
+ fwd_type=0,
+ block_flag=None,
+ *args,
+ **kwargs
+ ):
+ # print("mask_pos=", mask_pos)
+ # print("fwd_type=", fwd_type) # 0
+ batch_size = input_ids.shape[0]
+
+ if 't5' in self.config.model_type:
+ logits, sequence_mask_output = self.lm_model.encode(input_ids=input_ids, attention_mask=attention_mask, labels=labels)
+
+ else:
+
+ if fwd_type == 2:
+ assert inputs_embeds is not None
+ if token_type_ids is not None:
+ return self.lm_model.encode(input_ids=input_ids, attention_mask=attention_mask, token_type_ids=token_type_ids, mask_pos=mask_pos,
+ inputs_embeds=inputs_embeds)
+ else:
+ return self.lm_model.encode(input_ids=input_ids, attention_mask=attention_mask, mask_pos=mask_pos,
+ inputs_embeds=inputs_embeds)
+
+ elif fwd_type == 1:
+ return self.lm_model.embed_encode(input_ids)
+
+
+ # print("block_flag=", block_flag) # None
+
+ # if (self.model_args.prompt_ptuning or self.model_args.prompt_prefix) and block_flag is not None and block_flag[0] is not None:
+ # inputs_embeds = self.generate_continuous_prompt_inputs(input_ids, block_flag)
+ # print("inputs_embeds=", inputs_embeds)
+ if self.model_args.prompt_ptuning:
+
+ if inputs_embeds is None:
+ raw_embedding = self.embeddings(
+ input_ids=input_ids,
+ token_type_ids=token_type_ids,
+ )
+
+ prompts = self.get_prompt(batch_size=batch_size)
+ inputs_embeds = torch.cat((prompts, raw_embedding), dim=1)
+
+ prefix_attention_mask = torch.ones(batch_size, self.pre_seq_len).to(self.lm_model.device)
+ attention_mask = torch.cat((prefix_attention_mask, attention_mask), dim=1)
+
+
+ if fwd_type == 3:
+ if token_type_ids is not None:
+ prediction_mask_scores = self.lm_model.encode(input_ids=input_ids, attention_mask=attention_mask, token_type_ids=token_type_ids, mask_pos=mask_pos,
+ inputs_embeds=inputs_embeds, return_full_softmax=True)
+ else:
+ prediction_mask_scores = self.lm_model.encode(input_ids=input_ids, attention_mask=attention_mask, mask_pos=mask_pos, inputs_embeds=inputs_embeds, return_full_softmax=True)
+ if labels is not None:
+ return torch.zeros(1, out=prediction_mask_scores.new()), prediction_mask_scores
+ return prediction_mask_scores
+
+ if token_type_ids is not None:
+ logits, sequence_mask_output = self.lm_model.encode(input_ids=input_ids, attention_mask=attention_mask, token_type_ids=token_type_ids, mask_pos=mask_pos,
+ inputs_embeds=inputs_embeds)
+ else:
+ logits, sequence_mask_output = self.lm_model.encode(input_ids=input_ids, attention_mask=attention_mask, mask_pos=mask_pos, inputs_embeds=inputs_embeds)
+
+ if fwd_type == 4:
+ return logits, sequence_mask_output
+
+ loss = None
+ if labels is not None:
+ if self.num_labels == 1:
+ # Regression task
+ loss_fct = nn.KLDivLoss(log_target=True)
+ labels = torch.stack([1 - (labels.view(-1) - self.lb) / (self.ub - self.lb),
+ (labels.view(-1) - self.lb) / (self.ub - self.lb)], -1)
+ loss = loss_fct(logits.view(-1, 2), labels)
+ else:
+
+
+ if labels.shape == logits.shape:
+ loss = F.kl_div(F.log_softmax(logits, dim=-1, dtype=torch.float32),
+ labels, reduction='batchmean')
+ else:
+ loss_fct = nn.CrossEntropyLoss()
+
+ loss = loss_fct(logits.view(-1, logits.size(-1)), labels.view(-1))
+
+ output = (logits,)
+ if self.num_labels == 1:
+ # Regression output
+ output = (torch.exp(logits[..., 1].unsqueeze(-1)) * (self.ub - self.lb) + self.lb,)
+
+ return ((loss,) + output) if loss is not None else output
+
+
+ def from_pretrained(self, pretrained_model_name_or_path, *model_args, **kwargs):
+
+ self.lm_model = self.lm_model.from_pretrained(pretrained_model_name_or_path, *model_args, **kwargs)
+
+ if self.data_args.prompt:
+ self.lm_model.label_word_list = torch.tensor(self.data_args.label_word_list).long().cuda()
+ if output_modes_mapping[self.data_args.dataset_name] == 'regression':
+ # lower / upper bounds
+ self.lm_model.lb, self.lm_model.ub = bound_mapping[self.data_args.dataset_name]
+ self.lm_model.model_args = self.model_args
+ self.lm_model.data_args = self.data_args
+
+ return self
+
+ def load_model(self, checkpoint):
+
+ if os.path.isfile(checkpoint):
+ model_state_dict = torch.load(checkpoint)
+ self.load_state_dict(model_state_dict, strict=False)
+
+# Only prompt for BERT
+class PromptBertForSequenceClassification(LMForPromptFinetuning):
+ def __init__(self, config, model_args, data_args):
+ config.model_type = "bert"
+ model_args.prompt_only = True
+ super().__init__(config, model_args, data_args)
+
+# Prefix-tuning for BERT
+class PromptBertPrefixForSequenceClassification(LMForPromptFinetuning):
+ def __init__(self, config, model_args, data_args):
+ config.model_type = "bert"
+ model_args.prompt_prefix = True
+ super().__init__(config, model_args, data_args)
+
+# P-tuning for BERT
+class PromptBertPtuningForSequenceClassification(LMForPromptFinetuning):
+ def __init__(self, config, model_args, data_args):
+ config.model_type = "bert"
+ model_args.prompt_ptuning = True
+ super().__init__(config, model_args, data_args)
+
+# Adapter for BERT
+class PromptBertAdapterForSequenceClassification(LMForPromptFinetuning):
+ def __init__(self, config, model_args, data_args):
+ config.model_type = "bert"
+ model_args.prompt_adapter = True
+ super().__init__(config, model_args, data_args)
+
+# Prefix-tuning for RoBERTa
+class PromptRobertaPrefixForSequenceClassification(LMForPromptFinetuning):
+ def __init__(self, config, model_args, data_args):
+ config.model_type = "roberta"
+ model_args.prompt_prefix = True
+ super().__init__(config, model_args, data_args)
+
+# Only prompt for Roberta
+class PromptRobertaForSequenceClassification(LMForPromptFinetuning):
+ def __init__(self, config, model_args, data_args):
+ config.model_type = "roberta"
+ model_args.prompt_only = True
+ super().__init__(config, model_args, data_args)
+
+# P-tuning for Roberta
+class PromptRobertaPtuningForSequenceClassification(LMForPromptFinetuning):
+ def __init__(self, config, model_args, data_args):
+ config.model_type = "roberta"
+ model_args.prompt_ptuning = True
+ super().__init__(config, model_args, data_args)
+
+# Adapter for RoBERTa
+class PromptRobertaAdapterForSequenceClassification(LMForPromptFinetuning):
+ def __init__(self, config, model_args, data_args):
+ config.model_type = "roberta"
+ model_args.prompt_adapter = True
+ super().__init__(config, model_args, data_args)
+
+# Only prompt for DeBERTa
+class PromptDebertaForSequenceClassification(LMForPromptFinetuning):
+ def __init__(self, config, model_args, data_args):
+ config.model_type = "deberta"
+ model_args.prompt_only = True
+ super().__init__(config, model_args, data_args)
+
+# Prefix-tuning for DeBERTa
+class PromptDebertaPrefixForSequenceClassification(LMForPromptFinetuning):
+ def __init__(self, config, model_args, data_args):
+ config.model_type = "deberta"
+ model_args.prompt_prefix = True
+ super().__init__(config, model_args, data_args)
+
+# P-tuning for DeBERTa
+class PromptDebertaPtuningForSequenceClassification(LMForPromptFinetuning):
+ def __init__(self, config, model_args, data_args):
+ config.model_type = "deberta"
+ model_args.prompt_ptuning = True
+ super().__init__(config, model_args, data_args)
+
+# # Adapter for Deberta
+# class PromptDebertaAdapterForSequenceClassification(LMForPromptFinetuning):
+# def __init__(self, config, model_args, data_args):
+# config.model_type = "deberta"
+# model_args.prompt_adapter = True
+# super().__init__(config, model_args, data_args)
+
+# Only prompt for BERT
+class PromptDebertav2ForSequenceClassification(LMForPromptFinetuning):
+ def __init__(self, config, model_args, data_args):
+ config.model_type = "deberta-v2"
+ model_args.prompt_only = True
+ super().__init__(config, model_args, data_args)
+
+# Prefix-tuning for DeBERTa-v2
+class PromptDebertav2PrefixForSequenceClassification(LMForPromptFinetuning):
+ def __init__(self, config, model_args, data_args):
+ config.model_type = "deberta-v2"
+ model_args.prompt_prefix = True
+ super().__init__(config, model_args, data_args)
+
+# P-tuning for DeBERTa-v2
+class PromptDebertav2PtuningForSequenceClassification(LMForPromptFinetuning):
+ def __init__(self, config, model_args, data_args):
+ config.model_type = "deberta-v2"
+ model_args.prompt_ptuning = True
+ super().__init__(config, model_args, data_args)
+
+# # Adapter for Deberta-V2
+# class PromptDebertav2AdapterForSequenceClassification(LMForPromptFinetuning):
+# def __init__(self, config, model_args, data_args):
+# config.model_type = "deberta-v2"
+# model_args.prompt_adapter = True
+# super().__init__(config, model_args, data_args)
+
+
+
+# class BertForSequenceClassification(BertPreTrainedModel):
+# def __init__(self, config):
+# super().__init__(config)
+# self.num_labels = config.num_labels
+# self.config = config
+
+# self.bert = BertModel(config)
+# classifier_dropout = (
+# config.classifier_dropout if config.classifier_dropout is not None else config.hidden_dropout_prob
+# )
+# self.dropout = nn.Dropout(classifier_dropout)
+# self.classifier = nn.Linear(config.hidden_size, config.num_labels)
+
+# self.init_weights()
+
+# def forward(
+# self,
+# input_ids=None,
+# attention_mask=None,
+# token_type_ids=None,
+# position_ids=None,
+# head_mask=None,
+# inputs_embeds=None,
+# labels=None,
+# output_attentions=None,
+# output_hidden_states=None,
+# return_dict=None,
+# ):
+# r"""
+# labels (:obj:`torch.LongTensor` of shape :obj:`(batch_size,)`, `optional`):
+# Labels for computing the sequence classification/regression loss. Indices should be in :obj:`[0, ...,
+# config.num_labels - 1]`. If :obj:`config.num_labels == 1` a regression loss is computed (Mean-Square loss),
+# If :obj:`config.num_labels > 1` a classification loss is computed (Cross-Entropy).
+# """
+# return_dict = return_dict if return_dict is not None else self.config.use_return_dict
+
+# outputs = self.bert(
+# input_ids,
+# attention_mask=attention_mask,
+# token_type_ids=token_type_ids,
+# position_ids=position_ids,
+# head_mask=head_mask,
+# inputs_embeds=inputs_embeds,
+# output_attentions=output_attentions,
+# output_hidden_states=output_hidden_states,
+# return_dict=return_dict,
+# )
+
+# pooled_output = outputs[1]
+
+# pooled_output = self.dropout(pooled_output)
+# logits = self.classifier(pooled_output)
+
+# loss = None
+# if labels is not None:
+# if self.config.problem_type is None:
+# if self.num_labels == 1:
+# self.config.problem_type = "regression"
+# elif self.num_labels > 1 and (labels.dtype == torch.long or labels.dtype == torch.int):
+# self.config.problem_type = "single_label_classification"
+# else:
+# self.config.problem_type = "multi_label_classification"
+
+# if self.config.problem_type == "regression":
+# loss_fct = MSELoss()
+# if self.num_labels == 1:
+# loss = loss_fct(logits.squeeze(), labels.squeeze())
+# else:
+# loss = loss_fct(logits, labels)
+# elif self.config.problem_type == "single_label_classification":
+# loss_fct = CrossEntropyLoss()
+# loss = loss_fct(logits.view(-1, self.num_labels), labels.view(-1))
+# elif self.config.problem_type == "multi_label_classification":
+# loss_fct = BCEWithLogitsLoss()
+# loss = loss_fct(logits, labels)
+# if not return_dict:
+# output = (logits,) + outputs[2:]
+# return ((loss,) + output) if loss is not None else output
+
+# return SequenceClassifierOutput(
+# loss=loss,
+# logits=logits,
+# hidden_states=outputs.hidden_states,
+# attentions=outputs.attentions,
+# )
+
+
+class T5ForPromptFinetuning(T5ForConditionalGeneration):
+ def __init__(self, config):
+ super().__init__(config)
+
+
+ self.T5 = T5ForConditionalGeneration(config)
+
+ if self.config.use_pe:
+ self.T5 = freezer.freeze_lm(self.T5)
+
+ # These attributes should be assigned once the model is initialized
+ self.model_args = None
+ self.data_args = None
+ self.label_word_list = None
+
+ # For regression
+ self.lb = None
+ self.ub = None
+
+ # For label search.
+ self.return_full_softmax = None
+
+ def freeze_backbone(self, use_pe: bool=True):
+ if use_pe:
+ self.T5 = freezer.freeze_lm(self.T5)
+ else:
+ self.T5 = freezer.unfreeze_lm(self.T5)
+
+
+ def get_labels(self, input_ids):
+ batch_size = input_ids.size(0)
+ # new_labels = torch.tensor([3,32099,1] * batch_size).to(labels.device)
+ # prefix = torch.tensor([32099] * batch_size).to(labels.device)
+ # ending = torch.tensor([1] * batch_size).to(labels.device)
+ # prefix_labels = torch.cat((start.unsqueeze(1), prefix.unsqueeze(1)), 1)
+ # prefix_labels = torch.cat(( prefix_labels, labels.unsqueeze(1)), 1)
+ new_labels = torch.tensor([3, 32099,1]).to(input_ids.device).unsqueeze(0).repeat(batch_size, 1)
+ return new_labels
+
+
+ def encode(self, input_ids=None, attention_mask=None, token_type_ids=None, mask_pos=None, inputs_embeds=None,
+ return_full_softmax=False, labels=None):
+
+
+ if labels is not None:
+ t5_labels = self.get_labels(input_ids)
+ outputs = self.T5(input_ids=input_ids, attention_mask=attention_mask, labels=t5_labels)
+
+ prediction_mask_scores = outputs.logits[:, 2, :]
+
+ logits = []
+
+ for label_id in range(len(self.label_word_list)):
+ logits.append(prediction_mask_scores[:, self.label_word_list[label_id]].unsqueeze(-1))
+ logits = torch.cat(logits, -1)
+
+ return logits, prediction_mask_scores
+
+
+# p-tuning for bert
+class BertForPromptFinetuning(BertPreTrainedModel):
+
+ def __init__(self, config):
+ super().__init__(config)
+ self.num_labels = config.num_labels
+ self.bert = BertModel(config)
+ if self.config.use_pe:
+ self.bert = freezer.freeze_lm(self.bert)
+ self.cls = BertOnlyMLMHead(config)
+ self.init_weights()
+
+ # These attributes should be assigned once the model is initialized
+ self.model_args = None
+ self.data_args = None
+ self.label_word_list = None
+
+ # For regression
+ self.lb = None
+ self.ub = None
+ self.pre_seq_len = self.config.pre_seq_len
+ # For label search.
+ self.return_full_softmax = None
+
+ def freeze_backbone(self, use_pe: bool=True):
+ if use_pe:
+ self.bert = freezer.freeze_lm(self.bert)
+ else:
+ self.bert = freezer.unfreeze_lm(self.bert)
+
+ def embed_encode(self, input_ids):
+ embedding_output = self.bert.embeddings.word_embeddings(input_ids)
+ return embedding_output
+
+ def encode(self, input_ids=None, attention_mask=None, token_type_ids=None, mask_pos=None, inputs_embeds=None, return_full_softmax=False):
+ batch_size = input_ids.size(0)
+
+
+ if mask_pos is not None:
+ mask_pos = mask_pos.squeeze()
+
+ # Encode everything
+ if inputs_embeds is None:
+ outputs = self.bert(
+ input_ids,
+ attention_mask=attention_mask,
+ token_type_ids=token_type_ids
+ )
+ else:
+ outputs = self.bert(
+ None,
+ attention_mask=attention_mask,
+ token_type_ids=token_type_ids,
+ inputs_embeds=inputs_embeds
+ )
+
+
+ # Get token representation
+ sequence_output, pooled_output = outputs[:2]
+ sequence_output = sequence_output[:, self.pre_seq_len:, :].contiguous()
+ sequence_mask_output = sequence_output[torch.arange(sequence_output.size(0)), mask_pos]
+
+ # Logits over vocabulary tokens
+ prediction_mask_scores = self.cls(sequence_mask_output)
+
+ #sequence_mask_output = self.lm_head.dense(sequence_mask_output)
+
+ # Exit early and only return mask logits.
+ if return_full_softmax:
+ return prediction_mask_scores
+
+ # Return logits for each label
+ logits = []
+ for label_id in range(len(self.label_word_list)):
+ logits.append(prediction_mask_scores[:, self.label_word_list[label_id]].unsqueeze(-1))
+ logits = torch.cat(logits, -1)
+
+ # Regression task
+ if self.config.num_labels == 1:
+ logsoftmax = nn.LogSoftmax(-1)
+ logits = logsoftmax(logits) # Log prob of right polarity
+
+ # if self.model_args.hybrid == 1:
+ # cls_logits = self.classifier(sequence_output)
+ # return (logits, cls_logits), sequence_mask_output
+
+ return logits, sequence_mask_output
+
+
+ def forward(
+ self,
+ input_ids=None,
+ attention_mask=None,
+ token_type_ids=None,
+ mask_pos=None,
+ labels=None,
+ inputs_embeds=None,
+ fwd_type=0,
+ block_flag=None,
+ return_dict=None,
+ ):
+ if fwd_type == 2:
+ assert inputs_embeds is not None
+ return self.encode(input_ids=input_ids, attention_mask=attention_mask, token_type_ids=token_type_ids, mask_pos=mask_pos, inputs_embeds=inputs_embeds)
+
+ elif fwd_type == 1:
+ return self.embed_encode(input_ids)
+
+ if (self.model_args.prompt_ptuning or self.model_args.prompt_prefix) and block_flag is not None:
+ inputs_embeds = self.generate_continuous_prompt_inputs(input_ids, block_flag)
+
+ logits, sequence_mask_output = self.encode(input_ids, attention_mask, token_type_ids, mask_pos, inputs_embeds)
+
+ if self.model_args.hybrid == 1:
+
+ logits = logits[0]
+ cls_logits = logits[1]
+
+ loss = None
+ if labels is not None:
+ if self.num_labels == 1:
+ # Regression task
+ loss_fct = nn.KLDivLoss(log_target=True)
+ labels = torch.stack([1 - (labels.view(-1) - self.lb) / (self.ub - self.lb), (labels.view(-1) - self.lb) / (self.ub - self.lb)], -1)
+ loss = loss_fct(logits.view(-1, 2), labels)
+ else:
+
+ if labels.shape == logits.shape:
+ loss = F.kl_div(F.log_softmax(logits, dim=-1, dtype=torch.float32),
+ labels, reduction='batchmean')
+ else:
+ loss_fct = nn.CrossEntropyLoss()
+
+ loss = loss_fct(logits.view(-1, logits.size(-1)), labels.view(-1))
+
+ output = (logits,)
+ if self.num_labels == 1:
+ # Regression output
+ output = (torch.exp(logits[..., 1].unsqueeze(-1)) * (self.ub - self.lb) + self.lb,)
+
+ if not return_dict:
+ return ((loss,) + output) if loss is not None else output
+
+ return SequenceClassifierOutput(
+ loss=loss,
+ logits=logits,
+ )
+
+
+# add by wjn
+# Prefix-tuning for BERT
+class BertPrefixForPromptFinetuning(BertPreTrainedModel):
+
+ def __init__(self, config):
+ super().__init__(config)
+ self.num_labels = config.num_labels
+ self.bert = BertModel(config)
+ self.cls = BertOnlyMLMHead(config)
+ self.dropout = torch.nn.Dropout(config.hidden_dropout_prob)
+ self.init_weights()
+
+ if self.config.use_pe:
+ self.bert = freezer.freeze_lm(self.bert)
+
+ self.pre_seq_len = config.pre_seq_len
+ self.n_layer = config.num_hidden_layers
+ self.n_head = config.num_attention_heads
+ self.n_embd = config.hidden_size // config.num_attention_heads
+
+ self.prefix_tokens = torch.arange(self.pre_seq_len).long()
+ self.prefix_encoder = PrefixEncoder(config)
+
+ # These attributes should be assigned once the model is initialized
+ self.model_args = None
+ self.data_args = None
+ self.label_word_list = None
+
+ # For regression
+ self.lb = None
+ self.ub = None
+
+ # For label search.
+ self.return_full_softmax = None
+
+ def freeze_backbone(self, use_pe: bool=True):
+ if use_pe:
+ self.bert = freezer.freeze_lm(self.bert)
+ else:
+ self.bert = freezer.unfreeze_lm(self.bert)
+
+ def get_prompt(self, batch_size):
+ prefix_tokens = self.prefix_tokens.unsqueeze(0).expand(batch_size, -1).to(self.bert.device)
+ past_key_values = self.prefix_encoder(prefix_tokens)
+ # bsz, seqlen, _ = past_key_values.shape
+ past_key_values = past_key_values.view(
+ batch_size,
+ self.pre_seq_len,
+ self.n_layer * 2,
+ self.n_head,
+ self.n_embd
+ )
+ past_key_values = self.dropout(past_key_values)
+ past_key_values = past_key_values.permute([2, 0, 3, 1, 4]).split(2)
+ return past_key_values
+
+ def embed_encode(self, input_ids):
+ embedding_output = self.bert.embeddings.word_embeddings(input_ids)
+ return embedding_output
+
+ def encode(self, input_ids=None, attention_mask=None, token_type_ids=None, mask_pos=None, inputs_embeds=None, return_full_softmax=False):
+ batch_size = input_ids.size(0)
+
+ # add prefix for prompt-tuning
+ past_key_values = self.get_prompt(batch_size=batch_size)
+ prefix_attention_mask = torch.ones(batch_size, self.pre_seq_len).to(self.bert.device)
+ attention_mask = torch.cat((prefix_attention_mask, attention_mask), dim=1)
+
+ if mask_pos is not None:
+ mask_pos = mask_pos.squeeze()
+
+ # Encode everything
+
+ outputs = self.bert(
+ input_ids,
+ attention_mask=attention_mask,
+ token_type_ids=token_type_ids,
+ past_key_values=past_key_values,
+ )
+
+
+ # Get token representation
+ sequence_output, pooled_output = outputs[:2]
+ # sequence_output = sequence_output[:, self.pre_seq_len:, :].contiguous()
+ sequence_mask_output = sequence_output[torch.arange(sequence_output.size(0)), mask_pos]
+
+ # Logits over vocabulary tokens
+ prediction_mask_scores = self.cls(sequence_mask_output)
+
+ #sequence_mask_output = self.lm_head.dense(sequence_mask_output)
+
+ # Exit early and only return mask logits.
+ if return_full_softmax:
+ return prediction_mask_scores
+
+ # Return logits for each label
+ logits = []
+ for label_id in range(len(self.label_word_list)):
+ logits.append(prediction_mask_scores[:, self.label_word_list[label_id]].unsqueeze(-1))
+ logits = torch.cat(logits, -1)
+
+ # Regression task
+ if self.config.num_labels == 1:
+ logsoftmax = nn.LogSoftmax(-1)
+ logits = logsoftmax(logits) # Log prob of right polarity
+
+ # if self.model_args.hybrid == 1:
+ # cls_logits = self.classifier(sequence_output)
+ # return (logits, cls_logits), sequence_mask_output
+
+ return logits, sequence_mask_output
+
+
+ def forward(
+ self,
+ input_ids=None,
+ attention_mask=None,
+ token_type_ids=None,
+ mask_pos=None,
+ labels=None,
+ inputs_embeds=None,
+ fwd_type=0,
+ block_flag=None,
+ return_dict=None,
+ ):
+ if fwd_type == 2:
+ assert inputs_embeds is not None
+ return self.encode(input_ids=input_ids, attention_mask=attention_mask, token_type_ids=token_type_ids, mask_pos=mask_pos, inputs_embeds=inputs_embeds)
+
+ elif fwd_type == 1:
+ return self.embed_encode(input_ids)
+
+ if (self.model_args.prompt_ptuning or self.model_args.prompt_prefix) and block_flag is not None:
+ inputs_embeds = self.generate_continuous_prompt_inputs(input_ids, block_flag)
+
+ logits, sequence_mask_output = self.encode(input_ids, attention_mask, token_type_ids, mask_pos, inputs_embeds)
+
+ if self.model_args.hybrid == 1:
+
+ logits = logits[0]
+ cls_logits = logits[1]
+
+ loss = None
+ if labels is not None:
+ if self.num_labels == 1:
+ # Regression task
+ loss_fct = nn.KLDivLoss(log_target=True)
+ labels = torch.stack([1 - (labels.view(-1) - self.lb) / (self.ub - self.lb), (labels.view(-1) - self.lb) / (self.ub - self.lb)], -1)
+ loss = loss_fct(logits.view(-1, 2), labels)
+ else:
+
+ if labels.shape == logits.shape:
+ loss = F.kl_div(F.log_softmax(logits, dim=-1, dtype=torch.float32),
+ labels, reduction='batchmean')
+ else:
+ loss_fct = nn.CrossEntropyLoss()
+
+ loss = loss_fct(logits.view(-1, logits.size(-1)), labels.view(-1))
+
+ output = (logits,)
+ if self.num_labels == 1:
+ # Regression output
+ output = (torch.exp(logits[..., 1].unsqueeze(-1)) * (self.ub - self.lb) + self.lb,)
+
+ if not return_dict:
+ return ((loss,) + output) if loss is not None else output
+
+ return SequenceClassifierOutput(
+ loss=loss,
+ logits=logits,
+ )
+
+# Adapter for Bert
+class BertAdapterForPromptFinetuning(BertPreTrainedModel):
+
+ def __init__(self, config):
+ super().__init__(config)
+ self.num_labels = config.num_labels
+ self.bert = BertAdaModel(config)
+ self.cls = BertOnlyMLMHead(config)
+ self.init_weights()
+
+ if self.config.use_pe:
+ self.bert = freezer.freeze_lm_component(self.bert, "adapter")
+
+ # These attributes should be assigned once the model is initialized
+ self.model_args = None
+ self.data_args = None
+ self.label_word_list = None
+
+ # For regression
+ self.lb = None
+ self.ub = None
+
+ # For label search.
+ self.return_full_softmax = None
+
+ def freeze_backbone(self, use_pe: bool=True):
+ if use_pe:
+ self.bert = freezer.freeze_lm_component(self.bert, "adapter")
+ else:
+ self.bert = freezer.unfreeze_lm(self.bert)
+
+ def embed_encode(self, input_ids):
+ embedding_output = self.bert.embeddings.word_embeddings(input_ids)
+ return embedding_output
+
+ def encode(self, input_ids=None, attention_mask=None, token_type_ids=None, mask_pos=None, inputs_embeds=None, return_full_softmax=False):
+ batch_size = input_ids.size(0)
+
+
+ if mask_pos is not None:
+ mask_pos = mask_pos.squeeze()
+
+ # Encode everything
+ if inputs_embeds is None:
+ outputs = self.bert(
+ input_ids,
+ attention_mask=attention_mask,
+ token_type_ids=token_type_ids
+ )
+ else:
+ outputs = self.bert(
+ None,
+ attention_mask=attention_mask,
+ token_type_ids=token_type_ids,
+ inputs_embeds=inputs_embeds
+ )
+
+
+ # Get token representation
+ sequence_output, pooled_output = outputs[:2]
+ sequence_mask_output = sequence_output[torch.arange(sequence_output.size(0)), mask_pos]
+
+ # Logits over vocabulary tokens
+ prediction_mask_scores = self.cls(sequence_mask_output)
+
+ #sequence_mask_output = self.lm_head.dense(sequence_mask_output)
+
+ # Exit early and only return mask logits.
+ if return_full_softmax:
+ return prediction_mask_scores
+
+ # Return logits for each label
+ logits = []
+ for label_id in range(len(self.label_word_list)):
+ logits.append(prediction_mask_scores[:, self.label_word_list[label_id]].unsqueeze(-1))
+ logits = torch.cat(logits, -1)
+
+ # Regression task
+ if self.config.num_labels == 1:
+ logsoftmax = nn.LogSoftmax(-1)
+ logits = logsoftmax(logits) # Log prob of right polarity
+
+ # if self.model_args.hybrid == 1:
+ # cls_logits = self.classifier(sequence_output)
+ # return (logits, cls_logits), sequence_mask_output
+
+ return logits, sequence_mask_output
+
+
+ def forward(
+ self,
+ input_ids=None,
+ attention_mask=None,
+ token_type_ids=None,
+ mask_pos=None,
+ labels=None,
+ inputs_embeds=None,
+ fwd_type=0,
+ block_flag=None,
+ return_dict=None,
+ ):
+ if fwd_type == 2:
+ assert inputs_embeds is not None
+ return self.encode(input_ids=input_ids, attention_mask=attention_mask, token_type_ids=token_type_ids, mask_pos=mask_pos, inputs_embeds=inputs_embeds)
+
+ elif fwd_type == 1:
+ return self.embed_encode(input_ids)
+
+ if (self.model_args.prompt_ptuning or self.model_args.prompt_prefix) and block_flag is not None:
+ inputs_embeds = self.generate_continuous_prompt_inputs(input_ids, block_flag)
+
+ logits, sequence_mask_output = self.encode(input_ids, attention_mask, token_type_ids, mask_pos, inputs_embeds)
+
+ if self.model_args.hybrid == 1:
+
+ logits = logits[0]
+ cls_logits = logits[1]
+
+ loss = None
+ if labels is not None:
+ if self.num_labels == 1:
+ # Regression task
+ loss_fct = nn.KLDivLoss(log_target=True)
+ labels = torch.stack([1 - (labels.view(-1) - self.lb) / (self.ub - self.lb), (labels.view(-1) - self.lb) / (self.ub - self.lb)], -1)
+ loss = loss_fct(logits.view(-1, 2), labels)
+ else:
+
+ if labels.shape == logits.shape:
+ loss = F.kl_div(F.log_softmax(logits, dim=-1, dtype=torch.float32),
+ labels, reduction='batchmean')
+ else:
+ loss_fct = nn.CrossEntropyLoss()
+
+ loss = loss_fct(logits.view(-1, logits.size(-1)), labels.view(-1))
+
+ output = (logits,)
+ if self.num_labels == 1:
+ # Regression output
+ output = (torch.exp(logits[..., 1].unsqueeze(-1)) * (self.ub - self.lb) + self.lb,)
+
+ if not return_dict:
+ return ((loss,) + output) if loss is not None else output
+
+ return SequenceClassifierOutput(
+ loss=loss,
+ logits=logits,
+ )
+
+
+
+class RobertaForPromptFinetuning(RobertaPreTrainedModel):
+
+ def __init__(self, config):
+ super().__init__(config)
+ self.num_labels = config.num_labels
+ self.roberta = RobertaModel(config)
+ # self.classifier = RobertaClassificationHead(config)
+ self.lm_head = RobertaLMHead(config)
+ self.hidden_size = config.hidden_size
+
+ # self.map = nn.Linear(config.hidden_size, config.hidden_size)
+
+ if self.config.use_pe:
+ self.roberta = freezer.freeze_lm(self.roberta)
+
+ # These attributes should be assigned once the model is initialized
+ self.model_args = None
+ self.data_args = None
+ self.label_word_list = None
+ self.K = 1
+ self.step_size=1e-5
+ self.adv_lc = SymKlCriterion()
+ self.contra_lc = ContrastiveLoss()
+
+ #self.step_size=config.step_size
+
+ # For regression
+ self.lb = None
+ self.ub = None
+
+ self.tokenizer = None
+
+ self.prompt_embeddings = None
+ self.lstm_head = None
+ self.mlp_head = None
+ self.mlp = None
+
+ # For auto label search.
+ self.return_full_softmax = None
+ self.pre_seq_len = self.config.pre_seq_len
+ #self.init_weights()
+ # else:
+ # raise ValueError('unknown prompt_encoder_type.')
+
+ def freeze_backbone(self, use_pe: bool=True):
+ if use_pe:
+ self.roberta = freezer.freeze_lm(self.roberta)
+ else:
+ self.roberta = freezer.unfreeze_lm(self.roberta)
+
+ def get_constrast_loss(self,
+ input_ids=None,
+ attention_mask=None,
+ mask_pos=None,
+ labels=None,
+ inputs_embeds=None,
+ block_flag=None):
+
+ self.cos = nn.CosineSimilarity(dim=-1)
+
+ if self.model_args.prompt_ptuning or self.model_args.prompt_prefix:
+ inputs_embeds = self.generate_continuous_prompt_inputs(input_ids, block_flag)
+
+
+
+ _, sequence_mask_output_1 = self.encode(input_ids, attention_mask, mask_pos, inputs_embeds)
+ _, sequence_mask_output_2 = self.encode(input_ids, attention_mask, mask_pos, inputs_embeds)
+
+ sequence_mask_output_1= self.lm_head.dense(sequence_mask_output_1)
+ sequence_mask_output_2 = self.lm_head.dense(sequence_mask_output_2)
+ # input_args = [input_ids, attention_mask, mask_pos, labels, None, 1]
+ # embed = self.forward(*input_args)
+ #
+ # vat_args = [input_ids, attention_mask, mask_pos, labels, embed, 2]
+ #
+ # adv_logits, outputs = self.forward(*vat_args)
+ #
+ # logit_mask = F.softmax(logits, dim=-1)[torch.arange(adv_logits.size(0)), labels] > 0.7
+ #
+ # outputs = outputs[logit_mask]
+ # seq_outputs = sequence_mask_output[logit_mask]
+ # new_label = labels[logit_mask]
+ # #
+ # #
+ # rand_perm = torch.randperm(outputs.size(0))
+ # rand_outputs = outputs[rand_perm, :]
+ # rand_label = new_label[rand_perm]
+ # pair_label = (new_label == rand_label).long()
+ #
+ # seq_outputs = self.map(seq_outputs)
+ # rand_outputs = self.map(rand_outputs)
+
+ pair_labels = (labels.unsqueeze(1) == labels.unsqueeze(0)).float()
+ contra_loss = self.contra_lc(sequence_mask_output_1.unsqueeze(1), sequence_mask_output_2.unsqueeze(0), pair_labels)
+ if torch.isnan(contra_loss):
+ return 0
+
+ return contra_loss
+
+
+ def get_adv_loss(self,
+ input_ids=None,
+ attention_mask=None,
+ mask_pos=None,
+ labels=None,
+ inputs_embeds=None):
+
+ logits, sequence_mask_output = self.encode(input_ids, attention_mask, mask_pos, inputs_embeds)
+
+ input_args = [input_ids, attention_mask, mask_pos, labels, None, 1]
+ embed = self.forward(*input_args)
+ noise = generate_noise(embed, attention_mask)
+
+
+ for step in range(0, self.K):
+ vat_args = [input_ids, attention_mask, mask_pos, labels, embed + noise, 2]
+ adv_logits, _ = self.forward(*vat_args)
+ adv_loss = stable_kl(adv_logits, logits.detach(), reduce=False)
+ try:
+ delta_grad, = torch.autograd.grad(adv_loss, noise, only_inputs=True, retain_graph=False)
+ except:
+ import pdb
+ pdb.set_trace()
+
+ norm = delta_grad.norm()
+ if (torch.isnan(norm) or torch.isinf(norm)):
+ return 0
+ eff_delta_grad = delta_grad * self.step_size
+ delta_grad, eff_noise = norm_grad(delta_grad, eff_grad=eff_delta_grad)
+ noise = noise + delta_grad * self.step_size
+ # noise, eff_noise = self._norm_grad(delta_grad, eff_grad=eff_delta_grad, sentence_level=self.norm_level)
+ noise = noise.detach()
+ noise.requires_grad_()
+
+
+ vat_args = [input_ids, attention_mask, mask_pos, labels, embed + noise, 2]
+
+ adv_logits, sequence_mask_output = self.forward(*vat_args)
+ # ori_args = model(*ori_args)
+ # aug_args = [input_ids, token_type_ids, attention_mask, premise_mask, hyp_mask, task_id, 2, (embed + native_noise).detach()]
+
+ adv_loss = self.adv_lc(adv_logits, logits, reduction='none')
+ return adv_loss
+
+ def embed_encode(self, input_ids):
+ embedding_output = self.roberta.embeddings.word_embeddings(input_ids)
+ return embedding_output
+
+ def encode(self, input_ids=None, attention_mask=None, mask_pos=None, inputs_embeds=None, return_full_softmax=False):
+ batch_size = input_ids.size(0)
+
+ if mask_pos is not None:
+ mask_pos = mask_pos.squeeze()
+
+ # Encode everything
+ if inputs_embeds is None:
+ outputs = self.roberta(
+ input_ids,
+ attention_mask=attention_mask
+ )
+ else:
+ outputs = self.roberta(
+ None,
+ attention_mask=attention_mask,
+ inputs_embeds=inputs_embeds
+ )
+
+
+ # Get token representation
+ sequence_output, pooled_output = outputs[:2]
+ sequence_output = sequence_output[:, self.pre_seq_len:, :].contiguous()
+ sequence_mask_output = sequence_output[torch.arange(sequence_output.size(0)), mask_pos]
+
+
+
+ # Logits over vocabulary tokens
+ prediction_mask_scores = self.lm_head(sequence_mask_output)
+
+ #sequence_mask_output = self.lm_head.dense(sequence_mask_output)
+
+ # Exit early and only return mask logits.
+ if return_full_softmax:
+ return prediction_mask_scores
+
+ # Return logits for each label
+ logits = []
+
+ for label_id in range(len(self.label_word_list)):
+ # print("label_id=", label_id)
+ # print("self.label_word_list=", self.label_word_list)
+ # print("prediction_mask_scores.shape=", prediction_mask_scores.shape)
+ logits.append(prediction_mask_scores[:, self.label_word_list[label_id]].unsqueeze(-1))
+ logits = torch.cat(logits, -1)
+
+ # Regression task
+ if self.config.num_labels == 1:
+ logsoftmax = nn.LogSoftmax(-1)
+ logits = logsoftmax(logits) # Log prob of right polarity
+
+ # if self.model_args.hybrid == 1:
+ # cls_logits = self.classifier(sequence_output)
+ # return (logits, cls_logits), sequence_mask_output
+
+
+ return logits, sequence_mask_output
+
+ def generate_continuous_prompt_inputs(self, input_ids, block_flag):
+
+ inputs_embeds = self.embed_encode(input_ids)
+ bz = inputs_embeds.shape[0]
+
+ try:
+ replace_embeds = self.prompt_embeddings(
+ torch.LongTensor(list(range(1))).to(inputs_embeds.device))
+ except:
+ import pdb
+ pdb.set_trace()
+ replace_embeds = self.prompt_embeddings(
+ torch.LongTensor(list(range(1))))
+
+ replace_embeds = replace_embeds.unsqueeze(0) # [batch_size, prompt_length, embed_size]
+
+ # if self.model_args.prompt_encoder_type == "lstm":
+ # replace_embeds = self.lstm_head(replace_embeds)[0] # [batch_size, seq_len, 2 * hidden_dim]
+ # if self.prompt_length == 1:
+ # replace_embeds = self.mlp_head(replace_embeds)
+ # else:
+ # replace_embeds = self.mlp_head(replace_embeds).squeeze()
+
+ # elif self.model_args.prompt_encoder_type == "mlp":
+ replace_embeds = self.mlp(replace_embeds)
+ # else:
+ # raise ValueError("unknown prompt_encoder_type.")
+
+ blocked_indices = (block_flag == 1).nonzero(as_tuple=False).reshape((bz, self.model_args.prompt_length, 2))[:, :, 1]
+
+ for bidx in range(bz):
+ for i in range(blocked_indices.shape[1]):
+ inputs_embeds[bidx, blocked_indices[bidx, i], :] = replace_embeds[i, :]
+
+ return inputs_embeds
+
+
+
+ def forward(
+ self,
+ input_ids=None,
+ attention_mask=None,
+ mask_pos=None,
+ labels=None,
+ inputs_embeds=None,
+ fwd_type=0,
+ block_flag=None,
+ return_dict=None
+ ):
+ if fwd_type == 2:
+ assert inputs_embeds is not None
+ return self.encode(input_ids=input_ids, attention_mask=attention_mask, mask_pos=mask_pos, inputs_embeds=inputs_embeds)
+
+ elif fwd_type == 1:
+ return self.embed_encode(input_ids)
+
+ if (self.model_args.prompt_ptuning or self.model_args.prompt_prefix) and block_flag is not None:
+ inputs_embeds = self.generate_continuous_prompt_inputs(input_ids, block_flag)
+
+ logits, sequence_mask_output = self.encode(input_ids, attention_mask, mask_pos, inputs_embeds)
+
+ if self.model_args.hybrid == 1:
+
+ logits = logits[0]
+ cls_logits = logits[1]
+
+ loss = None
+ if labels is not None:
+ if self.num_labels == 1:
+ # Regression task
+ loss_fct = nn.KLDivLoss(log_target=True)
+ labels = torch.stack([1 - (labels.view(-1) - self.lb) / (self.ub - self.lb), (labels.view(-1) - self.lb) / (self.ub - self.lb)], -1)
+ loss = loss_fct(logits.view(-1, 2), labels)
+ else:
+
+ if labels.shape == logits.shape:
+ loss = F.kl_div(F.log_softmax(logits, dim=-1, dtype=torch.float32),
+ labels, reduction='batchmean')
+ else:
+ loss_fct = nn.CrossEntropyLoss()
+
+ loss = loss_fct(logits.view(-1, logits.size(-1)), labels.view(-1))
+
+ output = (logits,)
+ if self.num_labels == 1:
+ # Regression output
+ output = (torch.exp(logits[..., 1].unsqueeze(-1)) * (self.ub - self.lb) + self.lb,)
+
+ if not return_dict:
+ return ((loss,) + output) if loss is not None else output
+
+ return SequenceClassifierOutput(
+ loss=loss,
+ logits=logits,
+ )
+
+
+
+# add by wjn
+# Prefix-tuning for RoBERTa
+class RobertPrefixForPromptFinetuning(RobertaPreTrainedModel):
+
+ def __init__(self, config):
+ super().__init__(config)
+ self.num_labels = config.num_labels
+ self.roberta = RobertaModel(config)
+ self.cls = BertOnlyMLMHead(config)
+ self.dropout = torch.nn.Dropout(config.hidden_dropout_prob)
+ self.init_weights()
+
+ if self.config.use_pe:
+ self.roberta = freezer.freeze_lm(self.roberta)
+
+ self.pre_seq_len = config.pre_seq_len
+ self.n_layer = config.num_hidden_layers
+ self.n_head = config.num_attention_heads
+ self.n_embd = config.hidden_size // config.num_attention_heads
+
+ self.prefix_tokens = torch.arange(self.pre_seq_len).long()
+ self.prefix_encoder = PrefixEncoder(config)
+
+ # These attributes should be assigned once the model is initialized
+ self.model_args = None
+ self.data_args = None
+ self.label_word_list = None
+
+ # For regression
+ self.lb = None
+ self.ub = None
+
+ # For label search.
+ self.return_full_softmax = None
+
+ def freeze_backbone(self, use_pe: bool=True):
+ if use_pe:
+ self.roberta = freezer.freeze_lm(self.roberta)
+ else:
+ self.roberta = freezer.unfreeze_lm(self.roberta)
+
+ def get_prompt(self, batch_size):
+ prefix_tokens = self.prefix_tokens.unsqueeze(0).expand(batch_size, -1).to(self.roberta.device)
+ past_key_values = self.prefix_encoder(prefix_tokens)
+ # bsz, seqlen, _ = past_key_values.shape
+ past_key_values = past_key_values.view(
+ batch_size,
+ self.pre_seq_len,
+ self.n_layer * 2,
+ self.n_head,
+ self.n_embd
+ )
+ past_key_values = self.dropout(past_key_values)
+ past_key_values = past_key_values.permute([2, 0, 3, 1, 4]).split(2)
+ return past_key_values
+
+ def embed_encode(self, input_ids):
+ embedding_output = self.roberta.embeddings.word_embeddings(input_ids)
+ return embedding_output
+
+ def encode(self, input_ids=None, attention_mask=None, token_type_ids=None, mask_pos=None, inputs_embeds=None, return_full_softmax=False):
+ batch_size = input_ids.size(0)
+
+ # add prefix for prompt-tuning
+ past_key_values = self.get_prompt(batch_size=batch_size)
+ prefix_attention_mask = torch.ones(batch_size, self.pre_seq_len).to(self.roberta.device)
+ attention_mask = torch.cat((prefix_attention_mask, attention_mask), dim=1)
+
+ if mask_pos is not None:
+ mask_pos = mask_pos.squeeze()
+
+ # Encode everything
+ # print("input_ids.shape=", input_ids.shape)
+ # print("token_type_ids.shape=", token_type_ids.shape)
+
+ outputs = self.roberta(
+ input_ids,
+ attention_mask=attention_mask,
+ token_type_ids=token_type_ids,
+ past_key_values=past_key_values,
+ )
+
+
+ # Get token representation
+ sequence_output, pooled_output = outputs[:2]
+ # print("sequence_output.shape=", sequence_output.shape)
+ # sequence_output = sequence_output[:, self.pre_seq_len:, :].contiguous()
+ # print("sequence_output.size(0))=", sequence_output.size(0))
+ # print("mask_pos.shape=", mask_pos.shape)
+ # print("input_ids[mask_pos]=", input_ids[torch.arange(sequence_output.size(0)), mask_pos])
+ sequence_mask_output = sequence_output[torch.arange(sequence_output.size(0)), mask_pos]
+
+ # Logits over vocabulary tokens
+ prediction_mask_scores = self.cls(sequence_mask_output)
+
+ #sequence_mask_output = self.lm_head.dense(sequence_mask_output)
+
+ # Exit early and only return mask logits.
+ if return_full_softmax:
+ return prediction_mask_scores
+
+ # Return logits for each label
+ logits = []
+ # print("prediction_mask_scores.shape=", prediction_mask_scores.shape)
+ # print("self.label_word_list=", self.label_word_list)
+ for label_id in range(len(self.label_word_list)):
+ # print("label_id=", label_id)
+ logits.append(prediction_mask_scores[:, self.label_word_list[label_id]].unsqueeze(-1))
+ logits = torch.cat(logits, -1)
+ # print("logits=", logits)
+
+ # Regression task
+ if self.config.num_labels == 1:
+ logsoftmax = nn.LogSoftmax(-1)
+ logits = logsoftmax(logits) # Log prob of right polarity
+
+ # if self.model_args.hybrid == 1:
+ # cls_logits = self.classifier(sequence_output)
+ # return (logits, cls_logits), sequence_mask_output
+
+ return logits, sequence_mask_output
+
+
+ def forward(
+ self,
+ input_ids=None,
+ attention_mask=None,
+ token_type_ids=None,
+ mask_pos=None,
+ labels=None,
+ inputs_embeds=None,
+ fwd_type=0,
+ block_flag=None,
+ return_dict=None,
+ ):
+ # print("token_type_ids.shape=", token_type_ids.shape)
+ # print("token_type_ids=", token_type_ids)
+ if fwd_type == 2:
+ assert inputs_embeds is not None
+ return self.encode(input_ids=input_ids, attention_mask=attention_mask, token_type_ids=token_type_ids, mask_pos=mask_pos, inputs_embeds=inputs_embeds)
+
+ elif fwd_type == 1:
+ return self.embed_encode(input_ids)
+
+ if (self.model_args.prompt_ptuning or self.model_args.prompt_prefix) and block_flag is not None:
+ inputs_embeds = self.generate_continuous_prompt_inputs(input_ids, block_flag)
+
+ logits, sequence_mask_output = self.encode(input_ids, attention_mask, token_type_ids, mask_pos, inputs_embeds)
+
+ if self.model_args.hybrid == 1:
+
+ logits = logits[0]
+ cls_logits = logits[1]
+
+ loss = None
+ if labels is not None:
+ if self.num_labels == 1:
+ # Regression task
+ loss_fct = nn.KLDivLoss(log_target=True)
+ labels = torch.stack([1 - (labels.view(-1) - self.lb) / (self.ub - self.lb), (labels.view(-1) - self.lb) / (self.ub - self.lb)], -1)
+ loss = loss_fct(logits.view(-1, 2), labels)
+ else:
+
+ if labels.shape == logits.shape:
+ loss = F.kl_div(F.log_softmax(logits, dim=-1, dtype=torch.float32),
+ labels, reduction='batchmean')
+ else:
+ loss_fct = nn.CrossEntropyLoss()
+
+ loss = loss_fct(logits.view(-1, logits.size(-1)), labels.view(-1))
+
+ output = (logits,)
+ if self.num_labels == 1:
+ # Regression output
+ output = (torch.exp(logits[..., 1].unsqueeze(-1)) * (self.ub - self.lb) + self.lb,)
+
+ if not return_dict:
+ return ((loss,) + output) if loss is not None else output
+
+ return SequenceClassifierOutput(
+ loss=loss,
+ logits=logits,
+ )
+
+# Adapter for RoBERTa
+class RobertaAdapterForPromptFinetuning(RobertaPreTrainedModel):
+
+ def __init__(self, config):
+ super().__init__(config)
+ self.num_labels = config.num_labels
+ self.roberta = RobertaAdaModel(config)
+ # self.classifier = RobertaClassificationHead(config)
+ self.lm_head = RobertaLMHead(config)
+ self.hidden_size = config.hidden_size
+
+ # self.map = nn.Linear(config.hidden_size, config.hidden_size)
+
+
+ # These attributes should be assigned once the model is initialized
+ self.model_args = None
+ self.data_args = None
+ self.label_word_list = None
+ self.K = 1
+ self.step_size=1e-5
+ self.adv_lc = SymKlCriterion()
+ self.contra_lc = ContrastiveLoss()
+
+ #self.step_size=config.step_size
+
+ # For regression
+ self.lb = None
+ self.ub = None
+
+ self.tokenizer = None
+
+ self.prompt_embeddings = None
+ self.lstm_head = None
+ self.mlp_head = None
+ self.mlp = None
+
+ # For auto label search.
+ self.return_full_softmax = None
+
+ self.init_weights()
+ if self.config.use_pe:
+ self.roberta = freezer.freeze_lm_component(self.roberta, "adapter")
+ # else:
+ # raise ValueError('unknown prompt_encoder_type.')
+
+ def freeze_backbone(self, use_pe: bool=True):
+ if use_pe:
+ self.roberta = freezer.freeze_lm_component(self.roberta, "adapter")
+ else:
+ self.roberta = freezer.unfreeze_lm(self.roberta)
+
+
+ def get_constrast_loss(self,
+ input_ids=None,
+ attention_mask=None,
+ mask_pos=None,
+ labels=None,
+ inputs_embeds=None,
+ block_flag=None):
+
+ self.cos = nn.CosineSimilarity(dim=-1)
+
+ if self.model_args.prompt_ptuning or self.model_args.prompt_prefix:
+ inputs_embeds = self.generate_continuous_prompt_inputs(input_ids, block_flag)
+
+
+
+ _, sequence_mask_output_1 = self.encode(input_ids, attention_mask, mask_pos, inputs_embeds)
+ _, sequence_mask_output_2 = self.encode(input_ids, attention_mask, mask_pos, inputs_embeds)
+
+ sequence_mask_output_1= self.lm_head.dense(sequence_mask_output_1)
+ sequence_mask_output_2 = self.lm_head.dense(sequence_mask_output_2)
+ # input_args = [input_ids, attention_mask, mask_pos, labels, None, 1]
+ # embed = self.forward(*input_args)
+ #
+ # vat_args = [input_ids, attention_mask, mask_pos, labels, embed, 2]
+ #
+ # adv_logits, outputs = self.forward(*vat_args)
+ #
+ # logit_mask = F.softmax(logits, dim=-1)[torch.arange(adv_logits.size(0)), labels] > 0.7
+ #
+ # outputs = outputs[logit_mask]
+ # seq_outputs = sequence_mask_output[logit_mask]
+ # new_label = labels[logit_mask]
+ # #
+ # #
+ # rand_perm = torch.randperm(outputs.size(0))
+ # rand_outputs = outputs[rand_perm, :]
+ # rand_label = new_label[rand_perm]
+ # pair_label = (new_label == rand_label).long()
+ #
+ # seq_outputs = self.map(seq_outputs)
+ # rand_outputs = self.map(rand_outputs)
+
+ pair_labels = (labels.unsqueeze(1) == labels.unsqueeze(0)).float()
+ contra_loss = self.contra_lc(sequence_mask_output_1.unsqueeze(1), sequence_mask_output_2.unsqueeze(0), pair_labels)
+ if torch.isnan(contra_loss):
+ return 0
+
+ return contra_loss
+
+
+ def get_adv_loss(self,
+ input_ids=None,
+ attention_mask=None,
+ mask_pos=None,
+ labels=None,
+ inputs_embeds=None):
+
+ logits, sequence_mask_output = self.encode(input_ids, attention_mask, mask_pos, inputs_embeds)
+
+ input_args = [input_ids, attention_mask, mask_pos, labels, None, 1]
+ embed = self.forward(*input_args)
+ noise = generate_noise(embed, attention_mask)
+
+
+ for step in range(0, self.K):
+ vat_args = [input_ids, attention_mask, mask_pos, labels, embed + noise, 2]
+ adv_logits, _ = self.forward(*vat_args)
+ adv_loss = stable_kl(adv_logits, logits.detach(), reduce=False)
+ try:
+ delta_grad, = torch.autograd.grad(adv_loss, noise, only_inputs=True, retain_graph=False)
+ except:
+ import pdb
+ pdb.set_trace()
+
+ norm = delta_grad.norm()
+ if (torch.isnan(norm) or torch.isinf(norm)):
+ return 0
+ eff_delta_grad = delta_grad * self.step_size
+ delta_grad, eff_noise = norm_grad(delta_grad, eff_grad=eff_delta_grad)
+ noise = noise + delta_grad * self.step_size
+ # noise, eff_noise = self._norm_grad(delta_grad, eff_grad=eff_delta_grad, sentence_level=self.norm_level)
+ noise = noise.detach()
+ noise.requires_grad_()
+
+
+ vat_args = [input_ids, attention_mask, mask_pos, labels, embed + noise, 2]
+
+ adv_logits, sequence_mask_output = self.forward(*vat_args)
+ # ori_args = model(*ori_args)
+ # aug_args = [input_ids, token_type_ids, attention_mask, premise_mask, hyp_mask, task_id, 2, (embed + native_noise).detach()]
+
+ adv_loss = self.adv_lc(adv_logits, logits, reduction='none')
+ return adv_loss
+
+ def embed_encode(self, input_ids):
+ embedding_output = self.roberta.embeddings.word_embeddings(input_ids)
+ return embedding_output
+
+ def encode(self, input_ids=None, attention_mask=None, mask_pos=None, inputs_embeds=None, return_full_softmax=False):
+ batch_size = input_ids.size(0)
+
+ if mask_pos is not None:
+ mask_pos = mask_pos.squeeze()
+
+ # Encode everything
+ if inputs_embeds is None:
+ outputs = self.roberta(
+ input_ids,
+ attention_mask=attention_mask
+ )
+ else:
+ outputs = self.roberta(
+ None,
+ attention_mask=attention_mask,
+ inputs_embeds=inputs_embeds
+ )
+
+ # print("mask_pos.shape=", mask_pos.shape)
+ # Get token representation
+ sequence_output, pooled_output = outputs[:2]
+ sequence_mask_output = sequence_output[torch.arange(sequence_output.size(0)), mask_pos]
+
+
+ # print("sequence_mask_output.shape=", sequence_mask_output.shape) # torch.Size([4, 1, 128, 1024])
+ # Logits over vocabulary tokens
+ prediction_mask_scores = self.lm_head(sequence_mask_output)
+
+ #sequence_mask_output = self.lm_head.dense(sequence_mask_output)
+
+ # Exit early and only return mask logits.
+ if return_full_softmax:
+ return prediction_mask_scores
+
+ # Return logits for each label
+ logits = []
+ # print("self.label_word_list=", self.label_word_list) # e.g., tensor([ 7447, 35297], device='cuda:0')
+ for label_id in range(len(self.label_word_list)):
+ # print("label_id=", label_id) # e.g., 0
+ # print("self.label_word_list[label_id]=", self.label_word_list[label_id]) # e.g., tensor(7447, device='cuda:0')
+ # print("prediction_mask_scores.shape=", prediction_mask_scores.shape) # [4, 1, 128, 50265] [bz, 1, seq_len, vocab_size]
+ logits.append(prediction_mask_scores[:, self.label_word_list[label_id]].unsqueeze(-1))
+ logits = torch.cat(logits, -1)
+ # print("logits.shape=", logits.shape) # torch.Size([4, 1, 128, 2])
+ # Regression task
+ if self.config.num_labels == 1:
+ logsoftmax = nn.LogSoftmax(-1)
+ logits = logsoftmax(logits) # Log prob of right polarity
+
+ # if self.model_args.hybrid == 1:
+ # cls_logits = self.classifier(sequence_output)
+ # return (logits, cls_logits), sequence_mask_output
+
+
+ return logits, sequence_mask_output
+
+ def generate_continuous_prompt_inputs(self, input_ids, block_flag):
+
+ inputs_embeds = self.embed_encode(input_ids)
+ bz = inputs_embeds.shape[0]
+
+ try:
+ replace_embeds = self.prompt_embeddings(
+ torch.LongTensor(list(range(1))).to(inputs_embeds.device))
+ except:
+ import pdb
+ pdb.set_trace()
+ replace_embeds = self.prompt_embeddings(
+ torch.LongTensor(list(range(1))))
+
+ replace_embeds = replace_embeds.unsqueeze(0) # [batch_size, prompt_length, embed_size]
+
+ # if self.model_args.prompt_encoder_type == "lstm":
+ # replace_embeds = self.lstm_head(replace_embeds)[0] # [batch_size, seq_len, 2 * hidden_dim]
+ # if self.prompt_length == 1:
+ # replace_embeds = self.mlp_head(replace_embeds)
+ # else:
+ # replace_embeds = self.mlp_head(replace_embeds).squeeze()
+
+ # elif self.model_args.prompt_encoder_type == "mlp":
+ replace_embeds = self.mlp(replace_embeds)
+ # else:
+ # raise ValueError("unknown prompt_encoder_type.")
+
+ blocked_indices = (block_flag == 1).nonzero(as_tuple=False).reshape((bz, self.model_args.prompt_length, 2))[:, :, 1]
+
+ for bidx in range(bz):
+ for i in range(blocked_indices.shape[1]):
+ inputs_embeds[bidx, blocked_indices[bidx, i], :] = replace_embeds[i, :]
+
+ return inputs_embeds
+
+
+
+ def forward(
+ self,
+ input_ids=None,
+ attention_mask=None,
+ mask_pos=None,
+ labels=None,
+ inputs_embeds=None,
+ fwd_type=0,
+ block_flag=None,
+ return_dict=None
+ ):
+ if fwd_type == 2:
+ assert inputs_embeds is not None
+ return self.encode(input_ids=input_ids, attention_mask=attention_mask, mask_pos=mask_pos, inputs_embeds=inputs_embeds)
+
+ elif fwd_type == 1:
+ return self.embed_encode(input_ids)
+
+ if (self.model_args.prompt_ptuning or self.model_args.prompt_prefix) and block_flag is not None:
+ inputs_embeds = self.generate_continuous_prompt_inputs(input_ids, block_flag)
+
+ logits, sequence_mask_output = self.encode(input_ids, attention_mask, mask_pos, inputs_embeds)
+
+ if self.model_args.hybrid == 1:
+
+ logits = logits[0]
+ cls_logits = logits[1]
+
+ loss = None
+ if labels is not None:
+ if self.num_labels == 1:
+ # Regression task
+ loss_fct = nn.KLDivLoss(log_target=True)
+ labels = torch.stack([1 - (labels.view(-1) - self.lb) / (self.ub - self.lb), (labels.view(-1) - self.lb) / (self.ub - self.lb)], -1)
+ loss = loss_fct(logits.view(-1, 2), labels)
+ else:
+
+ if labels.shape == logits.shape:
+ loss = F.kl_div(F.log_softmax(logits, dim=-1, dtype=torch.float32),
+ labels, reduction='batchmean')
+ else:
+ loss_fct = nn.CrossEntropyLoss()
+
+ loss = loss_fct(logits.view(-1, logits.size(-1)), labels.view(-1))
+
+ output = (logits,)
+ if self.num_labels == 1:
+ # Regression output
+ output = (torch.exp(logits[..., 1].unsqueeze(-1)) * (self.ub - self.lb) + self.lb,)
+
+ if not return_dict:
+ return ((loss,) + output) if loss is not None else output
+
+ return SequenceClassifierOutput(
+ loss=loss,
+ logits=logits,
+ )
+
+
+
+
+class RobertaForSequenceClassification(RobertaPreTrainedModel):
+ _keys_to_ignore_on_load_missing = [r"position_ids"]
+
+ def __init__(self, config):
+ super().__init__(config)
+ self.num_labels = config.num_labels
+ self.config = config
+
+ self.roberta = RobertaModel(config, add_pooling_layer=False)
+
+ if self.config.use_pe:
+ self.roberta = freezer.freeze_lm(self.roberta)
+
+ #self.roberta = RobertaModel(config, add_pooling_layer=False)
+ self.classifier = RobertaClassificationHead(config)
+
+ self.init_weights()
+
+ def freeze_backbone(self, use_pe: bool=True):
+ if use_pe:
+ self.roberta = freezer.freeze_lm(self.roberta)
+ else:
+ self.roberta = freezer.unfreeze_lm(self.roberta)
+
+
+ def freeze_lm_component(self, component):
+
+ if 'attention' in component:
+ for name, param in self.roberta.named_parameters():
+ if 'attention' in name:
+ if 'output' in component:
+ if 'output' in name:
+ continue
+ else:
+ continue
+ param.requires_grad = False
+ elif 'feedforward' in component:
+ for name, param in self.roberta.named_parameters():
+ if 'dense' in name and 'attention' not in name:
+ if 'output' in component:
+ if 'output' in name:
+ continue
+ else:
+ if 'intermediate' in component:
+ if 'intermediate' in name:
+ continue
+ param.requires_grad = False
+ elif component == 'adapter':
+ for name, param in self.roberta.named_parameters():
+ if 'adapter' in name:
+ continue
+
+ param.requires_grad = False
+ elif 'embedding' in component:
+ for name, param in self.roberta.named_parameters():
+ if 'embedding' in name:
+ continue
+ # if 'lm_head' in name:
+ #
+ # if 'output' in name:
+ # continue
+
+ param.requires_grad = False
+ elif 'bias' in component:
+ for name, param in self.roberta.named_parameters():
+ if 'bias' in name:
+ continue
+ # if 'lm_head' in name:
+ #
+ # if 'output' in name:
+ # continue
+
+ param.requires_grad = False
+ elif 'head' in component:
+ for name, param in self.roberta.named_parameters():
+ param.requires_grad = False
+
+
+
+ self.unfreeze_classification_head()
+
+ def unfreeze_classification_head(self):
+ for name, param in self.roberta.named_parameters():
+ if 'lm_head' in name or ('cls' in name) or ('classifier' in name):
+ param.requires_grad = True
+
+
+ def forward(
+ self,
+ input_ids=None,
+ attention_mask=None,
+ token_type_ids=None,
+ position_ids=None,
+ head_mask=None,
+ inputs_embeds=None,
+ labels=None,
+ output_attentions=None,
+ output_hidden_states=None,
+ return_dict=None,
+ ):
+ r"""
+ labels (:obj:`torch.LongTensor` of shape :obj:`(batch_size,)`, `optional`):
+ Labels for computing the sequence classification/regression loss. Indices should be in :obj:`[0, ...,
+ config.num_labels - 1]`. If :obj:`config.num_labels == 1` a regression loss is computed (Mean-Square loss),
+ If :obj:`config.num_labels > 1` a classification loss is computed (Cross-Entropy).
+ """
+ return_dict = return_dict if return_dict is not None else self.config.use_return_dict
+
+ outputs = self.roberta(
+ input_ids,
+ attention_mask=attention_mask,
+ token_type_ids=token_type_ids,
+ position_ids=position_ids,
+ head_mask=head_mask,
+ inputs_embeds=inputs_embeds,
+ output_attentions=output_attentions,
+ output_hidden_states=output_hidden_states,
+ return_dict=return_dict,
+ )
+ sequence_output = outputs[0]
+ logits = self.classifier(sequence_output)
+
+ loss = None
+
+ loss_fct = nn.CrossEntropyLoss()
+ loss = loss_fct(logits.view(-1, self.num_labels), labels.view(-1))
+
+
+
+ #output = (logits,) + outputs[2:]
+ output = (logits,)
+
+ if not return_dict:
+ return ((loss,) + output) if loss is not None else output
+
+ return SequenceClassifierOutput(
+ loss=loss,
+ logits=logits,
+ )
+
+
+
+class DebertaForPromptFinetuning(DebertaPreTrainedModel):
+ _keys_to_ignore_on_load_unexpected = [r"pooler"]
+ _keys_to_ignore_on_load_missing = [r"position_ids", r"predictions.decoder.bias"]
+
+ def __init__(self, config):
+ super().__init__(config)
+ self.num_labels = config.num_labels
+ #self.deberta = DebertaV2Model(config)
+
+ self.deberta = DebertaModel(config)
+ self.cls = DebertaOnlyMLMHead(config)
+
+ if self.config.use_pe:
+ self.deberta = freezer.freeze_lm(self.deberta)
+
+ self.pooler = ContextPooler(config)
+ output_dim = self.pooler.output_dim
+
+ self.classifier = torch.nn.Linear(output_dim, self.num_labels)
+ drop_out = getattr(config, "cls_dropout", None)
+ drop_out = self.config.hidden_dropout_prob if drop_out is None else drop_out
+
+ self.dropout = StableDropout(drop_out)
+
+ classification_list = [self.pooler, self.dropout,self.classifier]
+
+ self.classifier = nn.Sequential(*classification_list)
+ # self.cls = DebertaV2OnlyMLMHead(config)
+
+ self.map = nn.Linear(config.hidden_size, config.hidden_size)
+ self.init_weights()
+
+ # These attributes should be assigned once the model is initialized
+ self.model_args = None
+ self.data_args = None
+ self.label_word_list = None
+ self.K = 1
+ self.step_size=1e-5
+ self.adv_lc = SymKlCriterion()
+ self.contra_lc = ContrastiveLoss()
+ # import pdb
+ # pdb.set_trace()
+ #self.step_size=config.step_size
+
+ # For regression
+ self.lb = None
+ self.ub = None
+
+ self.pre_seq_len = self.config.pre_seq_len
+ # For auto label search.
+ self.return_full_softmax = None
+
+ def freeze_backbone(self, use_pe: bool=True):
+ if use_pe:
+ self.deberta = freezer.freeze_lm(self.deberta)
+ else:
+ self.deberta = freezer.unfreeze_lm(self.deberta)
+
+ def get_constrast_loss(self,
+ input_ids=None,
+ attention_mask=None,
+ mask_pos=None,
+ labels=None,
+ inputs_embeds=None):
+
+ self.cos = nn.CosineSimilarity(dim=-1)
+
+
+ _, sequence_mask_output_1 = self.encode(input_ids, attention_mask, mask_pos, inputs_embeds)
+ _, sequence_mask_output_2 = self.encode(input_ids, attention_mask, mask_pos, inputs_embeds)
+
+ sequence_mask_output_1= self.lm_head.dense(sequence_mask_output_1)
+ sequence_mask_output_2 = self.lm_head.dense(sequence_mask_output_2)
+ # input_args = [input_ids, attention_mask, mask_pos, labels, None, 1]
+ # embed = self.forward(*input_args)
+ #
+ # vat_args = [input_ids, attention_mask, mask_pos, labels, embed, 2]
+ #
+ # adv_logits, outputs = self.forward(*vat_args)
+ #
+ # logit_mask = F.softmax(logits, dim=-1)[torch.arange(adv_logits.size(0)), labels] > 0.7
+ #
+ # outputs = outputs[logit_mask]
+ # seq_outputs = sequence_mask_output[logit_mask]
+ # new_label = labels[logit_mask]
+ # #
+ # #
+ # rand_perm = torch.randperm(outputs.size(0))
+ # rand_outputs = outputs[rand_perm, :]
+ # rand_label = new_label[rand_perm]
+ # pair_label = (new_label == rand_label).long()
+ #
+ # seq_outputs = self.map(seq_outputs)
+ # rand_outputs = self.map(rand_outputs)
+
+ pair_labels = (labels.unsqueeze(1) == labels.unsqueeze(0)).float()
+
+ # import pdb
+ # pdb.set_trace()
+
+
+ contra_loss = self.contra_lc(sequence_mask_output_1.unsqueeze(1), sequence_mask_output_2.unsqueeze(0), pair_labels)
+
+
+
+ if torch.isnan(contra_loss):
+ return 0
+
+ return contra_loss
+
+
+ def get_adv_loss(self,
+ input_ids=None,
+ attention_mask=None,
+ mask_pos=None,
+ labels=None,
+ inputs_embeds=None):
+
+ logits, sequence_mask_output = self.encode(input_ids, attention_mask, mask_pos, inputs_embeds)
+
+ input_args = [input_ids, attention_mask, mask_pos, labels, None, 1]
+ embed = self.forward(*input_args)
+ noise = generate_noise(embed, attention_mask)
+
+
+ for step in range(0, self.K):
+ vat_args = [input_ids, attention_mask, mask_pos, labels, embed + noise, 2]
+ adv_logits, _ = self.forward(*vat_args)
+ adv_loss = stable_kl(adv_logits, logits.detach(), reduce=False)
+ try:
+ delta_grad, = torch.autograd.grad(adv_loss, noise, only_inputs=True, retain_graph=False)
+ except:
+ import pdb
+ pdb.set_trace()
+
+ norm = delta_grad.norm()
+ if (torch.isnan(norm) or torch.isinf(norm)):
+ return 0
+ eff_delta_grad = delta_grad * self.step_size
+ delta_grad, eff_noise = norm_grad(delta_grad, eff_grad=eff_delta_grad)
+ noise = noise + delta_grad * self.step_size
+ # noise, eff_noise = self._norm_grad(delta_grad, eff_grad=eff_delta_grad, sentence_level=self.norm_level)
+ noise = noise.detach()
+ noise.requires_grad_()
+
+
+ vat_args = [input_ids, attention_mask, mask_pos, labels, embed + noise, 2]
+
+ adv_logits, sequence_mask_output = self.forward(*vat_args)
+ # ori_args = model(*ori_args)
+ # aug_args = [input_ids, token_type_ids, attention_mask, premise_mask, hyp_mask, task_id, 2, (embed + native_noise).detach()]
+
+ adv_loss = self.adv_lc(adv_logits, logits)
+ return adv_loss
+
+ def embed_encode(self, input_ids):
+ embedding_output = self.deberta.embeddings.word_embeddings(input_ids)
+ return embedding_output
+
+ def encode(self, input_ids=None, attention_mask=None, token_type_ids=None, mask_pos=None, inputs_embeds=None,
+ return_full_softmax=False):
+ batch_size = input_ids.size(0)
+
+ if mask_pos is not None:
+ mask_pos = mask_pos.squeeze()
+
+
+ # Encode everything
+ if inputs_embeds is None:
+ outputs = self.deberta(
+ input_ids,
+ attention_mask=attention_mask,
+ token_type_ids=token_type_ids
+ )
+ else:
+ outputs = self.deberta(
+ None,
+ attention_mask=attention_mask,
+ token_type_ids=token_type_ids,
+ inputs_embeds=inputs_embeds
+ )
+
+ # Get token representation
+ sequence_output = outputs[0]
+ sequence_output = sequence_output[:, self.pre_seq_len:, :].contiguous()
+ sequence_mask_output = sequence_output[torch.arange(sequence_output.size(0)), mask_pos]
+
+ # Logits over vocabulary tokens
+ prediction_mask_scores = self.cls(sequence_mask_output)
+
+ # sequence_mask_output = self.lm_head.dense(sequence_mask_output)
+
+ # Exit early and only return mask logits.
+ if return_full_softmax:
+ return prediction_mask_scores
+
+ # Return logits for each label
+ logits = []
+ for label_id in range(len(self.label_word_list)):
+ logits.append(prediction_mask_scores[:, self.label_word_list[label_id]].unsqueeze(-1))
+ logits = torch.cat(logits, -1)
+
+ # Regression task
+ if self.config.num_labels == 1:
+ logsoftmax = nn.LogSoftmax(-1)
+ logits = logsoftmax(logits) # Log prob of right polarity
+
+ if self.model_args.hybrid == 1:
+ cls_logits = self.classifier(sequence_output)
+ return (logits, cls_logits), sequence_mask_output
+
+ return logits, sequence_mask_output
+
+ def forward(
+ self,
+ input_ids=None,
+ attention_mask=None,
+ token_type_ids=None,
+ mask_pos=None,
+ labels=None,
+ inputs_embeds=None,
+ fwd_type=0,
+ block_flag=None
+ ):
+
+ if fwd_type == 2:
+ assert inputs_embeds is not None
+ return self.encode(input_ids=input_ids, attention_mask=attention_mask, token_type_ids=token_type_ids,
+ mask_pos=mask_pos, inputs_embeds=inputs_embeds)
+
+ elif fwd_type == 1:
+ return self.embed_encode(input_ids)
+
+
+
+ if (self.model_args.prompt_ptuning or self.model_args.prompt_prefix) and block_flag is not None:
+ inputs_embeds = self.generate_continuous_prompt_inputs(input_ids, block_flag)
+
+ logits, sequence_mask_output = self.encode(input_ids, attention_mask, token_type_ids, mask_pos, inputs_embeds)
+
+ if self.model_args.hybrid == 1:
+ logits = logits[0]
+ cls_logits = logits[1]
+
+ loss = None
+ if labels is not None:
+ if self.num_labels == 1:
+ # Regression task
+ loss_fct = nn.KLDivLoss(log_target=True)
+ labels = torch.stack([1 - (labels.view(-1) - self.lb) / (self.ub - self.lb),
+ (labels.view(-1) - self.lb) / (self.ub - self.lb)], -1)
+ loss = loss_fct(logits.view(-1, 2), labels)
+ else:
+
+ if labels.shape == logits.shape:
+ loss = F.kl_div(F.log_softmax(logits, dim=-1, dtype=torch.float32),
+ labels, reduction='batchmean')
+ else:
+ loss_fct = nn.CrossEntropyLoss()
+
+ loss = loss_fct(logits.view(-1, logits.size(-1)), labels.view(-1))
+
+ output = (logits,)
+ if self.num_labels == 1:
+ # Regression output
+ output = (torch.exp(logits[..., 1].unsqueeze(-1)) * (self.ub - self.lb) + self.lb,)
+
+ return ((loss,) + output) if loss is not None else output
+
+
+
+# add by wjn
+# Prefix-tuning for Deberta
+class DebertPrefixForPromptFinetuning(DebertaPreTrainedModel):
+
+ def __init__(self, config):
+ super().__init__(config)
+ self.num_labels = config.num_labels
+ #self.deberta = DebertaV2Model(config)
+
+ self.deberta = DebertaModel(config)
+ self.cls = DebertaOnlyMLMHead(config)
+
+ self.pooler = ContextPooler(config)
+ output_dim = self.pooler.output_dim
+
+ self.classifier = torch.nn.Linear(output_dim, self.num_labels)
+ drop_out = getattr(config, "cls_dropout", None)
+ drop_out = self.config.hidden_dropout_prob if drop_out is None else drop_out
+
+ self.dropout = StableDropout(drop_out)
+
+ classification_list = [self.pooler, self.dropout,self.classifier]
+
+ self.classifier = nn.Sequential(*classification_list)
+ # self.cls = DebertaV2OnlyMLMHead(config)
+
+ self.map = nn.Linear(config.hidden_size, config.hidden_size)
+ self.init_weights()
+
+ if self.config.use_pe:
+ self.deberta = freezer.freeze_lm(self.deberta)
+
+ self.pre_seq_len = config.pre_seq_len
+ self.n_layer = config.num_hidden_layers
+ self.n_head = config.num_attention_heads
+ self.n_embd = config.hidden_size // config.num_attention_heads
+
+ self.prefix_tokens = torch.arange(self.pre_seq_len).long()
+ self.prefix_encoder = PrefixEncoder(config)
+
+ # These attributes should be assigned once the model is initialized
+ self.model_args = None
+ self.data_args = None
+ self.label_word_list = None
+ self.K = 1
+ self.step_size=1e-5
+ self.adv_lc = SymKlCriterion()
+ self.contra_lc = ContrastiveLoss()
+ # import pdb
+ # pdb.set_trace()
+ #self.step_size=config.step_size
+
+ # For regression
+ self.lb = None
+ self.ub = None
+
+
+ # For auto label search.
+ self.return_full_softmax = None
+
+ def freeze_backbone(self, use_pe: bool=True):
+ if use_pe:
+ self.deberta = freezer.freeze_lm(self.deberta)
+ else:
+ self.deberta = freezer.unfreeze_lm(self.deberta)
+
+ def get_prompt(self, batch_size):
+ prefix_tokens = self.prefix_tokens.unsqueeze(0).expand(batch_size, -1).to(self.deberta.device)
+ past_key_values = self.prefix_encoder(prefix_tokens)
+ # bsz, seqlen, _ = past_key_values.shape
+ past_key_values = past_key_values.view(
+ batch_size,
+ self.pre_seq_len,
+ self.n_layer * 2,
+ self.n_head,
+ self.n_embd
+ )
+ past_key_values = self.dropout(past_key_values)
+ past_key_values = past_key_values.permute([2, 0, 3, 1, 4]).split(2)
+ return past_key_values
+
+
+ def get_constrast_loss(self,
+ input_ids=None,
+ attention_mask=None,
+ mask_pos=None,
+ labels=None,
+ inputs_embeds=None):
+
+ self.cos = nn.CosineSimilarity(dim=-1)
+
+
+ _, sequence_mask_output_1 = self.encode(input_ids, attention_mask, mask_pos, inputs_embeds)
+ _, sequence_mask_output_2 = self.encode(input_ids, attention_mask, mask_pos, inputs_embeds)
+
+ sequence_mask_output_1= self.lm_head.dense(sequence_mask_output_1)
+ sequence_mask_output_2 = self.lm_head.dense(sequence_mask_output_2)
+ # input_args = [input_ids, attention_mask, mask_pos, labels, None, 1]
+ # embed = self.forward(*input_args)
+ #
+ # vat_args = [input_ids, attention_mask, mask_pos, labels, embed, 2]
+ #
+ # adv_logits, outputs = self.forward(*vat_args)
+ #
+ # logit_mask = F.softmax(logits, dim=-1)[torch.arange(adv_logits.size(0)), labels] > 0.7
+ #
+ # outputs = outputs[logit_mask]
+ # seq_outputs = sequence_mask_output[logit_mask]
+ # new_label = labels[logit_mask]
+ # #
+ # #
+ # rand_perm = torch.randperm(outputs.size(0))
+ # rand_outputs = outputs[rand_perm, :]
+ # rand_label = new_label[rand_perm]
+ # pair_label = (new_label == rand_label).long()
+ #
+ # seq_outputs = self.map(seq_outputs)
+ # rand_outputs = self.map(rand_outputs)
+
+ pair_labels = (labels.unsqueeze(1) == labels.unsqueeze(0)).float()
+
+ # import pdb
+ # pdb.set_trace()
+
+ contra_loss = self.contra_lc(sequence_mask_output_1.unsqueeze(1), sequence_mask_output_2.unsqueeze(0), pair_labels)
+
+ if torch.isnan(contra_loss):
+ return 0
+
+ return contra_loss
+
+ def embed_encode(self, input_ids):
+ embedding_output = self.deberta.embeddings.word_embeddings(input_ids)
+ return embedding_output
+
+ def encode(self, input_ids=None, attention_mask=None, token_type_ids=None, mask_pos=None, inputs_embeds=None, return_full_softmax=False):
+ batch_size = input_ids.size(0)
+
+ # add prefix for prompt-tuning
+ past_key_values = self.get_prompt(batch_size=batch_size)
+ prefix_attention_mask = torch.ones(batch_size, self.pre_seq_len).to(self.deberta.device)
+ attention_mask = torch.cat((prefix_attention_mask, attention_mask), dim=1)
+
+ if mask_pos is not None:
+ mask_pos = mask_pos.squeeze()
+
+ # Encode everything
+
+ outputs = self.deberta(
+ input_ids,
+ attention_mask=attention_mask,
+ token_type_ids=token_type_ids,
+ past_key_values=past_key_values,
+ )
+
+
+ # Get token representation
+ sequence_output, pooled_output = outputs[:2]
+ # sequence_output = sequence_output[:, self.pre_seq_len:, :].contiguous()
+ sequence_mask_output = sequence_output[torch.arange(sequence_output.size(0)), mask_pos]
+
+ # Logits over vocabulary tokens
+ prediction_mask_scores = self.cls(sequence_mask_output)
+
+ #sequence_mask_output = self.lm_head.dense(sequence_mask_output)
+
+ # Exit early and only return mask logits.
+ if return_full_softmax:
+ return prediction_mask_scores
+
+ # Return logits for each label
+ logits = []
+ for label_id in range(len(self.label_word_list)):
+ logits.append(prediction_mask_scores[:, self.label_word_list[label_id]].unsqueeze(-1))
+ logits = torch.cat(logits, -1)
+
+ # Regression task
+ if self.config.num_labels == 1:
+ logsoftmax = nn.LogSoftmax(-1)
+ logits = logsoftmax(logits) # Log prob of right polarity
+
+ if self.model_args.hybrid == 1:
+ cls_logits = self.classifier(sequence_output)
+ return (logits, cls_logits), sequence_mask_output
+
+ return logits, sequence_mask_output
+
+
+ def forward(
+ self,
+ input_ids=None,
+ attention_mask=None,
+ token_type_ids=None,
+ mask_pos=None,
+ labels=None,
+ inputs_embeds=None,
+ fwd_type=0,
+ block_flag=None,
+ return_dict=None,
+ ):
+
+ if fwd_type == 2:
+ assert inputs_embeds is not None
+ return self.encode(input_ids=input_ids, attention_mask=attention_mask, token_type_ids=token_type_ids,
+ mask_pos=mask_pos, inputs_embeds=inputs_embeds)
+
+ elif fwd_type == 1:
+ return self.embed_encode(input_ids)
+
+
+
+ if (self.model_args.prompt_ptuning or self.model_args.prompt_prefix) and block_flag is not None:
+ inputs_embeds = self.generate_continuous_prompt_inputs(input_ids, block_flag)
+
+ logits, sequence_mask_output = self.encode(input_ids, attention_mask, token_type_ids, mask_pos, inputs_embeds)
+
+ if self.model_args.hybrid == 1:
+ logits = logits[0]
+ cls_logits = logits[1]
+
+ loss = None
+ if labels is not None:
+ if self.num_labels == 1:
+ # Regression task
+ loss_fct = nn.KLDivLoss(log_target=True)
+ labels = torch.stack([1 - (labels.view(-1) - self.lb) / (self.ub - self.lb),
+ (labels.view(-1) - self.lb) / (self.ub - self.lb)], -1)
+ loss = loss_fct(logits.view(-1, 2), labels)
+ else:
+
+ if labels.shape == logits.shape:
+ loss = F.kl_div(F.log_softmax(logits, dim=-1, dtype=torch.float32),
+ labels, reduction='batchmean')
+ else:
+ loss_fct = nn.CrossEntropyLoss()
+
+ loss = loss_fct(logits.view(-1, logits.size(-1)), labels.view(-1))
+
+ output = (logits,)
+ if self.num_labels == 1:
+ # Regression output
+ output = (torch.exp(logits[..., 1].unsqueeze(-1)) * (self.ub - self.lb) + self.lb,)
+
+ if not return_dict:
+ return ((loss,) + output) if loss is not None else output
+
+ return SequenceClassifierOutput(
+ loss=loss,
+ logits=logits,
+ )
+
+
+
+
+class Debertav2ForPromptFinetuning(DebertaV2PreTrainedModel):
+ _keys_to_ignore_on_load_unexpected = [r"pooler"]
+ _keys_to_ignore_on_load_missing = [r"position_ids", r"predictions.decoder.bias"]
+
+ def __init__(self, config):
+ super().__init__(config)
+ self.num_labels = config.num_labels
+ self.deberta = DebertaV2Model(config)
+
+ if self.config.use_pe:
+ self.deberta = freezer.freeze_lm(self.deberta)
+ self.cls = DebertaV2OnlyMLMHead(config)
+
+ #self.deberta = DebertaModel(config)
+ #self.cls = DebertaOnlyMLMHead(config)
+
+ self.pooler = ContextPooler(config)
+ output_dim = self.pooler.output_dim
+
+ self.classifier = torch.nn.Linear(output_dim, self.num_labels)
+ drop_out = getattr(config, "cls_dropout", None)
+ drop_out = self.config.hidden_dropout_prob if drop_out is None else drop_out
+
+ self.dropout = StableDropout(drop_out)
+
+ classification_list = [self.pooler, self.dropout,self.classifier]
+
+ self.classifier = nn.Sequential(*classification_list)
+ # self.cls = DebertaV2OnlyMLMHead(config)
+
+ self.map = nn.Linear(config.hidden_size, config.hidden_size)
+ self.init_weights()
+
+ # These attributes should be assigned once the model is initialized
+ self.model_args = None
+ self.data_args = None
+ self.label_word_list = None
+ self.K = 1
+ self.step_size=1e-5
+ self.adv_lc = SymKlCriterion()
+ self.contra_lc = ContrastiveLoss()
+ # import pdb
+ # pdb.set_trace()
+ #self.step_size=config.step_size
+
+ # For regression
+ self.lb = None
+ self.ub = None
+
+ self.pre_seq_len = self.config.pre_seq_len
+ # For auto label search.
+ self.return_full_softmax = None
+
+ def freeze_backbone(self, use_pe: bool=True):
+ if use_pe:
+ self.deberta = freezer.freeze_lm(self.deberta)
+ else:
+ self.deberta = freezer.unfreeze_lm(self.deberta)
+
+ def get_constrast_loss(self,
+ input_ids=None,
+ attention_mask=None,
+ mask_pos=None,
+ labels=None,
+ inputs_embeds=None):
+
+ self.cos = nn.CosineSimilarity(dim=-1)
+
+
+ _, sequence_mask_output_1 = self.encode(input_ids, attention_mask, mask_pos, inputs_embeds)
+ _, sequence_mask_output_2 = self.encode(input_ids, attention_mask, mask_pos, inputs_embeds)
+
+ sequence_mask_output_1= self.lm_head.dense(sequence_mask_output_1)
+ sequence_mask_output_2 = self.lm_head.dense(sequence_mask_output_2)
+ # input_args = [input_ids, attention_mask, mask_pos, labels, None, 1]
+ # embed = self.forward(*input_args)
+ #
+ # vat_args = [input_ids, attention_mask, mask_pos, labels, embed, 2]
+ #
+ # adv_logits, outputs = self.forward(*vat_args)
+ #
+ # logit_mask = F.softmax(logits, dim=-1)[torch.arange(adv_logits.size(0)), labels] > 0.7
+ #
+ # outputs = outputs[logit_mask]
+ # seq_outputs = sequence_mask_output[logit_mask]
+ # new_label = labels[logit_mask]
+ # #
+ # #
+ # rand_perm = torch.randperm(outputs.size(0))
+ # rand_outputs = outputs[rand_perm, :]
+ # rand_label = new_label[rand_perm]
+ # pair_label = (new_label == rand_label).long()
+ #
+ # seq_outputs = self.map(seq_outputs)
+ # rand_outputs = self.map(rand_outputs)
+
+ pair_labels = (labels.unsqueeze(1) == labels.unsqueeze(0)).float()
+
+ # import pdb
+ # pdb.set_trace()
+
+
+ contra_loss = self.contra_lc(sequence_mask_output_1.unsqueeze(1), sequence_mask_output_2.unsqueeze(0), pair_labels)
+
+
+
+ if torch.isnan(contra_loss):
+ return 0
+
+ return contra_loss
+
+
+
+ def get_adv_loss(self,
+ input_ids=None,
+ attention_mask=None,
+ mask_pos=None,
+ labels=None,
+ inputs_embeds=None):
+
+ logits, sequence_mask_output = self.encode(input_ids, attention_mask, mask_pos, inputs_embeds)
+
+ input_args = [input_ids, attention_mask, mask_pos, labels, None, 1]
+ embed = self.forward(*input_args)
+ noise = generate_noise(embed, attention_mask)
+
+
+ for step in range(0, self.K):
+ vat_args = [input_ids, attention_mask, mask_pos, labels, embed + noise, 2]
+ adv_logits, _ = self.forward(*vat_args)
+ adv_loss = stable_kl(adv_logits, logits.detach(), reduce=False)
+ try:
+ delta_grad, = torch.autograd.grad(adv_loss, noise, only_inputs=True, retain_graph=False)
+ except:
+ import pdb
+ pdb.set_trace()
+
+ norm = delta_grad.norm()
+ if (torch.isnan(norm) or torch.isinf(norm)):
+ return 0
+ eff_delta_grad = delta_grad * self.step_size
+ delta_grad, eff_noise = norm_grad(delta_grad, eff_grad=eff_delta_grad)
+ noise = noise + delta_grad * self.step_size
+ # noise, eff_noise = self._norm_grad(delta_grad, eff_grad=eff_delta_grad, sentence_level=self.norm_level)
+ noise = noise.detach()
+ noise.requires_grad_()
+
+
+ vat_args = [input_ids, attention_mask, mask_pos, labels, embed + noise, 2]
+
+ adv_logits, sequence_mask_output = self.forward(*vat_args)
+ # ori_args = model(*ori_args)
+ # aug_args = [input_ids, token_type_ids, attention_mask, premise_mask, hyp_mask, task_id, 2, (embed + native_noise).detach()]
+
+ adv_loss = self.adv_lc(adv_logits, logits)
+ return adv_loss
+
+ def embed_encode(self, input_ids):
+ embedding_output = self.deberta.embeddings.word_embeddings(input_ids)
+ return embedding_output
+
+ def encode(self, input_ids=None, attention_mask=None, mask_pos=None, inputs_embeds=None, return_full_softmax=False):
+ batch_size = input_ids.size(0)
+
+ if mask_pos is not None:
+ mask_pos = mask_pos.squeeze()
+
+ # Encode everything
+ if inputs_embeds is None:
+ outputs = self.deberta(
+ input_ids,
+ attention_mask=attention_mask
+ )
+ else:
+ outputs = self.deberta(
+ None,
+ attention_mask=attention_mask,
+ inputs_embeds=inputs_embeds
+ )
+
+
+ # Get token representation
+ sequence_output = outputs[0]
+ sequence_output = sequence_output[:, self.pre_seq_len:, :].contiguous()
+ sequence_mask_output = sequence_output[torch.arange(sequence_output.size(0)), mask_pos]
+
+
+ # Logits over vocabulary tokens
+ prediction_mask_scores = self.cls(sequence_mask_output)
+
+ #sequence_mask_output = self.lm_head.dense(sequence_mask_output)
+
+ # Exit early and only return mask logits.
+ if return_full_softmax:
+ return prediction_mask_scores
+
+ # Return logits for each label
+ logits = []
+ for label_id in range(len(self.label_word_list)):
+ logits.append(prediction_mask_scores[:, self.label_word_list[label_id]].unsqueeze(-1))
+ logits = torch.cat(logits, -1)
+
+ # Regression task
+ if self.config.num_labels == 1:
+ logsoftmax = nn.LogSoftmax(-1)
+ logits = logsoftmax(logits) # Log prob of right polarity
+
+ return logits, sequence_mask_output
+
+
+ def forward(
+ self,
+ input_ids=None,
+ attention_mask=None,
+ mask_pos=None,
+ labels=None,
+ inputs_embeds=None,
+ fwd_type=0,
+ block_flag=None,
+ return_dict=None
+ ):
+ if fwd_type == 2:
+ assert inputs_embeds is not None
+ return self.encode(input_ids=input_ids, attention_mask=attention_mask, mask_pos=mask_pos, inputs_embeds=inputs_embeds)
+
+ elif fwd_type == 1:
+ return self.embed_encode(input_ids)
+
+ logits, sequence_mask_output = self.encode(input_ids, attention_mask, mask_pos, inputs_embeds)
+
+ loss = None
+
+
+ if labels is not None:
+ if self.num_labels == 1:
+ # Regression task
+ loss_fct = nn.KLDivLoss(log_target=True)
+ labels = torch.stack([1 - (labels.view(-1) - self.lb) / (self.ub - self.lb), (labels.view(-1) - self.lb) / (self.ub - self.lb)], -1)
+ loss = loss_fct(logits.view(-1, 2), labels)
+ else:
+
+ if labels.shape == logits.shape:
+ loss = F.kl_div(F.log_softmax(logits, dim=-1, dtype=torch.float32),
+ labels, reduction='batchmean')
+ else:
+ loss_fct = nn.CrossEntropyLoss()
+
+ loss = loss_fct(logits.view(-1, logits.size(-1)), labels.view(-1))
+ if self.model_args.hybrid == 1:
+ cls_loss = loss_fct(cls_logits.view(-1, cls_logits.size(-1)), labels.view(-1))
+ loss = loss + cls_loss
+
+ output = (logits,)
+ if self.num_labels == 1:
+ # Regression output
+ output = (torch.exp(logits[..., 1].unsqueeze(-1)) * (self.ub - self.lb) + self.lb,)
+
+ if not return_dict:
+ return ((loss,) + output) if loss is not None else output
+
+ return SequenceClassifierOutput(
+ loss=loss,
+ logits=logits,
+ )
+
+
+class Debertav2PrefixForPromptFinetuning(DebertaV2PreTrainedModel):
+ _keys_to_ignore_on_load_unexpected = [r"pooler"]
+ _keys_to_ignore_on_load_missing = [r"position_ids", r"predictions.decoder.bias"]
+
+ def __init__(self, config):
+ super().__init__(config)
+ self.num_labels = config.num_labels
+ self.deberta = DebertaV2Model(config)
+ self.cls = DebertaV2OnlyMLMHead(config)
+
+ #self.deberta = DebertaModel(config)
+ #self.cls = DebertaOnlyMLMHead(config)
+
+ self.pooler = ContextPooler(config)
+ output_dim = self.pooler.output_dim
+
+ self.classifier = torch.nn.Linear(output_dim, self.num_labels)
+ drop_out = getattr(config, "cls_dropout", None)
+ drop_out = self.config.hidden_dropout_prob if drop_out is None else drop_out
+
+ self.dropout = StableDropout(drop_out)
+
+ classification_list = [self.pooler, self.dropout,self.classifier]
+
+ self.classifier = nn.Sequential(*classification_list)
+ # self.cls = DebertaV2OnlyMLMHead(config)
+
+ self.map = nn.Linear(config.hidden_size, config.hidden_size)
+ self.init_weights()
+
+ if self.config.use_pe:
+ self.deberta = freezer.freeze_lm(self.deberta)
+
+ self.pre_seq_len = config.pre_seq_len
+ self.n_layer = config.num_hidden_layers
+ self.n_head = config.num_attention_heads
+ self.n_embd = config.hidden_size // config.num_attention_heads
+
+ self.prefix_tokens = torch.arange(self.pre_seq_len).long()
+ self.prefix_encoder = PrefixEncoder(config)
+
+ # These attributes should be assigned once the model is initialized
+ self.model_args = None
+ self.data_args = None
+ self.label_word_list = None
+ self.K = 1
+ self.step_size=1e-5
+ self.adv_lc = SymKlCriterion()
+ self.contra_lc = ContrastiveLoss()
+ # import pdb
+ # pdb.set_trace()
+ #self.step_size=config.step_size
+
+ # For regression
+ self.lb = None
+ self.ub = None
+
+
+ # For auto label search.
+ self.return_full_softmax = None
+
+ def freeze_backbone(self, use_pe: bool=True):
+ if use_pe:
+ self.deberta = freezer.freeze_lm(self.deberta)
+ else:
+ self.deberta = freezer.unfreeze_lm(self.deberta)
+
+ def get_prompt(self, batch_size):
+ prefix_tokens = self.prefix_tokens.unsqueeze(0).expand(batch_size, -1).to(self.deberta.device)
+ past_key_values = self.prefix_encoder(prefix_tokens)
+ # bsz, seqlen, _ = past_key_values.shape
+ past_key_values = past_key_values.view(
+ batch_size,
+ self.pre_seq_len,
+ self.n_layer * 2,
+ self.n_head,
+ self.n_embd
+ )
+ past_key_values = self.dropout(past_key_values)
+ past_key_values = past_key_values.permute([2, 0, 3, 1, 4]).split(2)
+ return past_key_values
+
+ def get_constrast_loss(self,
+ input_ids=None,
+ attention_mask=None,
+ mask_pos=None,
+ labels=None,
+ inputs_embeds=None):
+
+ self.cos = nn.CosineSimilarity(dim=-1)
+
+
+ _, sequence_mask_output_1 = self.encode(input_ids, attention_mask, mask_pos, inputs_embeds)
+ _, sequence_mask_output_2 = self.encode(input_ids, attention_mask, mask_pos, inputs_embeds)
+
+ sequence_mask_output_1= self.lm_head.dense(sequence_mask_output_1)
+ sequence_mask_output_2 = self.lm_head.dense(sequence_mask_output_2)
+ # input_args = [input_ids, attention_mask, mask_pos, labels, None, 1]
+ # embed = self.forward(*input_args)
+ #
+ # vat_args = [input_ids, attention_mask, mask_pos, labels, embed, 2]
+ #
+ # adv_logits, outputs = self.forward(*vat_args)
+ #
+ # logit_mask = F.softmax(logits, dim=-1)[torch.arange(adv_logits.size(0)), labels] > 0.7
+ #
+ # outputs = outputs[logit_mask]
+ # seq_outputs = sequence_mask_output[logit_mask]
+ # new_label = labels[logit_mask]
+ # #
+ # #
+ # rand_perm = torch.randperm(outputs.size(0))
+ # rand_outputs = outputs[rand_perm, :]
+ # rand_label = new_label[rand_perm]
+ # pair_label = (new_label == rand_label).long()
+ #
+ # seq_outputs = self.map(seq_outputs)
+ # rand_outputs = self.map(rand_outputs)
+
+ pair_labels = (labels.unsqueeze(1) == labels.unsqueeze(0)).float()
+
+ # import pdb
+ # pdb.set_trace()
+
+
+ contra_loss = self.contra_lc(sequence_mask_output_1.unsqueeze(1), sequence_mask_output_2.unsqueeze(0), pair_labels)
+
+
+
+ if torch.isnan(contra_loss):
+ return 0
+
+ return contra_loss
+
+
+
+ def get_adv_loss(self,
+ input_ids=None,
+ attention_mask=None,
+ mask_pos=None,
+ labels=None,
+ inputs_embeds=None):
+
+ logits, sequence_mask_output = self.encode(input_ids, attention_mask, mask_pos, inputs_embeds)
+
+ input_args = [input_ids, attention_mask, mask_pos, labels, None, 1]
+ embed = self.forward(*input_args)
+ noise = generate_noise(embed, attention_mask)
+
+
+ for step in range(0, self.K):
+ vat_args = [input_ids, attention_mask, mask_pos, labels, embed + noise, 2]
+ adv_logits, _ = self.forward(*vat_args)
+ adv_loss = stable_kl(adv_logits, logits.detach(), reduce=False)
+ try:
+ delta_grad, = torch.autograd.grad(adv_loss, noise, only_inputs=True, retain_graph=False)
+ except:
+ import pdb
+ pdb.set_trace()
+
+ norm = delta_grad.norm()
+ if (torch.isnan(norm) or torch.isinf(norm)):
+ return 0
+ eff_delta_grad = delta_grad * self.step_size
+ delta_grad, eff_noise = norm_grad(delta_grad, eff_grad=eff_delta_grad)
+ noise = noise + delta_grad * self.step_size
+ # noise, eff_noise = self._norm_grad(delta_grad, eff_grad=eff_delta_grad, sentence_level=self.norm_level)
+ noise = noise.detach()
+ noise.requires_grad_()
+
+
+ vat_args = [input_ids, attention_mask, mask_pos, labels, embed + noise, 2]
+
+ adv_logits, sequence_mask_output = self.forward(*vat_args)
+ # ori_args = model(*ori_args)
+ # aug_args = [input_ids, token_type_ids, attention_mask, premise_mask, hyp_mask, task_id, 2, (embed + native_noise).detach()]
+
+ adv_loss = self.adv_lc(adv_logits, logits)
+ return adv_loss
+
+ def embed_encode(self, input_ids):
+ embedding_output = self.deberta.embeddings.word_embeddings(input_ids)
+ return embedding_output
+
+ def encode(self, input_ids=None, attention_mask=None, mask_pos=None, inputs_embeds=None, return_full_softmax=False):
+ batch_size = input_ids.size(0)
+
+ # add prefix for prompt-tuning
+ past_key_values = self.get_prompt(batch_size=batch_size)
+ prefix_attention_mask = torch.ones(batch_size, self.pre_seq_len).to(self.deberta.device)
+ attention_mask = torch.cat((prefix_attention_mask, attention_mask), dim=1)
+
+
+ if mask_pos is not None:
+ mask_pos = mask_pos.squeeze()
+
+ # Encode everything
+ outputs = self.deberta(
+ input_ids,
+ attention_mask=attention_mask,
+ past_key_values=past_key_values,
+ )
+
+
+ # Get token representation
+ sequence_output = outputs[0]
+ # sequence_output = sequence_output[:, self.pre_seq_len:, :].contiguous()
+ sequence_mask_output = sequence_output[torch.arange(sequence_output.size(0)), mask_pos]
+
+
+ # Logits over vocabulary tokens
+ prediction_mask_scores = self.cls(sequence_mask_output)
+
+ #sequence_mask_output = self.lm_head.dense(sequence_mask_output)
+
+ # Exit early and only return mask logits.
+ if return_full_softmax:
+ return prediction_mask_scores
+
+ # Return logits for each label
+ logits = []
+ for label_id in range(len(self.label_word_list)):
+ logits.append(prediction_mask_scores[:, self.label_word_list[label_id]].unsqueeze(-1))
+ logits = torch.cat(logits, -1)
+
+ # Regression task
+ if self.config.num_labels == 1:
+ logsoftmax = nn.LogSoftmax(-1)
+ logits = logsoftmax(logits) # Log prob of right polarity
+
+ return logits, sequence_mask_output
+
+
+ def forward(
+ self,
+ input_ids=None,
+ attention_mask=None,
+ mask_pos=None,
+ labels=None,
+ inputs_embeds=None,
+ fwd_type=0,
+ block_flag=None,
+ return_dict=None,
+ ):
+ if fwd_type == 2:
+ assert inputs_embeds is not None
+ return self.encode(input_ids=input_ids, attention_mask=attention_mask, mask_pos=mask_pos, inputs_embeds=inputs_embeds)
+
+ elif fwd_type == 1:
+ return self.embed_encode(input_ids)
+
+ logits, sequence_mask_output = self.encode(input_ids, attention_mask, mask_pos, inputs_embeds)
+
+ loss = None
+
+
+ if labels is not None:
+ if self.num_labels == 1:
+ # Regression task
+ loss_fct = nn.KLDivLoss(log_target=True)
+ labels = torch.stack([1 - (labels.view(-1) - self.lb) / (self.ub - self.lb), (labels.view(-1) - self.lb) / (self.ub - self.lb)], -1)
+ loss = loss_fct(logits.view(-1, 2), labels)
+ else:
+
+ if labels.shape == logits.shape:
+ loss = F.kl_div(F.log_softmax(logits, dim=-1, dtype=torch.float32),
+ labels, reduction='batchmean')
+ else:
+ loss_fct = nn.CrossEntropyLoss()
+
+ loss = loss_fct(logits.view(-1, logits.size(-1)), labels.view(-1))
+ if self.model_args.hybrid == 1:
+ cls_loss = loss_fct(cls_logits.view(-1, cls_logits.size(-1)), labels.view(-1))
+ loss = loss + cls_loss
+
+ output = (logits,)
+ if self.num_labels == 1:
+ # Regression output
+ output = (torch.exp(logits[..., 1].unsqueeze(-1)) * (self.ub - self.lb) + self.lb,)
+
+ if not return_dict:
+ return ((loss,) + output) if loss is not None else output
+
+ return SequenceClassifierOutput(
+ loss=loss,
+ logits=logits,
+ )
+
+
+
+num_labels_mapping = {
+ "cola": 2,
+ "mnli": 3,
+ "mrpc": 2,
+ "sst2": 2,
+ "sts-b": 1,
+ "qqp": 2,
+ "qnli": 2,
+ "rte": 2,
+ "wnli": 2,
+ "snli": 3,
+ "movie_rationales": 2,
+ "sst-5": 5,
+ "subj": 2,
+ "trec": 6,
+ "cr": 2,
+ "mpqa": 2,
+ "boolq": 2,
+ "cb": 3,
+ "ag_news": 4,
+ "yelp_polarity": 2,
+}
+
+output_modes_mapping = {
+ "cola": "classification",
+ "mnli": "classification",
+ "mnli-mm": "classification",
+ "mnli-mm-clue": "classification",
+ "mrpc": "classification",
+ "sst2": "classification",
+ "sst-2-clue": "classification",
+ "sts-b": "regression",
+ "qqp": "classification",
+ "qqp-clue": "classification",
+ "qnli": "classification",
+ "rte": "classification",
+ "rte-clue": "classification",
+ "wnli": "classification",
+ "snli": "classification",
+ "movie_rationales": "classification",
+ "mr-clue": "classification",
+ "sst-5": "classification",
+ "subj": "classification",
+ "subj-clue": "classification",
+ "trec": "classification",
+ "cr": "classification",
+ "mpqa": "classification",
+ "mpqa-clue": "classification",
+ "boolq": "classification",
+ "cb": "classification",
+ "ag_news": "classification",
+ "yelp_polarity": "classification"
+}
+
+# For regression task only: median
+median_mapping = {
+ "sts-b": 2.5
+}
+
+bound_mapping = {
+ "sts-b": (0, 5)
+}
diff --git a/model/utils.py b/model/utils.py
new file mode 100644
index 0000000..9082c7f
--- /dev/null
+++ b/model/utils.py
@@ -0,0 +1,434 @@
+from enum import Enum
+
+from model.head_for_token_classification import (
+ BertPrefixForTokenClassification,
+ BertAdapterForTokenClassification,
+ RobertaPrefixForTokenClassification,
+ RobertaAdapterForTokenClassification,
+ DebertaPrefixForTokenClassification,
+ DebertaV2PrefixForTokenClassification
+)
+
+from model.head_for_sequence_classification import (
+ BertPrefixForSequenceClassification,
+ BertPtuningForSequenceClassification,
+ BertAdapterForSequenceClassification,
+ RobertaPrefixForSequenceClassification,
+ RobertaPtuningForSequenceClassification,
+ RobertaAdapterForSequenceClassification,
+ DebertaPrefixForSequenceClassification
+)
+
+from model.head_for_question_answering import (
+ BertPrefixForQuestionAnswering,
+ BertAdapterForQuestionAnswering,
+ RobertaPrefixModelForQuestionAnswering,
+ RobertaAdapterForQuestionAnswering,
+ DebertaPrefixModelForQuestionAnswering
+)
+
+from model.prompt_for_sequence_classification import (
+ PromptBertForSequenceClassification,
+ PromptBertPrefixForSequenceClassification,
+ PromptBertPtuningForSequenceClassification,
+ PromptBertAdapterForSequenceClassification,
+ PromptRobertaForSequenceClassification,
+ PromptRobertaPrefixForSequenceClassification,
+ PromptRobertaPtuningForSequenceClassification,
+ PromptRobertaAdapterForSequenceClassification,
+ PromptDebertaForSequenceClassification,
+ PromptDebertaPrefixForSequenceClassification,
+ PromptDebertaPtuningForSequenceClassification,
+ # PromptDebertaAdapterForSequenceClassification,
+ PromptDebertav2ForSequenceClassification,
+ PromptDebertav2PrefixForSequenceClassification,
+ PromptDebertav2PtuningForSequenceClassification,
+ # PromptDebertav2AdapterForSequenceClassification
+)
+
+from model.multiple_choice import (
+ BertPrefixForMultipleChoice,
+ RobertaPrefixForMultipleChoice,
+ DebertaPrefixForMultipleChoice,
+ BertPromptForMultipleChoice,
+ RobertaPromptForMultipleChoice
+)
+
+from transformers import (
+ AutoConfig,
+ AutoModelForTokenClassification,
+ AutoModelForSequenceClassification,
+ AutoModelForQuestionAnswering,
+ AutoModelForMultipleChoice
+)
+
+
+class TaskType(Enum):
+ TOKEN_CLASSIFICATION = 1,
+ SEQUENCE_CLASSIFICATION = 2,
+ QUESTION_ANSWERING = 3,
+ MULTIPLE_CHOICE = 4
+
+# used for head fine-tuning
+AUTO_MODELS = {
+ TaskType.TOKEN_CLASSIFICATION: AutoModelForTokenClassification,
+ TaskType.SEQUENCE_CLASSIFICATION: AutoModelForSequenceClassification,
+ TaskType.QUESTION_ANSWERING: AutoModelForQuestionAnswering,
+ TaskType.MULTIPLE_CHOICE: AutoModelForMultipleChoice,
+}
+
+# used for head prefix-tuning
+HEAD_PREFIX_MODELS = {
+ "bert": {
+ TaskType.TOKEN_CLASSIFICATION: BertPrefixForTokenClassification,
+ TaskType.SEQUENCE_CLASSIFICATION: BertPrefixForSequenceClassification,
+ TaskType.QUESTION_ANSWERING: BertPrefixForQuestionAnswering,
+ TaskType.MULTIPLE_CHOICE: BertPrefixForMultipleChoice
+ },
+ "roberta": {
+ TaskType.TOKEN_CLASSIFICATION: RobertaPrefixForTokenClassification,
+ TaskType.SEQUENCE_CLASSIFICATION: RobertaPrefixForSequenceClassification,
+ TaskType.QUESTION_ANSWERING: RobertaPrefixModelForQuestionAnswering,
+ TaskType.MULTIPLE_CHOICE: RobertaPrefixForMultipleChoice,
+ },
+ "deberta": {
+ TaskType.TOKEN_CLASSIFICATION: DebertaPrefixForTokenClassification,
+ TaskType.SEQUENCE_CLASSIFICATION: DebertaPrefixForSequenceClassification,
+ TaskType.QUESTION_ANSWERING: DebertaPrefixModelForQuestionAnswering,
+ TaskType.MULTIPLE_CHOICE: DebertaPrefixForMultipleChoice,
+ },
+ "deberta-v2": {
+ TaskType.TOKEN_CLASSIFICATION: DebertaV2PrefixForTokenClassification,
+ TaskType.SEQUENCE_CLASSIFICATION: None,
+ TaskType.QUESTION_ANSWERING: None,
+ TaskType.MULTIPLE_CHOICE: None,
+ }
+}
+
+# used for head p-tuning
+HEAD_PTUNING_MODELS = {
+ "bert": {
+ TaskType.SEQUENCE_CLASSIFICATION: BertPtuningForSequenceClassification,
+ TaskType.MULTIPLE_CHOICE: BertPromptForMultipleChoice
+ },
+ "roberta": {
+ TaskType.SEQUENCE_CLASSIFICATION: RobertaPtuningForSequenceClassification,
+ TaskType.MULTIPLE_CHOICE: RobertaPromptForMultipleChoice
+ }
+}
+
+## add by wjn
+# used for head adapter-tuning
+HEAD_ADAPTER_MODELS = {
+ "bert": {
+ TaskType.TOKEN_CLASSIFICATION: BertAdapterForTokenClassification,
+ TaskType.SEQUENCE_CLASSIFICATION: BertAdapterForSequenceClassification,
+ TaskType.QUESTION_ANSWERING: BertAdapterForQuestionAnswering,
+ # TaskType.MULTIPLE_CHOICE: BertPrefixForMultipleChoice
+ },
+ "roberta": {
+ TaskType.TOKEN_CLASSIFICATION: RobertaAdapterForTokenClassification,
+ TaskType.SEQUENCE_CLASSIFICATION: RobertaAdapterForSequenceClassification,
+ TaskType.QUESTION_ANSWERING: RobertaAdapterForQuestionAnswering,
+ # TaskType.MULTIPLE_CHOICE: RobertaPrefixForMultipleChoice,
+ },
+}
+
+# used for prompt prefix-tuning
+PROMPT_PREFIX_MODELS = {
+ "bert": {
+ TaskType.SEQUENCE_CLASSIFICATION: PromptBertPrefixForSequenceClassification,
+ },
+ "roberta": {
+ TaskType.SEQUENCE_CLASSIFICATION: PromptRobertaPrefixForSequenceClassification,
+ },
+ "deberta": {
+ TaskType.SEQUENCE_CLASSIFICATION: PromptDebertaPrefixForSequenceClassification,
+ },
+ "deberta-v2": {
+ TaskType.SEQUENCE_CLASSIFICATION: PromptDebertav2PrefixForSequenceClassification,
+ },
+}
+
+
+# used for prompt only
+PROMPT_ONLY_MODELS = {
+ "bert": {
+ TaskType.SEQUENCE_CLASSIFICATION: PromptBertForSequenceClassification,
+ },
+ "roberta": {
+ TaskType.SEQUENCE_CLASSIFICATION: PromptRobertaForSequenceClassification,
+ },
+ "deberta": {
+ TaskType.SEQUENCE_CLASSIFICATION: PromptDebertaForSequenceClassification,
+ },
+ "deberta-v2": {
+ TaskType.SEQUENCE_CLASSIFICATION: PromptDebertav2ForSequenceClassification,
+ },
+}
+
+# used for prompt p-tuning
+PROMPT_PTUNING_MODELS = {
+ "bert": {
+ TaskType.SEQUENCE_CLASSIFICATION: PromptBertPtuningForSequenceClassification,
+ },
+ "roberta": {
+ TaskType.SEQUENCE_CLASSIFICATION: PromptRobertaPtuningForSequenceClassification,
+ },
+ "deberta": {
+ TaskType.SEQUENCE_CLASSIFICATION: PromptDebertaPtuningForSequenceClassification,
+ },
+ "deberta-v2": {
+ TaskType.SEQUENCE_CLASSIFICATION: PromptDebertav2PtuningForSequenceClassification,
+ },
+}
+
+# used for prompt adapter
+PROMPT_ADAPTER_MODELS = {
+ "bert": {
+ TaskType.SEQUENCE_CLASSIFICATION: PromptBertAdapterForSequenceClassification,
+ },
+ "roberta": {
+ TaskType.SEQUENCE_CLASSIFICATION: PromptRobertaAdapterForSequenceClassification,
+ },
+ # "deberta": {
+ # TaskType.SEQUENCE_CLASSIFICATION: PromptDebertaAdapterForSequenceClassification,
+ # },
+ # "deberta-v2": {
+ # TaskType.SEQUENCE_CLASSIFICATION: PromptDebertav2AdapterForSequenceClassification,
+ # },
+}
+
+
+
+def get_model(data_args, model_args, task_type: TaskType, config: AutoConfig, fix_bert: bool = False):
+
+
+ # whether to fixed parameters of backbone (use pe)
+ config.use_pe = model_args.use_pe
+
+ if model_args.head_only:
+ model_class = AUTO_MODELS[task_type]
+ model = model_class.from_pretrained(
+ model_args.model_name_or_path,
+ config=config,
+ revision=model_args.model_revision,
+ )
+ elif model_args.head_prefix:
+ config.hidden_dropout_prob = model_args.hidden_dropout_prob
+ config.pre_seq_len = model_args.pre_seq_len
+ config.prefix_projection = model_args.prefix_projection
+ config.prefix_hidden_size = model_args.prefix_hidden_size
+
+ model_class = HEAD_PREFIX_MODELS[config.model_type][task_type]
+ model = model_class.from_pretrained(
+ model_args.model_name_or_path,
+ config=config,
+ revision=model_args.model_revision,
+ )
+ elif model_args.head_ptuning:
+ config.pre_seq_len = model_args.pre_seq_len
+ model_class = HEAD_PTUNING_MODELS[config.model_type][task_type]
+ model = model_class.from_pretrained(
+ model_args.model_name_or_path,
+ config=config,
+ revision=model_args.model_revision,
+ )
+ elif model_args.head_adapter:
+ config.adapter_choice = model_args.adapter_choice
+ config.adapter_dim = model_args.adapter_dim
+ model_class = HEAD_ADAPTER_MODELS[config.model_type][task_type]
+ model = model_class.from_pretrained(
+ model_args.model_name_or_path,
+ config=config,
+ revision=model_args.model_revision,
+ )
+ elif model_args.prompt_only:
+ config.pre_seq_len = 0
+ model_class = PROMPT_ONLY_MODELS[config.model_type][task_type]
+ model = model_class(config, model_args, data_args)
+
+ elif model_args.prompt_prefix:
+ config.hidden_dropout_prob = model_args.hidden_dropout_prob
+ config.pre_seq_len = model_args.pre_seq_len
+ config.prefix_projection = model_args.prefix_projection
+ config.prefix_hidden_size = model_args.prefix_hidden_size
+ model_class = PROMPT_PREFIX_MODELS[config.model_type][task_type]
+ model = model_class(config, model_args, data_args)
+ # model = model_class.from_pretrained(
+ # model_args.model_name_or_path,
+ # config=config,
+ # revision=model_args.model_revision,
+ # )
+ elif model_args.prompt_ptuning:
+ config.pre_seq_len = model_args.pre_seq_len
+ model_class = PROMPT_PTUNING_MODELS[config.model_type][task_type]
+ model = model_class(config, model_args, data_args)
+ # model = model_class.from_pretrained(
+ # model_args.model_name_or_path,
+ # config=config,
+ # revision=model_args.model_revision,
+ # )
+ elif model_args.prompt_adapter:
+ config.adapter_choice = model_args.adapter_choice
+ config.adapter_dim = model_args.adapter_dim
+ model_class = PROMPT_ADAPTER_MODELS[config.model_type][task_type]
+ model = model_class(config, model_args, data_args)
+ # model = model_class.from_pretrained(
+ # model_args.model_name_or_path,
+ # config=config,
+ # revision=model_args.model_revision,
+ # )
+
+
+ else:
+ model_class = AUTO_MODELS[task_type]
+ model = model_class.from_pretrained(
+ model_args.model_name_or_path,
+ config=config,
+ revision=model_args.model_revision,
+ )
+
+ # bert_param = 0
+ if model_args.head_only and model_args.use_pe:
+ if config.model_type == "bert":
+ for param in model.bert.parameters():
+ param.requires_grad = False
+ # for _, param in model.bert.named_parameters():
+ # bert_param += param.numel()
+ elif config.model_type == "roberta":
+ for param in model.roberta.parameters():
+ param.requires_grad = False
+ # for _, param in model.roberta.named_parameters():
+ # bert_param += param.numel()
+ elif config.model_type == "deberta":
+ for param in model.deberta.parameters():
+ param.requires_grad = False
+ # for _, param in model.deberta.named_parameters():
+ # bert_param += param.numel()
+ all_param = 0
+ for _, param in model.named_parameters():
+ if param.requires_grad is True:
+ all_param += param.numel()
+ total_param = all_param
+ print('***** total param is {} *****'.format(total_param))
+ return model
+
+
+def get_model_deprecated(model_args, task_type: TaskType, config: AutoConfig, fix_bert: bool = False):
+ if model_args.prefix:
+ config.hidden_dropout_prob = model_args.hidden_dropout_prob
+ config.pre_seq_len = model_args.pre_seq_len
+ config.prefix_projection = model_args.prefix_projection
+ config.prefix_hidden_size = model_args.prefix_hidden_size
+
+ if task_type == TaskType.TOKEN_CLASSIFICATION:
+ from model.head_for_token_classification import BertPrefixModel, RobertaPrefixModel, DebertaPrefixModel, DebertaV2PrefixModel
+ elif task_type == TaskType.SEQUENCE_CLASSIFICATION:
+ from model.head_for_sequence_classification import BertPrefixModel, RobertaPrefixModel, DebertaPrefixModel, DebertaV2PrefixModel
+ elif task_type == TaskType.QUESTION_ANSWERING:
+ from model.head_for_question_answering import BertPrefixModel, RobertaPrefixModel, DebertaPrefixModel, DebertaV2PrefixModel
+ elif task_type == TaskType.MULTIPLE_CHOICE:
+ from model.multiple_choice import BertPrefixModel
+
+ if config.model_type == "bert":
+ model = BertPrefixModel.from_pretrained(
+ model_args.model_name_or_path,
+ config=config,
+ revision=model_args.model_revision,
+ )
+ elif config.model_type == "roberta":
+ model = RobertaPrefixModel.from_pretrained(
+ model_args.model_name_or_path,
+ config=config,
+ revision=model_args.model_revision,
+ )
+ elif config.model_type == "deberta":
+ model = DebertaPrefixModel.from_pretrained(
+ model_args.model_name_or_path,
+ config=config,
+ revision=model_args.model_revision,
+ )
+ elif config.model_type == "deberta-v2":
+ model = DebertaV2PrefixModel.from_pretrained(
+ model_args.model_name_or_path,
+ config=config,
+ revision=model_args.model_revision,
+ )
+ else:
+ raise NotImplementedError
+
+
+ elif model_args.prompt:
+ config.pre_seq_len = model_args.pre_seq_len
+
+ from model.head_for_sequence_classification import BertPromptModel, RobertaPromptModel
+ if config.model_type == "bert":
+ model = BertPromptModel.from_pretrained(
+ model_args.model_name_or_path,
+ config=config,
+ revision=model_args.model_revision,
+ )
+ elif config.model_type == "roberta":
+ model = RobertaPromptModel.from_pretrained(
+ model_args.model_name_or_path,
+ config=config,
+ revision=model_args.model_revision,
+ )
+ else:
+ raise NotImplementedError
+
+
+ else:
+ if task_type == TaskType.TOKEN_CLASSIFICATION:
+ model = AutoModelForTokenClassification.from_pretrained(
+ model_args.model_name_or_path,
+ config=config,
+ revision=model_args.model_revision,
+ )
+
+ elif task_type == TaskType.SEQUENCE_CLASSIFICATION:
+ model = AutoModelForSequenceClassification.from_pretrained(
+ model_args.model_name_or_path,
+ config=config,
+ revision=model_args.model_revision,
+ )
+
+ elif task_type == TaskType.QUESTION_ANSWERING:
+ model = AutoModelForQuestionAnswering.from_pretrained(
+ model_args.model_name_or_path,
+ config=config,
+ revision=model_args.model_revision,
+ )
+ elif task_type == TaskType.MULTIPLE_CHOICE:
+ model = AutoModelForMultipleChoice.from_pretrained(
+ model_args.model_name_or_path,
+ config=config,
+ revision=model_args.model_revision,
+ )
+
+ bert_param = 0
+ if fix_bert:
+ if config.model_type == "bert":
+ for param in model.bert.parameters():
+ param.requires_grad = False
+ for _, param in model.bert.named_parameters():
+ bert_param += param.numel()
+ elif config.model_type == "roberta":
+ for param in model.roberta.parameters():
+ param.requires_grad = False
+ for _, param in model.roberta.named_parameters():
+ bert_param += param.numel()
+ elif config.model_type == "deberta":
+ for param in model.deberta.parameters():
+ param.requires_grad = False
+ for _, param in model.deberta.named_parameters():
+ bert_param += param.numel()
+ all_param = 0
+ for _, param in model.named_parameters():
+ all_param += param.numel()
+ total_param = all_param - bert_param
+ print('***** total param is {} *****'.format(total_param))
+ return model
+
+
diff --git a/requirements.txt b/requirements.txt
new file mode 100644
index 0000000..93b243d
--- /dev/null
+++ b/requirements.txt
@@ -0,0 +1,5 @@
+datasets==1.15.1
+numpy==1.19.2
+tqdm==4.62.3
+transformers==4.11.3
+seqeval==1.2.2
diff --git a/run.py b/run.py
new file mode 100644
index 0000000..66aeedb
--- /dev/null
+++ b/run.py
@@ -0,0 +1,154 @@
+import logging
+import os
+import sys
+import numpy as np
+from typing import Dict
+
+import datasets
+import transformers
+from transformers import set_seed, Trainer
+from transformers.trainer_utils import get_last_checkpoint
+
+from arguments import get_args
+
+from tasks.utils import *
+
+os.environ["WANDB_DISABLED"] = "true"
+
+logger = logging.getLogger(__name__)
+
+def train(trainer, resume_from_checkpoint=None, last_checkpoint=None):
+ checkpoint = None
+ if resume_from_checkpoint is not None:
+ checkpoint = resume_from_checkpoint
+ elif last_checkpoint is not None:
+ checkpoint = last_checkpoint
+ train_result = trainer.train(resume_from_checkpoint=checkpoint)
+ # trainer.save_model(),
+
+ try:
+ metrics = train_result.metrics
+
+ trainer.log_metrics("train", metrics)
+ trainer.save_metrics("train", metrics)
+ trainer.save_state()
+
+ trainer.log_best_metrics()
+ except:
+ pass
+
+def evaluate(trainer):
+ logger.info("*** Evaluate ***")
+ metrics = trainer.evaluate()
+
+ trainer.log_metrics("eval", metrics)
+ trainer.save_metrics("eval", metrics)
+
+def predict(trainer, predict_dataset=None):
+ if predict_dataset is None:
+ logger.info("No dataset is available for testing")
+
+ elif isinstance(predict_dataset, dict):
+
+ for dataset_name, d in predict_dataset.items():
+ logger.info("*** Predict: %s ***" % dataset_name)
+ predictions, labels, metrics = trainer.predict(d, metric_key_prefix="predict")
+ predictions = np.argmax(predictions, axis=2)
+
+ trainer.log_metrics("predict", metrics)
+ trainer.save_metrics("predict", metrics)
+
+ else:
+ logger.info("*** Predict ***")
+ predictions, labels, metrics = trainer.predict(predict_dataset, metric_key_prefix="predict")
+ predictions = np.argmax(predictions, axis=2)
+
+ trainer.log_metrics("predict", metrics)
+ trainer.save_metrics("predict", metrics)
+
+if __name__ == '__main__':
+
+ args = get_args()
+
+ _, data_args, training_args, semi_training_args, _ = args
+
+ logging.basicConfig(
+ format="%(asctime)s - %(levelname)s - %(name)s - %(message)s",
+ datefmt="%m/%d/%Y %H:%M:%S",
+ handlers=[logging.StreamHandler(sys.stdout)],
+ )
+
+ log_level = training_args.get_process_log_level()
+ logger.setLevel(log_level)
+ datasets.utils.logging.set_verbosity(log_level)
+ transformers.utils.logging.set_verbosity(log_level)
+ transformers.utils.logging.enable_default_handler()
+ transformers.utils.logging.enable_explicit_format()
+
+ # Log on each process the small summary:
+ logger.warning(
+ f"Process rank: {training_args.local_rank}, device: {training_args.device}, n_gpu: {training_args.n_gpu}"
+ + f"distributed training: {bool(training_args.local_rank != -1)}, 16-bits training: {training_args.fp16}"
+ )
+ logger.info(f"Training/evaluation parameters {training_args}")
+
+
+ if not os.path.isdir("checkpoints") or not os.path.exists("checkpoints"):
+ os.mkdir("checkpoints")
+
+ if data_args.task_name.lower() == "superglue":
+ assert data_args.dataset_name.lower() in SUPERGLUE_DATASETS
+ from tasks.superglue.get_trainer import get_trainer
+
+ elif data_args.task_name.lower() == "glue":
+ assert data_args.dataset_name.lower() in GLUE_DATASETS
+ from tasks.glue.get_trainer import get_trainer
+
+ elif data_args.task_name.lower() == "ner":
+ assert data_args.dataset_name.lower() in NER_DATASETS
+ from tasks.ner.get_trainer import get_trainer
+
+ elif data_args.task_name.lower() == "srl":
+ assert data_args.dataset_name.lower() in SRL_DATASETS
+ from tasks.srl.get_trainer import get_trainer
+
+ elif data_args.task_name.lower() == "qa":
+ assert data_args.dataset_name.lower() in QA_DATASETS
+ from tasks.qa.get_trainer import get_trainer
+
+ elif data_args.task_name.lower() == "other_cls":
+ assert data_args.dataset_name.lower() in OTHER_DATASETS
+ from tasks.other_cls.get_trainer import get_trainer
+
+ else:
+ raise NotImplementedError('Task {} is not implemented. Please choose a task from: {}'.format(data_args.task_name, ", ".join(TASKS)))
+
+ set_seed(training_args.seed)
+
+ trainer, predict_dataset = get_trainer(args)
+
+ last_checkpoint = None
+ if os.path.isdir(training_args.output_dir) and training_args.do_train and not training_args.overwrite_output_dir:
+ last_checkpoint = get_last_checkpoint(training_args.output_dir)
+ if last_checkpoint is None and len(os.listdir(training_args.output_dir)) > 0:
+ raise ValueError(
+ f"Output directory ({training_args.output_dir}) already exists and is not empty. "
+ "Use --overwrite_output_dir to overcome."
+ )
+ elif last_checkpoint is not None and training_args.resume_from_checkpoint is None:
+ logger.info(
+ f"Checkpoint detected, resuming training at {last_checkpoint}. To avoid this behavior, change "
+ "the `--output_dir` or add `--overwrite_output_dir` to train from scratch."
+ )
+
+
+ if training_args.do_train:
+ train(trainer, training_args.resume_from_checkpoint, last_checkpoint)
+
+ # if training_args.do_eval:
+ # evaluate(trainer)
+
+ # if training_args.do_predict:
+ # predict(trainer, predict_dataset)
+
+
\ No newline at end of file
diff --git a/run_script/run_boolq_bert.sh b/run_script/run_boolq_bert.sh
new file mode 100644
index 0000000..c1f1b22
--- /dev/null
+++ b/run_script/run_boolq_bert.sh
@@ -0,0 +1,28 @@
+export TASK_NAME=superglue
+export DATASET_NAME=boolq
+export CUDA_VISIBLE_DEVICES=0
+
+bs=32
+lr=5e-3
+dropout=0.1
+psl=40
+epoch=100
+
+python3 run.py \
+ --model_name_or_path bert-large-cased \
+ --task_name $TASK_NAME \
+ --dataset_name $DATASET_NAME \
+ --do_train \
+ --do_eval \
+ --max_seq_length 128 \
+ --per_device_train_batch_size $bs \
+ --learning_rate $lr \
+ --num_train_epochs $epoch \
+ --pre_seq_len $psl \
+ --output_dir checkpoints/$DATASET_NAME-bert/ \
+ --overwrite_output_dir \
+ --hidden_dropout_prob $dropout \
+ --seed 11 \
+ --save_strategy no \
+ --evaluation_strategy epoch \
+ --prefix
diff --git a/run_script/run_boolq_roberta.sh b/run_script/run_boolq_roberta.sh
new file mode 100644
index 0000000..68b2a6c
--- /dev/null
+++ b/run_script/run_boolq_roberta.sh
@@ -0,0 +1,28 @@
+export TASK_NAME=superglue
+export DATASET_NAME=boolq
+export CUDA_VISIBLE_DEVICES=0
+
+bs=32
+lr=7e-3
+dropout=0.1
+psl=8
+epoch=100
+
+python3 run.py \
+ --model_name_or_path roberta-large \
+ --task_name $TASK_NAME \
+ --dataset_name $DATASET_NAME \
+ --do_train \
+ --do_eval \
+ --max_seq_length 128 \
+ --per_device_train_batch_size $bs \
+ --learning_rate $lr \
+ --num_train_epochs $epoch \
+ --pre_seq_len $psl \
+ --output_dir checkpoints/$DATASET_NAME-roberta/ \
+ --overwrite_output_dir \
+ --hidden_dropout_prob $dropout \
+ --seed 11 \
+ --save_strategy no \
+ --evaluation_strategy epoch \
+ --prefix
diff --git a/run_script/run_conll03_roberta.sh b/run_script/run_conll03_roberta.sh
new file mode 100644
index 0000000..7466809
--- /dev/null
+++ b/run_script/run_conll03_roberta.sh
@@ -0,0 +1,29 @@
+export TASK_NAME=ner
+export DATASET_NAME=conll2003
+export CUDA_VISIBLE_DEVICES=0
+
+bs=16
+epoch=30
+psl=11
+lr=3e-2
+dropout=0.1
+
+python3 run.py \
+ --model_name_or_path roberta-large \
+ --task_name $TASK_NAME \
+ --dataset_name $DATASET_NAME \
+ --do_train \
+ --do_eval \
+ --do_predict \
+ --max_seq_length 152 \
+ --per_device_train_batch_size $bs \
+ --learning_rate $lr \
+ --num_train_epochs $epoch \
+ --pre_seq_len $psl \
+ --output_dir checkpoints/$DATASET_NAME/ \
+ --overwrite_output_dir \
+ --hidden_dropout_prob $dropout \
+ --seed 11 \
+ --save_strategy no \
+ --evaluation_strategy epoch \
+ --prefix
diff --git a/run_script/run_conll04_bert.sh b/run_script/run_conll04_bert.sh
new file mode 100644
index 0000000..ed25b19
--- /dev/null
+++ b/run_script/run_conll04_bert.sh
@@ -0,0 +1,29 @@
+export TASK_NAME=ner
+export DATASET_NAME=conll2004
+export CUDA_VISIBLE_DEVICES=0
+
+bs=32
+lr=2e-2
+dropout=0.2
+psl=128
+epoch=40
+
+python3 run.py \
+ --model_name_or_path bert-large-uncased \
+ --task_name $TASK_NAME \
+ --dataset_name $DATASET_NAME \
+ --do_train \
+ --do_eval \
+ --do_predict \
+ --max_seq_length 128 \
+ --per_device_train_batch_size $bs \
+ --learning_rate $lr \
+ --num_train_epochs $epoch \
+ --pre_seq_len $psl \
+ --output_dir checkpoints/$DATASET_NAME/ \
+ --overwrite_output_dir \
+ --hidden_dropout_prob $dropout \
+ --seed 11 \
+ --save_strategy no \
+ --evaluation_strategy epoch \
+ --prefix
diff --git a/run_script/run_conll04_roberta.sh b/run_script/run_conll04_roberta.sh
new file mode 100644
index 0000000..470704f
--- /dev/null
+++ b/run_script/run_conll04_roberta.sh
@@ -0,0 +1,30 @@
+export TASK_NAME=ner
+export DATASET_NAME=conll2004
+export CUDA_VISIBLE_DEVICES=0
+
+bs=32
+lr=6e-2
+dropout=0.1
+psl=144
+epoch=80
+
+python3 run.py \
+ --model_name_or_path roberta-large \
+ --task_name $TASK_NAME \
+ --dataset_name $DATASET_NAME \
+ --do_train \
+ --do_eval \
+ --do_predict \
+ --max_seq_length 128 \
+ --per_device_train_batch_size $bs \
+ --learning_rate $lr \
+ --num_train_epochs $epoch \
+ --pre_seq_len $psl \
+ --output_dir checkpoints/$DATASET_NAME/ \
+ --overwrite_output_dir \
+ --hidden_dropout_prob $dropout \
+ --seed 11 \
+ --save_strategy no \
+ --evaluation_strategy epoch \
+ --prefix
+
diff --git a/run_script/run_conll05_roberta.sh b/run_script/run_conll05_roberta.sh
new file mode 100644
index 0000000..494a578
--- /dev/null
+++ b/run_script/run_conll05_roberta.sh
@@ -0,0 +1,29 @@
+export TASK_NAME=srl
+export DATASET_NAME=conll2005
+export CUDA_VISIBLE_DEVICES=0
+
+bs=16
+lr=6e-3
+dropout=0.1
+psl=224
+epoch=15
+
+python3 run.py \
+ --model_name_or_path roberta-large \
+ --task_name $TASK_NAME \
+ --dataset_name $DATASET_NAME \
+ --do_train \
+ --do_eval \
+ --do_predict \
+ --max_seq_length 128 \
+ --per_device_train_batch_size $bs \
+ --learning_rate $lr \
+ --num_train_epochs $epoch \
+ --pre_seq_len $psl \
+ --output_dir checkpoints/$DATASET_NAME/ \
+ --overwrite_output_dir \
+ --hidden_dropout_prob $dropout \
+ --seed 11 \
+ --save_strategy no \
+ --evaluation_strategy epoch \
+ --prefix
diff --git a/run_script/run_conll12_bert.sh b/run_script/run_conll12_bert.sh
new file mode 100644
index 0000000..b02aca6
--- /dev/null
+++ b/run_script/run_conll12_bert.sh
@@ -0,0 +1,29 @@
+export TASK_NAME=srl
+export DATASET_NAME=conll2012
+export CUDA_VISIBLE_DEVICES=0
+
+bs=16
+lr=5e-3
+dropout=0.1
+psl=128
+epoch=45
+
+python3 run.py \
+ --model_name_or_path roberta-large \
+ --task_name $TASK_NAME \
+ --dataset_name $DATASET_NAME \
+ --do_train \
+ --do_eval \
+ --do_predict \
+ --max_seq_length 128 \
+ --per_device_train_batch_size $bs \
+ --learning_rate $lr \
+ --num_train_epochs $epoch \
+ --pre_seq_len $psl \
+ --output_dir checkpoints/$DATASET_NAME/ \
+ --overwrite_output_dir \
+ --hidden_dropout_prob $dropout \
+ --seed 11 \
+ --save_strategy no \
+ --evaluation_strategy epoch \
+ --prefix
diff --git a/run_script/run_conll12_roberta.sh b/run_script/run_conll12_roberta.sh
new file mode 100644
index 0000000..c90a36e
--- /dev/null
+++ b/run_script/run_conll12_roberta.sh
@@ -0,0 +1,29 @@
+export TASK_NAME=srl
+export DATASET_NAME=conll2012
+export CUDA_VISIBLE_DEVICES=0
+
+bs=32
+lr=5e-3
+dropout=0.1
+psl=64
+epoch=45
+
+python3 run.py \
+ --model_name_or_path roberta-large \
+ --task_name $TASK_NAME \
+ --dataset_name $DATASET_NAME \
+ --do_train \
+ --do_eval \
+ --do_predict \
+ --max_seq_length 128 \
+ --per_device_train_batch_size $bs \
+ --learning_rate $lr \
+ --num_train_epochs $epoch \
+ --pre_seq_len $psl \
+ --output_dir checkpoints/$DATASET_NAME/ \
+ --overwrite_output_dir \
+ --hidden_dropout_prob $dropout \
+ --seed 11 \
+ --save_strategy no \
+ --evaluation_strategy epoch \
+ --prefix
diff --git a/run_script/run_copa_bert.sh b/run_script/run_copa_bert.sh
new file mode 100644
index 0000000..8bfc024
--- /dev/null
+++ b/run_script/run_copa_bert.sh
@@ -0,0 +1,28 @@
+export TASK_NAME=superglue
+export DATASET_NAME=copa
+export CUDA_VISIBLE_DEVICES=0
+
+bs=16
+lr=1e-2
+dropout=0.1
+psl=16
+epoch=80
+
+python3 run.py \
+ --model_name_or_path bert-large-cased \
+ --task_name $TASK_NAME \
+ --dataset_name $DATASET_NAME \
+ --do_train \
+ --do_eval \
+ --max_seq_length 128 \
+ --per_device_train_batch_size $bs \
+ --learning_rate $lr \
+ --num_train_epochs $epoch \
+ --pre_seq_len $psl \
+ --output_dir checkpoints/$DATASET_NAME-bert/ \
+ --overwrite_output_dir \
+ --hidden_dropout_prob $dropout \
+ --seed 44 \
+ --save_strategy no \
+ --evaluation_strategy epoch \
+ --prefix
diff --git a/run_script/run_copa_roberta.sh b/run_script/run_copa_roberta.sh
new file mode 100644
index 0000000..ae4f1e8
--- /dev/null
+++ b/run_script/run_copa_roberta.sh
@@ -0,0 +1,28 @@
+export TASK_NAME=superglue
+export DATASET_NAME=copa
+export CUDA_VISIBLE_DEVICES=0
+
+bs=16
+lr=9e-3
+dropout=0.1
+psl=8
+epoch=120
+
+python3 run.py \
+ --model_name_or_path roberta-large \
+ --task_name $TASK_NAME \
+ --dataset_name $DATASET_NAME \
+ --do_train \
+ --do_eval \
+ --max_seq_length 128 \
+ --per_device_train_batch_size $bs \
+ --learning_rate $lr \
+ --num_train_epochs $epoch \
+ --pre_seq_len $psl \
+ --output_dir checkpoints/$DATASET_NAME-roberta/ \
+ --overwrite_output_dir \
+ --hidden_dropout_prob $dropout \
+ --seed 11 \
+ --save_strategy no \
+ --evaluation_strategy epoch \
+ --prefix
diff --git a/run_script/run_ontonotes_bert.sh b/run_script/run_ontonotes_bert.sh
new file mode 100644
index 0000000..9362b19
--- /dev/null
+++ b/run_script/run_ontonotes_bert.sh
@@ -0,0 +1,29 @@
+export TASK_NAME=ner
+export DATASET_NAME=ontonotes
+export CUDA_VISIBLE_DEVICES=0
+
+bs=16
+lr=1e-2
+dropout=0.1
+psl=16
+epoch=30
+
+python3 run.py \
+ --model_name_or_path bert-large-uncased \
+ --task_name $TASK_NAME \
+ --dataset_name $DATASET_NAME \
+ --do_train \
+ --do_eval \
+ --do_predict \
+ --max_seq_length 128 \
+ --per_device_train_batch_size $bs \
+ --learning_rate $lr \
+ --num_train_epochs $epoch \
+ --pre_seq_len $psl \
+ --output_dir checkpoints/$DATASET_NAME/ \
+ --overwrite_output_dir \
+ --hidden_dropout_prob $dropout \
+ --seed 11 \
+ --save_strategy no \
+ --evaluation_strategy epoch \
+ --prefix
diff --git a/run_script/run_ontonotes_roberta.sh b/run_script/run_ontonotes_roberta.sh
new file mode 100644
index 0000000..fe58854
--- /dev/null
+++ b/run_script/run_ontonotes_roberta.sh
@@ -0,0 +1,29 @@
+export TASK_NAME=ner
+export DATASET_NAME=ontonotes
+export CUDA_VISIBLE_DEVICES=0
+
+bs=16
+lr=7e-3
+dropout=0.1
+psl=48
+epoch=60
+
+python3 run.py \
+ --model_name_or_path roberta-large \
+ --task_name $TASK_NAME \
+ --dataset_name $DATASET_NAME \
+ --do_train \
+ --do_eval \
+ --do_predict \
+ --max_seq_length 128 \
+ --per_device_train_batch_size $bs \
+ --learning_rate $lr \
+ --num_train_epochs $epoch \
+ --pre_seq_len $psl \
+ --output_dir checkpoints/$DATASET_NAME-roberta/ \
+ --overwrite_output_dir \
+ --hidden_dropout_prob $dropout \
+ --seed 14 \
+ --save_strategy no \
+ --evaluation_strategy epoch \
+ --prefix
diff --git a/run_script/run_rte_bert.sh b/run_script/run_rte_bert.sh
new file mode 100644
index 0000000..a8327fa
--- /dev/null
+++ b/run_script/run_rte_bert.sh
@@ -0,0 +1,28 @@
+export TASK_NAME=superglue
+export DATASET_NAME=rte
+export CUDA_VISIBLE_DEVICES=0
+
+bs=32
+lr=1e-2
+dropout=0.1
+psl=20
+epoch=60
+
+python3 run.py \
+ --model_name_or_path bert-large-cased \
+ --task_name $TASK_NAME \
+ --dataset_name $DATASET_NAME \
+ --do_train \
+ --do_eval \
+ --max_seq_length 128 \
+ --per_device_train_batch_size $bs \
+ --learning_rate $lr \
+ --num_train_epochs $epoch \
+ --pre_seq_len $psl \
+ --output_dir checkpoints/$DATASET_NAME-bert/ \
+ --overwrite_output_dir \
+ --hidden_dropout_prob $dropout \
+ --seed 11 \
+ --save_strategy no \
+ --evaluation_strategy epoch \
+ --prefix
diff --git a/run_script/run_rte_roberta.sh b/run_script/run_rte_roberta.sh
new file mode 100644
index 0000000..2f8aa46
--- /dev/null
+++ b/run_script/run_rte_roberta.sh
@@ -0,0 +1,28 @@
+export TASK_NAME=superglue
+export DATASET_NAME=rte
+export CUDA_VISIBLE_DEVICES=7
+
+bs=32
+lr=5e-3
+dropout=0.1
+psl=128
+epoch=100
+
+python3 run.py \
+ --model_name_or_path /wjn/pre-trained-lm/roberta-large \
+ --task_name $TASK_NAME \
+ --dataset_name $DATASET_NAME \
+ --do_train \
+ --do_eval \
+ --max_seq_length 128 \
+ --per_device_train_batch_size $bs \
+ --learning_rate $lr \
+ --num_train_epochs $epoch \
+ --pre_seq_len $psl \
+ --output_dir checkpoints/$DATASET_NAME-roberta/ \
+ --overwrite_output_dir \
+ --hidden_dropout_prob $dropout \
+ --seed 11 \
+ --save_strategy no \
+ --evaluation_strategy epoch \
+ --head_prefix \
diff --git a/run_script/run_squad_roberta.sh b/run_script/run_squad_roberta.sh
new file mode 100644
index 0000000..cb0222e
--- /dev/null
+++ b/run_script/run_squad_roberta.sh
@@ -0,0 +1,27 @@
+export TASK_NAME=qa
+export DATASET_NAME=squad
+export CUDA_VISIBLE_DEVICES=0
+
+bs=8
+lr=5e-3
+dropout=0.2
+psl=16
+epoch=30
+
+python3 run.py \
+ --model_name_or_path roberta-large \
+ --task_name $TASK_NAME \
+ --dataset_name $DATASET_NAME \
+ --do_train \
+ --do_eval \
+ --per_device_train_batch_size $bs \
+ --learning_rate $lr \
+ --num_train_epochs $epoch \
+ --pre_seq_len $psl \
+ --output_dir checkpoints/$DATASET_NAME/ \
+ --overwrite_output_dir \
+ --hidden_dropout_prob $dropout \
+ --seed 11 \
+ --save_strategy no \
+ --evaluation_strategy epoch \
+ --prefix
diff --git a/run_script/run_squad_v2_roberta.sh b/run_script/run_squad_v2_roberta.sh
new file mode 100644
index 0000000..c5d2445
--- /dev/null
+++ b/run_script/run_squad_v2_roberta.sh
@@ -0,0 +1,27 @@
+export TASK_NAME=qa
+export DATASET_NAME=squad_v2
+export CUDA_VISIBLE_DEVICES=0
+
+bs=8
+lr=5e-3
+dropout=0.2
+psl=8
+epoch=10
+
+python3 run.py \
+ --model_name_or_path /wjn/pre-trained-lm/roberta-large \
+ --task_name $TASK_NAME \
+ --dataset_name $DATASET_NAME \
+ --do_train \
+ --do_eval \
+ --per_device_train_batch_size $bs \
+ --learning_rate $lr \
+ --num_train_epochs $epoch \r
+ --pre_seq_len $psl \
+ --output_dir checkpoints/$DATASET_NAME/ \
+ --overwrite_output_dir \
+ --hidden_dropout_prob $dropout \
+ --seed 11 \
+ --save_strategy no \
+ --evaluation_strategy epoch \
+ --prefix
diff --git a/run_script/run_wic_bert.sh b/run_script/run_wic_bert.sh
new file mode 100644
index 0000000..498832b
--- /dev/null
+++ b/run_script/run_wic_bert.sh
@@ -0,0 +1,30 @@
+export TASK_NAME=superglue
+export DATASET_NAME=wic
+export CUDA_VISIBLE_DEVICES=0
+
+bs=16
+lr=1e-4
+dropout=0.1
+psl=20
+epoch=80
+
+python3 run.py \
+ --model_name_or_path bert-large-cased \
+ --task_name $TASK_NAME \
+ --dataset_name $DATASET_NAME \
+ --do_train \
+ --do_eval \
+ --max_seq_length 128 \
+ --per_device_train_batch_size $bs \
+ --learning_rate $lr \
+ --num_train_epochs $epoch \
+ --pre_seq_len $psl \
+ --output_dir checkpoints/$DATASET_NAME-bert/ \
+ --overwrite_output_dir \
+ --hidden_dropout_prob $dropout \
+ --seed 44 \
+ --save_strategy no \
+ --evaluation_strategy epoch \
+ --prefix \
+ --prefix_projection \
+ --template_id 1
diff --git a/run_script/run_wic_roberta.sh b/run_script/run_wic_roberta.sh
new file mode 100644
index 0000000..108ee80
--- /dev/null
+++ b/run_script/run_wic_roberta.sh
@@ -0,0 +1,28 @@
+export TASK_NAME=superglue
+export DATASET_NAME=wic
+export CUDA_VISIBLE_DEVICES=0
+
+bs=32
+lr=1e-2
+dropout=0.1
+psl=8
+epoch=50
+
+python3 run.py \
+ --model_name_or_path roberta-large \
+ --task_name $TASK_NAME \
+ --dataset_name $DATASET_NAME \
+ --do_train \
+ --do_eval \
+ --max_seq_length 128 \
+ --per_device_train_batch_size $bs \
+ --learning_rate $lr \
+ --num_train_epochs $epoch \
+ --pre_seq_len $psl \
+ --output_dir checkpoints/$DATASET_NAME-roberta/ \
+ --overwrite_output_dir \
+ --hidden_dropout_prob $dropout \
+ --seed 11 \
+ --save_strategy no \
+ --evaluation_strategy epoch \
+ --prefix
diff --git a/run_script/run_wsc_bert.sh b/run_script/run_wsc_bert.sh
new file mode 100644
index 0000000..4a3c136
--- /dev/null
+++ b/run_script/run_wsc_bert.sh
@@ -0,0 +1,28 @@
+export TASK_NAME=superglue
+export DATASET_NAME=wsc
+export CUDA_VISIBLE_DEVICES=0
+
+bs=16
+lr=5e-3
+dropout=0.1
+psl=20
+epoch=80
+
+python3 run.py \
+ --model_name_or_path bert-large-cased \
+ --task_name $TASK_NAME \
+ --dataset_name $DATASET_NAME \
+ --do_train \
+ --do_eval \
+ --max_seq_length 128 \
+ --per_device_train_batch_size $bs \
+ --learning_rate $lr \
+ --num_train_epochs $epoch \
+ --pre_seq_len $psl \
+ --output_dir checkpoints/$DATASET_NAME-bert/ \
+ --overwrite_output_dir \
+ --hidden_dropout_prob $dropout \
+ --seed 44 \
+ --save_strategy no \
+ --evaluation_strategy epoch \
+ --prefix
diff --git a/run_script/run_wsc_roberta.sh b/run_script/run_wsc_roberta.sh
new file mode 100644
index 0000000..55764d6
--- /dev/null
+++ b/run_script/run_wsc_roberta.sh
@@ -0,0 +1,28 @@
+export TASK_NAME=superglue
+export DATASET_NAME=wsc
+export CUDA_VISIBLE_DEVICES=0
+
+bs=16
+lr=1e-2
+dropout=0.1
+psl=8
+epoch=10
+
+python3 run.py \
+ --model_name_or_path roberta-large \
+ --task_name $TASK_NAME \
+ --dataset_name $DATASET_NAME \
+ --do_train \
+ --do_eval \
+ --max_seq_length 128 \
+ --per_device_train_batch_size $bs \
+ --learning_rate $lr \
+ --num_train_epochs $epoch \
+ --pre_seq_len $psl \
+ --output_dir checkpoints/$DATASET_NAME-bert/ \
+ --overwrite_output_dir \
+ --hidden_dropout_prob $dropout \
+ --seed 44 \
+ --save_strategy no \
+ --evaluation_strategy epoch \
+ --prefix
diff --git a/run_script_fewshot/run_agnews_roberta.sh b/run_script_fewshot/run_agnews_roberta.sh
new file mode 100644
index 0000000..352fe93
--- /dev/null
+++ b/run_script_fewshot/run_agnews_roberta.sh
@@ -0,0 +1,36 @@
+export TASK_NAME=other_cls
+export DATASET_NAME=ag_news
+export CUDA_VISIBLE_DEVICES=0
+
+bs=4
+lr=5e-5
+dropout=0.1
+psl=16
+epoch=100
+
+python3 run.py \
+ --model_name_or_path /wjn/pre-trained-lm/roberta-large \
+ --task_name $TASK_NAME \
+ --dataset_name $DATASET_NAME \
+ --overwrite_cache \
+ --do_train \
+ --do_eval \
+ --do_predict \
+ --max_seq_length 128 \
+ --per_device_train_batch_size $bs \
+ --learning_rate $lr \
+ --num_train_epochs $epoch \
+ --pre_seq_len $psl \
+ --output_dir checkpoints/$DATASET_NAME-roberta/ \
+ --overwrite_output_dir \
+ --hidden_dropout_prob $dropout \
+ --seed 42 \
+ --save_strategy no \
+ --evaluation_strategy epoch \
+ --num_examples_per_label 16 \
+ --head_only \
+ # --use_pe
+ # --adapter \
+ # --adapter_dim 256 \
+ # --adapter_choice list \
+
diff --git a/run_script_fewshot/run_boolq_roberta.sh b/run_script_fewshot/run_boolq_roberta.sh
new file mode 100644
index 0000000..fc4aa17
--- /dev/null
+++ b/run_script_fewshot/run_boolq_roberta.sh
@@ -0,0 +1,39 @@
+export TASK_NAME=superglue
+export DATASET_NAME=boolq
+export CUDA_VISIBLE_DEVICES=2
+
+bs=4
+lr=5e-5
+dropout=0.1
+psl=20
+epoch=100
+
+python3 run.py \
+ --model_name_or_path /wjn/pre-trained-lm/roberta-large \
+ --task_name $TASK_NAME \
+ --dataset_name $DATASET_NAME \
+ --overwrite_cache \
+ --do_train \
+ --do_eval \
+ --do_predict \
+ --max_seq_length 384 \
+ --per_device_train_batch_size $bs \
+ --learning_rate $lr \
+ --num_train_epochs $epoch \
+ --pre_seq_len $psl \
+ --output_dir checkpoints/$DATASET_NAME-roberta/ \
+ --overwrite_output_dir \
+ --hidden_dropout_prob $dropout \
+ --seed 42 \
+ --save_strategy no \
+ --evaluation_strategy epoch \
+ --num_examples_per_label 16 \
+ --prompt_ptuning \
+ # --use_pe
+
+ # --head_prefix \
+ # --use_pe
+ # --adapter \
+ # --adapter_dim 256 \
+ # --adapter_choice list \
+
diff --git a/run_script_fewshot/run_cb_roberta.sh b/run_script_fewshot/run_cb_roberta.sh
new file mode 100644
index 0000000..d551044
--- /dev/null
+++ b/run_script_fewshot/run_cb_roberta.sh
@@ -0,0 +1,41 @@
+export TASK_NAME=superglue
+export DATASET_NAME=cb
+export CUDA_VISIBLE_DEVICES=0
+# export CUDA_VISIBLE_DEVICES=2
+# export CUDA_VISIBLE_DEVICES=5
+
+bs=4
+lr=5e-6
+dropout=0.1
+psl=28
+epoch=100
+
+python3 run.py \
+ --model_name_or_path /wjn/pre-trained-lm/roberta-large \
+ --task_name $TASK_NAME \
+ --dataset_name $DATASET_NAME \
+ --overwrite_cache \
+ --do_train \
+ --do_eval \
+ --do_predict \
+ --max_seq_length 128 \
+ --per_device_train_batch_size $bs \
+ --learning_rate $lr \
+ --num_train_epochs $epoch \
+ --pre_seq_len $psl \
+ --output_dir checkpoints/$DATASET_NAME-roberta/ \
+ --overwrite_output_dir \
+ --hidden_dropout_prob $dropout \
+ --seed 42 \
+ --save_strategy no \
+ --evaluation_strategy epoch \
+ --num_examples_per_label 16 \
+ --prompt_only \
+ # --use_pe
+
+ # --head_prefix \
+ # --use_pe
+ # --adapter \
+ # --adapter_dim 256 \
+ # --adapter_choice list \
+
diff --git a/run_script_fewshot/run_copa_roberta.sh b/run_script_fewshot/run_copa_roberta.sh
new file mode 100644
index 0000000..29953bb
--- /dev/null
+++ b/run_script_fewshot/run_copa_roberta.sh
@@ -0,0 +1,39 @@
+export TASK_NAME=superglue
+export DATASET_NAME=copa
+export CUDA_VISIBLE_DEVICES=6
+
+bs=4
+lr=5e-5
+dropout=0.1
+psl=8
+epoch=100
+
+python3 run.py \
+ --model_name_or_path /wjn/pre-trained-lm/roberta-large \
+ --task_name $TASK_NAME \
+ --dataset_name $DATASET_NAME \
+ --overwrite_cache \
+ --do_train \
+ --do_eval \
+ --do_predict \
+ --max_seq_length 384 \
+ --per_device_train_batch_size $bs \
+ --learning_rate $lr \
+ --num_train_epochs $epoch \
+ --pre_seq_len $psl \
+ --output_dir checkpoints/$DATASET_NAME-roberta/ \
+ --overwrite_output_dir \
+ --hidden_dropout_prob $dropout \
+ --seed 42 \
+ --save_strategy no \
+ --evaluation_strategy epoch \
+ --num_examples_per_label 16 \
+ --head_prefix \
+ --use_pe
+
+ # --head_prefix \
+ # --use_pe
+ # --adapter \
+ # --adapter_dim 256 \
+ # --adapter_choice list \
+
diff --git a/run_script_fewshot/run_mnli_roberta.sh b/run_script_fewshot/run_mnli_roberta.sh
new file mode 100644
index 0000000..12e6bf2
--- /dev/null
+++ b/run_script_fewshot/run_mnli_roberta.sh
@@ -0,0 +1,39 @@
+export TASK_NAME=glue
+export DATASET_NAME=mnli
+export CUDA_VISIBLE_DEVICES=1
+
+bs=4
+lr=5e-5
+dropout=0.1
+psl=20
+epoch=100
+
+python3 run.py \
+ --model_name_or_path /wjn/pre-trained-lm/roberta-large \
+ --task_name $TASK_NAME \
+ --dataset_name $DATASET_NAME \
+ --overwrite_cache \
+ --do_train \
+ --do_eval \
+ --do_predict \
+ --max_seq_length 128 \
+ --per_device_train_batch_size $bs \
+ --learning_rate $lr \
+ --num_train_epochs $epoch \
+ --pre_seq_len $psl \
+ --output_dir checkpoints/$DATASET_NAME-roberta/ \
+ --overwrite_output_dir \
+ --hidden_dropout_prob $dropout \
+ --seed 42 \
+ --save_strategy no \
+ --evaluation_strategy epoch \
+ --num_examples_per_label 16 \
+ --prompt_ptuning \
+ --use_pe
+
+ # --head_prefix \
+ # --use_pe
+ # --adapter \
+ # --adapter_dim 256 \
+ # --adapter_choice list \
+
diff --git a/run_script_fewshot/run_mr_roberta.sh b/run_script_fewshot/run_mr_roberta.sh
new file mode 100644
index 0000000..c65f512
--- /dev/null
+++ b/run_script_fewshot/run_mr_roberta.sh
@@ -0,0 +1,36 @@
+export TASK_NAME=other_cls
+export DATASET_NAME=movie_rationales
+export CUDA_VISIBLE_DEVICES=0
+
+bs=4
+lr=5e-5
+dropout=0.1
+psl=16
+epoch=100
+
+python3 run.py \
+ --model_name_or_path /wjn/pre-trained-lm/roberta-large \
+ --task_name $TASK_NAME \
+ --dataset_name $DATASET_NAME \
+ --overwrite_cache \
+ --do_train \
+ --do_eval \
+ --do_predict \
+ --max_seq_length 256 \
+ --per_device_train_batch_size $bs \
+ --learning_rate $lr \
+ --num_train_epochs $epoch \
+ --pre_seq_len $psl \
+ --output_dir checkpoints/$DATASET_NAME-roberta/ \
+ --overwrite_output_dir \
+ --hidden_dropout_prob $dropout \
+ --seed 42 \
+ --save_strategy no \
+ --evaluation_strategy epoch \
+ --num_examples_per_label 16 \
+ --prompt_ptuning \
+ # --use_pe
+ # --adapter \
+ # --adapter_dim 256 \
+ # --adapter_choice list \
+
diff --git a/run_script_fewshot/run_mrpc_roberta.sh b/run_script_fewshot/run_mrpc_roberta.sh
new file mode 100644
index 0000000..4284940
--- /dev/null
+++ b/run_script_fewshot/run_mrpc_roberta.sh
@@ -0,0 +1,39 @@
+export TASK_NAME=glue
+export DATASET_NAME=mrpc
+export CUDA_VISIBLE_DEVICES=1
+
+bs=4
+lr=5e-5
+dropout=0.1
+psl=20
+epoch=100
+
+python3 run.py \
+ --model_name_or_path /wjn/pre-trained-lm/roberta-large \
+ --task_name $TASK_NAME \
+ --dataset_name $DATASET_NAME \
+ --overwrite_cache \
+ --do_train \
+ --do_eval \
+ --do_predict \
+ --max_seq_length 128 \
+ --per_device_train_batch_size $bs \
+ --learning_rate $lr \
+ --num_train_epochs $epoch \
+ --pre_seq_len $psl \
+ --output_dir checkpoints/$DATASET_NAME-roberta/ \
+ --overwrite_output_dir \
+ --hidden_dropout_prob $dropout \
+ --seed 42 \
+ --save_strategy no \
+ --evaluation_strategy epoch \
+ --num_examples_per_label 16 \
+ --prompt_ptuning \
+ --use_pe
+
+ # --head_prefix \
+ # --use_pe
+ # --adapter \
+ # --adapter_dim 256 \
+ # --adapter_choice list \
+
diff --git a/run_script_fewshot/run_qnli_roberta.sh b/run_script_fewshot/run_qnli_roberta.sh
new file mode 100644
index 0000000..1585b62
--- /dev/null
+++ b/run_script_fewshot/run_qnli_roberta.sh
@@ -0,0 +1,39 @@
+export TASK_NAME=glue
+export DATASET_NAME=qnli
+export CUDA_VISIBLE_DEVICES=1
+
+bs=4
+lr=5e-5
+dropout=0.1
+psl=20
+epoch=20
+
+python3 run.py \
+ --model_name_or_path /wjn/pre-trained-lm/roberta-large \
+ --task_name $TASK_NAME \
+ --dataset_name $DATASET_NAME \
+ --overwrite_cache \
+ --do_train \
+ --do_eval \
+ --do_predict \
+ --max_seq_length 128 \
+ --per_device_train_batch_size $bs \
+ --learning_rate $lr \
+ --num_train_epochs $epoch \
+ --pre_seq_len $psl \
+ --output_dir checkpoints/$DATASET_NAME-roberta/ \
+ --overwrite_output_dir \
+ --hidden_dropout_prob $dropout \
+ --seed 42 \
+ --save_strategy no \
+ --evaluation_strategy epoch \
+ --num_examples_per_label 16 \
+ --prompt_ptuning \
+ # --use_pe
+
+ # --head_prefix \
+ # --use_pe
+ # --adapter \
+ # --adapter_dim 256 \
+ # --adapter_choice list \
+
diff --git a/run_script_fewshot/run_qqp_roberta.sh b/run_script_fewshot/run_qqp_roberta.sh
new file mode 100644
index 0000000..320291b
--- /dev/null
+++ b/run_script_fewshot/run_qqp_roberta.sh
@@ -0,0 +1,39 @@
+export TASK_NAME=glue
+export DATASET_NAME=qqp
+export CUDA_VISIBLE_DEVICES=0
+
+bs=4
+lr=5e-5
+dropout=0.1
+psl=20
+epoch=20
+
+python3 run.py \
+ --model_name_or_path /wjn/pre-trained-lm/roberta-large \
+ --task_name $TASK_NAME \
+ --dataset_name $DATASET_NAME \
+ --overwrite_cache \
+ --do_train \
+ --do_eval \
+ --do_predict \
+ --max_seq_length 128 \
+ --per_device_train_batch_size $bs \
+ --learning_rate $lr \
+ --num_train_epochs $epoch \
+ --pre_seq_len $psl \
+ --output_dir checkpoints/$DATASET_NAME-roberta/ \
+ --overwrite_output_dir \
+ --hidden_dropout_prob $dropout \
+ --seed 42 \
+ --save_strategy no \
+ --evaluation_strategy epoch \
+ --num_examples_per_label 16 \
+ --prompt_ptuning \
+ # --use_pe
+
+ # --head_prefix \
+ # --use_pe
+ # --adapter \
+ # --adapter_dim 256 \
+ # --adapter_choice list \
+
diff --git a/run_script_fewshot/run_rte_bert.sh b/run_script_fewshot/run_rte_bert.sh
new file mode 100644
index 0000000..a8327fa
--- /dev/null
+++ b/run_script_fewshot/run_rte_bert.sh
@@ -0,0 +1,28 @@
+export TASK_NAME=superglue
+export DATASET_NAME=rte
+export CUDA_VISIBLE_DEVICES=0
+
+bs=32
+lr=1e-2
+dropout=0.1
+psl=20
+epoch=60
+
+python3 run.py \
+ --model_name_or_path bert-large-cased \
+ --task_name $TASK_NAME \
+ --dataset_name $DATASET_NAME \
+ --do_train \
+ --do_eval \
+ --max_seq_length 128 \
+ --per_device_train_batch_size $bs \
+ --learning_rate $lr \
+ --num_train_epochs $epoch \
+ --pre_seq_len $psl \
+ --output_dir checkpoints/$DATASET_NAME-bert/ \
+ --overwrite_output_dir \
+ --hidden_dropout_prob $dropout \
+ --seed 11 \
+ --save_strategy no \
+ --evaluation_strategy epoch \
+ --prefix
diff --git a/run_script_fewshot/run_rte_roberta.sh b/run_script_fewshot/run_rte_roberta.sh
new file mode 100644
index 0000000..b41aa41
--- /dev/null
+++ b/run_script_fewshot/run_rte_roberta.sh
@@ -0,0 +1,41 @@
+export TASK_NAME=superglue
+export DATASET_NAME=rte
+export CUDA_VISIBLE_DEVICES=0
+# export CUDA_VISIBLE_DEVICES=2
+# export CUDA_VISIBLE_DEVICES=5
+
+bs=4
+lr=1e-2
+dropout=0.1
+psl=20
+epoch=100
+
+python3 run.py \
+ --model_name_or_path /wjn/pre-trained-lm/roberta-large \
+ --task_name $TASK_NAME \
+ --dataset_name $DATASET_NAME \
+ --overwrite_cache \
+ --do_train \
+ --do_eval \
+ --do_predict \
+ --max_seq_length 128 \
+ --per_device_train_batch_size $bs \
+ --learning_rate $lr \
+ --num_train_epochs $epoch \
+ --pre_seq_len $psl \
+ --output_dir checkpoints/$DATASET_NAME-roberta/ \
+ --overwrite_output_dir \
+ --hidden_dropout_prob $dropout \
+ --seed 42 \
+ --save_strategy no \
+ --evaluation_strategy epoch \
+ --num_examples_per_label 16 \
+ --prompt_ptuning \
+ --use_pe
+
+ # --head_prefix \
+ # --use_pe
+ # --adapter \
+ # --adapter_dim 256 \
+ # --adapter_choice list \
+
diff --git a/run_script_fewshot/run_sst2_roberta.sh b/run_script_fewshot/run_sst2_roberta.sh
new file mode 100644
index 0000000..eb8ae88
--- /dev/null
+++ b/run_script_fewshot/run_sst2_roberta.sh
@@ -0,0 +1,36 @@
+export TASK_NAME=glue
+export DATASET_NAME=sst2
+export CUDA_VISIBLE_DEVICES=1
+
+bs=4
+lr=5e-5
+dropout=0.1
+psl=16
+epoch=100
+
+python3 run.py \
+ --model_name_or_path /wjn/pre-trained-lm/roberta-large \
+ --task_name $TASK_NAME \
+ --dataset_name $DATASET_NAME \
+ --overwrite_cache \
+ --do_train \
+ --do_eval \
+ --do_predict \
+ --max_seq_length 128 \
+ --per_device_train_batch_size $bs \
+ --learning_rate $lr \
+ --num_train_epochs $epoch \
+ --pre_seq_len $psl \
+ --output_dir checkpoints/$DATASET_NAME-roberta/ \
+ --overwrite_output_dir \
+ --hidden_dropout_prob $dropout \
+ --seed 42 \
+ --save_strategy no \
+ --evaluation_strategy epoch \
+ --num_examples_per_label 16 \
+ --prompt_ptuning \
+ # --use_pe
+ # --adapter \
+ # --adapter_dim 256 \
+ # --adapter_choice list \
+
diff --git a/run_script_fewshot/run_trec_roberta.sh b/run_script_fewshot/run_trec_roberta.sh
new file mode 100644
index 0000000..bf12d2b
--- /dev/null
+++ b/run_script_fewshot/run_trec_roberta.sh
@@ -0,0 +1,36 @@
+export TASK_NAME=other_cls
+export DATASET_NAME=trec
+export CUDA_VISIBLE_DEVICES=0
+
+bs=4
+lr=5e-5
+dropout=0.1
+psl=16
+epoch=100
+
+python3 run.py \
+ --model_name_or_path /wjn/pre-trained-lm/roberta-large \
+ --task_name $TASK_NAME \
+ --dataset_name $DATASET_NAME \
+ --overwrite_cache \
+ --do_train \
+ --do_eval \
+ --do_predict \
+ --max_seq_length 128 \
+ --per_device_train_batch_size $bs \
+ --learning_rate $lr \
+ --num_train_epochs $epoch \
+ --pre_seq_len $psl \
+ --output_dir checkpoints/$DATASET_NAME-roberta/ \
+ --overwrite_output_dir \
+ --hidden_dropout_prob $dropout \
+ --seed 42 \
+ --save_strategy no \
+ --evaluation_strategy epoch \
+ --num_examples_per_label 16 \
+ --prompt_ptuning \
+ # --use_pe
+ # --adapter \
+ # --adapter_dim 256 \
+ # --adapter_choice list \
+
diff --git a/run_script_fewshot/run_yelp_roberta.sh b/run_script_fewshot/run_yelp_roberta.sh
new file mode 100644
index 0000000..ff62908
--- /dev/null
+++ b/run_script_fewshot/run_yelp_roberta.sh
@@ -0,0 +1,36 @@
+export TASK_NAME=other_cls
+export DATASET_NAME=yelp_polarity
+export CUDA_VISIBLE_DEVICES=0
+
+bs=4
+lr=5e-5
+dropout=0.1
+psl=16
+epoch=100
+
+python3 run.py \
+ --model_name_or_path /wjn/pre-trained-lm/roberta-large \
+ --task_name $TASK_NAME \
+ --dataset_name $DATASET_NAME \
+ --overwrite_cache \
+ --do_train \
+ --do_eval \
+ --do_predict \
+ --max_seq_length 128 \
+ --per_device_train_batch_size $bs \
+ --learning_rate $lr \
+ --num_train_epochs $epoch \
+ --pre_seq_len $psl \
+ --output_dir checkpoints/$DATASET_NAME-roberta/ \
+ --overwrite_output_dir \
+ --hidden_dropout_prob $dropout \
+ --seed 42 \
+ --save_strategy no \
+ --evaluation_strategy epoch \
+ --num_examples_per_label 16 \
+ --prompt_ptuning \
+ # --use_pe
+ # --adapter \
+ # --adapter_dim 256 \
+ # --adapter_choice list \
+
diff --git a/run_script_semi/run_boolq_roberta.sh b/run_script_semi/run_boolq_roberta.sh
new file mode 100644
index 0000000..660718a
--- /dev/null
+++ b/run_script_semi/run_boolq_roberta.sh
@@ -0,0 +1,58 @@
+export TASK_NAME=superglue
+export DATASET_NAME=boolq
+export CUDA_VISIBLE_DEVICES=6
+# export CUDA_VISIBLE_DEVICES=2
+# export CUDA_VISIBLE_DEVICES=5
+
+bs=4
+lr=5e-3
+student_lr=5e-4
+dropout=0.1
+psl=32
+student_psl=32
+tea_train_epoch=100
+tea_tune_epoch=20
+stu_train_epoch=100
+self_train_epoch=15
+
+pe_type=prompt_prefix
+
+rm -rf checkpoints/self-training/$DATASET_NAME-roberta/$pe_type/iteration
+
+python3 run.py \
+ --model_name_or_path /wjn/pre-trained-lm/roberta-large \
+ --resume_from_checkpoint checkpoints/self-training/$DATASET_NAME-roberta/$pe_type/checkpoint-60 \
+ --task_name $TASK_NAME \
+ --dataset_name $DATASET_NAME \
+ --overwrite_cache \
+ --do_train \
+ --do_eval \
+ --max_seq_length 128 \
+ --per_device_train_batch_size $bs \
+ --learning_rate $lr \
+ --num_train_epochs $tea_train_epoch \
+ --pre_seq_len $psl \
+ --output_dir checkpoints/self-training/$DATASET_NAME-roberta/$pe_type \
+ --overwrite_output_dir \
+ --hidden_dropout_prob $dropout \
+ --seed 42 \
+ --save_strategy epoch \
+ --evaluation_strategy epoch \
+ --save_total_limit=4 \
+ --load_best_model_at_end \
+ --metric_for_best_model accuracy \
+ --report_to none \
+ --num_examples_per_label 16 \
+ --$pe_type \
+ --use_semi \
+ --unlabeled_data_num 4096 \
+ --unlabeled_data_batch_size 16 \
+ --teacher_training_epoch $tea_train_epoch \
+ --teacher_tuning_epoch $tea_tune_epoch \
+ --student_training_epoch $stu_train_epoch \
+ --self_training_epoch $self_train_epoch \
+ --pseudo_sample_num_or_ratio 1024 \
+ --student_pre_seq_len $student_psl \
+ --post_student_train
+
+
\ No newline at end of file
diff --git a/run_script_semi/run_cb_roberta.sh b/run_script_semi/run_cb_roberta.sh
new file mode 100644
index 0000000..77ce531
--- /dev/null
+++ b/run_script_semi/run_cb_roberta.sh
@@ -0,0 +1,58 @@
+export TASK_NAME=superglue
+export DATASET_NAME=cb
+export CUDA_VISIBLE_DEVICES=7
+# export CUDA_VISIBLE_DEVICES=2
+# export CUDA_VISIBLE_DEVICES=5
+
+bs=4
+lr=1e-5
+student_lr=5e-5
+dropout=0.1
+psl=128
+student_psl=128
+tea_train_epoch=100
+tea_tune_epoch=20
+stu_train_epoch=100
+self_train_epoch=15
+
+pe_type=prompt_prefix
+
+rm -rf checkpoints/self-training/$DATASET_NAME-roberta/$pe_type/iteration
+
+python3 run.py \
+ --model_name_or_path /wjn/pre-trained-lm/roberta-large \
+ --resume_from_checkpoint checkpoints/self-training/$DATASET_NAME-roberta/$pe_type/checkpoint-60 \
+ --task_name $TASK_NAME \
+ --dataset_name $DATASET_NAME \
+ --overwrite_cache \
+ --do_train \
+ --do_eval \
+ --max_seq_length 128 \
+ --per_device_train_batch_size $bs \
+ --learning_rate $lr \
+ --num_train_epochs $tea_train_epoch \
+ --pre_seq_len $psl \
+ --output_dir checkpoints/self-training/$DATASET_NAME-roberta/$pe_type \
+ --overwrite_output_dir \
+ --hidden_dropout_prob $dropout \
+ --seed 42 \
+ --save_strategy epoch \
+ --evaluation_strategy epoch \
+ --save_total_limit=4 \
+ --load_best_model_at_end \
+ --metric_for_best_model accuracy \
+ --report_to none \
+ --num_examples_per_label 16 \
+ --$pe_type \
+ --use_semi \
+ --unlabeled_data_num 4096 \
+ --unlabeled_data_batch_size 16 \
+ --teacher_training_epoch $tea_train_epoch \
+ --teacher_tuning_epoch $tea_tune_epoch \
+ --student_training_epoch $stu_train_epoch \
+ --self_training_epoch $self_train_epoch \
+ --pseudo_sample_num_or_ratio 1024 \
+ --student_pre_seq_len $student_psl \
+ --post_student_train
+
+
\ No newline at end of file
diff --git a/run_script_semi/run_mnli_roberta.sh b/run_script_semi/run_mnli_roberta.sh
new file mode 100644
index 0000000..5f8d0fe
--- /dev/null
+++ b/run_script_semi/run_mnli_roberta.sh
@@ -0,0 +1,58 @@
+export TASK_NAME=glue
+export DATASET_NAME=mnli
+export CUDA_VISIBLE_DEVICES=7
+# export CUDA_VISIBLE_DEVICES=2
+# export CUDA_VISIBLE_DEVICES=5
+
+bs=4
+lr=3e-6
+student_lr=3e-6
+dropout=0.1
+psl=128
+student_psl=128
+tea_train_epoch=100
+tea_tune_epoch=20
+stu_train_epoch=100
+self_train_epoch=15
+
+pe_type=prompt_adapter
+
+rm -rf checkpoints/self-training/$DATASET_NAME-roberta/$pe_type/iteration
+
+python3 run.py \
+ --model_name_or_path /wjn/pre-trained-lm/roberta-large \
+ --resume_from_checkpoint checkpoints/self-training/$DATASET_NAME-roberta/$pe_type/checkpoint-60 \
+ --task_name $TASK_NAME \
+ --dataset_name $DATASET_NAME \
+ --overwrite_cache \
+ --do_train \
+ --do_eval \
+ --max_seq_length 128 \
+ --per_device_train_batch_size $bs \
+ --learning_rate $lr \
+ --num_train_epochs $tea_train_epoch \
+ --pre_seq_len $psl \
+ --output_dir checkpoints/self-training/$DATASET_NAME-roberta/$pe_type \
+ --overwrite_output_dir \
+ --hidden_dropout_prob $dropout \
+ --seed 42 \
+ --save_strategy epoch \
+ --evaluation_strategy epoch \
+ --save_total_limit=4 \
+ --load_best_model_at_end \
+ --metric_for_best_model accuracy \
+ --report_to none \
+ --num_examples_per_label 16 \
+ --$pe_type \
+ --use_semi \
+ --unlabeled_data_num 4096 \
+ --unlabeled_data_batch_size 16 \
+ --teacher_training_epoch $tea_train_epoch \
+ --teacher_tuning_epoch $tea_tune_epoch \
+ --student_training_epoch $stu_train_epoch \
+ --self_training_epoch $self_train_epoch \
+ --pseudo_sample_num_or_ratio 1024 \
+ --student_pre_seq_len $student_psl \
+ --post_student_train
+
+
\ No newline at end of file
diff --git a/run_script_semi/run_mrpc_roberta.sh b/run_script_semi/run_mrpc_roberta.sh
new file mode 100644
index 0000000..0cf98ed
--- /dev/null
+++ b/run_script_semi/run_mrpc_roberta.sh
@@ -0,0 +1,58 @@
+export TASK_NAME=glue
+export DATASET_NAME=mrpc
+export CUDA_VISIBLE_DEVICES=5
+# export CUDA_VISIBLE_DEVICES=2
+# export CUDA_VISIBLE_DEVICES=5
+
+bs=4
+lr=1e-5
+student_lr=5e-5
+dropout=0.1
+psl=128
+student_psl=128
+tea_train_epoch=20
+tea_tune_epoch=20
+stu_train_epoch=100
+self_train_epoch=15
+
+pe_type=prompt_adapter
+
+rm -rf checkpoints/self-training/$DATASET_NAME-roberta/$pe_type/iteration
+
+python3 run.py \
+ --model_name_or_path /wjn/pre-trained-lm/roberta-large \
+ --resume_from_checkpoint checkpoints/self-training/$DATASET_NAME-roberta/$pe_type/checkpoint-60 \
+ --task_name $TASK_NAME \
+ --dataset_name $DATASET_NAME \
+ --overwrite_cache \
+ --do_train \
+ --do_eval \
+ --max_seq_length 128 \
+ --per_device_train_batch_size $bs \
+ --learning_rate $lr \
+ --num_train_epochs $tea_train_epoch \
+ --pre_seq_len $psl \
+ --output_dir checkpoints/self-training/$DATASET_NAME-roberta/$pe_type \
+ --overwrite_output_dir \
+ --hidden_dropout_prob $dropout \
+ --seed 42 \
+ --save_strategy epoch \
+ --evaluation_strategy epoch \
+ --save_total_limit=4 \
+ --load_best_model_at_end \
+ --metric_for_best_model f1 \
+ --report_to none \
+ --num_examples_per_label 16 \
+ --$pe_type \
+ --use_semi \
+ --unlabeled_data_num 4096 \
+ --unlabeled_data_batch_size 16 \
+ --teacher_training_epoch $tea_train_epoch \
+ --teacher_tuning_epoch $tea_tune_epoch \
+ --student_training_epoch $stu_train_epoch \
+ --student_learning_rate $student_lr \
+ --self_training_epoch $self_train_epoch \
+ --pseudo_sample_num_or_ratio 1024 \
+ --student_pre_seq_len $student_psl \
+ --post_student_train
+
\ No newline at end of file
diff --git a/run_script_semi/run_qnli_roberta.sh b/run_script_semi/run_qnli_roberta.sh
new file mode 100644
index 0000000..1a02459
--- /dev/null
+++ b/run_script_semi/run_qnli_roberta.sh
@@ -0,0 +1,57 @@
+export TASK_NAME=glue
+export DATASET_NAME=qnli
+export CUDA_VISIBLE_DEVICES=4
+# export CUDA_VISIBLE_DEVICES=2
+# export CUDA_VISIBLE_DEVICES=5
+
+bs=4
+lr=1e-5
+student_lr=5e-3
+dropout=0.1
+psl=16
+student_psl=15
+tea_train_epoch=100
+tea_tune_epoch=20
+stu_train_epoch=100
+self_train_epoch=15
+
+pe_type=head_only
+
+rm -rf checkpoints/self-training/$DATASET_NAME-roberta/$pe_type/iteration
+
+python3 run.py \
+ --model_name_or_path /wjn/pre-trained-lm/roberta-large \
+ --resume_from_checkpoint checkpoints/self-training/$DATASET_NAME-roberta/$pe_type/checkpoint-60 \
+ --task_name $TASK_NAME \
+ --dataset_name $DATASET_NAME \
+ --overwrite_cache \
+ --do_train \
+ --do_eval \
+ --max_seq_length 128 \
+ --per_device_train_batch_size $bs \
+ --learning_rate $lr \
+ --num_train_epochs $tea_train_epoch \
+ --pre_seq_len $psl \
+ --output_dir checkpoints/self-training/$DATASET_NAME-roberta/$pe_type \
+ --overwrite_output_dir \
+ --hidden_dropout_prob $dropout \
+ --seed 42 \
+ --save_strategy epoch \
+ --evaluation_strategy epoch \
+ --save_total_limit=4 \
+ --load_best_model_at_end \
+ --metric_for_best_model accuracy \
+ --report_to none \
+ --num_examples_per_label 16 \
+ --$pe_type \
+ --use_semi \
+ --unlabeled_data_num 4096 \
+ --unlabeled_data_batch_size 16 \
+ --teacher_training_epoch $tea_train_epoch \
+ --teacher_tuning_epoch $tea_tune_epoch \
+ --student_training_epoch $stu_train_epoch \
+ --self_training_epoch $self_train_epoch \
+ --pseudo_sample_num_or_ratio 1024 \
+ --student_pre_seq_len $student_psl \
+ --post_student_train
+
\ No newline at end of file
diff --git a/run_script_semi/run_qqp_roberta.sh b/run_script_semi/run_qqp_roberta.sh
new file mode 100644
index 0000000..32d0b9c
--- /dev/null
+++ b/run_script_semi/run_qqp_roberta.sh
@@ -0,0 +1,57 @@
+export TASK_NAME=glue
+export DATASET_NAME=qqp
+export CUDA_VISIBLE_DEVICES=7
+# export CUDA_VISIBLE_DEVICES=2
+# export CUDA_VISIBLE_DEVICES=5
+
+bs=4
+lr=3e-6
+student_lr=3e-6
+dropout=0.1
+psl=4
+student_psl=4
+tea_train_epoch=60
+tea_tune_epoch=20
+stu_train_epoch=100
+self_train_epoch=15
+
+pe_type=prompt_adapter
+
+rm -rf checkpoints/self-training/$DATASET_NAME-roberta/$pe_type/iteration
+
+python3 run.py \
+ --model_name_or_path /wjn/pre-trained-lm/roberta-large \
+ --resume_from_checkpoint checkpoints/self-training/$DATASET_NAME-roberta/$pe_type/checkpoint-60 \
+ --task_name $TASK_NAME \
+ --dataset_name $DATASET_NAME \
+ --overwrite_cache \
+ --do_train \
+ --do_eval \
+ --max_seq_length 128 \
+ --per_device_train_batch_size $bs \
+ --learning_rate $lr \
+ --num_train_epochs $tea_train_epoch \
+ --pre_seq_len $psl \
+ --output_dir checkpoints/self-training/$DATASET_NAME-roberta/$pe_type \
+ --overwrite_output_dir \
+ --hidden_dropout_prob $dropout \
+ --seed 42 \
+ --save_strategy epoch \
+ --evaluation_strategy epoch \
+ --save_total_limit=4 \
+ --load_best_model_at_end \
+ --metric_for_best_model accuracy \
+ --report_to none \
+ --num_examples_per_label 16 \
+ --$pe_type \
+ --use_semi \
+ --unlabeled_data_num 4096 \
+ --unlabeled_data_batch_size 16 \
+ --teacher_training_epoch $tea_train_epoch \
+ --teacher_tuning_epoch $tea_tune_epoch \
+ --student_training_epoch $stu_train_epoch \
+ --self_training_epoch $self_train_epoch \
+ --pseudo_sample_num_or_ratio 1024 \
+ --student_pre_seq_len $student_psl \
+ --post_student_train
+
\ No newline at end of file
diff --git a/run_script_semi/run_rte_roberta.sh b/run_script_semi/run_rte_roberta.sh
new file mode 100644
index 0000000..4804019
--- /dev/null
+++ b/run_script_semi/run_rte_roberta.sh
@@ -0,0 +1,57 @@
+export TASK_NAME=glue
+export DATASET_NAME=rte
+export CUDA_VISIBLE_DEVICES=2
+# export CUDA_VISIBLE_DEVICES=2
+# export CUDA_VISIBLE_DEVICES=5
+
+bs=4
+lr=1e-5
+student_lr=5e-4
+dropout=0.1
+psl=4
+student_psl=8
+tea_train_epoch=20
+tea_tune_epoch=20
+stu_train_epoch=100
+self_train_epoch=15
+
+pe_type=prompt_prefix
+
+rm -rf checkpoints/self-training/$DATASET_NAME-roberta/$pe_type/iteration
+
+python3 run.py \
+ --model_name_or_path /wjn/pre-trained-lm/roberta-large \
+ --resume_from_checkpoint checkpoints/self-training/$DATASET_NAME-roberta/$pe_type/checkpoint-60 \
+ --task_name $TASK_NAME \
+ --dataset_name $DATASET_NAME \
+ --overwrite_cache \
+ --do_train \
+ --do_eval \
+ --max_seq_length 128 \
+ --per_device_train_batch_size $bs \
+ --learning_rate $lr \
+ --num_train_epochs $tea_train_epoch \
+ --pre_seq_len $psl \
+ --output_dir checkpoints/self-training/$DATASET_NAME-roberta/$pe_type \
+ --overwrite_output_dir \
+ --hidden_dropout_prob $dropout \
+ --seed 42 \
+ --save_strategy epoch \
+ --evaluation_strategy epoch \
+ --save_total_limit=4 \
+ --load_best_model_at_end \
+ --metric_for_best_model accuracy \
+ --report_to none \
+ --num_examples_per_label 16 \
+ --$pe_type \
+ --use_semi \
+ --unlabeled_data_num -1 \
+ --unlabeled_data_batch_size 16 \
+ --teacher_training_epoch $tea_train_epoch \
+ --teacher_tuning_epoch $tea_tune_epoch \
+ --student_training_epoch $stu_train_epoch \
+ --self_training_epoch $self_train_epoch \
+ --pseudo_sample_num_or_ratio 1024 \
+ --student_pre_seq_len $student_psl \
+ --post_student_train
+
\ No newline at end of file
diff --git a/run_script_semi/run_sst2_roberta.sh b/run_script_semi/run_sst2_roberta.sh
new file mode 100644
index 0000000..01589a1
--- /dev/null
+++ b/run_script_semi/run_sst2_roberta.sh
@@ -0,0 +1,57 @@
+export TASK_NAME=glue
+export DATASET_NAME=sst2
+export CUDA_VISIBLE_DEVICES=3
+# export CUDA_VISIBLE_DEVICES=2
+# export CUDA_VISIBLE_DEVICES=5
+
+bs=4
+lr=3e-6
+student_lr=5e-5
+dropout=0.1
+psl=128
+student_psl=4
+tea_train_epoch=100
+tea_tune_epoch=20
+stu_train_epoch=100
+self_train_epoch=15
+
+pe_type=prompt_adapter
+
+rm -rf checkpoints/self-training/$DATASET_NAME-roberta/$pe_type/iteration
+
+python3 run.py \
+ --model_name_or_path /wjn/pre-trained-lm/roberta-large \
+ --resume_from_checkpoint checkpoints/self-training/$DATASET_NAME-roberta/$pe_type/checkpoint-60 \
+ --task_name $TASK_NAME \
+ --dataset_name $DATASET_NAME \
+ --overwrite_cache \
+ --do_train \
+ --do_eval \
+ --max_seq_length 128 \
+ --per_device_train_batch_size $bs \
+ --learning_rate $lr \
+ --num_train_epochs $tea_train_epoch \
+ --pre_seq_len $psl \
+ --output_dir checkpoints/self-training/$DATASET_NAME-roberta/$pe_type \
+ --overwrite_output_dir \
+ --hidden_dropout_prob $dropout \
+ --seed 42 \
+ --save_strategy epoch \
+ --evaluation_strategy epoch \
+ --save_total_limit=4 \
+ --load_best_model_at_end \
+ --metric_for_best_model accuracy \
+ --report_to none \
+ --num_examples_per_label 16 \
+ --$pe_type \
+ --use_semi \
+ --unlabeled_data_num 4096 \
+ --unlabeled_data_batch_size 16 \
+ --teacher_training_epoch $tea_train_epoch \
+ --teacher_tuning_epoch $tea_tune_epoch \
+ --student_training_epoch $stu_train_epoch \
+ --self_training_epoch $self_train_epoch \
+ --pseudo_sample_num_or_ratio 1024 \
+ --student_pre_seq_len $student_psl \
+ --post_student_train
+
\ No newline at end of file
diff --git a/search.py b/search.py
new file mode 100644
index 0000000..f00d2e2
--- /dev/null
+++ b/search.py
@@ -0,0 +1,35 @@
+import json
+import os
+import sys
+
+from glob import glob
+
+from tasks.utils import *
+
+TASK = sys.argv[1]
+MODEL = sys.argv[2]
+PARADIGM = sys.argv[3]
+ISPE = sys.argv[4]
+
+if len(sys.argv) == 6:
+ METRIC = sys.argv[5]
+elif TASK in GLUE_DATASETS + SUPERGLUE_DATASETS + OTHER_DATASETS:
+ METRIC = "accuracy"
+elif TASK in NER_DATASETS + SRL_DATASETS + QA_DATASETS:
+ METRIC = "f1"
+
+best_score = 0
+
+files = glob(f"./checkpoints/{TASK}-{MODEL}-search/{PARADIGM}/{ISPE}/*/best_results.json")
+
+for f in files:
+ metrics = json.load(open(f, 'r'))
+ if metrics["best_eval_"+METRIC] > best_score:
+ best_score = metrics["best_eval_"+METRIC]
+ best_metrics = metrics
+ best_file_name = f
+
+print(f"best_{METRIC}: {best_score}")
+print(f"best_metrics: {best_metrics}")
+print(f"best_file: {best_file_name}")
+
diff --git a/search_script/search_copa_roberta.sh b/search_script/search_copa_roberta.sh
new file mode 100644
index 0000000..761cba7
--- /dev/null
+++ b/search_script/search_copa_roberta.sh
@@ -0,0 +1,34 @@
+export TASK_NAME=superglue
+export DATASET_NAME=copa
+export CUDA_VISIBLE_DEVICES=8
+
+
+for lr in 5e-3 7e-3 1e-2
+do
+ for psl in 4 8 16 32 64 128
+ do
+ for epoch in 20 40 60 80 100 120
+ do
+ python3 run.py \
+ --model_name_or_path roberta-large \
+ --task_name $TASK_NAME \
+ --dataset_name $DATASET_NAME \
+ --do_train \
+ --do_eval \
+ --max_seq_length 128 \
+ --per_device_train_batch_size 16 \
+ --learning_rate $lr \
+ --num_train_epochs $epoch \
+ --pre_seq_len $psl \
+ --output_dir checkpoints/$DATASET_NAME-roberta-search/$DATASET_NAME-$epoch-$lr-$psl/ \
+ --overwrite_output_dir \
+ --hidden_dropout_prob 0.1 \
+ --seed 11 \
+ --save_strategy no \
+ --evaluation_strategy epoch \
+ --prefix
+ done
+ done
+done
+
+python3 search.py $DATASET_NAME roberta
\ No newline at end of file
diff --git a/search_script/search_roberta.sh b/search_script/search_roberta.sh
new file mode 100644
index 0000000..e9c2655
--- /dev/null
+++ b/search_script/search_roberta.sh
@@ -0,0 +1,92 @@
+export TASK_NAME=other_cls # glue / superglue / other_cls
+export DATASET_NAME=yelp_polarity
+export CUDA_VISIBLE_DEVICES=3
+
+bs=4
+dropout=0.1
+shot=16 # shot=-1 means full data
+seq_len=128
+
+paradigm=prompt_adapter # parameter-efficient paradigm
+is_pe=use_pe
+is_pe=no_pe
+
+# prompt_only / head_only w. use_pe (lr: 5e-4 5e-3 7e-3 1e-2)
+# prompt_only / head_only w/o. use_pe (lr: 5e-6 1e-5 5e-4 5e-3)
+# prompt_prefix / head_prefix w. use_pe (lr: 5e-4 5e-3 7e-3 1e-2)
+# prompt_prefix / head_prefix w/o. use_pe (lr: 5e-6 1e-5 5e-4 5e-3)
+# prompt_ptuning / head_ptuning w. use_pe (lr: 5e-4 5e-3 7e-3 1e-2)
+# prompt_ptuning / head_ptuning w/o. use_pe (lr: 5e-6 1e-5 5e-4 5e-3)
+# prompt_adapter / head_adapter w. use_pe (lr: 3e-6 5e-6 1e-5 5e-5)
+# prompt_adapter / head_adapter w/o. use_pe (lr: 3e-6 5e-6 1e-5 5e-5)
+
+# if choose use_pe: lr can be set among 5e-4 5e-3 7e-3 1e-2
+# if not choose use_ps, lr can be set among 5e-6 1e-5 5e-5 5e-4
+
+if [ "$paradigm" = "head_only" ] || [ "$paradigm" = "prompt_only" ]; then
+ if [ "$is_pe" = "use_pe" ]; then
+ lr_list=(5e-4 5e-3 7e-3 1e-2)
+ elif [ "$is_pe" = "no_pe" ]; then
+ lr_list=(5e-6 1e-5 5e-4 5e-3)
+ fi
+
+elif [ "$paradigm" = "head_prefix" ] || [ "$paradigm" = "prompt_prefix" ]; then
+ if [ "$is_pe" = "use_pe" ]; then
+ lr_list=(5e-4 5e-3 7e-3 1e-2)
+ elif [ "$is_pe" = "no_pe" ]; then
+ lr_list=(5e-6 1e-5 5e-4 5e-3)
+ fi
+
+elif [ "$paradigm" = "head_ptuning" ] || [ "$paradigm" = "prompt_ptuning" ]; then
+ if [ "$is_pe" = "use_pe" ]; then
+ lr_list=(5e-4 5e-3 7e-3 1e-2)
+ elif [ "$is_pe" = "no_pe" ]; then
+ lr_list=(5e-6 1e-5 5e-4 5e-3)
+ fi
+
+elif [ "$paradigm" = "head_adapter" ] || [ "$paradigm" = "prompt_adapter" ]; then
+ if [ "$is_pe" = "use_pe" ]; then
+ lr_list=(3e-6 5e-6 1e-5 5e-5)
+ elif [ "$is_pe" = "no_pe" ]; then
+ lr_list=(3e-6 5e-6 1e-5 5e-5)
+ fi
+
+fi
+
+if [ "$DATASET_NAME" = "boolq" ]; then
+ seq_len=384
+elif [ "$DATASET_NAME" = "movie_rationales" ]; then
+ seq_len=256
+fi
+
+for lr in "${lr_list[@]}"
+do
+ for psl in 4 8 16 32 64 128
+ do
+ for epoch in 20 60 100
+ do
+ python3 run.py \
+ --model_name_or_path /wjn/pre-trained-lm/roberta-large \
+ --task_name $TASK_NAME \
+ --dataset_name $DATASET_NAME \
+ --overwrite_cache \
+ --do_train \
+ --do_eval \
+ --max_seq_length $seq_len \
+ --per_device_train_batch_size $bs \
+ --learning_rate $lr \
+ --num_train_epochs $epoch \
+ --pre_seq_len $psl \
+ --output_dir checkpoints/$DATASET_NAME-roberta-search/$paradigm/$is_pe/$DATASET_NAME-$epoch-$lr-$psl/ \
+ --overwrite_output_dir \
+ --hidden_dropout_prob $dropout \
+ --seed 42 \
+ --save_strategy no \
+ --evaluation_strategy epoch \
+ --num_examples_per_label $shot \
+ --prompt_adapter \
+ # --use_pe
+ done
+ done
+done
+# python3 search.py $DATASET_NAME roberta $paradigm $is_pe
\ No newline at end of file
diff --git a/tasks/.DS_Store b/tasks/.DS_Store
new file mode 100644
index 0000000..9b346d1
Binary files /dev/null and b/tasks/.DS_Store differ
diff --git a/tasks/glue/dataset.py b/tasks/glue/dataset.py
new file mode 100644
index 0000000..80bc839
--- /dev/null
+++ b/tasks/glue/dataset.py
@@ -0,0 +1,299 @@
+import torch
+from torch.utils import data
+from torch.utils.data import Dataset
+from datasets.arrow_dataset import Dataset as HFDataset
+from datasets.load import load_dataset, load_metric
+from transformers import (
+ AutoTokenizer,
+ DataCollatorWithPadding,
+ EvalPrediction,
+ default_data_collator,
+)
+import numpy as np
+import logging
+from typing import Optional
+
+
+# add by wjn
+def random_sampling(raw_datasets: load_dataset, data_type: str="train", num_examples_per_label: Optional[int]=16):
+ assert data_type in ["train", "dev", "test"]
+ label_list = raw_datasets[data_type]["label"] # [0, 1, 0, 0, ...]
+ label_dict = dict()
+ # 记录每个label对应的样本索引
+ for ei, label in enumerate(label_list):
+ if label not in label_dict.keys():
+ label_dict[label] = list()
+ label_dict[label].append(ei)
+ # 对于每个类别,随机采样k个样本
+ few_example_ids = list()
+ for label, eid_list in label_dict.items():
+ # examples = deepcopy(eid_list)
+ # shuffle(examples)
+ idxs = np.random.choice(len(eid_list), size=num_examples_per_label, replace=False)
+ selected_eids = [eid_list[i] for i in idxs]
+ few_example_ids.extend(selected_eids)
+ # 保存没有被选中的example id
+ num_examples = len(label_list)
+ un_selected_examples_ids = [idx for idx in range(num_examples) if idx not in few_example_ids]
+ return few_example_ids, un_selected_examples_ids
+
+task_to_test_key = {
+ "cola": "matt",
+ "mnli": "accuracy",
+ "mrpc": "f1",
+ "qnli": "accuracy",
+ "qqp": "f1",
+ "rte": "accuracy",
+ "sst2": "accuracy",
+ "stsb": "accuracy",
+ "wnli": "accuracy",
+}
+
+task_to_keys = {
+ "cola": ("sentence", None),
+ "mnli": ("premise", "hypothesis"),
+ "mrpc": ("sentence1", "sentence2"),
+ "qnli": ("question", "sentence"),
+ "qqp": ("question1", "question2"),
+ "rte": ("sentence1", "sentence2"),
+ "sst2": ("sentence", None),
+ "stsb": ("sentence1", "sentence2"),
+ "wnli": ("sentence1", "sentence2"),
+}
+
+task_to_template = {
+ "cola": [{"prefix_template": "", "suffix_template": "This is ."}, None],
+ "mnli": [None, {"prefix_template": " ? , ", "suffix_template": ""}],
+ "mrpc": [None, {"prefix_template": " . , ", "suffix_template": ""}],
+ "qnli": [None, {"prefix_template": "? ,", "suffix_template": ""}],
+ "qqp": [None, {"prefix_template": " ,", "suffix_template": ""}],
+ "rte": [None, {"prefix_template": " ? , ", "suffix_template": ""}], # prefix / suffix template in each segment.
+ "sst2": [{"prefix_template": "", "suffix_template": "It was ."}, None], # prefix / suffix template in each segment.
+
+}
+
+# add by wjn
+label_words_mapping = {
+ "cola": {"unacceptable": ["incorrect"], "acceptable": ["correct"]},
+ "mnli": {"contradiction": ["No"], "entailment": "Yes", "neutral": ["Maybe"]},
+ "mrpc": {"not_equivalent": ["No"], "equivalent": ["Yes"]},
+ "qnli": {"not_entailment" : ["No"], "entailment": ["Yes"]},
+ "qqp": {"not_duplicate": "No", "duplicate": "Yes"},
+ "rte": {"not_entailment": ["No"], "entailment": ["Yes"]},
+ "sst2": {"negative": ["terrible"], "positive": ["great"]}, # e.g., {"0": ["great"], "1": [bad]}
+}
+
+
+logger = logging.getLogger(__name__)
+
+
+class GlueDataset():
+ def __init__(
+ self,
+ tokenizer: AutoTokenizer,
+ data_args,
+ training_args,
+ semi_training_args=None,
+ use_prompt=None
+ ) -> None:
+ super().__init__()
+ raw_datasets = load_dataset("glue", data_args.dataset_name)
+ self.tokenizer = tokenizer
+ self.data_args = data_args
+ #labels
+ self.is_regression = data_args.dataset_name == "stsb"
+ if not self.is_regression:
+ self.label_list = raw_datasets["train"].features["label"].names
+ self.num_labels = len(self.label_list)
+ else:
+ self.num_labels = 1
+
+
+ # === generate template ===== add by wjn
+ self.use_prompt = False
+ if data_args.dataset_name in task_to_template.keys():
+ self.use_prompt = use_prompt
+
+ if self.use_prompt:
+ if 't5' in type(tokenizer).__name__.lower():
+ self.special_token_mapping = {
+ 'cls': 3, 'mask': 32099, 'sep': tokenizer.eos_token_id,
+ 'sep+': tokenizer.eos_token_id,
+ 'pseudo_token': tokenizer.unk_token_id
+ }
+ else:
+ self.special_token_mapping = {
+ 'cls': tokenizer.cls_token_id, 'mask': tokenizer.mask_token_id, 'sep': tokenizer.sep_token_id,
+ 'sep+': tokenizer.sep_token_id,
+ 'pseudo_token': tokenizer.unk_token_id
+ }
+ self.template = task_to_template[data_args.dataset_name] # dict
+
+ # === generate label word mapping ===== add by wjn
+ if self.use_prompt:
+ assert data_args.dataset_name in label_words_mapping.keys(), "You must define label word mapping for the task {}".format(data_args.dataset_name)
+ self.label_to_word = label_words_mapping[data_args.dataset_name] # e.g., {"0": ["great"], "1": [bad]}
+ self.label_to_word = {label: label_word[0] if type(label_word) == list else label_word for label, label_word in self.label_to_word.items()}
+
+ for key in self.label_to_word:
+ # For RoBERTa/BART/T5, tokenization also considers space, so we use space+word as label words.
+ if self.label_to_word[key][0] not in ['<', '[', '.', ',']:
+ # Make sure space+word is in the vocabulary
+ assert len(tokenizer.tokenize(' ' + self.label_to_word[key])) == 1
+ self.label_to_word[key] = tokenizer.convert_tokens_to_ids(tokenizer.tokenize(' ' + self.label_to_word[key])[0])
+ else:
+ self.label_to_word[key] = tokenizer.convert_tokens_to_ids(self.label_to_word[key])
+ logger.info("Label {} to word {} ({})".format(key, tokenizer._convert_id_to_token(self.label_to_word[key]), self.label_to_word[key]))
+
+ if len(self.label_list) > 1:
+ self.label_word_list = [self.label_to_word[label] for label in self.label_list]
+ else:
+ # Regression task
+ # '0' represents low polarity and '1' represents high polarity.
+ self.label_word_list = [self.label_to_word[label] for label in ['0', '1']]
+ # =============
+
+ # Preprocessing the raw_datasets
+ self.sentence1_key, self.sentence2_key = task_to_keys[data_args.dataset_name]
+
+ # Padding strategy
+ if data_args.pad_to_max_length:
+ self.padding = "max_length"
+ else:
+ # We will pad later, dynamically at batch creation, to the max sequence length in each batch
+ self.padding = False
+
+ # Some models have set the order of the labels to use, so let's make sure we do use it.
+ if not self.is_regression:
+ self.label2id = {l: i for i, l in enumerate(self.label_list)}
+ self.id2label = {id: label for label, id in self.label2id.items()}
+
+ if data_args.max_seq_length > tokenizer.model_max_length:
+ logger.warning(
+ f"The max_seq_length passed ({data_args.max_seq_length}) is larger than the maximum length for the"
+ f"model ({tokenizer.model_max_length}). Using max_seq_length={tokenizer.model_max_length}."
+ )
+ self.max_seq_length = min(data_args.max_seq_length, tokenizer.model_max_length)
+
+ raw_datasets = raw_datasets.map(
+ self.preprocess_function,
+ batched=True,
+ load_from_cache_file=not data_args.overwrite_cache,
+ desc="Running tokenizer on dataset",
+ )
+
+ if training_args.do_train:
+ self.train_dataset = raw_datasets["train"]
+ if data_args.max_train_samples is not None:
+ self.train_dataset = self.train_dataset.select(range(data_args.max_train_samples))
+
+ if training_args.do_eval:
+ self.eval_dataset = raw_datasets["validation_matched" if data_args.dataset_name == "mnli" else "validation"]
+ if data_args.max_eval_samples is not None:
+ self.eval_dataset = self.eval_dataset.select(range(data_args.max_eval_samples))
+
+ if training_args.do_predict or data_args.dataset_name is not None or data_args.test_file is not None:
+ self.predict_dataset = raw_datasets["test_matched" if data_args.dataset_name == "mnli" else "test"]
+ if data_args.max_predict_samples is not None:
+ self.predict_dataset = self.predict_dataset.select(range(data_args.max_predict_samples))
+
+ # add by wjn
+ self.unlabeled_dataset = None
+ if semi_training_args.use_semi is True:
+ assert data_args.num_examples_per_label is not None and data_args.num_examples_per_label != -1
+
+ # 随机采样few-shot training / dev data(传入label_list,对每个label进行采样,最后得到索引列表)
+ if data_args.num_examples_per_label is not None and data_args.num_examples_per_label != -1:
+ train_examples_idx_list, un_selected_idx_list = random_sampling(
+ raw_datasets=raw_datasets,
+ data_type="train",
+ num_examples_per_label=data_args.num_examples_per_label
+ )
+ self.all_train_dataset = self.train_dataset
+ self.train_dataset = self.all_train_dataset.select(train_examples_idx_list)
+ print("Randomly sampling {}-shot training examples for each label. Total examples number is {}".format(
+ data_args.num_examples_per_label,
+ len(self.train_dataset)
+ ))
+
+ if semi_training_args.use_semi is True:
+ self.unlabeled_dataset = self.all_train_dataset.select(un_selected_idx_list)
+ print("The number of unlabeled data is {}".format(len(self.unlabeled_dataset)))
+
+ self.metric = load_metric("./metrics/glue", data_args.dataset_name)
+
+ if data_args.pad_to_max_length:
+ self.data_collator = default_data_collator
+ elif training_args.fp16:
+ self.data_collator = DataCollatorWithPadding(tokenizer, pad_to_multiple_of=8)
+
+ # ==== define test key ====== add by wjn
+ self.test_key = task_to_test_key[data_args.dataset_name]
+
+
+ def preprocess_function(self, examples):
+ # add by wjn
+ # adding prompt into each example
+ if self.use_prompt:
+ # if use prompt, insert template into example
+ examples = self.prompt_preprocess_function(examples)
+
+ # Tokenize the texts
+ args = (
+ (examples[self.sentence1_key],) if self.sentence2_key is None else (examples[self.sentence1_key], examples[self.sentence2_key])
+ )
+ result = self.tokenizer(*args, padding=self.padding, max_length=self.max_seq_length, truncation=True)
+
+ if self.use_prompt:
+ mask_pos = []
+ for input_ids in result["input_ids"]:
+ mask_pos.append(input_ids.index(self.special_token_mapping["mask"]))
+ result["mask_pos"] = mask_pos
+
+ return result
+
+ # add by wjn
+ # process data for prompt (add template)
+ def prompt_preprocess_function(self, examples):
+
+ def replace_mask_token(template):
+ return template.replace("", self.tokenizer.convert_ids_to_tokens(self.special_token_mapping["mask"]))
+
+ sequence1_prefix_template = replace_mask_token(self.template[0]["prefix_template"] if self.template[0] is not None else "")
+ sequence1_suffix_template = replace_mask_token(self.template[0]["suffix_template"] if self.template[0] is not None else "")
+ sequence2_prefix_template = replace_mask_token(self.template[1]["prefix_template"] if self.template[1] is not None else "")
+ sequence2_suffix_template = replace_mask_token(self.template[1]["suffix_template"] if self.template[1] is not None else "")
+ example_num = len(examples[self.sentence1_key])
+ for example_id in range(example_num):
+ sequence1 = examples[self.sentence1_key][example_id]
+ if self.sentence2_key is None:
+ sequence1 = sequence1[:self.data_args.max_seq_length - len(sequence1_suffix_template) - 10]
+ examples[self.sentence1_key][example_id] = "{}{}{}".format(
+ sequence1_prefix_template, sequence1, sequence1_suffix_template)
+
+ if self.sentence2_key is not None:
+ sequence2 = examples[self.sentence2_key][example_id]
+ sequence2 = sequence2[:self.data_args.max_seq_length - len(sequence1) - len(sequence1_prefix_template) - len(sequence1_suffix_template) - len(sequence2_prefix_template)- 10]
+ examples[self.sentence2_key][example_id] = "{}{}{}".format(
+ sequence2_prefix_template, sequence2, sequence2_suffix_template)
+
+ # examples[self.sentence1_key][example_id] = "{}{}{}".format(
+ # sequence1_prefix_template, examples[self.sentence1_key][example_id], sequence1_suffix_template)
+ # if self.sentence2_key is not None:
+ # examples[self.sentence2_key][example_id] = "{}{}{}".format(
+ # sequence2_prefix_template, examples[self.sentence2_key][example_id], sequence2_suffix_template)
+ return examples
+
+ def compute_metrics(self, p: EvalPrediction):
+ preds = p.predictions[0] if isinstance(p.predictions, tuple) else p.predictions
+ preds = np.squeeze(preds) if self.is_regression else np.argmax(preds, axis=1)
+ if self.data_args.dataset_name is not None:
+ result = self.metric.compute(predictions=preds, references=p.label_ids)
+ if len(result) > 1:
+ result["combined_score"] = np.mean(list(result.values())).item()
+ return result
+ elif self.is_regression:
+ return {"mse": ((preds - p.label_ids) ** 2).mean().item()}
+ else:
+ return {"accuracy": (preds == p.label_ids).astype(np.float32).mean().item()}
+
diff --git a/tasks/glue/get_trainer.py b/tasks/glue/get_trainer.py
new file mode 100644
index 0000000..df4584c
--- /dev/null
+++ b/tasks/glue/get_trainer.py
@@ -0,0 +1,96 @@
+import logging
+import os
+import random
+import sys
+
+from transformers import (
+ AutoConfig,
+ AutoTokenizer,
+)
+
+from model.utils import get_model, TaskType
+from tasks.glue.dataset import GlueDataset
+from training.trainer_base import BaseTrainer
+from training.self_trainer import SelfTrainer
+
+logger = logging.getLogger(__name__)
+
+def get_trainer(args):
+ model_args, data_args, training_args, semi_training_args, _ = args
+
+ tokenizer = AutoTokenizer.from_pretrained(
+ model_args.model_name_or_path,
+ use_fast=model_args.use_fast_tokenizer,
+ revision=model_args.model_revision,
+ )
+
+ # add by wjn check if use prompt template
+ use_prompt = False
+ if model_args.prompt_prefix or model_args.prompt_ptuning or model_args.prompt_adapter or model_args.prompt_only:
+ use_prompt = True
+
+ dataset = GlueDataset(tokenizer, data_args, training_args, semi_training_args=semi_training_args, use_prompt=use_prompt)
+
+ data_args.label_word_list = None # add by wjn
+ if use_prompt:
+ data_args.label_word_list = dataset.label_word_list # add by wjn
+
+ if training_args.do_train:
+ for index in random.sample(range(len(dataset.train_dataset)), 3):
+ logger.info(f"Sample {index} of the training set: {dataset.train_dataset[index]}.")
+
+ if not dataset.is_regression:
+ config = AutoConfig.from_pretrained(
+ model_args.model_name_or_path,
+ num_labels=dataset.num_labels,
+ label2id=dataset.label2id,
+ id2label=dataset.id2label,
+ finetuning_task=data_args.dataset_name,
+ revision=model_args.model_revision,
+ )
+ else:
+ config = AutoConfig.from_pretrained(
+ model_args.model_name_or_path,
+ num_labels=dataset.num_labels,
+ finetuning_task=data_args.dataset_name,
+ revision=model_args.model_revision,
+ )
+
+ model = get_model(data_args, model_args, TaskType.SEQUENCE_CLASSIFICATION, config)
+
+ # Initialize our Trainer
+
+ if semi_training_args.use_semi:
+ model_args.pre_seq_len = semi_training_args.student_pre_seq_len
+ student_model = get_model(data_args, model_args, TaskType.SEQUENCE_CLASSIFICATION, config)
+ trainer = SelfTrainer(
+ teacher_base_model=model,
+ student_base_model=student_model,
+ training_args=training_args,
+ semi_training_args=semi_training_args,
+ train_dataset=dataset.train_dataset if training_args.do_train else None,
+ unlabeled_dataset=dataset.unlabeled_dataset,
+ eval_dataset=dataset.eval_dataset if training_args.do_eval else None,
+ compute_metrics=dataset.compute_metrics,
+ tokenizer=tokenizer,
+ teacher_data_collator=dataset.data_collator,
+ student_data_collator=dataset.data_collator,
+ test_key=dataset.test_key,
+ task_type="cls",
+ num_classes=len(dataset.label2id),
+ )
+
+ return trainer, None
+
+ trainer = BaseTrainer(
+ model=model,
+ args=training_args,
+ train_dataset=dataset.train_dataset if training_args.do_train else None,
+ eval_dataset=dataset.eval_dataset if training_args.do_eval else None,
+ compute_metrics=dataset.compute_metrics,
+ tokenizer=tokenizer,
+ data_collator=dataset.data_collator,
+ test_key=dataset.test_key,
+ )
+
+ return trainer, None
\ No newline at end of file
diff --git a/tasks/ner/dataset.py b/tasks/ner/dataset.py
new file mode 100644
index 0000000..4e50aef
--- /dev/null
+++ b/tasks/ner/dataset.py
@@ -0,0 +1,160 @@
+import torch
+from torch.utils import data
+from torch.utils.data import Dataset
+from datasets.arrow_dataset import Dataset as HFDataset
+from datasets.load import load_dataset, load_metric
+from transformers import AutoTokenizer, DataCollatorForTokenClassification, AutoConfig
+import numpy as np
+
+# add by wjn
+def random_sampling(raw_datasets: load_dataset, data_type: str="train", num_examples_per_label: Optional[int]=16):
+ assert data_type in ["train", "dev", "test"]
+ label_list = raw_datasets[data_type]["label"] # [0, 1, 0, 0, ...]
+ label_dict = dict()
+ # 记录每个label对应的样本索引
+ for ei, label in enumerate(label_list):
+ if label not in label_dict.keys():
+ label_dict[label] = list()
+ label_dict[label].append(ei)
+ # 对于每个类别,随机采样k个样本
+ few_example_ids = list()
+ for label, eid_list in label_dict.items():
+ # examples = deepcopy(eid_list)
+ # shuffle(examples)
+ idxs = np.random.choice(len(eid_list), size=num_examples_per_label, replace=False)
+ selected_eids = [eid_list[i] for i in idxs]
+ few_example_ids.extend(selected_eids)
+ return few_example_ids
+
+
+class NERDataset():
+ def __init__(self, tokenizer: AutoTokenizer, data_args, training_args) -> None:
+ super().__init__()
+ raw_datasets = load_dataset(f'tasks/ner/datasets/{data_args.dataset_name}.py')
+ self.tokenizer = tokenizer
+
+ if training_args.do_train:
+ column_names = raw_datasets["train"].column_names
+ features = raw_datasets["train"].features
+ else:
+ column_names = raw_datasets["validation"].column_names
+ features = raw_datasets["validation"].features
+
+ self.label_column_name = f"{data_args.task_name}_tags"
+ self.label_list = features[self.label_column_name].feature.names
+ self.label_to_id = {l: i for i, l in enumerate(self.label_list)}
+ self.num_labels = len(self.label_list)
+
+ if training_args.do_train:
+ train_dataset = raw_datasets['train']
+ if data_args.max_train_samples is not None:
+ train_dataset = train_dataset.select(range(data_args.max_train_samples))
+ self.train_dataset = train_dataset.map(
+ self.tokenize_and_align_labels,
+ batched=True,
+ load_from_cache_file=True,
+ desc="Running tokenizer on train dataset",
+ )
+ if training_args.do_eval:
+ eval_dataset = raw_datasets['validation']
+ if data_args.max_eval_samples is not None:
+ eval_dataset = eval_dataset.select(range(data_args.max_eval_samples))
+ self.eval_dataset = eval_dataset.map(
+ self.tokenize_and_align_labels,
+ batched=True,
+ load_from_cache_file=True,
+ desc="Running tokenizer on validation dataset",
+ )
+ if training_args.do_predict:
+ predict_dataset = raw_datasets['test']
+ if data_args.max_predict_samples is not None:
+ predict_dataset = predict_dataset.select(range(data_args.max_predict_samples))
+ self.predict_dataset = predict_dataset.map(
+ self.tokenize_and_align_labels,
+ batched=True,
+ load_from_cache_file=True,
+ desc="Running tokenizer on test dataset",
+ )
+
+ # add by wjn
+ # 随机采样few-shot training / dev data(传入label_list,对每个label进行采样,最后得到索引列表)
+ if data_args.num_examples_per_label is not None:
+ train_examples_idx_list = random_sampling(
+ raw_datasets=raw_datasets,
+ data_type="train",
+ num_examples_per_label=data_args.num_examples_per_label
+ )
+ self.train_dataset = self.train_dataset.select(train_examples_idx_list)
+ print("Randomly sampling {}-shot training examples for each label. Total examples number is {}".format(
+ data_args.num_examples_per_label,
+ len(self.train_dataset)
+ ))
+
+ self.data_collator = DataCollatorForTokenClassification(self.tokenizer, pad_to_multiple_of=8 if training_args.fp16 else None)
+
+ self.metric = load_metric('seqeval')
+
+
+ def compute_metrics(self, p):
+ predictions, labels = p
+ predictions = np.argmax(predictions, axis=2)
+
+ # Remove ignored index (special tokens)
+ true_predictions = [
+ [self.label_list[p] for (p, l) in zip(prediction, label) if l != -100]
+ for prediction, label in zip(predictions, labels)
+ ]
+ true_labels = [
+ [self.label_list[l] for (p, l) in zip(prediction, label) if l != -100]
+ for prediction, label in zip(predictions, labels)
+ ]
+
+ results = self.metric.compute(predictions=true_predictions, references=true_labels)
+ return {
+ "precision": results["overall_precision"],
+ "recall": results["overall_recall"],
+ "f1": results["overall_f1"],
+ "accuracy": results["overall_accuracy"],
+ }
+
+ def tokenize_and_align_labels(self, examples):
+ tokenized_inputs = self.tokenizer(
+ examples['tokens'],
+ padding=False,
+ truncation=True,
+ # We use this argument because the texts in our dataset are lists of words (with a label for each word).
+ is_split_into_words=True,
+ )
+
+ labels = []
+ for i, label in enumerate(examples[self.label_column_name]):
+ word_ids = [None]
+ for j, word in enumerate(examples['tokens'][i]):
+ token = self.tokenizer.encode(word, add_special_tokens=False)
+ # print(token)
+ word_ids += [j] * len(token)
+ word_ids += [None]
+
+ # word_ids = tokenized_inputs.word_ids(batch_index=i)
+ previous_word_idx = None
+ label_ids = []
+ for word_idx in word_ids:
+ # Special tokens have a word id that is None. We set the label to -100 so they are automatically
+ # ignored in the loss function.
+ if word_idx is None:
+ label_ids.append(-100)
+ # We set the label for the first token of each word.
+ elif word_idx != previous_word_idx:
+ label_ids.append(label[word_idx])
+ # label_ids.append(self.label_to_id[label[word_idx]])
+ # For the other tokens in a word, we set the label to either the current label or -100, depending on
+ # the label_all_tokens flag.
+ else:
+ label_ids.append(-100)
+ previous_word_idx = word_idx
+
+ labels.append(label_ids)
+ tokenized_inputs["labels"] = labels
+ return tokenized_inputs
+
+
\ No newline at end of file
diff --git a/tasks/ner/datasets/conll2003.py b/tasks/ner/datasets/conll2003.py
new file mode 100644
index 0000000..c5448f8
--- /dev/null
+++ b/tasks/ner/datasets/conll2003.py
@@ -0,0 +1,238 @@
+# coding=utf-8
+# Copyright 2020 HuggingFace Datasets Authors.
+#
+# Licensed under the Apache License, Version 2.0 (the "License");
+# you may not use this file except in compliance with the License.
+# You may obtain a copy of the License at
+#
+# http://www.apache.org/licenses/LICENSE-2.0
+#
+# Unless required by applicable law or agreed to in writing, software
+# distributed under the License is distributed on an "AS IS" BASIS,
+# WITHOUT WARRANTIES OR CONDITIONS OF ANY KIND, either express or implied.
+# See the License for the specific language governing permissions and
+# limitations under the License.
+
+# Lint as: python3
+"""Introduction to the CoNLL-2003 Shared Task: Language-Independent Named Entity Recognition"""
+
+import datasets
+
+
+logger = datasets.logging.get_logger(__name__)
+
+
+_CITATION = """\
+@inproceedings{tjong-kim-sang-de-meulder-2003-introduction,
+ title = "Introduction to the {C}o{NLL}-2003 Shared Task: Language-Independent Named Entity Recognition",
+ author = "Tjong Kim Sang, Erik F. and
+ De Meulder, Fien",
+ booktitle = "Proceedings of the Seventh Conference on Natural Language Learning at {HLT}-{NAACL} 2003",
+ year = "2003",
+ url = "https://www.aclweb.org/anthology/W03-0419",
+ pages = "142--147",
+}
+"""
+
+_DESCRIPTION = """\
+The shared task of CoNLL-2003 concerns language-independent named entity recognition. We will concentrate on
+four types of named entities: persons, locations, organizations and names of miscellaneous entities that do
+not belong to the previous three groups.
+The CoNLL-2003 shared task data files contain four columns separated by a single space. Each word has been put on
+a separate line and there is an empty line after each sentence. The first item on each line is a word, the second
+a part-of-speech (POS) tag, the third a syntactic chunk tag and the fourth the named entity tag. The chunk tags
+and the named entity tags have the format I-TYPE which means that the word is inside a phrase of type TYPE. Only
+if two phrases of the same type immediately follow each other, the first word of the second phrase will have tag
+B-TYPE to show that it starts a new phrase. A word with tag O is not part of a phrase. Note the dataset uses IOB2
+tagging scheme, whereas the original dataset uses IOB1.
+For more details see https://www.clips.uantwerpen.be/conll2003/ner/ and https://www.aclweb.org/anthology/W03-0419
+"""
+
+_URL = "../../../data/CoNLL03/"
+_TRAINING_FILE = "train.txt"
+_DEV_FILE = "valid.txt"
+_TEST_FILE = "test.txt"
+
+
+class Conll2003Config(datasets.BuilderConfig):
+ """BuilderConfig for Conll2003"""
+
+ def __init__(self, **kwargs):
+ """BuilderConfig forConll2003.
+ Args:
+ **kwargs: keyword arguments forwarded to super.
+ """
+ super(Conll2003Config, self).__init__(**kwargs)
+
+
+class Conll2003(datasets.GeneratorBasedBuilder):
+ """Conll2003 dataset."""
+
+ BUILDER_CONFIGS = [
+ Conll2003Config(name="conll2003", version=datasets.Version("1.0.0"), description="Conll2003 dataset"),
+ ]
+
+ def _info(self):
+ return datasets.DatasetInfo(
+ description=_DESCRIPTION,
+ features=datasets.Features(
+ {
+ "id": datasets.Value("string"),
+ "tokens": datasets.Sequence(datasets.Value("string")),
+ "pos_tags": datasets.Sequence(
+ datasets.features.ClassLabel(
+ names=[
+ '"',
+ "''",
+ "#",
+ "$",
+ "(",
+ ")",
+ ",",
+ ".",
+ ":",
+ "``",
+ "CC",
+ "CD",
+ "DT",
+ "EX",
+ "FW",
+ "IN",
+ "JJ",
+ "JJR",
+ "JJS",
+ "LS",
+ "MD",
+ "NN",
+ "NNP",
+ "NNPS",
+ "NNS",
+ "NN|SYM",
+ "PDT",
+ "POS",
+ "PRP",
+ "PRP$",
+ "RB",
+ "RBR",
+ "RBS",
+ "RP",
+ "SYM",
+ "TO",
+ "UH",
+ "VB",
+ "VBD",
+ "VBG",
+ "VBN",
+ "VBP",
+ "VBZ",
+ "WDT",
+ "WP",
+ "WP$",
+ "WRB",
+ ]
+ )
+ ),
+ "chunk_tags": datasets.Sequence(
+ datasets.features.ClassLabel(
+ names=[
+ "O",
+ "B-ADJP",
+ "I-ADJP",
+ "B-ADVP",
+ "I-ADVP",
+ "B-CONJP",
+ "I-CONJP",
+ "B-INTJ",
+ "I-INTJ",
+ "B-LST",
+ "I-LST",
+ "B-NP",
+ "I-NP",
+ "B-PP",
+ "I-PP",
+ "B-PRT",
+ "I-PRT",
+ "B-SBAR",
+ "I-SBAR",
+ "B-UCP",
+ "I-UCP",
+ "B-VP",
+ "I-VP",
+ ]
+ )
+ ),
+ "ner_tags": datasets.Sequence(
+ datasets.features.ClassLabel(
+ names=[
+ "O",
+ "B-PER",
+ "I-PER",
+ "B-ORG",
+ "I-ORG",
+ "B-LOC",
+ "I-LOC",
+ "B-MISC",
+ "I-MISC",
+ ]
+ )
+ ),
+ }
+ ),
+ supervised_keys=None,
+ homepage="https://www.aclweb.org/anthology/W03-0419/",
+ citation=_CITATION,
+ )
+
+ def _split_generators(self, dl_manager):
+ """Returns SplitGenerators."""
+ urls_to_download = {
+ "train": f"{_URL}{_TRAINING_FILE}",
+ "dev": f"{_URL}{_DEV_FILE}",
+ "test": f"{_URL}{_TEST_FILE}",
+ }
+ downloaded_files = dl_manager.download_and_extract(urls_to_download)
+
+ return [
+ datasets.SplitGenerator(name=datasets.Split.TRAIN, gen_kwargs={"filepath": downloaded_files["train"]}),
+ datasets.SplitGenerator(name=datasets.Split.VALIDATION, gen_kwargs={"filepath": downloaded_files["dev"]}),
+ datasets.SplitGenerator(name=datasets.Split.TEST, gen_kwargs={"filepath": downloaded_files["test"]}),
+ ]
+
+ def _generate_examples(self, filepath):
+ logger.info("⏳ Generating examples from = %s", filepath)
+ with open(filepath, encoding="utf-8") as f:
+ guid = 0
+ tokens = []
+ pos_tags = []
+ chunk_tags = []
+ ner_tags = []
+ for line in f:
+ if line.startswith("-DOCSTART-") or line == "" or line == "\n":
+ if tokens:
+ yield guid, {
+ "id": str(guid),
+ "tokens": tokens,
+ "pos_tags": pos_tags,
+ "chunk_tags": chunk_tags,
+ "ner_tags": ner_tags,
+ }
+ guid += 1
+ tokens = []
+ pos_tags = []
+ chunk_tags = []
+ ner_tags = []
+ else:
+ # conll2003 tokens are space separated
+ splits = line.split(" ")
+ tokens.append(splits[0])
+ pos_tags.append(splits[1])
+ chunk_tags.append(splits[2])
+ ner_tags.append(splits[3].rstrip())
+ # last example
+ yield guid, {
+ "id": str(guid),
+ "tokens": tokens,
+ "pos_tags": pos_tags,
+ "chunk_tags": chunk_tags,
+ "ner_tags": ner_tags,
+ }
\ No newline at end of file
diff --git a/tasks/ner/datasets/conll2004.py b/tasks/ner/datasets/conll2004.py
new file mode 100644
index 0000000..3eb14ff
--- /dev/null
+++ b/tasks/ner/datasets/conll2004.py
@@ -0,0 +1,130 @@
+# coding=utf-8
+# Copyright 2020 HuggingFace Datasets Authors.
+#
+# Licensed under the Apache License, Version 2.0 (the "License");
+# you may not use this file except in compliance with the License.
+# You may obtain a copy of the License at
+#
+# http://www.apache.org/licenses/LICENSE-2.0
+#
+# Unless required by applicable law or agreed to in writing, software
+# distributed under the License is distributed on an "AS IS" BASIS,
+# WITHOUT WARRANTIES OR CONDITIONS OF ANY KIND, either express or implied.
+# See the License for the specific language governing permissions and
+# limitations under the License.
+
+# Lint as: python3
+"""Introduction to the CoNLL-2003 Shared Task: Language-Independent Named Entity Recognition"""
+
+import datasets
+
+
+logger = datasets.logging.get_logger(__name__)
+
+
+_CITATION = """\
+@inproceedings{tjong-kim-sang-de-meulder-2003-introduction,
+ title = "Introduction to the {C}o{NLL}-2003 Shared Task: Language-Independent Named Entity Recognition",
+ author = "Tjong Kim Sang, Erik F. and
+ De Meulder, Fien",
+ booktitle = "Proceedings of the Seventh Conference on Natural Language Learning at {HLT}-{NAACL} 2003",
+ year = "2003",
+ url = "https://www.aclweb.org/anthology/W03-0419",
+ pages = "142--147",
+}
+"""
+
+_DESCRIPTION = """\
+The shared task of CoNLL-2003 concerns language-independent named entity recognition. We will concentrate on
+four types of named entities: persons, locations, organizations and names of miscellaneous entities that do
+not belong to the previous three groups.
+The CoNLL-2003 shared task data files contain four columns separated by a single space. Each word has been put on
+a separate line and there is an empty line after each sentence. The first item on each line is a word, the second
+a part-of-speech (POS) tag, the third a syntactic chunk tag and the fourth the named entity tag. The chunk tags
+and the named entity tags have the format I-TYPE which means that the word is inside a phrase of type TYPE. Only
+if two phrases of the same type immediately follow each other, the first word of the second phrase will have tag
+B-TYPE to show that it starts a new phrase. A word with tag O is not part of a phrase. Note the dataset uses IOB2
+tagging scheme, whereas the original dataset uses IOB1.
+For more details see https://www.clips.uantwerpen.be/conll2003/ner/ and https://www.aclweb.org/anthology/W03-0419
+"""
+
+_URL = "../../../data/CoNLL04/"
+_TRAINING_FILE = "train.txt"
+_DEV_FILE = "dev.txt"
+_TEST_FILE = "test.txt"
+
+
+class CoNLL2004Config(datasets.BuilderConfig):
+ """BuilderConfig for CoNLL2004"""
+
+ def __init__(self, **kwargs):
+ """BuilderConfig for CoNLL2004 5.0.
+ Args:
+ **kwargs: keyword arguments forwarded to super.
+ """
+ super(CoNLL2004Config, self).__init__(**kwargs)
+
+
+class CoNLL2004(datasets.GeneratorBasedBuilder):
+ """CoNLL2004 dataset."""
+
+ BUILDER_CONFIGS = [
+ CoNLL2004Config(name="CoNLL2004", version=datasets.Version("1.0.0"), description="CoNLL2004 dataset"),
+ ]
+
+ def _info(self):
+ return datasets.DatasetInfo(
+ description=_DESCRIPTION,
+ features=datasets.Features(
+ {
+ "id": datasets.Value("string"),
+ "tokens": datasets.Sequence(datasets.Value("string")),
+ "ner_tags": datasets.Sequence(
+ datasets.features.ClassLabel(
+ names= ['O', 'B-Loc', 'B-Peop', 'B-Org', 'B-Other', 'I-Loc', 'I-Peop', 'I-Org', 'I-Other']
+ )
+ ),
+ }
+ ),
+ supervised_keys=None,
+ homepage="https://catalog.ldc.upenn.edu/LDC2013T19",
+ citation=_CITATION,
+ )
+
+ def _split_generators(self, dl_manager):
+ """Returns SplitGenerators."""
+ urls_to_download = {
+ "train": f"{_URL}{_TRAINING_FILE}",
+ "dev": f"{_URL}{_DEV_FILE}",
+ "test": f"{_URL}{_TEST_FILE}",
+ }
+ downloaded_files = dl_manager.download_and_extract(urls_to_download)
+
+ return [
+ datasets.SplitGenerator(name=datasets.Split.TRAIN, gen_kwargs={"filepath": downloaded_files["train"]}),
+ datasets.SplitGenerator(name=datasets.Split.VALIDATION, gen_kwargs={"filepath": downloaded_files["dev"]}),
+ datasets.SplitGenerator(name=datasets.Split.TEST, gen_kwargs={"filepath": downloaded_files["test"]}),
+ ]
+
+ def _generate_examples(self, filepath):
+ logger.info("⏳ Generating examples from = %s", filepath)
+ with open(filepath, encoding="utf-8") as f:
+ guid = 0
+ tokens = []
+ ner_tags = []
+ for line in f:
+ if line.startswith("-DOCSTART-") or line == "" or line == "\n":
+ if tokens:
+ yield guid, {
+ "id": str(guid),
+ "tokens": tokens,
+ "ner_tags": ner_tags,
+ }
+ guid += 1
+ tokens = []
+ ner_tags = []
+ else:
+ # OntoNotes 5.0 tokens are space separated
+ splits = line.split(" ")
+ tokens.append(splits[0])
+ ner_tags.append(splits[-1].rstrip())
\ No newline at end of file
diff --git a/tasks/ner/datasets/ontonotes.py b/tasks/ner/datasets/ontonotes.py
new file mode 100644
index 0000000..e814ed8
--- /dev/null
+++ b/tasks/ner/datasets/ontonotes.py
@@ -0,0 +1,136 @@
+# coding=utf-8
+# Copyright 2020 HuggingFace Datasets Authors.
+#
+# Licensed under the Apache License, Version 2.0 (the "License");
+# you may not use this file except in compliance with the License.
+# You may obtain a copy of the License at
+#
+# http://www.apache.org/licenses/LICENSE-2.0
+#
+# Unless required by applicable law or agreed to in writing, software
+# distributed under the License is distributed on an "AS IS" BASIS,
+# WITHOUT WARRANTIES OR CONDITIONS OF ANY KIND, either express or implied.
+# See the License for the specific language governing permissions and
+# limitations under the License.
+
+# Lint as: python3
+"""Introduction to the CoNLL-2003 Shared Task: Language-Independent Named Entity Recognition"""
+
+import datasets
+
+
+logger = datasets.logging.get_logger(__name__)
+
+
+_CITATION = """\
+@inproceedings{tjong-kim-sang-de-meulder-2003-introduction,
+ title = "Introduction to the {C}o{NLL}-2003 Shared Task: Language-Independent Named Entity Recognition",
+ author = "Tjong Kim Sang, Erik F. and
+ De Meulder, Fien",
+ booktitle = "Proceedings of the Seventh Conference on Natural Language Learning at {HLT}-{NAACL} 2003",
+ year = "2003",
+ url = "https://www.aclweb.org/anthology/W03-0419",
+ pages = "142--147",
+}
+"""
+
+_DESCRIPTION = """\
+The shared task of CoNLL-2003 concerns language-independent named entity recognition. We will concentrate on
+four types of named entities: persons, locations, organizations and names of miscellaneous entities that do
+not belong to the previous three groups.
+The CoNLL-2003 shared task data files contain four columns separated by a single space. Each word has been put on
+a separate line and there is an empty line after each sentence. The first item on each line is a word, the second
+a part-of-speech (POS) tag, the third a syntactic chunk tag and the fourth the named entity tag. The chunk tags
+and the named entity tags have the format I-TYPE which means that the word is inside a phrase of type TYPE. Only
+if two phrases of the same type immediately follow each other, the first word of the second phrase will have tag
+B-TYPE to show that it starts a new phrase. A word with tag O is not part of a phrase. Note the dataset uses IOB2
+tagging scheme, whereas the original dataset uses IOB1.
+For more details see https://www.clips.uantwerpen.be/conll2003/ner/ and https://www.aclweb.org/anthology/W03-0419
+"""
+
+_URL = "../../../data/ontoNotes/"
+_TRAINING_FILE = "train.sd.conllx"
+_DEV_FILE = "dev.sd.conllx"
+_TEST_FILE = "test.sd.conllx"
+
+
+class OntoNotesConfig(datasets.BuilderConfig):
+ """BuilderConfig for OntoNotes 5.0"""
+
+ def __init__(self, **kwargs):
+ """BuilderConfig forOntoNotes 5.0.
+ Args:
+ **kwargs: keyword arguments forwarded to super.
+ """
+ super(OntoNotesConfig, self).__init__(**kwargs)
+
+
+class OntoNotes(datasets.GeneratorBasedBuilder):
+ """OntoNotes 5.0 dataset."""
+
+ BUILDER_CONFIGS = [
+ OntoNotesConfig(name="ontoNotes", version=datasets.Version("5.0.0"), description="ontoNotes 5.0 dataset"),
+ ]
+
+ def _info(self):
+ return datasets.DatasetInfo(
+ description=_DESCRIPTION,
+ features=datasets.Features(
+ {
+ "id": datasets.Value("string"),
+ "tokens": datasets.Sequence(datasets.Value("string")),
+ "ner_tags": datasets.Sequence(
+ datasets.features.ClassLabel(
+ names=['B-CARDINAL', 'B-DATE', 'B-EVENT', 'B-FAC', 'B-GPE', 'B-LANGUAGE', 'B-LAW', 'B-LOC', 'B-MONEY', 'B-NORP', 'B-ORDINAL', 'B-ORG', 'B-PERCENT', 'B-PERSON', 'B-PRODUCT', 'B-QUANTITY', 'B-TIME', 'B-WORK_OF_ART', 'I-CARDINAL', 'I-DATE', 'I-EVENT', 'I-FAC', 'I-GPE', 'I-LANGUAGE', 'I-LAW', 'I-LOC', 'I-MONEY', 'I-NORP', 'I-ORDINAL', 'I-ORG', 'I-PERCENT', 'I-PERSON', 'I-PRODUCT', 'I-QUANTITY', 'I-TIME', 'I-WORK_OF_ART', 'O']
+ )
+ ),
+ }
+ ),
+ supervised_keys=None,
+ homepage="https://catalog.ldc.upenn.edu/LDC2013T19",
+ citation=_CITATION,
+ )
+
+ def _split_generators(self, dl_manager):
+ """Returns SplitGenerators."""
+ urls_to_download = {
+ "train": f"{_URL}{_TRAINING_FILE}",
+ "dev": f"{_URL}{_DEV_FILE}",
+ "test": f"{_URL}{_TEST_FILE}",
+ }
+ downloaded_files = dl_manager.download_and_extract(urls_to_download)
+
+ return [
+ datasets.SplitGenerator(name=datasets.Split.TRAIN, gen_kwargs={"filepath": downloaded_files["train"]}),
+ datasets.SplitGenerator(name=datasets.Split.VALIDATION, gen_kwargs={"filepath": downloaded_files["dev"]}),
+ datasets.SplitGenerator(name=datasets.Split.TEST, gen_kwargs={"filepath": downloaded_files["test"]}),
+ ]
+
+ def _generate_examples(self, filepath):
+ logger.info("⏳ Generating examples from = %s", filepath)
+ with open(filepath, encoding="utf-8") as f:
+ guid = 0
+ tokens = []
+ ner_tags = []
+ for line in f:
+ if line.startswith("-DOCSTART-") or line == "" or line == "\n":
+ if tokens:
+ yield guid, {
+ "id": str(guid),
+ "tokens": tokens,
+ "ner_tags": ner_tags,
+ }
+ guid += 1
+ tokens = []
+ ner_tags = []
+ else:
+ # OntoNotes 5.0 tokens are space separated
+ splits = line.split("\t")
+ tokens.append(splits[1])
+ ner_tags.append(splits[-1].rstrip())
+ # last example
+ # yield guid, {
+ # "id": str(guid),
+ # "tokens": tokens,
+ # "ner_tags": ner_tags,
+ # }
\ No newline at end of file
diff --git a/tasks/ner/get_trainer.py b/tasks/ner/get_trainer.py
new file mode 100644
index 0000000..f32839a
--- /dev/null
+++ b/tasks/ner/get_trainer.py
@@ -0,0 +1,74 @@
+import logging
+import os
+import random
+import sys
+
+from transformers import (
+ AutoConfig,
+ AutoTokenizer,
+)
+
+from tasks.ner.dataset import NERDataset
+from training.trainer_exp import ExponentialTrainer
+from model.utils import get_model, TaskType
+from tasks.utils import ADD_PREFIX_SPACE, USE_FAST
+
+logger = logging.getLogger(__name__)
+
+
+def get_trainer(args):
+ model_args, data_args, training_args, qa_args = args
+
+ log_level = training_args.get_process_log_level()
+ logger.setLevel(log_level)
+
+ model_type = AutoConfig.from_pretrained(model_args.model_name_or_path).model_type
+
+ add_prefix_space = ADD_PREFIX_SPACE[model_type]
+
+ use_fast = USE_FAST[model_type]
+
+ tokenizer = AutoTokenizer.from_pretrained(
+ model_args.model_name_or_path,
+ use_fast=use_fast,
+ revision=model_args.model_revision,
+ add_prefix_space=add_prefix_space,
+ )
+
+ dataset = NERDataset(tokenizer, data_args, training_args)
+
+ if training_args.do_train:
+ for index in random.sample(range(len(dataset.train_dataset)), 3):
+ logger.info(f"Sample {index} of the training set: {dataset.train_dataset[index]}.")
+
+ if data_args.dataset_name == "conll2003":
+ config = AutoConfig.from_pretrained(
+ model_args.model_name_or_path,
+ num_labels=dataset.num_labels,
+ label2id=dataset.label_to_id,
+ id2label={i: l for l, i in dataset.label_to_id.items()},
+ revision=model_args.model_revision,
+ )
+ else:
+ config = AutoConfig.from_pretrained(
+ model_args.model_name_or_path,
+ num_labels=dataset.num_labels,
+ label2id=dataset.label_to_id,
+ id2label={i: l for l, i in dataset.label_to_id.items()},
+ revision=model_args.model_revision,
+ )
+
+ model = get_model(data_args, model_args, TaskType.TOKEN_CLASSIFICATION, config, fix_bert=True)
+
+ trainer = ExponentialTrainer(
+ model=model,
+ args=training_args,
+ train_dataset=dataset.train_dataset if training_args.do_train else None,
+ eval_dataset=dataset.eval_dataset if training_args.do_eval else None,
+ predict_dataset=dataset.predict_dataset if training_args.do_predict else None,
+ tokenizer=tokenizer,
+ data_collator=dataset.data_collator,
+ compute_metrics=dataset.compute_metrics,
+ test_key="f1"
+ )
+ return trainer, dataset.predict_dataset
diff --git a/tasks/other_cls/dataset.py b/tasks/other_cls/dataset.py
new file mode 100644
index 0000000..de0b929
--- /dev/null
+++ b/tasks/other_cls/dataset.py
@@ -0,0 +1,303 @@
+import torch
+from torch.utils import data
+from torch.utils.data import Dataset
+from datasets.arrow_dataset import Dataset as HFDataset
+from datasets.load import load_dataset, load_metric
+from transformers import (
+ AutoTokenizer,
+ DataCollatorWithPadding,
+ EvalPrediction,
+ default_data_collator,
+)
+import numpy as np
+import logging
+from typing import Optional
+
+
+# add by wjn
+def random_sampling(raw_datasets: load_dataset, data_type: str="train", num_examples_per_label: Optional[int]=16, label_set: list=None):
+ assert data_type in ["train", "dev", "test"]
+
+ label_list = raw_datasets[data_type]["label"] if "label" in raw_datasets[data_type].features else raw_datasets[data_type]["coarse_label"] # [0, 1, 0, 0, ...]
+ label_dict = dict()
+ # 记录每个label对应的样本索引
+ for ei, label in enumerate(label_list):
+ # print(label)
+ # if int(label) not in label_set: # 部分task存在-1的label,需要剔除
+ # continue
+ if label not in label_dict.keys():
+ label_dict[label] = list()
+ label_dict[label].append(ei)
+ # 对于每个类别,随机采样k个样本
+ few_example_ids = list()
+ for label, eid_list in label_dict.items():
+ # examples = deepcopy(eid_list)
+ # shuffle(examples)
+ idxs = np.random.choice(len(eid_list), size=num_examples_per_label, replace=False)
+ selected_eids = [eid_list[i] for i in idxs]
+ few_example_ids.extend(selected_eids)
+
+ # 保存没有被选中的example id
+ num_examples = len(label_list)
+ un_selected_examples_ids = [idx for idx in range(num_examples) if idx not in few_example_ids]
+ return few_example_ids, un_selected_examples_ids
+
+task_to_test_key = {
+ "movie_rationales": "accuracy",
+ "cr": "accuracy",
+ "snli": "accuracy",
+ "ag_news": "accuracy",
+ "yelp_polarity": "accuracy",
+ "trec": "accuracy",
+}
+
+task_to_keys = {
+ "movie_rationales": ("review", None),
+ "cr": ("sentence", None),
+ "snli": ("premise", "hypothesis"),
+ "ag_news": ("text", None),
+ "yelp_polarity": ("text", None),
+ "trec": ("text", None),
+}
+
+task_to_template = {
+ "movie_rationales": [{"prefix_template": "", "suffix_template": "It was !"}, None],
+ "cr": [{"prefix_template": "", "suffix_template": "It was ."}, None],
+ "snli": [None, {"prefix_template": " ? , ", "suffix_template": ""}],
+ "ag_news": [{"prefix_template": " News: ", "suffix_template": ""}, None],
+ "yelp_polarity": [{"prefix_template": "", "suffix_template": "It was ."}, None],
+ "trec": [{"prefix_template": " question: ", "suffix_template": ""}, None],
+
+}
+
+# add by wjn
+label_words_mapping = {
+ "movie_rationales": {"NEG": ["terrible"], "POS": ["great"]},
+ "cr": {"negative": ["terrible"], "positive": ["great"]},
+ "snli": {"contradiction": ["No"], "neutral": ["Maybe"], "entailment": ["Yes"]},
+ "ag_news": {"World" : ["World"], "Sports": ["Sports"], "Business": ["Business"], "Sci/Tech": ["Tech"]},
+ "yelp_polarity": {"1": ["bad"], "2": ["great"]},
+ "trec": {"DESC": ["Description"], "ENTY": ["Entity"], "ABBR": ["Abbreviation"], "HUM": ["Human"], "NUM": ["Numeric"], "LOC": ["Location"]}
+}
+
+
+logger = logging.getLogger(__name__)
+
+
+class OtherCLSDataset():
+ def __init__(
+ self,
+ tokenizer: AutoTokenizer,
+ data_args,
+ training_args,
+ semi_training_args=None,
+ use_prompt=None
+ ) -> None:
+ super().__init__()
+ raw_datasets = load_dataset(data_args.dataset_name)
+ self.tokenizer = tokenizer
+ self.data_args = data_args
+ #labels
+ self.is_regression = False
+ if not self.is_regression:
+ if self.data_args.dataset_name == "trec":
+ self.label_list = raw_datasets["train"].features["coarse_label"].names
+ else:
+ self.label_list = raw_datasets["train"].features["label"].names
+ self.num_labels = len(self.label_list)
+ else:
+ self.num_labels = 1
+ # print("self.label_list=", self.label_list)
+
+ # === generate template ===== add by wjn
+ self.use_prompt = False
+ if data_args.dataset_name in task_to_template.keys():
+ self.use_prompt = use_prompt
+
+ if self.use_prompt:
+ if 't5' in type(tokenizer).__name__.lower():
+ self.special_token_mapping = {
+ 'cls': 3, 'mask': 32099, 'sep': tokenizer.eos_token_id,
+ 'sep+': tokenizer.eos_token_id,
+ 'pseudo_token': tokenizer.unk_token_id
+ }
+ else:
+ self.special_token_mapping = {
+ 'cls': tokenizer.cls_token_id, 'mask': tokenizer.mask_token_id, 'sep': tokenizer.sep_token_id,
+ 'sep+': tokenizer.sep_token_id,
+ 'pseudo_token': tokenizer.unk_token_id
+ }
+ self.template = task_to_template[data_args.dataset_name] # dict
+
+ # === generate label word mapping ===== add by wjn
+ if self.use_prompt:
+ assert data_args.dataset_name in label_words_mapping.keys(), "You must define label word mapping for the task {}".format(data_args.dataset_name)
+ self.label_to_word = label_words_mapping[data_args.dataset_name] # e.g., {"0": ["great"], "1": [bad]}
+ self.label_to_word = {label: label_word[0] if type(label_word) == list else label_word for label, label_word in self.label_to_word.items()}
+
+ for key in self.label_to_word:
+ # For RoBERTa/BART/T5, tokenization also considers space, so we use space+word as label words.
+ if self.label_to_word[key][0] not in ['<', '[', '.', ',']:
+ # Make sure space+word is in the vocabulary
+ # assert len(tokenizer.tokenize(' ' + self.label_to_word[key])) == 1
+ self.label_to_word[key] = tokenizer.convert_tokens_to_ids(tokenizer.tokenize(' ' + self.label_to_word[key])[0])
+ else:
+ self.label_to_word[key] = tokenizer.convert_tokens_to_ids(self.label_to_word[key])
+ logger.info("Label {} to word {} ({})".format(key, tokenizer._convert_id_to_token(self.label_to_word[key]), self.label_to_word[key]))
+
+ if len(self.label_list) > 1:
+ self.label_word_list = [self.label_to_word[label] for label in self.label_list]
+ else:
+ # Regression task
+ # '0' represents low polarity and '1' represents high polarity.
+ self.label_word_list = [self.label_to_word[label] for label in ['0', '1']]
+ # =============
+
+ # Preprocessing the raw_datasets
+ self.sentence1_key, self.sentence2_key = task_to_keys[data_args.dataset_name]
+
+ # Padding strategy
+ if data_args.pad_to_max_length:
+ self.padding = "max_length"
+ else:
+ # We will pad later, dynamically at batch creation, to the max sequence length in each batch
+ self.padding = False
+
+ # Some models have set the order of the labels to use, so let's make sure we do use it.
+ if not self.is_regression:
+ self.label2id = {l: i for i, l in enumerate(self.label_list)}
+ self.id2label = {id: label for label, id in self.label2id.items()}
+
+ if data_args.max_seq_length > tokenizer.model_max_length:
+ logger.warning(
+ f"The max_seq_length passed ({data_args.max_seq_length}) is larger than the maximum length for the"
+ f"model ({tokenizer.model_max_length}). Using max_seq_length={tokenizer.model_max_length}."
+ )
+ self.max_seq_length = min(data_args.max_seq_length, tokenizer.model_max_length)
+
+ raw_datasets = raw_datasets.map(
+ self.preprocess_function,
+ batched=True,
+ load_from_cache_file=not data_args.overwrite_cache,
+ desc="Running tokenizer on dataset",
+ )
+
+ if training_args.do_train:
+ self.train_dataset = raw_datasets["train"]
+ if data_args.max_train_samples is not None:
+ self.train_dataset = self.train_dataset.select(range(data_args.max_train_samples))
+
+ if training_args.do_eval and data_args.dataset_name not in ["ag_news", "yelp_polarity", "trec"]:
+ self.eval_dataset = raw_datasets["validation_matched" if data_args.dataset_name == "mnli" else "validation"]
+ if data_args.max_eval_samples is not None:
+ self.eval_dataset = self.eval_dataset.select(range(data_args.max_eval_samples))
+
+ if training_args.do_predict or data_args.dataset_name is not None or data_args.test_file is not None:
+ self.predict_dataset = raw_datasets["test_matched" if data_args.dataset_name == "mnli" else "test"]
+ if data_args.max_predict_samples is not None:
+ self.predict_dataset = self.predict_dataset.select(range(data_args.max_predict_samples))
+
+ if data_args.dataset_name in ["ag_news", "yelp_polarity", "trec"]:
+ self.eval_dataset = self.predict_dataset
+ # add by wjn
+ self.unlabeled_dataset = None
+ if semi_training_args.use_semi is True:
+ assert data_args.num_examples_per_label is not None and data_args.num_examples_per_label != -1
+
+ # 随机采样few-shot training / dev data(传入label_list,对每个label进行采样,最后得到索引列表)
+ if data_args.num_examples_per_label is not None and data_args.num_examples_per_label != -1:
+ train_examples_idx_list, un_selected_idx_list = random_sampling(
+ raw_datasets=raw_datasets,
+ data_type="train",
+ num_examples_per_label=data_args.num_examples_per_label,
+ label_set=list(self.id2label.keys())
+ )
+ self.all_train_dataset = self.train_dataset
+ self.train_dataset = self.all_train_dataset.select(train_examples_idx_list)
+ print("Randomly sampling {}-shot training examples for each label. Total examples number is {}".format(
+ data_args.num_examples_per_label,
+ len(self.train_dataset)
+ ))
+
+ if semi_training_args.use_semi is True:
+ self.unlabeled_dataset = self.all_train_dataset.select(un_selected_idx_list)
+ print("The number of unlabeled data is {}".format(len(self.unlabeled_dataset)))
+
+ self.metric = load_metric("./metrics/accuracy", data_args.dataset_name)
+
+ if data_args.pad_to_max_length:
+ self.data_collator = default_data_collator
+ elif training_args.fp16:
+ self.data_collator = DataCollatorWithPadding(tokenizer, pad_to_multiple_of=8)
+
+ # ==== define test key ====== add by wjn
+ self.test_key = task_to_test_key[data_args.dataset_name]
+
+
+ def preprocess_function(self, examples):
+ # add by wjn
+ if self.data_args.dataset_name == "trec":
+ examples["label"] = []
+ for label in examples["coarse_label"]:
+ examples["label"].append(label)
+
+
+ # add by wjn
+ # adding prompt into each example
+ if self.use_prompt:
+ # if use prompt, insert template into example
+ examples = self.prompt_preprocess_function(examples)
+
+ # Tokenize the texts
+ args = (
+ (examples[self.sentence1_key],) if self.sentence2_key is None else (examples[self.sentence1_key], examples[self.sentence2_key])
+ )
+ result = self.tokenizer(*args, padding=self.padding, max_length=self.max_seq_length, truncation=True)
+
+ if self.use_prompt:
+ mask_pos = []
+ for input_ids in result["input_ids"]:
+ mask_pos.append(input_ids.index(self.special_token_mapping["mask"]))
+ result["mask_pos"] = mask_pos
+
+ return result
+
+ # add by wjn
+ # process data for prompt (add template)
+ def prompt_preprocess_function(self, examples):
+
+ def replace_mask_token(template):
+ return template.replace("", self.tokenizer.convert_ids_to_tokens(self.special_token_mapping["mask"]))
+
+ sequence1_prefix_template = replace_mask_token(self.template[0]["prefix_template"] if self.template[0] is not None else "")
+ sequence1_suffix_template = replace_mask_token(self.template[0]["suffix_template"] if self.template[0] is not None else "")
+ sequence2_prefix_template = replace_mask_token(self.template[1]["prefix_template"] if self.template[1] is not None else "")
+ sequence2_suffix_template = replace_mask_token(self.template[1]["suffix_template"] if self.template[1] is not None else "")
+ example_num = len(examples[self.sentence1_key])
+ for example_id in range(example_num):
+ sequence1 = examples[self.sentence1_key][example_id]
+ if self.sentence2_key is None:
+ sequence1 = sequence1[:self.data_args.max_seq_length - len(sequence1_suffix_template) - 10]
+ examples[self.sentence1_key][example_id] = "{}{}{}".format(
+ sequence1_prefix_template, sequence1, sequence1_suffix_template)
+
+ if self.sentence2_key is not None:
+ sequence2 = examples[self.sentence2_key][example_id]
+ sequence2 = sequence2[:self.data_args.max_seq_length - len(sequence1) - len(sequence1_prefix_template) - len(sequence1_suffix_template) - len(sequence2_prefix_template)- 10]
+ examples[self.sentence2_key][example_id] = "{}{}{}".format(
+ sequence2_prefix_template, sequence2, sequence2_suffix_template)
+ return examples
+
+ def compute_metrics(self, p: EvalPrediction):
+ preds = p.predictions[0] if isinstance(p.predictions, tuple) else p.predictions
+ preds = np.squeeze(preds) if self.is_regression else np.argmax(preds, axis=1)
+ if self.data_args.dataset_name is not None:
+ result = self.metric.compute(predictions=preds, references=p.label_ids)
+ if len(result) > 1:
+ result["combined_score"] = np.mean(list(result.values())).item()
+ return result
+ elif self.is_regression:
+ return {"mse": ((preds - p.label_ids) ** 2).mean().item()}
+ else:
+ return {"accuracy": (preds == p.label_ids).astype(np.float32).mean().item()}
+
diff --git a/tasks/other_cls/get_trainer.py b/tasks/other_cls/get_trainer.py
new file mode 100644
index 0000000..7be57b8
--- /dev/null
+++ b/tasks/other_cls/get_trainer.py
@@ -0,0 +1,96 @@
+import logging
+import os
+import random
+import sys
+
+from transformers import (
+ AutoConfig,
+ AutoTokenizer,
+)
+
+from model.utils import get_model, TaskType
+from tasks.other_cls.dataset import OtherCLSDataset
+from training.trainer_base import BaseTrainer
+from training.self_trainer import SelfTrainer
+
+logger = logging.getLogger(__name__)
+
+def get_trainer(args):
+ model_args, data_args, training_args, semi_training_args, _ = args
+
+ tokenizer = AutoTokenizer.from_pretrained(
+ model_args.model_name_or_path,
+ use_fast=model_args.use_fast_tokenizer,
+ revision=model_args.model_revision,
+ )
+
+ # add by wjn check if use prompt template
+ use_prompt = False
+ if model_args.prompt_prefix or model_args.prompt_ptuning or model_args.prompt_adapter or model_args.prompt_only:
+ use_prompt = True
+
+ dataset = OtherCLSDataset(tokenizer, data_args, training_args, semi_training_args=semi_training_args, use_prompt=use_prompt)
+
+ data_args.label_word_list = None # add by wjn
+ if use_prompt:
+ data_args.label_word_list = dataset.label_word_list # add by wjn
+
+ if training_args.do_train:
+ for index in random.sample(range(len(dataset.train_dataset)), 3):
+ logger.info(f"Sample {index} of the training set: {dataset.train_dataset[index]}.")
+
+ if not dataset.is_regression:
+ config = AutoConfig.from_pretrained(
+ model_args.model_name_or_path,
+ num_labels=dataset.num_labels,
+ label2id=dataset.label2id,
+ id2label=dataset.id2label,
+ finetuning_task=data_args.dataset_name,
+ revision=model_args.model_revision,
+ )
+ else:
+ config = AutoConfig.from_pretrained(
+ model_args.model_name_or_path,
+ num_labels=dataset.num_labels,
+ finetuning_task=data_args.dataset_name,
+ revision=model_args.model_revision,
+ )
+
+ model = get_model(data_args, model_args, TaskType.SEQUENCE_CLASSIFICATION, config)
+
+ # Initialize our Trainer
+
+ if semi_training_args.use_semi:
+ model_args.pre_seq_len = semi_training_args.student_pre_seq_len
+ student_model = get_model(data_args, model_args, TaskType.SEQUENCE_CLASSIFICATION, config)
+ trainer = SelfTrainer(
+ teacher_base_model=model,
+ student_base_model=student_model,
+ training_args=training_args,
+ semi_training_args=semi_training_args,
+ train_dataset=dataset.train_dataset if training_args.do_train else None,
+ unlabeled_dataset=dataset.unlabeled_dataset,
+ eval_dataset=dataset.eval_dataset if training_args.do_eval else None,
+ compute_metrics=dataset.compute_metrics,
+ tokenizer=tokenizer,
+ teacher_data_collator=dataset.data_collator,
+ student_data_collator=dataset.data_collator,
+ test_key=dataset.test_key,
+ task_type="cls",
+ num_classes=len(dataset.label2id),
+ )
+
+ return trainer, None
+
+ trainer = BaseTrainer(
+ model=model,
+ args=training_args,
+ train_dataset=dataset.train_dataset if training_args.do_train else None,
+ eval_dataset=dataset.eval_dataset if training_args.do_eval else None,
+ compute_metrics=dataset.compute_metrics,
+ tokenizer=tokenizer,
+ data_collator=dataset.data_collator,
+ test_key=dataset.test_key,
+ )
+
+ return trainer, None
\ No newline at end of file
diff --git a/tasks/qa/dataset.py b/tasks/qa/dataset.py
new file mode 100644
index 0000000..191f38b
--- /dev/null
+++ b/tasks/qa/dataset.py
@@ -0,0 +1,207 @@
+import torch
+from torch.utils.data.sampler import RandomSampler, SequentialSampler
+from torch.utils.data import DataLoader
+from datasets.arrow_dataset import Dataset as HFDataset
+from datasets.load import load_metric, load_dataset
+from transformers import AutoTokenizer, DataCollatorForTokenClassification, BertConfig
+from transformers import default_data_collator, EvalPrediction
+import numpy as np
+import logging
+from typing import Optional
+from tasks.qa.utils_qa import postprocess_qa_predictions
+
+# add by wjn
+def random_sampling(raw_datasets: load_dataset, data_type: str="train", num_examples_per_label: Optional[int]=16):
+ assert data_type in ["train", "dev", "test"]
+ example_id_list = raw_datasets[data_type]["id"] # [0, 1, 0, 0, ...]
+ # 对于每个类别,随机采样k个样本
+ few_example_ids = list()
+ few_example_ids = np.random.choice(len(example_id_list), size=num_examples_per_label, replace=False)
+ # few_example_ids = [example_id_list[i] for i in idxs]
+ return few_example_ids
+
+
+class SQuAD:
+
+ def __init__(self, tokenizer: AutoTokenizer, data_args, training_args, qa_args) -> None:
+ self.data_args = data_args
+ self.training_args = training_args
+ self.qa_args = qa_args
+ self.version_2 = data_args.dataset_name == "squad_v2"
+
+ raw_datasets = load_dataset(data_args.dataset_name)
+ column_names = raw_datasets['train'].column_names
+ self.question_column_name = "question"
+ self.context_column_name = "context"
+ self.answer_column_name = "answers"
+
+ self.tokenizer = tokenizer
+
+ self.pad_on_right = tokenizer.padding_side == "right" # True
+ self.max_seq_len = 384 #data_args.max_seq_length
+
+ if training_args.do_train:
+ self.train_dataset = raw_datasets['train']
+ self.train_dataset = self.train_dataset.map(
+ self.prepare_train_dataset,
+ batched=True,
+ remove_columns=column_names,
+ load_from_cache_file=True,
+ desc="Running tokenizer on train dataset",
+ )
+ if data_args.max_train_samples is not None:
+ self.train_dataset = self.train_dataset.select(range(data_args.max_train_samples))
+
+ if training_args.do_eval:
+ self.eval_examples = raw_datasets['validation']
+ if data_args.max_eval_samples is not None:
+ self.eval_examples = self.eval_examples.select(range(data_args.max_eval_samples))
+ self.eval_dataset = self.eval_examples.map(
+ self.prepare_eval_dataset,
+ batched=True,
+ remove_columns=column_names,
+ load_from_cache_file=True,
+ desc="Running tokenizer on validation dataset",
+ )
+ if data_args.max_eval_samples is not None:
+ self.eval_dataset = self.eval_dataset.select(range(data_args.max_eval_samples))
+
+ # add by wjn
+ # 随机采样few-shot training / dev data(传入label_list,对每个label进行采样,最后得到索引列表)
+ if data_args.num_examples_per_label is not None:
+ train_examples_idx_list = random_sampling(
+ raw_datasets=raw_datasets,
+ data_type="train",
+ num_examples_per_label=data_args.num_examples_per_label
+ )
+ self.train_dataset = self.train_dataset.select(train_examples_idx_list)
+ print("Randomly sampling {}-shot training examples for each label. Total examples number is {}".format(
+ data_args.num_examples_per_label,
+ len(self.train_dataset)
+ ))
+
+ self.predict_dataset = None
+
+ self.data_collator = default_data_collator
+
+ self.metric = load_metric(data_args.dataset_name)
+
+ def prepare_train_dataset(self, examples):
+ examples['question'] = [q.lstrip() for q in examples['question']]
+
+ tokenized = self.tokenizer(
+ examples['question' if self.pad_on_right else 'context'],
+ examples['context' if self.pad_on_right else 'question'],
+ truncation='only_second' if self.pad_on_right else 'only_first',
+ max_length=self.max_seq_len,
+ stride=128,
+ return_overflowing_tokens=True,
+ return_offsets_mapping=True,
+ padding="max_length",
+ )
+
+ sample_maping = tokenized.pop("overflow_to_sample_mapping")
+ offset_mapping = tokenized.pop("offset_mapping")
+ tokenized["start_positions"] = []
+ tokenized["end_positions"] = []
+
+ for i, offsets in enumerate(offset_mapping):
+ input_ids = tokenized['input_ids'][i]
+ cls_index = input_ids.index(self.tokenizer.cls_token_id)
+
+ sequence_ids = tokenized.sequence_ids(i)
+ sample_index = sample_maping[i]
+ answers = examples['answers'][sample_index]
+
+ if len(answers['answer_start']) == 0:
+ tokenized["start_positions"].append(cls_index)
+ tokenized["end_positions"].append(cls_index)
+ else:
+ start_char = answers["answer_start"][0]
+ end_char = start_char + len(answers["text"][0])
+
+ token_start_index = 0
+ while sequence_ids[token_start_index] != (1 if self.pad_on_right else 0):
+ token_start_index += 1
+
+ token_end_index = len(input_ids) - 1
+ while sequence_ids[token_end_index] != (1 if self.pad_on_right else 0):
+ token_end_index -= 1
+
+ # Detect if the answer is out of the span
+ # (in which case this feature is labeled with the CLS index).
+ if not (offsets[token_start_index][0] <= start_char and offsets[token_end_index][1] >= end_char):
+ tokenized["start_positions"].append(cls_index)
+ tokenized["end_positions"].append(cls_index)
+ else:
+ # Otherwise move the token_start_index and token_end_index to the two ends of the answer.
+ # Note: we could go after the last offset if the answer is the last word (edge case).
+ while token_start_index < len(offsets) and offsets[token_start_index][0] <= start_char:
+ token_start_index += 1
+ tokenized["start_positions"].append(token_start_index - 1)
+ while offsets[token_end_index][1] >= end_char:
+ token_end_index -= 1
+ tokenized["end_positions"].append(token_end_index + 1)
+
+ return tokenized
+
+ def prepare_eval_dataset(self, examples):
+ # if self.version_2:
+ examples['question'] = [q.lstrip() for q in examples['question']]
+
+ tokenized = self.tokenizer(
+ examples['question' if self.pad_on_right else 'context'],
+ examples['context' if self.pad_on_right else 'question'],
+ truncation='only_second' if self.pad_on_right else 'only_first',
+ max_length=self.max_seq_len,
+ stride=128,
+ return_overflowing_tokens=True,
+ return_offsets_mapping=True,
+ padding="max_length",
+ )
+
+ sample_mapping = tokenized.pop("overflow_to_sample_mapping")
+ tokenized["example_id"] = []
+
+ for i in range(len(tokenized["input_ids"])):
+ # Grab the sequence corresponding to that example (to know what is the context and what is the question).
+ sequence_ids = tokenized.sequence_ids(i)
+ context_index = 1 if self.pad_on_right else 0
+
+ # One example can give several spans, this is the index of the example containing this span of text.
+ sample_index = sample_mapping[i]
+ tokenized["example_id"].append(examples["id"][sample_index])
+
+ # Set to None the offset_mapping that are not part of the context so it's easy to determine if a token
+ # position is part of the context or not.
+ tokenized["offset_mapping"][i] = [
+ (o if sequence_ids[k] == context_index else None)
+ for k, o in enumerate(tokenized["offset_mapping"][i])
+ ]
+ return tokenized
+
+ def compute_metrics(self, p: EvalPrediction):
+ return self.metric.compute(predictions=p.predictions, references=p.label_ids)
+
+ def post_processing_function(self, examples, features, predictions, stage='eval'):
+ predictions = postprocess_qa_predictions(
+ examples=examples,
+ features=features,
+ predictions=predictions,
+ version_2_with_negative=self.version_2,
+ n_best_size=self.qa_args.n_best_size,
+ max_answer_length=self.qa_args.max_answer_length,
+ null_score_diff_threshold=self.qa_args.null_score_diff_threshold,
+ output_dir=self.training_args.output_dir,
+ prefix=stage,
+ log_level=logging.INFO
+ )
+ if self.version_2: # squad_v2
+ formatted_predictions = [
+ {"id": k, "prediction_text": v, "no_answer_probability": 0.0} for k, v in predictions.items()
+ ]
+ else:
+ formatted_predictions = [{"id": k, "prediction_text": v} for k, v in predictions.items()]
+
+ references = [{"id": ex["id"], "answers": ex['answers']} for ex in examples]
+ return EvalPrediction(predictions=formatted_predictions, label_ids=references)
diff --git a/tasks/qa/get_trainer.py b/tasks/qa/get_trainer.py
new file mode 100644
index 0000000..00a620a
--- /dev/null
+++ b/tasks/qa/get_trainer.py
@@ -0,0 +1,50 @@
+import logging
+import os
+import random
+import sys
+
+from transformers import (
+ AutoConfig,
+ AutoTokenizer,
+)
+
+from tasks.qa.dataset import SQuAD
+from training.trainer_qa import QuestionAnsweringTrainer
+from model.utils import get_model, TaskType
+
+logger = logging.getLogger(__name__)
+
+def get_trainer(args):
+ model_args, data_args, training_args, semi_training_args, qa_args = args
+
+ config = AutoConfig.from_pretrained(
+ model_args.model_name_or_path,
+ num_labels=2,
+ revision=model_args.model_revision,
+ )
+
+ tokenizer = AutoTokenizer.from_pretrained(
+ model_args.model_name_or_path,
+ revision=model_args.model_revision,
+ use_fast=True,
+ )
+
+ model = get_model(data_args, model_args, TaskType.QUESTION_ANSWERING, config, fix_bert=True)
+
+ dataset = SQuAD(tokenizer, data_args, training_args, qa_args)
+
+ trainer = QuestionAnsweringTrainer(
+ model=model,
+ args=training_args,
+ train_dataset=dataset.train_dataset if training_args.do_train else None,
+ eval_dataset=dataset.eval_dataset if training_args.do_eval else None,
+ eval_examples=dataset.eval_examples if training_args.do_eval else None,
+ tokenizer=tokenizer,
+ data_collator=dataset.data_collator,
+ post_process_function=dataset.post_processing_function,
+ compute_metrics=dataset.compute_metrics,
+ )
+
+ return trainer, dataset.predict_dataset
+
+
diff --git a/tasks/qa/utils_qa.py b/tasks/qa/utils_qa.py
new file mode 100644
index 0000000..159cbf2
--- /dev/null
+++ b/tasks/qa/utils_qa.py
@@ -0,0 +1,427 @@
+# coding=utf-8
+# Copyright 2020 The HuggingFace Team All rights reserved.
+#
+# Licensed under the Apache License, Version 2.0 (the "License");
+# you may not use this file except in compliance with the License.
+# You may obtain a copy of the License at
+#
+# http://www.apache.org/licenses/LICENSE-2.0
+#
+# Unless required by applicable law or agreed to in writing, software
+# distributed under the License is distributed on an "AS IS" BASIS,
+# WITHOUT WARRANTIES OR CONDITIONS OF ANY KIND, either express or implied.
+# See the License for the specific language governing permissions and
+# limitations under the License.
+"""
+Post-processing utilities for question answering.
+"""
+import collections
+import json
+import logging
+import os
+from typing import Optional, Tuple
+
+import numpy as np
+from tqdm.auto import tqdm
+
+
+logger = logging.getLogger(__name__)
+
+
+def postprocess_qa_predictions(
+ examples,
+ features,
+ predictions: Tuple[np.ndarray, np.ndarray],
+ version_2_with_negative: bool = False,
+ n_best_size: int = 20,
+ max_answer_length: int = 30,
+ null_score_diff_threshold: float = 0.0,
+ output_dir: Optional[str] = None,
+ prefix: Optional[str] = None,
+ log_level: Optional[int] = logging.WARNING,
+):
+ """
+ Post-processes the predictions of a question-answering model to convert them to answers that are substrings of the
+ original contexts. This is the base postprocessing functions for models that only return start and end logits.
+
+ Args:
+ examples: The non-preprocessed dataset (see the main script for more information).
+ features: The processed dataset (see the main script for more information).
+ predictions (:obj:`Tuple[np.ndarray, np.ndarray]`):
+ The predictions of the model: two arrays containing the start logits and the end logits respectively. Its
+ first dimension must match the number of elements of :obj:`features`.
+ version_2_with_negative (:obj:`bool`, `optional`, defaults to :obj:`False`):
+ Whether or not the underlying dataset contains examples with no answers.
+ n_best_size (:obj:`int`, `optional`, defaults to 20):
+ The total number of n-best predictions to generate when looking for an answer.
+ max_answer_length (:obj:`int`, `optional`, defaults to 30):
+ The maximum length of an answer that can be generated. This is needed because the start and end predictions
+ are not conditioned on one another.
+ null_score_diff_threshold (:obj:`float`, `optional`, defaults to 0):
+ The threshold used to select the null answer: if the best answer has a score that is less than the score of
+ the null answer minus this threshold, the null answer is selected for this example (note that the score of
+ the null answer for an example giving several features is the minimum of the scores for the null answer on
+ each feature: all features must be aligned on the fact they `want` to predict a null answer).
+
+ Only useful when :obj:`version_2_with_negative` is :obj:`True`.
+ output_dir (:obj:`str`, `optional`):
+ If provided, the dictionaries of predictions, n_best predictions (with their scores and logits) and, if
+ :obj:`version_2_with_negative=True`, the dictionary of the scores differences between best and null
+ answers, are saved in `output_dir`.
+ prefix (:obj:`str`, `optional`):
+ If provided, the dictionaries mentioned above are saved with `prefix` added to their names.
+ log_level (:obj:`int`, `optional`, defaults to ``logging.WARNING``):
+ ``logging`` log level (e.g., ``logging.WARNING``)
+ """
+ assert len(predictions) == 2, "`predictions` should be a tuple with two elements (start_logits, end_logits)."
+ all_start_logits, all_end_logits = predictions
+
+ assert len(predictions[0]) == len(features), f"Got {len(predictions[0])} predictions and {len(features)} features."
+
+ # Build a map example to its corresponding features.
+ example_id_to_index = {k: i for i, k in enumerate(examples["id"])}
+ features_per_example = collections.defaultdict(list)
+ for i, feature in enumerate(features):
+ features_per_example[example_id_to_index[feature["example_id"]]].append(i)
+
+ # The dictionaries we have to fill.
+ all_predictions = collections.OrderedDict()
+ all_nbest_json = collections.OrderedDict()
+ if version_2_with_negative:
+ scores_diff_json = collections.OrderedDict()
+
+ # Logging.
+ logger.setLevel(log_level)
+ logger.info(f"Post-processing {len(examples)} example predictions split into {len(features)} features.")
+
+ # Let's loop over all the examples!
+ for example_index, example in enumerate(tqdm(examples)):
+ # Those are the indices of the features associated to the current example.
+ feature_indices = features_per_example[example_index]
+
+ min_null_prediction = None
+ prelim_predictions = []
+ # Looping through all the features associated to the current example.
+ for feature_index in feature_indices:
+ # We grab the predictions of the model for this feature.
+ start_logits = all_start_logits[feature_index]
+ end_logits = all_end_logits[feature_index]
+ # This is what will allow us to map some the positions in our logits to span of texts in the original
+ # context.
+ offset_mapping = features[feature_index]["offset_mapping"]
+ # Optional `token_is_max_context`, if provided we will remove answers that do not have the maximum context
+ # available in the current feature.
+ token_is_max_context = features[feature_index].get("token_is_max_context", None)
+
+ # Update minimum null prediction.
+ feature_null_score = start_logits[0] + end_logits[0]
+ if min_null_prediction is None or min_null_prediction["score"] > feature_null_score:
+ min_null_prediction = {
+ "offsets": (0, 0),
+ "score": feature_null_score,
+ "start_logit": start_logits[0],
+ "end_logit": end_logits[0],
+ }
+
+ # Go through all possibilities for the `n_best_size` greater start and end logits.
+ start_indexes = np.argsort(start_logits)[-1 : -n_best_size - 1 : -1].tolist()
+ end_indexes = np.argsort(end_logits)[-1 : -n_best_size - 1 : -1].tolist()
+ for start_index in start_indexes:
+ for end_index in end_indexes:
+ # Don't consider out-of-scope answers, either because the indices are out of bounds or correspond
+ # to part of the input_ids that are not in the context.
+ if (
+ start_index >= len(offset_mapping)
+ or end_index >= len(offset_mapping)
+ or offset_mapping[start_index] is None
+ or offset_mapping[end_index] is None
+ ):
+ continue
+ # Don't consider answers with a length that is either < 0 or > max_answer_length.
+ if end_index < start_index or end_index - start_index + 1 > max_answer_length:
+ continue
+ # Don't consider answer that don't have the maximum context available (if such information is
+ # provided).
+ if token_is_max_context is not None and not token_is_max_context.get(str(start_index), False):
+ continue
+ prelim_predictions.append(
+ {
+ "offsets": (offset_mapping[start_index][0], offset_mapping[end_index][1]),
+ "score": start_logits[start_index] + end_logits[end_index],
+ "start_logit": start_logits[start_index],
+ "end_logit": end_logits[end_index],
+ }
+ )
+
+ if version_2_with_negative:
+ # Add the minimum null prediction
+ prelim_predictions.append(min_null_prediction)
+ null_score = min_null_prediction["score"]
+
+ # Only keep the best `n_best_size` predictions.
+ predictions = sorted(prelim_predictions, key=lambda x: x["score"], reverse=True)[:n_best_size]
+
+ # Add back the minimum null prediction if it was removed because of its low score.
+ if version_2_with_negative and not any(p["offsets"] == (0, 0) for p in predictions):
+ predictions.append(min_null_prediction)
+
+ # Use the offsets to gather the answer text in the original context.
+ context = example["context"]
+ for pred in predictions:
+ offsets = pred.pop("offsets")
+ pred["text"] = context[offsets[0] : offsets[1]]
+
+ # In the very rare edge case we have not a single non-null prediction, we create a fake prediction to avoid
+ # failure.
+ if len(predictions) == 0 or (len(predictions) == 1 and predictions[0]["text"] == ""):
+ predictions.insert(0, {"text": "empty", "start_logit": 0.0, "end_logit": 0.0, "score": 0.0})
+
+ # Compute the softmax of all scores (we do it with numpy to stay independent from torch/tf in this file, using
+ # the LogSumExp trick).
+ scores = np.array([pred.pop("score") for pred in predictions])
+ exp_scores = np.exp(scores - np.max(scores))
+ probs = exp_scores / exp_scores.sum()
+
+ # Include the probabilities in our predictions.
+ for prob, pred in zip(probs, predictions):
+ pred["probability"] = prob
+
+ # Pick the best prediction. If the null answer is not possible, this is easy.
+ if not version_2_with_negative:
+ all_predictions[example["id"]] = predictions[0]["text"]
+ else:
+ # Otherwise we first need to find the best non-empty prediction.
+ i = 0
+ while predictions[i]["text"] == "":
+ i += 1
+ best_non_null_pred = predictions[i]
+
+ # Then we compare to the null prediction using the threshold.
+ score_diff = null_score - best_non_null_pred["start_logit"] - best_non_null_pred["end_logit"]
+ scores_diff_json[example["id"]] = float(score_diff) # To be JSON-serializable.
+ if score_diff > null_score_diff_threshold:
+ all_predictions[example["id"]] = ""
+ else:
+ all_predictions[example["id"]] = best_non_null_pred["text"]
+
+ # Make `predictions` JSON-serializable by casting np.float back to float.
+ all_nbest_json[example["id"]] = [
+ {k: (float(v) if isinstance(v, (np.float16, np.float32, np.float64)) else v) for k, v in pred.items()}
+ for pred in predictions
+ ]
+
+ # If we have an output_dir, let's save all those dicts.
+ if output_dir is not None:
+ assert os.path.isdir(output_dir), f"{output_dir} is not a directory."
+
+ prediction_file = os.path.join(
+ output_dir, "predictions.json" if prefix is None else f"{prefix}_predictions.json"
+ )
+ nbest_file = os.path.join(
+ output_dir, "nbest_predictions.json" if prefix is None else f"{prefix}_nbest_predictions.json"
+ )
+ if version_2_with_negative:
+ null_odds_file = os.path.join(
+ output_dir, "null_odds.json" if prefix is None else f"{prefix}_null_odds.json"
+ )
+
+ logger.info(f"Saving predictions to {prediction_file}.")
+ with open(prediction_file, "w") as writer:
+ writer.write(json.dumps(all_predictions, indent=4) + "\n")
+ logger.info(f"Saving nbest_preds to {nbest_file}.")
+ with open(nbest_file, "w") as writer:
+ writer.write(json.dumps(all_nbest_json, indent=4) + "\n")
+ if version_2_with_negative:
+ logger.info(f"Saving null_odds to {null_odds_file}.")
+ with open(null_odds_file, "w") as writer:
+ writer.write(json.dumps(scores_diff_json, indent=4) + "\n")
+
+ return all_predictions
+
+
+def postprocess_qa_predictions_with_beam_search(
+ examples,
+ features,
+ predictions: Tuple[np.ndarray, np.ndarray],
+ version_2_with_negative: bool = False,
+ n_best_size: int = 20,
+ max_answer_length: int = 30,
+ start_n_top: int = 5,
+ end_n_top: int = 5,
+ output_dir: Optional[str] = None,
+ prefix: Optional[str] = None,
+ log_level: Optional[int] = logging.WARNING,
+):
+ """
+ Post-processes the predictions of a question-answering model with beam search to convert them to answers that are substrings of the
+ original contexts. This is the postprocessing functions for models that return start and end logits, indices, as well as
+ cls token predictions.
+
+ Args:
+ examples: The non-preprocessed dataset (see the main script for more information).
+ features: The processed dataset (see the main script for more information).
+ predictions (:obj:`Tuple[np.ndarray, np.ndarray]`):
+ The predictions of the model: two arrays containing the start logits and the end logits respectively. Its
+ first dimension must match the number of elements of :obj:`features`.
+ version_2_with_negative (:obj:`bool`, `optional`, defaults to :obj:`False`):
+ Whether or not the underlying dataset contains examples with no answers.
+ n_best_size (:obj:`int`, `optional`, defaults to 20):
+ The total number of n-best predictions to generate when looking for an answer.
+ max_answer_length (:obj:`int`, `optional`, defaults to 30):
+ The maximum length of an answer that can be generated. This is needed because the start and end predictions
+ are not conditioned on one another.
+ start_n_top (:obj:`int`, `optional`, defaults to 5):
+ The number of top start logits too keep when searching for the :obj:`n_best_size` predictions.
+ end_n_top (:obj:`int`, `optional`, defaults to 5):
+ The number of top end logits too keep when searching for the :obj:`n_best_size` predictions.
+ output_dir (:obj:`str`, `optional`):
+ If provided, the dictionaries of predictions, n_best predictions (with their scores and logits) and, if
+ :obj:`version_2_with_negative=True`, the dictionary of the scores differences between best and null
+ answers, are saved in `output_dir`.
+ prefix (:obj:`str`, `optional`):
+ If provided, the dictionaries mentioned above are saved with `prefix` added to their names.
+ log_level (:obj:`int`, `optional`, defaults to ``logging.WARNING``):
+ ``logging`` log level (e.g., ``logging.WARNING``)
+ """
+ assert len(predictions) == 5, "`predictions` should be a tuple with five elements."
+ start_top_log_probs, start_top_index, end_top_log_probs, end_top_index, cls_logits = predictions
+
+ assert len(predictions[0]) == len(
+ features
+ ), f"Got {len(predictions[0])} predicitions and {len(features)} features."
+
+ # Build a map example to its corresponding features.
+ example_id_to_index = {k: i for i, k in enumerate(examples["id"])}
+ features_per_example = collections.defaultdict(list)
+ for i, feature in enumerate(features):
+ features_per_example[example_id_to_index[feature["example_id"]]].append(i)
+
+ # The dictionaries we have to fill.
+ all_predictions = collections.OrderedDict()
+ all_nbest_json = collections.OrderedDict()
+ scores_diff_json = collections.OrderedDict() if version_2_with_negative else None
+
+ # Logging.
+ logger.setLevel(log_level)
+ logger.info(f"Post-processing {len(examples)} example predictions split into {len(features)} features.")
+
+ # Let's loop over all the examples!
+ for example_index, example in enumerate(tqdm(examples)):
+ # Those are the indices of the features associated to the current example.
+ feature_indices = features_per_example[example_index]
+
+ min_null_score = None
+ prelim_predictions = []
+
+ # Looping through all the features associated to the current example.
+ for feature_index in feature_indices:
+ # We grab the predictions of the model for this feature.
+ start_log_prob = start_top_log_probs[feature_index]
+ start_indexes = start_top_index[feature_index]
+ end_log_prob = end_top_log_probs[feature_index]
+ end_indexes = end_top_index[feature_index]
+ feature_null_score = cls_logits[feature_index]
+ # This is what will allow us to map some the positions in our logits to span of texts in the original
+ # context.
+ offset_mapping = features[feature_index]["offset_mapping"]
+ # Optional `token_is_max_context`, if provided we will remove answers that do not have the maximum context
+ # available in the current feature.
+ token_is_max_context = features[feature_index].get("token_is_max_context", None)
+
+ # Update minimum null prediction
+ if min_null_score is None or feature_null_score < min_null_score:
+ min_null_score = feature_null_score
+
+ # Go through all possibilities for the `n_start_top`/`n_end_top` greater start and end logits.
+ for i in range(start_n_top):
+ for j in range(end_n_top):
+ start_index = int(start_indexes[i])
+ j_index = i * end_n_top + j
+ end_index = int(end_indexes[j_index])
+ # Don't consider out-of-scope answers (last part of the test should be unnecessary because of the
+ # p_mask but let's not take any risk)
+ if (
+ start_index >= len(offset_mapping)
+ or end_index >= len(offset_mapping)
+ or offset_mapping[start_index] is None
+ or offset_mapping[end_index] is None
+ ):
+ continue
+ # Don't consider answers with a length negative or > max_answer_length.
+ if end_index < start_index or end_index - start_index + 1 > max_answer_length:
+ continue
+ # Don't consider answer that don't have the maximum context available (if such information is
+ # provided).
+ if token_is_max_context is not None and not token_is_max_context.get(str(start_index), False):
+ continue
+ prelim_predictions.append(
+ {
+ "offsets": (offset_mapping[start_index][0], offset_mapping[end_index][1]),
+ "score": start_log_prob[i] + end_log_prob[j_index],
+ "start_log_prob": start_log_prob[i],
+ "end_log_prob": end_log_prob[j_index],
+ }
+ )
+
+ # Only keep the best `n_best_size` predictions.
+ predictions = sorted(prelim_predictions, key=lambda x: x["score"], reverse=True)[:n_best_size]
+
+ # Use the offsets to gather the answer text in the original context.
+ context = example["context"]
+ for pred in predictions:
+ offsets = pred.pop("offsets")
+ pred["text"] = context[offsets[0] : offsets[1]]
+
+ # In the very rare edge case we have not a single non-null prediction, we create a fake prediction to avoid
+ # failure.
+ if len(predictions) == 0:
+ predictions.insert(0, {"text": "", "start_logit": -1e-6, "end_logit": -1e-6, "score": -2e-6})
+
+ # Compute the softmax of all scores (we do it with numpy to stay independent from torch/tf in this file, using
+ # the LogSumExp trick).
+ scores = np.array([pred.pop("score") for pred in predictions])
+ exp_scores = np.exp(scores - np.max(scores))
+ probs = exp_scores / exp_scores.sum()
+
+ # Include the probabilities in our predictions.
+ for prob, pred in zip(probs, predictions):
+ pred["probability"] = prob
+
+ # Pick the best prediction and set the probability for the null answer.
+ all_predictions[example["id"]] = predictions[0]["text"]
+ if version_2_with_negative:
+ scores_diff_json[example["id"]] = float(min_null_score)
+
+ # Make `predictions` JSON-serializable by casting np.float back to float.
+ all_nbest_json[example["id"]] = [
+ {k: (float(v) if isinstance(v, (np.float16, np.float32, np.float64)) else v) for k, v in pred.items()}
+ for pred in predictions
+ ]
+
+ # If we have an output_dir, let's save all those dicts.
+ if output_dir is not None:
+ assert os.path.isdir(output_dir), f"{output_dir} is not a directory."
+
+ prediction_file = os.path.join(
+ output_dir, "predictions.json" if prefix is None else f"{prefix}_predictions.json"
+ )
+ nbest_file = os.path.join(
+ output_dir, "nbest_predictions.json" if prefix is None else f"{prefix}_nbest_predictions.json"
+ )
+ if version_2_with_negative:
+ null_odds_file = os.path.join(
+ output_dir, "null_odds.json" if prefix is None else f"{prefix}_null_odds.json"
+ )
+
+ logger.info(f"Saving predictions to {prediction_file}.")
+ with open(prediction_file, "w") as writer:
+ writer.write(json.dumps(all_predictions, indent=4) + "\n")
+ logger.info(f"Saving nbest_preds to {nbest_file}.")
+ with open(nbest_file, "w") as writer:
+ writer.write(json.dumps(all_nbest_json, indent=4) + "\n")
+ if version_2_with_negative:
+ logger.info(f"Saving null_odds to {null_odds_file}.")
+ with open(null_odds_file, "w") as writer:
+ writer.write(json.dumps(scores_diff_json, indent=4) + "\n")
+
+ return all_predictions, scores_diff_json
\ No newline at end of file
diff --git a/tasks/srl/dataset.py b/tasks/srl/dataset.py
new file mode 100644
index 0000000..e99a7d0
--- /dev/null
+++ b/tasks/srl/dataset.py
@@ -0,0 +1,179 @@
+from typing import OrderedDict
+import torch
+from torch.utils import data
+from torch.utils.data import Dataset
+from datasets.arrow_dataset import Dataset as HFDataset
+from datasets.load import load_dataset, load_metric
+from transformers import AutoTokenizer, DataCollatorForTokenClassification, AutoConfig
+import numpy as np
+from typing import Optional
+
+# add by wjn
+def random_sampling(raw_datasets: load_dataset, data_type: str="train", num_examples_per_label: Optional[int]=16):
+ assert data_type in ["train", "dev", "test"]
+ label_list = raw_datasets[data_type]["label"] # [0, 1, 0, 0, ...]
+ label_dict = dict()
+ # 记录每个label对应的样本索引
+ for ei, label in enumerate(label_list):
+ if label not in label_dict.keys():
+ label_dict[label] = list()
+ label_dict[label].append(ei)
+ # 对于每个类别,随机采样k个样本
+ few_example_ids = list()
+ for label, eid_list in label_dict.items():
+ # examples = deepcopy(eid_list)
+ # shuffle(examples)
+ idxs = np.random.choice(len(eid_list), size=num_examples_per_label, replace=False)
+ selected_eids = [eid_list[i] for i in idxs]
+ few_example_ids.extend(selected_eids)
+ return few_example_ids
+
+
+class SRLDataset(Dataset):
+ def __init__(self, tokenizer: AutoTokenizer, data_args, training_args) -> None:
+ super().__init__()
+ raw_datasets = load_dataset(f'tasks/srl/datasets/{data_args.dataset_name}.py')
+ self.tokenizer = tokenizer
+
+ if training_args.do_train:
+ column_names = raw_datasets["train"].column_names
+ features = raw_datasets["train"].features
+ else:
+ column_names = raw_datasets["validation"].column_names
+ features = raw_datasets["validation"].features
+
+ self.label_column_name = f"tags"
+ self.label_list = features[self.label_column_name].feature.names
+ self.label_to_id = {l: i for i, l in enumerate(self.label_list)}
+ self.num_labels = len(self.label_list)
+
+ if training_args.do_train:
+ train_dataset = raw_datasets['train']
+ if data_args.max_train_samples is not None:
+ train_dataset = train_dataset.select(range(data_args.max_train_samples))
+ self.train_dataset = train_dataset.map(
+ self.tokenize_and_align_labels,
+ batched=True,
+ load_from_cache_file=True,
+ desc="Running tokenizer on train dataset",
+ )
+ if training_args.do_eval:
+ eval_dataset = raw_datasets['validation']
+ if data_args.max_eval_samples is not None:
+ eval_dataset = eval_dataset.select(range(data_args.max_eval_samples))
+ self.eval_dataset = eval_dataset.map(
+ self.tokenize_and_align_labels,
+ batched=True,
+ load_from_cache_file=True,
+ desc="Running tokenizer on validation dataset",
+ )
+
+ if training_args.do_predict:
+ if data_args.dataset_name == "conll2005":
+ self.predict_dataset = OrderedDict()
+ self.predict_dataset['wsj'] = raw_datasets['test_wsj'].map(
+ self.tokenize_and_align_labels,
+ batched=True,
+ load_from_cache_file=True,
+ desc="Running tokenizer on WSJ test dataset",
+ )
+
+ self.predict_dataset['brown'] = raw_datasets['test_brown'].map(
+ self.tokenize_and_align_labels,
+ batched=True,
+ load_from_cache_file=True,
+ desc="Running tokenizer on Brown test dataset",
+ )
+ else:
+ self.predict_dataset = raw_datasets['test_wsj'].map(
+ self.tokenize_and_align_labels,
+ batched=True,
+ load_from_cache_file=True,
+ desc="Running tokenizer on WSJ test dataset",
+ )
+
+ # add by wjn
+ # 随机采样few-shot training / dev data(传入label_list,对每个label进行采样,最后得到索引列表)
+ if data_args.num_examples_per_label is not None:
+ train_examples_idx_list = random_sampling(
+ raw_datasets=raw_datasets,
+ data_type="train",
+ num_examples_per_label=data_args.num_examples_per_label
+ )
+ self.train_dataset = self.train_dataset.select(train_examples_idx_list)
+ print("Randomly sampling {}-shot training examples for each label. Total examples number is {}".format(
+ data_args.num_examples_per_label,
+ len(self.train_dataset)
+ ))
+
+ self.data_collator = DataCollatorForTokenClassification(self.tokenizer, pad_to_multiple_of=8 if training_args.fp16 else None)
+ self.metric = load_metric("seqeval")
+
+
+ def compute_metrics(self, p):
+ predictions, labels = p
+ predictions = np.argmax(predictions, axis=2)
+
+ # Remove ignored index (special tokens)
+ true_predictions = [
+ [self.label_list[p] for (p, l) in zip(prediction, label) if l != -100]
+ for prediction, label in zip(predictions, labels)
+ ]
+ true_labels = [
+ [self.label_list[l] for (p, l) in zip(prediction, label) if l != -100]
+ for prediction, label in zip(predictions, labels)
+ ]
+
+ results = self.metric.compute(predictions=true_predictions, references=true_labels)
+ return {
+ "precision": results["overall_precision"],
+ "recall": results["overall_recall"],
+ "f1": results["overall_f1"],
+ "accuracy": results["overall_accuracy"],
+ }
+
+ def tokenize_and_align_labels(self, examples):
+ for i, tokens in enumerate(examples['tokens']):
+ examples['tokens'][i] = tokens + ["[SEP]"] + [tokens[int(examples['index'][i])]]
+
+ tokenized_inputs = self.tokenizer(
+ examples['tokens'],
+ padding=False,
+ truncation=True,
+ # We use this argument because the texts in our dataset are lists of words (with a label for each word).
+ is_split_into_words=True,
+ )
+
+ # print(tokenized_inputs['input_ids'][0])
+
+ labels = []
+ for i, label in enumerate(examples['tags']):
+ word_ids = [None]
+ for j, word in enumerate(examples['tokens'][i][:-2]):
+ token = self.tokenizer.encode(word, add_special_tokens=False)
+ word_ids += [j] * len(token)
+ word_ids += [None]
+ verb = examples['tokens'][i][int(examples['index'][i])]
+ word_ids += [None] * len(self.tokenizer.encode(verb, add_special_tokens=False))
+ word_ids += [None]
+
+ # word_ids = tokenized_inputs.word_ids(batch_index=i)
+ previous_word_idx = None
+ label_ids = []
+ for word_idx in word_ids:
+ # Special tokens have a word id that is None. We set the label to -100 so they are automatically
+ # ignored in the loss function.
+ if word_idx is None:
+ label_ids.append(-100)
+ # We set the label for the first token of each word.
+ elif word_idx != previous_word_idx:
+ label_ids.append(label[word_idx])
+ # For the other tokens in a word, we set the label to either the current label or -100, depending on
+ # the label_all_tokens flag.
+ else:
+ label_ids.append(-100)
+ previous_word_idx = word_idx
+
+ labels.append(label_ids)
+ tokenized_inputs["labels"] = labels
+ return tokenized_inputs
\ No newline at end of file
diff --git a/tasks/srl/datasets/conll2005.py b/tasks/srl/datasets/conll2005.py
new file mode 100644
index 0000000..3304d67
--- /dev/null
+++ b/tasks/srl/datasets/conll2005.py
@@ -0,0 +1,131 @@
+# coding=utf-8
+# Copyright 2020 HuggingFace Datasets Authors.
+#
+# Licensed under the Apache License, Version 2.0 (the "License");
+# you may not use this file except in compliance with the License.
+# You may obtain a copy of the License at
+#
+# http://www.apache.org/licenses/LICENSE-2.0
+#
+# Unless required by applicable law or agreed to in writing, software
+# distributed under the License is distributed on an "AS IS" BASIS,
+# WITHOUT WARRANTIES OR CONDITIONS OF ANY KIND, either express or implied.
+# See the License for the specific language governing permissions and
+# limitations under the License.
+
+# Lint as: python3
+"""Introduction to the CoNLL-2003 Shared Task: Language-Independent Named Entity Recognition"""
+
+import datasets
+
+
+logger = datasets.logging.get_logger(__name__)
+
+
+_CITATION = """\
+@inproceedings{tjong-kim-sang-de-meulder-2003-introduction,
+ title = "Introduction to the {C}o{NLL}-2003 Shared Task: Language-Independent Named Entity Recognition",
+ author = "Tjong Kim Sang, Erik F. and
+ De Meulder, Fien",
+ booktitle = "Proceedings of the Seventh Conference on Natural Language Learning at {HLT}-{NAACL} 2003",
+ year = "2003",
+ url = "https://www.aclweb.org/anthology/W03-0419",
+ pages = "142--147",
+}
+"""
+
+_DESCRIPTION = """\
+The shared task of CoNLL-2003 concerns language-independent named entity recognition. We will concentrate on
+four types of named entities: persons, locations, organizations and names of miscellaneous entities that do
+not belong to the previous three groups.
+The CoNLL-2003 shared task data files contain four columns separated by a single space. Each word has been put on
+a separate line and there is an empty line after each sentence. The first item on each line is a word, the second
+a part-of-speech (POS) tag, the third a syntactic chunk tag and the fourth the named entity tag. The chunk tags
+and the named entity tags have the format I-TYPE which means that the word is inside a phrase of type TYPE. Only
+if two phrases of the same type immediately follow each other, the first word of the second phrase will have tag
+B-TYPE to show that it starts a new phrase. A word with tag O is not part of a phrase. Note the dataset uses IOB2
+tagging scheme, whereas the original dataset uses IOB1.
+For more details see https://www.clips.uantwerpen.be/conll2003/ner/ and https://www.aclweb.org/anthology/W03-0419
+"""
+
+_URL = "../../../data/CoNLL05/"
+_TRAINING_FILE = "conll05.train.txt"
+_DEV_FILE = "conll05.devel.txt"
+_TEST_WSJ_FILE = "conll05.test.wsj.txt"
+_TEST_BROWN_FILE = "conll05.test.brown.txt"
+
+
+class Conll2005Config(datasets.BuilderConfig):
+ """BuilderConfig for Conll2003"""
+
+ def __init__(self, **kwargs):
+ """BuilderConfig forConll2005.
+ Args:
+ **kwargs: keyword arguments forwarded to super.
+ """
+ super(Conll2005Config, self).__init__(**kwargs)
+
+
+class Conll2005(datasets.GeneratorBasedBuilder):
+ """Conll2003 dataset."""
+
+ BUILDER_CONFIGS = [
+ Conll2005Config(name="conll2005", version=datasets.Version("1.0.0"), description="Conll2005 dataset"),
+ ]
+
+ def _info(self):
+ return datasets.DatasetInfo(
+ description=_DESCRIPTION,
+ features=datasets.Features(
+ {
+ "id": datasets.Value("string"),
+ "index": datasets.Value("string"),
+ "tokens": datasets.Sequence(datasets.Value("string")),
+ "tags": datasets.Sequence(
+ datasets.features.ClassLabel(
+ names=['B-C-AM-TMP', 'B-C-AM-DIR', 'B-C-A2', 'B-R-AM-EXT', 'B-C-A0', 'I-AM-NEG', 'I-AM-ADV', 'B-C-V', 'B-C-AM-MNR', 'B-R-A3', 'I-AM-TM', 'B-V', 'B-R-A4', 'B-A5', 'I-A4', 'I-R-AM-LOC', 'I-C-A1', 'B-R-AA', 'I-C-A0', 'B-C-AM-EXT', 'I-C-AM-DIS', 'I-C-A5', 'B-A0', 'B-C-A4', 'B-C-AM-CAU', 'B-C-AM-NEG', 'B-AM-NEG', 'I-AM-MNR', 'I-R-A2', 'I-R-AM-TMP', 'B-AM', 'I-R-AM-PNC', 'B-AM-LOC', 'B-AM-REC', 'B-A2', 'I-AM-EXT', 'I-V', 'B-A3', 'B-A4', 'B-R-A0', 'I-AM-MOD', 'I-C-AM-CAU', 'B-R-AM-CAU', 'B-A1', 'B-R-AM-TMP', 'I-R-AM-EXT', 'B-C-AM-ADV', 'B-AM-ADV', 'B-R-A2', 'B-AM-CAU', 'B-R-AM-DIR', 'I-A5', 'B-C-AM-DIS', 'I-C-AM-MNR', 'B-AM-PNC', 'I-C-AM-LOC', 'I-R-A3', 'I-R-AM-ADV', 'I-A0', 'B-AM-EXT', 'B-R-AM-PNC', 'I-AM-DIS', 'I-AM-REC', 'B-C-AM-LOC', 'B-R-AM-ADV', 'I-AM', 'I-AM-CAU', 'I-AM-TMP', 'I-A1', 'I-C-A4', 'B-R-AM-LOC', 'I-C-A2', 'B-C-A5', 'O', 'B-R-AM-MNR', 'I-C-A3', 'I-R-AM-DIR', 'I-AM-PRD', 'B-AM-TM', 'I-A2', 'I-AA', 'I-AM-LOC', 'I-AM-PNC', 'B-AM-MOD', 'B-AM-DIR', 'B-R-A1', 'B-AM-TMP', 'B-AM-MNR', 'I-R-A0', 'B-AM-PRD', 'I-AM-DIR', 'B-AM-DIS', 'I-C-AM-ADV', 'I-R-A1', 'B-C-A3', 'I-R-AM-MNR', 'I-R-A4', 'I-C-AM-PNC', 'I-C-AM-TMP', 'I-C-V', 'I-A3', 'I-C-AM-EXT', 'B-C-A1', 'B-AA', 'I-C-AM-DIR', 'B-C-AM-PNC']
+ )
+ ),
+ }
+ ),
+ supervised_keys=None,
+ homepage="https://www.aclweb.org/anthology/W03-0419/",
+ citation=_CITATION,
+ )
+
+ def _split_generators(self, dl_manager):
+ """Returns SplitGenerators."""
+ urls_to_download = {
+ "train": f"{_URL}{_TRAINING_FILE}",
+ "dev": f"{_URL}{_DEV_FILE}",
+ "test_wsj": f"{_URL}{_TEST_WSJ_FILE}",
+ "test_brown": f"{_URL}{_TEST_BROWN_FILE}"
+ }
+ downloaded_files = dl_manager.download_and_extract(urls_to_download)
+
+ return [
+ datasets.SplitGenerator(name="train", gen_kwargs={"filepath": downloaded_files["train"]}),
+ datasets.SplitGenerator(name="validation", gen_kwargs={"filepath": downloaded_files["dev"]}),
+ datasets.SplitGenerator(name="test_wsj", gen_kwargs={"filepath": downloaded_files["test_wsj"]}),
+ datasets.SplitGenerator(name="test_brown", gen_kwargs={"filepath": downloaded_files["test_brown"]}),
+ ]
+
+ def _generate_examples(self, filepath):
+ logger.info("⏳ Generating examples from = %s", filepath)
+ with open(filepath, encoding="utf-8") as f:
+ guid = 0
+ for line in f:
+ if line != '':
+ index = line.split()[0]
+
+ text = ' '.join(line.split()[1:]).strip()
+ tokens = text.split("|||")[0].split()
+ labels = text.split("|||")[1].split()
+ yield guid, {
+ "id": str(guid),
+ "index": index,
+ "tokens": tokens,
+ "tags": labels
+ }
+
+ guid += 1
\ No newline at end of file
diff --git a/tasks/srl/datasets/conll2012.py b/tasks/srl/datasets/conll2012.py
new file mode 100644
index 0000000..2ac51f9
--- /dev/null
+++ b/tasks/srl/datasets/conll2012.py
@@ -0,0 +1,152 @@
+# coding=utf-8
+# Copyright 2020 HuggingFace Datasets Authors.
+#
+# Licensed under the Apache License, Version 2.0 (the "License");
+# you may not use this file except in compliance with the License.
+# You may obtain a copy of the License at
+#
+# http://www.apache.org/licenses/LICENSE-2.0
+#
+# Unless required by applicable law or agreed to in writing, software
+# distributed under the License is distributed on an "AS IS" BASIS,
+# WITHOUT WARRANTIES OR CONDITIONS OF ANY KIND, either express or implied.
+# See the License for the specific language governing permissions and
+# limitations under the License.
+
+# Lint as: python3
+"""Introduction to the CoNLL-2003 Shared Task: Language-Independent Named Entity Recognition"""
+
+import datasets
+
+
+logger = datasets.logging.get_logger(__name__)
+
+
+_CITATION = """\
+@inproceedings{tjong-kim-sang-de-meulder-2003-introduction,
+ title = "Introduction to the {C}o{NLL}-2003 Shared Task: Language-Independent Named Entity Recognition",
+ author = "Tjong Kim Sang, Erik F. and
+ De Meulder, Fien",
+ booktitle = "Proceedings of the Seventh Conference on Natural Language Learning at {HLT}-{NAACL} 2003",
+ year = "2003",
+ url = "https://www.aclweb.org/anthology/W03-0419",
+ pages = "142--147",
+}
+"""
+
+_DESCRIPTION = """\
+The shared task of CoNLL-2003 concerns language-independent named entity recognition. We will concentrate on
+four types of named entities: persons, locations, organizations and names of miscellaneous entities that do
+not belong to the previous three groups.
+The CoNLL-2003 shared task data files contain four columns separated by a single space. Each word has been put on
+a separate line and there is an empty line after each sentence. The first item on each line is a word, the second
+a part-of-speech (POS) tag, the third a syntactic chunk tag and the fourth the named entity tag. The chunk tags
+and the named entity tags have the format I-TYPE which means that the word is inside a phrase of type TYPE. Only
+if two phrases of the same type immediately follow each other, the first word of the second phrase will have tag
+B-TYPE to show that it starts a new phrase. A word with tag O is not part of a phrase. Note the dataset uses IOB2
+tagging scheme, whereas the original dataset uses IOB1.
+For more details see https://www.clips.uantwerpen.be/conll2003/ner/ and https://www.aclweb.org/anthology/W03-0419
+"""
+
+_URL = "../../../data/CoNLL12/"
+_TRAINING_FILE = "conll2012.train.txt"
+_DEV_FILE = "conll2012.devel.txt"
+_TEST_WSJ_FILE = "conll2012.test.txt"
+# _TEST_BROWN_FILE = "conll.test.brown.txt"
+CONLL12_LABELS = ['B-ARG0', 'B-ARGM-MNR', 'B-V', 'B-ARG1', 'B-ARG2', 'I-ARG2', 'O', 'I-ARG1', 'B-ARGM-ADV',
+ 'B-ARGM-LOC', 'I-ARGM-LOC', 'I-ARG0', 'B-ARGM-TMP', 'I-ARGM-TMP', 'B-ARGM-PRP',
+ 'I-ARGM-PRP', 'B-ARGM-PRD', 'I-ARGM-PRD', 'B-R-ARGM-TMP', 'B-ARGM-DIR', 'I-ARGM-DIR',
+ 'B-ARGM-DIS', 'B-ARGM-MOD', 'I-ARGM-ADV', 'I-ARGM-DIS', 'B-R-ARGM-LOC', 'B-ARG4',
+ 'I-ARG4', 'B-R-ARG1', 'B-R-ARG0', 'I-R-ARG0', 'B-ARG3', 'B-ARGM-NEG', 'B-ARGM-CAU',
+ 'I-ARGM-MNR', 'I-R-ARG1', 'B-C-ARG1', 'I-C-ARG1', 'B-ARGM-EXT', 'I-ARGM-EXT', 'I-ARGM-CAU',
+ 'I-ARG3', 'B-C-ARGM-ADV', 'I-C-ARGM-ADV', 'B-ARGM-LVB', 'B-ARGM-REC', 'B-R-ARG3',
+ 'B-R-ARG2', 'B-C-ARG0', 'I-C-ARG0', 'B-ARGM-ADJ', 'B-C-ARG2', 'I-C-ARG2', 'B-R-ARGM-CAU',
+ 'B-R-ARGM-DIR', 'B-ARGM-GOL', 'I-ARGM-GOL', 'B-ARGM-DSP', 'I-ARGM-ADJ', 'I-R-ARG2',
+ 'I-ARGM-NEG', 'B-ARGM-PRR', 'B-R-ARGM-ADV', 'I-R-ARGM-ADV', 'I-R-ARGM-LOC', 'B-ARGA',
+ 'B-R-ARGM-MNR', 'I-R-ARGM-MNR', 'B-ARGM-COM', 'I-ARGM-COM', 'B-ARGM-PRX', 'I-ARGM-REC',
+ 'B-R-ARG4', 'B-C-ARGM-LOC', 'I-C-ARGM-LOC', 'I-R-ARGM-DIR', 'I-ARGA', 'B-C-ARGM-TMP',
+ 'I-C-ARGM-TMP', 'B-C-ARGM-CAU', 'I-C-ARGM-CAU', 'B-R-ARGM-PRD', 'I-R-ARGM-PRD',
+ 'I-R-ARG3', 'B-C-ARG4', 'I-C-ARG4', 'B-ARGM-PNC', 'I-ARGM-PNC', 'B-ARG5', 'I-ARG5',
+ 'B-C-ARGM-PRP', 'I-C-ARGM-PRP', 'B-C-ARGM-MNR', 'I-C-ARGM-MNR', 'I-R-ARGM-TMP',
+ 'B-R-ARG5', 'I-ARGM-DSP', 'B-C-ARGM-DSP', 'I-C-ARGM-DSP', 'B-C-ARG3', 'I-C-ARG3',
+ 'B-R-ARGM-COM', 'I-R-ARGM-COM', 'B-R-ARGM-PRP', 'I-R-ARGM-PRP', 'I-R-ARGM-CAU',
+ 'B-R-ARGM-GOL', 'I-R-ARGM-GOL', 'B-R-ARGM-EXT', 'I-R-ARGM-EXT', 'I-R-ARG4',
+ 'B-C-ARGM-EXT', 'I-C-ARGM-EXT', 'I-ARGM-MOD', 'B-C-ARGM-MOD', 'I-C-ARGM-MOD']
+
+
+class Conll2005Config(datasets.BuilderConfig):
+ """BuilderConfig for Conll2003"""
+
+ def __init__(self, **kwargs):
+ """BuilderConfig forConll2005.
+ Args:
+ **kwargs: keyword arguments forwarded to super.
+ """
+ super(Conll2005Config, self).__init__(**kwargs)
+
+
+class Conll2005(datasets.GeneratorBasedBuilder):
+ """Conll2003 dataset."""
+
+ BUILDER_CONFIGS = [
+ Conll2005Config(name="conll2012", version=datasets.Version("1.0.0"), description="Conll2012 dataset"),
+ ]
+
+ def _info(self):
+ return datasets.DatasetInfo(
+ description=_DESCRIPTION,
+ features=datasets.Features(
+ {
+ "id": datasets.Value("string"),
+ "index": datasets.Value("string"),
+ "tokens": datasets.Sequence(datasets.Value("string")),
+ "tags": datasets.Sequence(
+ datasets.features.ClassLabel(
+ names=CONLL12_LABELS
+ )
+ ),
+ }
+ ),
+ supervised_keys=None,
+ homepage="https://www.aclweb.org/anthology/W03-0419/",
+ citation=_CITATION,
+ )
+
+ def _split_generators(self, dl_manager):
+ """Returns SplitGenerators."""
+ urls_to_download = {
+ "train": f"{_URL}{_TRAINING_FILE}",
+ "dev": f"{_URL}{_DEV_FILE}",
+ "test_wsj": f"{_URL}{_TEST_WSJ_FILE}",
+ # "test_brown": f"{_URL}{_TEST_BROWN_FILE}"
+ }
+ downloaded_files = dl_manager.download_and_extract(urls_to_download)
+
+ return [
+ datasets.SplitGenerator(name="train", gen_kwargs={"filepath": downloaded_files["train"]}),
+ datasets.SplitGenerator(name="validation", gen_kwargs={"filepath": downloaded_files["dev"]}),
+ datasets.SplitGenerator(name="test_wsj", gen_kwargs={"filepath": downloaded_files["test_wsj"]}),
+ # datasets.SplitGenerator(name="test_brown", gen_kwargs={"filepath": downloaded_files["test_brown"]}),
+ ]
+
+ def _generate_examples(self, filepath):
+ logger.info("⏳ Generating examples from = %s", filepath)
+ with open(filepath, encoding="utf-8") as f:
+ guid = 0
+ for line in f:
+ if line != '':
+ if line.split() == []:
+ continue
+ index = line.split()[0]
+
+ text = ' '.join(line.split()[1:]).strip()
+ tokens = text.split("|||")[0].split()
+ labels = text.split("|||")[1].split()
+ yield guid, {
+ "id": str(guid),
+ "index": index,
+ "tokens": tokens,
+ "tags": labels
+ }
+
+ guid += 1
\ No newline at end of file
diff --git a/tasks/srl/get_trainer.py b/tasks/srl/get_trainer.py
new file mode 100644
index 0000000..e6c1747
--- /dev/null
+++ b/tasks/srl/get_trainer.py
@@ -0,0 +1,61 @@
+import logging
+import os
+import random
+import sys
+
+from transformers import (
+ AutoConfig,
+ AutoTokenizer,
+)
+
+from tasks.srl.dataset import SRLDataset
+from training.trainer_exp import ExponentialTrainer
+from model.utils import get_model, TaskType
+from tasks.utils import ADD_PREFIX_SPACE, USE_FAST
+
+logger = logging.getLogger(__name__)
+
+def get_trainer(args):
+ model_args, data_args, training_args, _ = args
+
+ model_type = AutoConfig.from_pretrained(model_args.model_name_or_path).model_type
+
+ add_prefix_space = ADD_PREFIX_SPACE[model_type]
+
+ use_fast = USE_FAST[model_type]
+
+ tokenizer = AutoTokenizer.from_pretrained(
+ model_args.model_name_or_path,
+ use_fast=use_fast,
+ revision=model_args.model_revision,
+ add_prefix_space=add_prefix_space,
+ )
+
+ dataset = SRLDataset(tokenizer, data_args, training_args)
+
+ config = AutoConfig.from_pretrained(
+ model_args.model_name_or_path,
+ num_labels=dataset.num_labels,
+ revision=model_args.model_revision,
+ )
+
+ if training_args.do_train:
+ for index in random.sample(range(len(dataset.train_dataset)), 3):
+ logger.info(f"Sample {index} of the training set: {dataset.train_dataset[index]}.")
+
+ model = get_model(data_args, model_args, TaskType.TOKEN_CLASSIFICATION, config, fix_bert=False)
+
+
+ trainer = ExponentialTrainer(
+ model=model,
+ args=training_args,
+ train_dataset=dataset.train_dataset if training_args.do_train else None,
+ eval_dataset=dataset.eval_dataset if training_args.do_eval else None,
+ predict_dataset=dataset.predict_dataset if training_args.do_predict else None,
+ tokenizer=tokenizer,
+ data_collator=dataset.data_collator,
+ compute_metrics=dataset.compute_metrics,
+ test_key="f1"
+ )
+
+ return trainer, dataset.predict_dataset
\ No newline at end of file
diff --git a/tasks/superglue/dataset.py b/tasks/superglue/dataset.py
new file mode 100644
index 0000000..6fc5876
--- /dev/null
+++ b/tasks/superglue/dataset.py
@@ -0,0 +1,423 @@
+from datasets.load import load_dataset, load_metric
+from transformers import (
+ AutoTokenizer,
+ DataCollatorWithPadding,
+ EvalPrediction,
+ default_data_collator,
+)
+import numpy as np
+import logging
+from collections import defaultdict
+from typing import Optional
+
+# add by wjn
+def random_sampling(raw_datasets: load_dataset, data_type: str="train", num_examples_per_label: Optional[int]=16):
+ assert data_type in ["train", "validation", "test"]
+ label_list = raw_datasets[data_type]["label"] # [0, 1, 0, 0, ...]
+ label_dict = dict()
+ # 记录每个label对应的样本索引
+ for ei, label in enumerate(label_list):
+ if label not in label_dict.keys():
+ label_dict[label] = list()
+ label_dict[label].append(ei)
+ # 对于每个类别,随机采样k个样本
+ few_example_ids = list()
+ for label, eid_list in label_dict.items():
+ # examples = deepcopy(eid_list)
+ # shuffle(examples)
+ idxs = np.random.choice(len(eid_list), size=num_examples_per_label, replace=False)
+ selected_eids = [eid_list[i] for i in idxs]
+ few_example_ids.extend(selected_eids)
+ # 保存没有被选中的example id
+ num_examples = len(label_list)
+ un_selected_examples_ids = [idx for idx in range(num_examples) if idx not in few_example_ids]
+ return few_example_ids, un_selected_examples_ids
+
+task_to_test_key = {
+ "boolq": "accuracy",
+ "cb": "accuracy",
+ "rte": "accuracy",
+ "wic": "accuracy",
+ "wsc": "accuracy",
+ "copa": "accuracy",
+ "record": "f1",
+ "multirc": "f1"
+}
+
+task_to_keys = {
+ "boolq": ("question", "passage"),
+ "cb": ("premise", "hypothesis"),
+ "rte": ("premise", "hypothesis"),
+ "wic": ("processed_sentence1", None),
+ "wsc": ("span2_word_text", "span1_text"),
+ "copa": (None, None),
+ "record": (None, None),
+ "multirc": ("paragraph", "question_answer")
+}
+
+task_to_template = {
+ "boolq" : [{"prefix_template": "Question: ", "suffix_template": ""}, {"prefix_template": "Passage: ", "suffix_template": " Answer: ."}],
+ "cb": [None, {"prefix_template": "? ,", "suffix_template": ""}],
+ "rte": [None, {"prefix_template": "? ,", "suffix_template": ""}], # prefix / suffix template in each segment.
+ # "wic": [None, None],
+ # "wsc": [None, None],
+ # "copa": [None, None],
+ # "record": [None, None],
+ # "multirc": [None, None],
+}
+
+# add by wjn
+label_words_mapping = {
+ "boolq": {"True": ["Yes"], "False": ["No"]},
+ "cb": {"contradiction": ["No"], "neutral": ["Maybe"], "entailment": ["Yes"]},
+ "rte": {"not_entailment": ["No"], "entailment": ["Yes"]}
+}
+
+
+logger = logging.getLogger(__name__)
+
+
+class SuperGlueDataset():
+ def __init__(
+ self,
+ tokenizer: AutoTokenizer,
+ data_args,
+ training_args,
+ semi_training_args=None,
+ use_prompt=False
+ ) -> None:
+ super().__init__()
+ raw_datasets = load_dataset("super_glue", data_args.dataset_name)
+ self.tokenizer = tokenizer
+ self.data_args = data_args
+
+ self.multiple_choice = data_args.dataset_name in ["copa"]
+
+ if data_args.dataset_name == "record":
+ self.num_labels = 2
+ self.label_list = ["0", "1"]
+ elif not self.multiple_choice:
+ self.label_list = raw_datasets["train"].features["label"].names
+ self.num_labels = len(self.label_list)
+ else:
+ self.num_labels = 1
+
+ # === generate template ===== add by wjn
+ self.use_prompt = False
+ if data_args.dataset_name in task_to_template.keys():
+ self.use_prompt = use_prompt
+
+ if self.use_prompt:
+ if 't5' in type(tokenizer).__name__.lower():
+ self.special_token_mapping = {
+ 'cls': 3, 'mask': 32099, 'sep': tokenizer.eos_token_id,
+ 'sep+': tokenizer.eos_token_id,
+ 'pseudo_token': tokenizer.unk_token_id
+ }
+ else:
+ self.special_token_mapping = {
+ 'cls': tokenizer.cls_token_id, 'mask': tokenizer.mask_token_id, 'sep': tokenizer.sep_token_id,
+ 'sep+': tokenizer.sep_token_id,
+ 'pseudo_token': tokenizer.unk_token_id
+ }
+ self.template = task_to_template[data_args.dataset_name] # dict
+
+
+ # === generate label word mapping ===== add by wjn
+ if self.use_prompt:
+ assert data_args.dataset_name in label_words_mapping.keys(), "You must define label word mapping for the task {}".format(data_args.dataset_name)
+ self.label_to_word = label_words_mapping[data_args.dataset_name] # e.g., {"0": ["great"], "1": [bad]}
+ self.label_to_word = {label: label_word[0] if type(label_word) == list else label_word for label, label_word in self.label_to_word.items()}
+
+ for key in self.label_to_word:
+ # For RoBERTa/BART/T5, tokenization also considers space, so we use space+word as label words.
+ if self.label_to_word[key][0] not in ['<', '[', '.', ',']:
+ # Make sure space+word is in the vocabulary
+ assert len(tokenizer.tokenize(' ' + self.label_to_word[key])) == 1
+ self.label_to_word[key] = tokenizer.convert_tokens_to_ids(tokenizer.tokenize(' ' + self.label_to_word[key])[0])
+ else:
+ self.label_to_word[key] = tokenizer.convert_tokens_to_ids(self.label_to_word[key])
+ logger.info("Label {} to word {} ({})".format(key, tokenizer._convert_id_to_token(self.label_to_word[key]), self.label_to_word[key]))
+
+ if len(self.label_list) > 1:
+ self.label_word_list = [self.label_to_word[label] for label in self.label_list]
+ else:
+ # Regression task
+ # '0' represents low polarity and '1' represents high polarity.
+ self.label_word_list = [self.label_to_word[label] for label in ['0', '1']]
+ # =============
+
+ # Preprocessing the raw_datasets
+ self.sentence1_key, self.sentence2_key = task_to_keys[data_args.dataset_name]
+
+ # Padding strategy
+ if data_args.pad_to_max_length:
+ self.padding = "max_length"
+ else:
+ # We will pad later, dynamically at batch creation, to the max sequence length in each batch
+ self.padding = False
+
+ if not self.multiple_choice:
+ self.label2id = {l: i for i, l in enumerate(self.label_list)}
+ self.id2label = {id: label for label, id in self.label2id.items()}
+ print(f"{self.label2id}")
+ print(f"{self.id2label}")
+
+ if data_args.max_seq_length > tokenizer.model_max_length:
+ logger.warning(
+ f"The max_seq_length passed ({data_args.max_seq_length}) is larger than the maximum length for the"
+ f"model ({tokenizer.model_max_length}). Using max_seq_length={tokenizer.model_max_length}."
+ )
+
+ self.max_seq_length = min(data_args.max_seq_length, tokenizer.model_max_length)
+
+ if data_args.dataset_name == "record":
+ raw_datasets = raw_datasets.map(
+ self.record_preprocess_function,
+ batched=True,
+ load_from_cache_file=not data_args.overwrite_cache,
+ remove_columns=raw_datasets["train"].column_names,
+ desc="Running tokenizer on dataset",
+ )
+ else:
+ raw_datasets = raw_datasets.map(
+ self.preprocess_function,
+ batched=True,
+ load_from_cache_file=not data_args.overwrite_cache,
+ desc="Running tokenizer on dataset",
+ )
+
+ if training_args.do_train:
+ self.train_dataset = raw_datasets["train"]
+ if data_args.max_train_samples is not None:
+ self.train_dataset = self.train_dataset.select(range(data_args.max_train_samples))
+
+ if training_args.do_eval:
+ self.eval_dataset = raw_datasets["validation"]
+ if data_args.max_eval_samples is not None:
+ self.eval_dataset = self.eval_dataset.select(range(data_args.max_eval_samples))
+
+ if training_args.do_predict or data_args.dataset_name is not None or data_args.test_file is not None:
+ self.predict_dataset = raw_datasets["test"]
+ if data_args.max_predict_samples is not None:
+ self.predict_dataset = self.predict_dataset.select(range(data_args.max_predict_samples))
+
+
+ # add by wjn
+ self.unlabeled_dataset = None
+ if semi_training_args.use_semi is True:
+ assert data_args.num_examples_per_label is not None and data_args.num_examples_per_label != -1
+
+ # 随机采样few-shot training / dev data(传入label_list,对每个label进行采样,最后得到索引列表)
+ if data_args.num_examples_per_label is not None and data_args.num_examples_per_label != -1:
+ train_examples_idx_list, un_selected_idx_list = random_sampling(
+ raw_datasets=raw_datasets,
+ data_type="train",
+ num_examples_per_label=data_args.num_examples_per_label
+ )
+ self.all_train_dataset = self.train_dataset
+ self.train_dataset = self.all_train_dataset.select(train_examples_idx_list)
+ print("Randomly sampling {}-shot training examples for each label. Total examples number is {}".format(
+ data_args.num_examples_per_label,
+ len(self.train_dataset)
+ ))
+
+ if semi_training_args.use_semi is True:
+ self.unlabeled_dataset = self.all_train_dataset.select(un_selected_idx_list)
+ print("The number of unlabeled data is {}".format(len(self.unlabeled_dataset)))
+
+ self.metric = load_metric("./metrics/super_glue", data_args.dataset_name)
+
+ if data_args.pad_to_max_length:
+ self.data_collator = default_data_collator
+ elif training_args.fp16:
+ self.data_collator = DataCollatorWithPadding(tokenizer, pad_to_multiple_of=8)
+
+ # self.test_key = "accuracy" if data_args.dataset_name not in ["record", "multirc"] else "f1"
+
+ # ==== define test key ====== add by wjn
+ self.test_key = task_to_test_key[data_args.dataset_name]
+
+ def preprocess_function(self, examples):
+
+ # print("examples[self.sentence1_key]=", examples[self.sentence1_key][0])
+
+ # WSC
+ if self.data_args.dataset_name == "wsc":
+ examples["span2_word_text"] = []
+ for text, span2_index, span2_word in zip(examples["text"], examples["span2_index"], examples["span2_text"]):
+ if self.data_args.template_id == 0:
+ examples["span2_word_text"].append(span2_word + ": " + text)
+ elif self.data_args.template_id == 1:
+ words_a = text.split()
+ words_a[span2_index] = "*" + words_a[span2_index] + "*"
+ examples["span2_word_text"].append(' '.join(words_a))
+
+ # WiC
+ if self.data_args.dataset_name == "wic":
+ examples["processed_sentence1"] = []
+ if self.data_args.template_id == 1:
+ self.sentence2_key = "processed_sentence2"
+ examples["processed_sentence2"] = []
+ for sentence1, sentence2, word, start1, end1, start2, end2 in zip(examples["sentence1"], examples["sentence2"], examples["word"], examples["start1"], examples["end1"], examples["start2"], examples["end2"]):
+ if self.data_args.template_id == 0: #ROBERTA
+ examples["processed_sentence1"].append(f"{sentence1} {sentence2} Does {word} have the same meaning in both sentences?")
+ elif self.data_args.template_id == 1: #BERT
+ examples["processed_sentence1"].append(word + ": " + sentence1)
+ examples["processed_sentence2"].append(word + ": " + sentence2)
+
+ # MultiRC
+ if self.data_args.dataset_name == "multirc":
+ examples["question_answer"] = []
+ for question, answer in zip(examples["question"], examples["answer"]):
+ examples["question_answer"].append(f"{question} {answer}")
+
+ # COPA
+ if self.data_args.dataset_name == "copa":
+ examples["text_a"] = []
+ for premise, question in zip(examples["premise"], examples["question"]):
+ joiner = "because" if question == "cause" else "so"
+ text_a = f"{premise} {joiner}"
+ examples["text_a"].append(text_a)
+
+ result1 = self.tokenizer(examples["text_a"], examples["choice1"], padding=self.padding, max_length=self.max_seq_length, truncation=True)
+ result2 = self.tokenizer(examples["text_a"], examples["choice2"], padding=self.padding, max_length=self.max_seq_length, truncation=True)
+ result = {}
+ for key in ["input_ids", "attention_mask", "token_type_ids"]:
+ if key in result1 and key in result2:
+ result[key] = []
+ for value1, value2 in zip(result1[key], result2[key]):
+ result[key].append([value1, value2])
+ return result
+
+ # add by wjn
+ # adding prompt into each example
+ if self.use_prompt:
+ # if use prompt, insert template into example
+ examples = self.prompt_preprocess_function(examples)
+
+
+ args = (
+ (examples[self.sentence1_key],) if self.sentence2_key is None else (examples[self.sentence1_key], examples[self.sentence2_key])
+ )
+ result = self.tokenizer(*args, padding=self.padding, max_length=self.max_seq_length, truncation=True)
+
+ # add by wjn
+ # adding mask pos feature
+
+ # print("self.use_prompt=", self.use_prompt)
+ if self.use_prompt:
+ mask_pos = []
+ for input_ids in result["input_ids"]:
+ mask_pos.append(input_ids.index(self.special_token_mapping["mask"]))
+ result["mask_pos"] = mask_pos
+ # print("result=", result)
+ # print("*"*100)
+ # print("result.keys()=", result.keys())
+ # print("result['token_type_ids']=", result["token_type_ids"][0])
+ return result
+
+ # add by wjn
+ # process data for prompt (add template)
+ def prompt_preprocess_function(self, examples):
+
+ def replace_mask_token(template):
+ return template.replace("", self.tokenizer.convert_ids_to_tokens(self.special_token_mapping["mask"]))
+
+ sequence1_prefix_template = replace_mask_token(self.template[0]["prefix_template"] if self.template[0] is not None else "")
+ sequence1_suffix_template = replace_mask_token(self.template[0]["suffix_template"] if self.template[0] is not None else "")
+ sequence2_prefix_template = replace_mask_token(self.template[1]["prefix_template"] if self.template[1] is not None else "")
+ sequence2_suffix_template = replace_mask_token(self.template[1]["suffix_template"] if self.template[1] is not None else "")
+ example_num = len(examples[self.sentence1_key])
+ for example_id in range(example_num):
+ sequence1 = examples[self.sentence1_key][example_id]
+ if self.sentence2_key is None:
+ sequence1 = sequence1[:self.data_args.max_seq_length - len(sequence1_suffix_template) - 10]
+ examples[self.sentence1_key][example_id] = "{}{}{}".format(
+ sequence1_prefix_template, sequence1, sequence1_suffix_template)
+
+ if self.sentence2_key is not None:
+ sequence2 = examples[self.sentence2_key][example_id]
+ sequence2 = sequence2[:self.data_args.max_seq_length - len(sequence1) - len(sequence1_prefix_template) - len(sequence1_suffix_template) - len(sequence2_prefix_template)- 10]
+ examples[self.sentence2_key][example_id] = "{}{}{}".format(
+ sequence2_prefix_template, sequence2, sequence2_suffix_template)
+ return examples
+
+
+ def compute_metrics(self, p: EvalPrediction):
+ preds = p.predictions[0] if isinstance(p.predictions, tuple) else p.predictions
+ preds = np.argmax(preds, axis=1)
+
+ if self.data_args.dataset_name == "record":
+ return self.reocrd_compute_metrics(p)
+
+ if self.data_args.dataset_name == "multirc":
+ from sklearn.metrics import f1_score
+ return {"f1": f1_score(preds, p.label_ids)}
+
+ if self.data_args.dataset_name is not None:
+ result = self.metric.compute(predictions=preds, references=p.label_ids)
+ if len(result) > 1:
+ result["combined_score"] = np.mean(list(result.values())).item()
+ return result
+ elif self.is_regression:
+ return {"mse": ((preds - p.label_ids) ** 2).mean().item()}
+ else:
+ return {"accuracy": (preds == p.label_ids).astype(np.float32).mean().item()}
+
+ def reocrd_compute_metrics(self, p: EvalPrediction):
+ from tasks.superglue.utils import f1_score, exact_match_score, metric_max_over_ground_truths
+ probs = p.predictions[0] if isinstance(p.predictions, tuple) else p.predictions
+ examples = self.eval_dataset
+ qid2pred = defaultdict(list)
+ qid2ans = {}
+ for prob, example in zip(probs, examples):
+ qid = example['question_id']
+ qid2pred[qid].append((prob[1], example['entity']))
+ if qid not in qid2ans:
+ qid2ans[qid] = example['answers']
+ n_correct, n_total = 0, 0
+ f1, em = 0, 0
+ for qid in qid2pred:
+ preds = sorted(qid2pred[qid], reverse=True)
+ entity = preds[0][1]
+ n_total += 1
+ n_correct += (entity in qid2ans[qid])
+ f1 += metric_max_over_ground_truths(f1_score, entity, qid2ans[qid])
+ em += metric_max_over_ground_truths(exact_match_score, entity, qid2ans[qid])
+ acc = n_correct / n_total
+ f1 = f1 / n_total
+ em = em / n_total
+ return {'f1': f1, 'exact_match': em}
+
+ def record_preprocess_function(self, examples, split="train"):
+ results = {
+ "index": list(),
+ "question_id": list(),
+ "input_ids": list(),
+ "attention_mask": list(),
+ "token_type_ids": list(),
+ "label": list(),
+ "entity": list(),
+ "answers": list()
+ }
+ for idx, passage in enumerate(examples["passage"]):
+ query, entities, answers = examples["query"][idx], examples["entities"][idx], examples["answers"][idx]
+ index = examples["idx"][idx]
+ passage = passage.replace("@highlight\n", "- ")
+
+ for ent_idx, ent in enumerate(entities):
+ question = query.replace("@placeholder", ent)
+ result = self.tokenizer(passage, question, padding=self.padding, max_length=self.max_seq_length, truncation=True)
+ label = 1 if ent in answers else 0
+
+ results["input_ids"].append(result["input_ids"])
+ results["attention_mask"].append(result["attention_mask"])
+ if "token_type_ids" in result: results["token_type_ids"].append(result["token_type_ids"])
+ results["label"].append(label)
+ results["index"].append(index)
+ results["question_id"].append(index["query"])
+ results["entity"].append(ent)
+ results["answers"].append(answers)
+
+ return results
diff --git a/tasks/superglue/dataset_record.py b/tasks/superglue/dataset_record.py
new file mode 100644
index 0000000..75f74fd
--- /dev/null
+++ b/tasks/superglue/dataset_record.py
@@ -0,0 +1,251 @@
+import torch
+from torch.utils import data
+from torch.utils.data import Dataset
+from datasets.arrow_dataset import Dataset as HFDataset
+from datasets.load import load_dataset, load_metric
+from transformers import (
+ AutoTokenizer,
+ DataCollatorWithPadding,
+ EvalPrediction,
+ default_data_collator,
+ DataCollatorForLanguageModeling
+)
+import random
+import numpy as np
+import logging
+
+from tasks.superglue.dataset import SuperGlueDataset
+
+from dataclasses import dataclass
+from transformers.data.data_collator import DataCollatorMixin
+from transformers.file_utils import PaddingStrategy
+from transformers.tokenization_utils_base import PreTrainedTokenizerBase
+from typing import Any, Callable, Dict, List, NewType, Optional, Tuple, Union
+
+logger = logging.getLogger(__name__)
+
+@dataclass
+class DataCollatorForMultipleChoice(DataCollatorMixin):
+ tokenizer: PreTrainedTokenizerBase
+ padding: Union[bool, str, PaddingStrategy] = True
+ max_length: Optional[int] = None
+ pad_to_multiple_of: Optional[int] = None
+ label_pad_token_id: int = -100
+ return_tensors: str = "pt"
+
+ def torch_call(self, features):
+ label_name = "label" if "label" in features[0].keys() else "labels"
+ labels = [feature[label_name] for feature in features] if label_name in features[0].keys() else None
+ batch = self.tokenizer.pad(
+ features,
+ padding=self.padding,
+ max_length=self.max_length,
+ pad_to_multiple_of=self.pad_to_multiple_of,
+ # Conversion to tensors will fail if we have labels as they are not of the same length yet.
+ return_tensors="pt" if labels is None else None,
+ )
+
+ if labels is None:
+ return batch
+
+ sequence_length = torch.tensor(batch["input_ids"]).shape[1]
+ padding_side = self.tokenizer.padding_side
+ if padding_side == "right":
+ batch[label_name] = [
+ list(label) + [self.label_pad_token_id] * (sequence_length - len(label)) for label in labels
+ ]
+ else:
+ batch[label_name] = [
+ [self.label_pad_token_id] * (sequence_length - len(label)) + list(label) for label in labels
+ ]
+
+ batch = {k: torch.tensor(v, dtype=torch.int64) for k, v in batch.items()}
+ print(batch)
+ input_list = [sample['input_ids'] for sample in batch]
+
+ choice_nums = list(map(len, input_list))
+ max_choice_num = max(choice_nums)
+
+ def pad_choice_dim(data, choice_num):
+ if len(data) < choice_num:
+ data = np.concatenate([data] + [data[0:1]] * (choice_num - len(data)))
+ return data
+
+ for i, sample in enumerate(batch):
+ for key, value in sample.items():
+ if key != 'label':
+ sample[key] = pad_choice_dim(value, max_choice_num)
+ else:
+ sample[key] = value
+ # sample['loss_mask'] = np.array([1] * choice_nums[i] + [0] * (max_choice_num - choice_nums[i]),
+ # dtype=np.int64)
+
+ return batch
+
+
+class SuperGlueDatasetForRecord(SuperGlueDataset):
+ def __init__(self, tokenizer: AutoTokenizer, data_args, training_args) -> None:
+ raw_datasets = load_dataset("super_glue", data_args.dataset_name)
+ self.tokenizer = tokenizer
+ self.data_args = data_args
+ #labels
+ self.multiple_choice = data_args.dataset_name in ["copa", "record"]
+
+ if not self.multiple_choice:
+ self.label_list = raw_datasets["train"].features["label"].names
+ self.num_labels = len(self.label_list)
+ else:
+ self.num_labels = 1
+
+ # Padding strategy
+ if data_args.pad_to_max_length:
+ self.padding = "max_length"
+ else:
+ # We will pad later, dynamically at batch creation, to the max sequence length in each batch
+ self.padding = False
+
+ # Some models have set the order of the labels to use, so let's make sure we do use it.
+ self.label_to_id = None
+
+ if self.label_to_id is not None:
+ self.label2id = self.label_to_id
+ self.id2label = {id: label for label, id in self.label2id.items()}
+ elif not self.multiple_choice:
+ self.label2id = {l: i for i, l in enumerate(self.label_list)}
+ self.id2label = {id: label for label, id in self.label2id.items()}
+
+
+ if data_args.max_seq_length > tokenizer.model_max_length:
+ logger.warning(
+ f"The max_seq_length passed ({data_args.max_seq_length}) is larger than the maximum length for the"
+ f"model ({tokenizer.model_max_length}). Using max_seq_length={tokenizer.model_max_length}."
+ )
+ self.max_seq_length = min(data_args.max_seq_length, tokenizer.model_max_length)
+
+ if training_args.do_train:
+ self.train_dataset = raw_datasets["train"]
+ if data_args.max_train_samples is not None:
+ self.train_dataset = self.train_dataset.select(range(data_args.max_train_samples))
+
+ self.train_dataset = self.train_dataset.map(
+ self.prepare_train_dataset,
+ batched=True,
+ load_from_cache_file=not data_args.overwrite_cache,
+ remove_columns=raw_datasets["train"].column_names,
+ desc="Running tokenizer on train dataset",
+ )
+
+ if training_args.do_eval:
+ self.eval_dataset = raw_datasets["validation"]
+ if data_args.max_eval_samples is not None:
+ self.eval_dataset = self.eval_dataset.select(range(data_args.max_eval_samples))
+
+ self.eval_dataset = self.eval_dataset.map(
+ self.prepare_eval_dataset,
+ batched=True,
+ load_from_cache_file=not data_args.overwrite_cache,
+ remove_columns=raw_datasets["train"].column_names,
+ desc="Running tokenizer on validation dataset",
+ )
+
+ self.metric = load_metric("super_glue", data_args.dataset_name)
+
+ self.data_collator = DataCollatorForMultipleChoice(tokenizer)
+ # if data_args.pad_to_max_length:
+ # self.data_collator = default_data_collator
+ # elif training_args.fp16:
+ # self.data_collator = DataCollatorWithPadding(tokenizer, pad_to_multiple_of=8)
+ def preprocess_function(self, examples):
+ results = {
+ "input_ids": list(),
+ "attention_mask": list(),
+ "token_type_ids": list(),
+ "label": list()
+ }
+ for passage, query, entities, answers in zip(examples["passage"], examples["query"], examples["entities"], examples["answers"]):
+ passage = passage.replace("@highlight\n", "- ")
+
+ input_ids = []
+ attention_mask = []
+ token_type_ids = []
+
+ for _, ent in enumerate(entities):
+ question = query.replace("@placeholder", ent)
+ result = self.tokenizer(passage, question, padding=self.padding, max_length=self.max_seq_length, truncation=True)
+
+ input_ids.append(result["input_ids"])
+ attention_mask.append(result["attention_mask"])
+ if "token_type_ids" in result: token_type_ids.append(result["token_type_ids"])
+ label = 1 if ent in answers else 0
+
+ result["label"].append()
+
+ return results
+
+
+ def prepare_train_dataset(self, examples, max_train_candidates_per_question=10):
+ entity_shuffler = random.Random(44)
+ results = {
+ "input_ids": list(),
+ "attention_mask": list(),
+ "token_type_ids": list(),
+ "label": list()
+ }
+ for passage, query, entities, answers in zip(examples["passage"], examples["query"], examples["entities"], examples["answers"]):
+ passage = passage.replace("@highlight\n", "- ")
+
+ for answer in answers:
+ input_ids = []
+ attention_mask = []
+ token_type_ids = []
+ candidates = [ent for ent in entities if ent not in answers]
+ # if len(candidates) < max_train_candidates_per_question - 1:
+ # continue
+ if len(candidates) > max_train_candidates_per_question - 1:
+ entity_shuffler.shuffle(candidates)
+ candidates = candidates[:max_train_candidates_per_question - 1]
+ candidates = [answer] + candidates
+
+ for ent in candidates:
+ question = query.replace("@placeholder", ent)
+ result = self.tokenizer(passage, question, padding=self.padding, max_length=self.max_seq_length, truncation=True)
+ input_ids.append(result["input_ids"])
+ attention_mask.append(result["attention_mask"])
+ if "token_type_ids" in result: token_type_ids.append(result["token_type_ids"])
+
+ results["input_ids"].append(input_ids)
+ results["attention_mask"].append(attention_mask)
+ if len(token_type_ids) > 0: results["token_type_ids"].append(token_type_ids)
+ results["label"].append(0)
+
+ return results
+
+
+ def prepare_eval_dataset(self, examples):
+
+ results = {
+ "input_ids": list(),
+ "attention_mask": list(),
+ "token_type_ids": list(),
+ "label": list()
+ }
+ for passage, query, entities, answers in zip(examples["passage"], examples["query"], examples["entities"], examples["answers"]):
+ passage = passage.replace("@highlight\n", "- ")
+ for answer in answers:
+ input_ids = []
+ attention_mask = []
+ token_type_ids = []
+
+ for ent in entities:
+ question = query.replace("@placeholder", ent)
+ result = self.tokenizer(passage, question, padding=self.padding, max_length=self.max_seq_length, truncation=True)
+ input_ids.append(result["input_ids"])
+ attention_mask.append(result["attention_mask"])
+ if "token_type_ids" in result: token_type_ids.append(result["token_type_ids"])
+
+ results["input_ids"].append(input_ids)
+ results["attention_mask"].append(attention_mask)
+ if len(token_type_ids) > 0: results["token_type_ids"].append(token_type_ids)
+ results["label"].append(0)
+
+ return results
diff --git a/tasks/superglue/get_trainer.py b/tasks/superglue/get_trainer.py
new file mode 100644
index 0000000..847d3d3
--- /dev/null
+++ b/tasks/superglue/get_trainer.py
@@ -0,0 +1,104 @@
+import logging
+import os
+import random
+import sys
+
+from transformers import (
+ AutoConfig,
+ AutoTokenizer,
+)
+
+from model.utils import get_model, TaskType
+from tasks.superglue.dataset import SuperGlueDataset
+from training.trainer_base import BaseTrainer
+from training.trainer_exp import ExponentialTrainer
+from training.self_trainer import SelfTrainer
+
+logger = logging.getLogger(__name__)
+
+def get_trainer(args):
+ model_args, data_args, training_args, semi_training_args, _ = args
+
+ log_level = training_args.get_process_log_level()
+ logger.setLevel(log_level)
+
+ tokenizer = AutoTokenizer.from_pretrained(
+ model_args.model_name_or_path,
+ use_fast=model_args.use_fast_tokenizer,
+ revision=model_args.model_revision,
+ )
+
+ # add by wjn check if use prompt template
+ use_prompt = False
+ if model_args.prompt_prefix or model_args.prompt_ptuning or model_args.prompt_adapter or model_args.prompt_only:
+ use_prompt = True
+
+ dataset = SuperGlueDataset(tokenizer, data_args, training_args, semi_training_args=semi_training_args, use_prompt=use_prompt)
+
+ data_args.label_word_list = None # add by wjn
+ if use_prompt:
+ data_args.label_word_list = dataset.label_word_list # add by wjn
+
+ if training_args.do_train:
+ for index in random.sample(range(len(dataset.train_dataset)), 3):
+ logger.info(f"Sample {index} of the training set: {dataset.train_dataset[index]}.")
+
+ if not dataset.multiple_choice:
+ config = AutoConfig.from_pretrained(
+ model_args.model_name_or_path,
+ num_labels=dataset.num_labels,
+ label2id=dataset.label2id,
+ id2label=dataset.id2label,
+ finetuning_task=data_args.dataset_name,
+ revision=model_args.model_revision,
+ )
+ else:
+ config = AutoConfig.from_pretrained(
+ model_args.model_name_or_path,
+ num_labels=dataset.num_labels,
+ finetuning_task=data_args.dataset_name,
+ revision=model_args.model_revision,
+ )
+
+ if not dataset.multiple_choice:
+ model = get_model(data_args, model_args, TaskType.SEQUENCE_CLASSIFICATION, config)
+ else:
+ model = get_model(data_args, model_args, TaskType.MULTIPLE_CHOICE, config)
+
+
+ # Initialize our Trainer
+
+ if semi_training_args.use_semi:
+ model_args.pre_seq_len = semi_training_args.student_pre_seq_len
+ student_model = get_model(data_args, model_args, TaskType.SEQUENCE_CLASSIFICATION, config)
+ trainer = SelfTrainer(
+ teacher_base_model=model,
+ student_base_model=student_model,
+ training_args=training_args,
+ semi_training_args=semi_training_args,
+ train_dataset=dataset.train_dataset if training_args.do_train else None,
+ unlabeled_dataset=dataset.unlabeled_dataset,
+ eval_dataset=dataset.eval_dataset if training_args.do_eval else None,
+ compute_metrics=dataset.compute_metrics,
+ tokenizer=tokenizer,
+ teacher_data_collator=dataset.data_collator,
+ student_data_collator=dataset.data_collator,
+ test_key=dataset.test_key,
+ task_type="cls",
+ num_classes=len(dataset.label2id),
+ )
+
+ return trainer, None
+
+ trainer = BaseTrainer(
+ model=model,
+ args=training_args,
+ train_dataset=dataset.train_dataset if training_args.do_train else None,
+ eval_dataset=dataset.eval_dataset if training_args.do_eval else None,
+ compute_metrics=dataset.compute_metrics,
+ tokenizer=tokenizer,
+ data_collator=dataset.data_collator,
+ test_key=dataset.test_key
+ )
+
+ return trainer, None
diff --git a/tasks/superglue/utils.py b/tasks/superglue/utils.py
new file mode 100644
index 0000000..3db8265
--- /dev/null
+++ b/tasks/superglue/utils.py
@@ -0,0 +1,47 @@
+import re
+import string
+from collections import defaultdict, Counter
+
+
+
+def normalize_answer(s):
+ """Lower text and remove punctuation, articles and extra whitespace."""
+
+ def remove_articles(text):
+ return re.sub(r'\b(a|an|the)\b', ' ', text)
+
+ def white_space_fix(text):
+ return ' '.join(text.split())
+
+ def remove_punc(text):
+ exclude = set(string.punctuation)
+ return ''.join(ch for ch in text if ch not in exclude)
+
+ def lower(text):
+ return text.lower()
+
+ return white_space_fix(remove_articles(remove_punc(lower(s))))
+
+def f1_score(prediction, ground_truth):
+ prediction_tokens = normalize_answer(prediction).split()
+ ground_truth_tokens = normalize_answer(ground_truth).split()
+ common = Counter(prediction_tokens) & Counter(ground_truth_tokens)
+ num_same = sum(common.values())
+ if num_same == 0:
+ return 0
+ precision = 1.0 * num_same / len(prediction_tokens)
+ recall = 1.0 * num_same / len(ground_truth_tokens)
+ f1 = (2 * precision * recall) / (precision + recall)
+ return f1
+
+
+def exact_match_score(prediction, ground_truth):
+ return normalize_answer(prediction) == normalize_answer(ground_truth)
+
+
+def metric_max_over_ground_truths(metric_fn, prediction, ground_truths):
+ scores_for_ground_truths = []
+ for ground_truth in ground_truths:
+ score = metric_fn(prediction, ground_truth)
+ scores_for_ground_truths.append(score)
+ return max(scores_for_ground_truths)
\ No newline at end of file
diff --git a/tasks/utils.py b/tasks/utils.py
new file mode 100644
index 0000000..c27ba18
--- /dev/null
+++ b/tasks/utils.py
@@ -0,0 +1,47 @@
+from tasks.glue.dataset import task_to_keys as glue_tasks
+from tasks.superglue.dataset import task_to_keys as superglue_tasks
+from datasets.load import load_dataset, load_metric
+
+
+GLUE_DATASETS = list(glue_tasks.keys())
+SUPERGLUE_DATASETS = list(superglue_tasks.keys())
+NER_DATASETS = ["conll2003", "conll2004", "ontonotes"]
+SRL_DATASETS = ["conll2005", "conll2012"]
+QA_DATASETS = ["squad", "squad_v2"]
+OTHER_DATASETS = ["movie_rationales", "cr", "snli", "trec", "ag_news", "yelp_polarity"]
+
+
+TASKS = ["glue", "superglue", "ner", "srl", "qa", "other_cls"]
+
+DATASETS = GLUE_DATASETS + SUPERGLUE_DATASETS + NER_DATASETS + SRL_DATASETS + QA_DATASETS + OTHER_DATASETS
+
+ADD_PREFIX_SPACE = {
+ 'bert': False,
+ 'roberta': True,
+ 'deberta': True,
+ 'gpt2': True,
+ 'deberta-v2': True,
+}
+
+USE_FAST = {
+ 'bert': True,
+ 'roberta': True,
+ 'deberta': True,
+ 'gpt2': True,
+ 'deberta-v2': False,
+}
+
+
+
+
+
+
+# def random_sampling(sentences, labels, num):
+# """randomly sample subset of the training pairs"""
+# assert len(sentences) == len(labels)
+# if num > len(labels):
+# assert False, f"you tried to randomly sample {num}, which is more than the total size of the pool {len(labels)}"
+# idxs = np.random.choice(len(labels), size=num, replace=False)
+# selected_sentences = [sentences[i] for i in idxs]
+# selected_labels = [labels[i] for i in idxs]
+# return deepcopy(selected_sentences), deepcopy(selected_labels)
\ No newline at end of file
diff --git a/training/sampler.py b/training/sampler.py
new file mode 100644
index 0000000..251cad4
--- /dev/null
+++ b/training/sampler.py
@@ -0,0 +1,141 @@
+"""
+Author: Subhabrata Mukherjee (submukhe@microsoft.com)
+Code for Uncertainty-aware Self-training (UST) for few-shot learning.
+"""
+
+from sklearn.utils import shuffle
+
+import logging
+import numpy as np
+import os
+import random
+
+
+logger = logging.getLogger('UST')
+
+def get_BALD_acquisition(y_T):
+
+ expected_entropy = - np.mean(np.sum(y_T * np.log(y_T + 1e-10), axis=-1), axis=0)
+ expected_p = np.mean(y_T, axis=0)
+ entropy_expected_p = - np.sum(expected_p * np.log(expected_p + 1e-10), axis=-1)
+ return (entropy_expected_p - expected_entropy)
+
+def sample_by_bald_difficulty(tokenizer, X, y_mean, y_var, y, num_samples, num_classes, y_T):
+
+ logger.info ("Sampling by difficulty BALD acquisition function")
+ BALD_acq = get_BALD_acquisition(y_T)
+ p_norm = np.maximum(np.zeros(len(BALD_acq)), BALD_acq)
+ p_norm = p_norm / np.sum(p_norm)
+ indices = np.random.choice(len(X['input_ids']), num_samples, p=p_norm, replace=False)
+ X_s = {"input_ids": X["input_ids"][indices], "token_type_ids": X["token_type_ids"][indices], "attention_mask": X["attention_mask"][indices]}
+ y_s = y[indices]
+ w_s = y_var[indices][:,0]
+ return X_s, y_s, w_s
+
+
+def sample_by_bald_easiness(tokenizer, X, y_mean, y_var, y, num_samples, num_classes, y_T):
+
+ logger.info ("Sampling by easy BALD acquisition function")
+ BALD_acq = get_BALD_acquisition(y_T)
+ p_norm = np.maximum(np.zeros(len(BALD_acq)), (1. - BALD_acq)/np.sum(1. - BALD_acq))
+ p_norm = p_norm / np.sum(p_norm)
+ logger.info (p_norm[:10])
+ indices = np.random.choice(len(X['input_ids']), num_samples, p=p_norm, replace=False)
+ X_s = {"input_ids": X["input_ids"][indices], "token_type_ids": X["token_type_ids"][indices], "attention_mask": X["attention_mask"][indices]}
+ y_s = y[indices]
+ w_s = y_var[indices][:,0]
+ return X_s, y_s, w_s
+
+
+def sample_by_bald_class_easiness(tokenizer, X, y_mean, y_var, y, num_samples, num_classes, y_T):
+
+ logger.info ("Sampling by easy BALD acquisition function per class")
+ BALD_acq = get_BALD_acquisition(y_T)
+ BALD_acq = (1. - BALD_acq)/np.sum(1. - BALD_acq)
+ logger.info (BALD_acq)
+ samples_per_class = num_samples // num_classes
+ X_s_input_ids, X_s_token_type_ids, X_s_attention_mask, X_s_mask_pos, y_s, w_s = [], [], [], [], [], []
+ for label in range(num_classes):
+ # X_input_ids, X_token_type_ids, X_attention_mask = np.array(X['input_ids'])[y == label], np.array(X['token_type_ids'])[y == label], np.array(X['attention_mask'])[y == label]
+ X_input_ids, X_attention_mask = np.array(X['input_ids'])[y == label], np.array(X['attention_mask'])[y == label]
+ if "token_type_ids" in X.features:
+ X_token_type_ids = np.array(X['token_type_ids'])[y == label]
+ if "mask_pos" in X.features:
+ X_mask_pos = np.array(X['mask_pos'])[y == label]
+ y_ = y[y==label]
+ y_var_ = y_var[y == label]
+ # p = y_mean[y == label]
+ p_norm = BALD_acq[y==label]
+ p_norm = np.maximum(np.zeros(len(p_norm)), p_norm)
+ p_norm = p_norm/np.sum(p_norm)
+ if len(X_input_ids) < samples_per_class:
+ logger.info ("Sampling with replacement.")
+ replace = True
+ else:
+ replace = False
+ # print("====== label: {} ======".format(label))
+ # print("len(X_input_ids)=", len(X_input_ids))
+ # print("samples_per_class=", samples_per_class)
+ # print("p_norm=", p_norm)
+ # print("replace=", replace)
+ if len(X_input_ids) == 0: # add by wjn
+ continue
+ indices = np.random.choice(len(X_input_ids), samples_per_class, p=p_norm, replace=replace)
+ X_s_input_ids.extend(X_input_ids[indices])
+ # X_s_token_type_ids.extend(X_token_type_ids[indices])
+ X_s_attention_mask.extend(X_attention_mask[indices])
+ if "token_type_ids" in X.features:
+ X_s_token_type_ids.extend(X_token_type_ids[indices])
+ if "mask_pos" in X.features:
+ X_s_mask_pos.extend(X_mask_pos[indices])
+ y_s.extend(y_[indices])
+ w_s.extend(y_var_[indices][:,0])
+ # X_s_input_ids, X_s_token_type_ids, X_s_attention_mask, y_s, w_s = shuffle(X_s_input_ids, X_s_token_type_ids, X_s_attention_mask, y_s, w_s)
+ if "token_type_ids" in X.features and "mask_pos" not in X.features:
+ X_s_input_ids, X_s_token_type_ids, X_s_attention_mask, y_s, w_s = shuffle(X_s_input_ids, X_s_token_type_ids, X_s_attention_mask, y_s, w_s)
+ elif "token_type_ids" not in X.features and "mask_pos" in X.features:
+ X_s_input_ids, X_s_mask_pos, X_s_attention_mask, y_s, w_s = shuffle(X_s_input_ids, X_s_mask_pos, X_s_attention_mask, y_s, w_s)
+ elif "token_type_ids" in X.features and "mask_pos" in X.features:
+ X_s_input_ids, X_s_token_type_ids, X_s_mask_pos, X_s_attention_mask, y_s, w_s = shuffle(X_s_input_ids, X_s_token_type_ids, X_s_mask_pos, X_s_attention_mask, y_s, w_s)
+ else:
+ X_s_input_ids, X_s_attention_mask, y_s, w_s = shuffle(X_s_input_ids, X_s_attention_mask, y_s, w_s)
+
+ # return {'input_ids': np.array(X_s_input_ids), 'token_type_ids': np.array(X_s_token_type_ids), 'attention_mask': np.array(X_s_attention_mask)}, np.array(y_s), np.array(w_s)
+
+ pseudo_labeled_input = {
+ 'input_ids': np.array(X_s_input_ids),
+ 'attention_mask': np.array(X_s_attention_mask)
+ }
+ if "token_type_ids" in X.features:
+ pseudo_labeled_input['token_type_ids'] = np.array(X_s_token_type_ids)
+ if "mask_pos" in X.features:
+ pseudo_labeled_input['mask_pos'] = np.array(X_s_mask_pos)
+ return pseudo_labeled_input, np.array(y_s), np.array(w_s)
+
+
+def sample_by_bald_class_difficulty(tokenizer, X, y_mean, y_var, y, num_samples, num_classes, y_T):
+
+ logger.info ("Sampling by difficulty BALD acquisition function per class")
+ BALD_acq = get_BALD_acquisition(y_T)
+ samples_per_class = num_samples // num_classes
+ X_s_input_ids, X_s_token_type_ids, X_s_attention_mask, y_s, w_s = [], [], [], [], []
+ for label in range(num_classes):
+ X_input_ids, X_token_type_ids, X_attention_mask = X['input_ids'][y == label], X['token_type_ids'][y == label], X['attention_mask'][y == label]
+ y_ = y[y==label]
+ y_var_ = y_var[y == label]
+ p_norm = BALD_acq[y==label]
+ p_norm = np.maximum(np.zeros(len(p_norm)), p_norm)
+ p_norm = p_norm/np.sum(p_norm)
+ if len(X_input_ids) < samples_per_class:
+ replace = True
+ logger.info ("Sampling with replacement.")
+ else:
+ replace = False
+ indices = np.random.choice(len(X_input_ids), samples_per_class, p=p_norm, replace=replace)
+ X_s_input_ids.extend(X_input_ids[indices])
+ X_s_token_type_ids.extend(X_token_type_ids[indices])
+ X_s_attention_mask.extend(X_attention_mask[indices])
+ y_s.extend(y_[indices])
+ w_s.extend(y_var_[indices][:,0])
+ X_s_input_ids, X_s_token_type_ids, X_s_attention_mask, y_s, w_s = shuffle(X_s_input_ids, X_s_token_type_ids, X_s_attention_mask, y_s, w_s)
+ return {'input_ids': np.array(X_s_input_ids), 'token_type_ids': np.array(X_s_token_type_ids), 'attention_mask': np.array(X_s_attention_mask)}, np.array(y_s), np.array(w_s)
diff --git a/training/self_trainer.py b/training/self_trainer.py
new file mode 100644
index 0000000..958bd27
--- /dev/null
+++ b/training/self_trainer.py
@@ -0,0 +1,608 @@
+import os
+from typing import Dict, OrderedDict
+from tqdm import tqdm
+import torch
+import torch.nn as nn
+from typing import Union, Optional, Callable, List, Tuple
+from transformers import Trainer
+import datasets
+import numpy as np
+from typing import Optional, List
+from datasets import Dataset, DatasetInfo, NamedSplit, DatasetDict
+from datasets.table import Table, list_table_cache_files
+from torch.utils.data import RandomSampler, DistributedSampler, DataLoader
+from transformers import PreTrainedModel, DataCollator, PreTrainedTokenizerBase, EvalPrediction, TrainerCallback
+from transformers.trainer_pt_utils import DistributedSamplerWithLoop, get_length_grouped_indices
+from transformers.trainer_pt_utils import DistributedLengthGroupedSampler as DistributedLengthGroupedSamplerOri
+from transformers.trainer_pt_utils import LengthGroupedSampler as LengthGroupedSamplerOri
+# from transformers.trainer_utils import has_length
+from transformers.training_args import ParallelMode
+from transformers.trainer_pt_utils import (
+ DistributedTensorGatherer,
+ SequentialDistributedSampler,
+ nested_concat,
+ )
+from transformers.utils import logging
+from transformers.trainer_utils import denumpify_detensorize, TrainOutput
+from training.sampler import sample_by_bald_class_easiness
+from training.trainer_base import BaseTrainer
+
+
+logger = logging.get_logger('Self-training')
+
+WEIGHTS_NAME = "pytorch_model.bin"
+WEIGHTS_INDEX_NAME = "pytorch_model.bin.index.json"
+
+class DatasetK(Dataset):
+ def __init__(
+ self,
+ arrow_table: Table,
+ info: Optional[DatasetInfo] = None,
+ split: Optional[NamedSplit] = None,
+ indices_table: Optional[Table] = None,
+ fingerprint: Optional[str] = None,
+ ):
+ self.custom_cache_files = None
+ super(DatasetK, self).__init__(arrow_table, info, split, indices_table, fingerprint)
+
+
+ @property
+ def cache_files(self) -> List[dict]:
+ """The cache files containing the Apache Arrow table backing the dataset."""
+ if self.custom_cache_files:
+ return self.custom_cache_files
+ cache_files = list_table_cache_files(self._data)
+ if self._indices is not None:
+ cache_files += list_table_cache_files(self._indices)
+ return [{"filename": cache_filename} for cache_filename in cache_files]
+
+ def set_cache_files(self, custom_cache_files):
+ self.custom_cache_files = custom_cache_files
+
+
+# add by wjn
+def random_sampling(raw_datasets, num_examples_per_label: Optional[int]=16):
+ label_list = raw_datasets["label"] # [0, 1, 0, 0, ...]
+ label_dict = dict()
+ # 记录每个label对应的样本索引
+ for ei, label in enumerate(label_list):
+ if label not in label_dict.keys():
+ label_dict[label] = list()
+ label_dict[label].append(ei)
+ # 对于每个类别,随机采样k个样本
+ few_example_ids = list()
+ for label, eid_list in label_dict.items():
+ # examples = deepcopy(eid_list)
+ # shuffle(examples)
+ idxs = np.random.choice(len(eid_list), size=num_examples_per_label, replace=False)
+ selected_eids = [eid_list[i] for i in idxs]
+ few_example_ids.extend(selected_eids)
+ return few_example_ids
+
+class TeacherTrainer(BaseTrainer):
+ def __init__(
+ self,
+ model: Union[PreTrainedModel, nn.Module] = None,
+ args = None,
+ data_collator: Optional[DataCollator] = None,
+ train_dataset: Optional[Dataset] = None,
+ eval_dataset: Optional[Dataset] = None,
+ tokenizer: Optional[PreTrainedTokenizerBase] = None,
+ model_init: Callable[[], PreTrainedModel] = None,
+ compute_metrics: Optional[Callable[[EvalPrediction], Dict]] = None,
+ callbacks: Optional[List[TrainerCallback]] = None,
+ optimizers: Tuple[torch.optim.Optimizer, torch.optim.lr_scheduler.LambdaLR] = (None, None),
+ test_key: str = "accuracy"
+ ):
+ super(TeacherTrainer, self).__init__(model, args, data_collator, train_dataset, eval_dataset, tokenizer, model_init, compute_metrics, callbacks, optimizers)
+ self.predict_dataset = eval_dataset
+ self.test_key = test_key
+ # if self.args.do_adv:
+ # self.fgm = FGM(self.model)
+ # for callback in callbacks:
+ # callback.trainer = self
+ self.best_metrics = OrderedDict({
+ "best_epoch": 0,
+ f"best_eval_{self.test_key}": 0,
+ })
+ self.global_step_ = 0
+
+
+ def mc_evaluate(
+ self,
+ unlabeled_dataset: Optional[Dataset] = None,
+ unlabeled_data_num: int = -1,
+ description: str = "Evaluate on Unlabeled Data via MC Dropout Uncertainty Estimation",
+ prediction_loss_only: Optional[bool] = None,
+ ignore_keys: Optional[List[str]] = None,
+ metric_key_prefix: str = "eval",
+ T: int = 30,
+ num_classes: int = 0
+ ):
+ """
+ Prediction/evaluation loop, shared by `Trainer.evaluate()` and `Trainer.predict()`.
+
+ Works both with or without labels.
+ """
+ args = self.args
+
+ prediction_loss_only = prediction_loss_only if prediction_loss_only is not None else args.prediction_loss_only
+ is_sample = True
+ if unlabeled_data_num == -1 or unlabeled_data_num >= len(unlabeled_dataset):
+ unlabeled_data_num = len(unlabeled_dataset)
+ is_sample = False
+
+ if is_sample:
+ recalled_examples_idx_list = random_sampling(
+ raw_datasets=unlabeled_dataset,
+ num_examples_per_label=unlabeled_data_num // num_classes
+ )
+ unlabeled_dataset = unlabeled_dataset.select(recalled_examples_idx_list)
+ unlabeled_data_num = len(unlabeled_dataset)
+
+ unlabeled_dataloader = self.get_eval_dataloader(unlabeled_dataset)
+ model = self._wrap_model(self.model, training=True, dataloader=unlabeled_dataloader) # reset training to True
+
+ batch_size = unlabeled_dataloader.batch_size
+ # unlabeled_data_num = self.num_examples(unlabeled_dataloader)
+ logger.info(f"***** Running {description} *****")
+ logger.info(f" Num examples = {unlabeled_data_num}")
+ logger.info(f" Batch size = {batch_size}")
+
+ # world_size = max(1, args.world_size)
+
+ # if not prediction_loss_only:
+ # # The actual number of eval_sample can be greater than num_examples in distributed settings (when we pass
+ # # a batch size to the sampler)
+ # make_multiple_of = None
+ # if hasattr(unlabeled_dataloader, "sampler") and isinstance(unlabeled_dataloader.sampler, SequentialDistributedSampler):
+ # make_multiple_of = unlabeled_dataloader.sampler.batch_size
+
+ model.train() # 开启train模式,允许模型进行Dropout
+
+ if args.past_index >= 0:
+ self._past = None
+
+ self.callback_handler.eval_dataloader = unlabeled_dataloader
+
+ # y_T = np.zeros((T, unlabeled_data_num, num_classes))
+ y_T = list()
+
+ for i in tqdm(range(T)):
+ y_pred = []
+
+ for step, inputs in enumerate(unlabeled_dataloader):
+ _, logits, __ = self.prediction_step(model, inputs, prediction_loss_only, ignore_keys=ignore_keys)
+ y_pred.extend(logits.detach().cpu().numpy().tolist())
+ # print("y_pred.shape=", torch.Tensor(y_pred).shape) # [n, num_class]
+ predict_proba = torch.softmax(torch.Tensor(y_pred).to(logits.device), -1)
+ # print("predict_proba.shape=", predict_proba.shape) # [n, num_class]
+ # y_T[i] = predict_proba.detach().cpu().numpy().tolist()
+ y_T.append(predict_proba.detach().cpu().numpy().tolist())
+
+ y_T = np.array(y_T)
+ #compute mean
+ y_mean = np.mean(y_T, axis=0)
+ # print("y_mean.shape=", y_mean.shape) # e.g., (4095, 3) [n, class_num]
+ # print("(unlabeled_data_num, num_classes)=", (unlabeled_data_num, num_classes))
+ assert y_mean.shape == (unlabeled_data_num, num_classes)
+
+ #compute majority prediction
+ y_pred = np.array([np.argmax(np.bincount(row)) for row in np.transpose(np.argmax(y_T, axis=-1))])
+ assert y_pred.shape == (unlabeled_data_num,)
+
+ #compute variance
+ y_var = np.var(y_T, axis=0)
+ assert y_var.shape == (unlabeled_data_num, num_classes)
+
+ return unlabeled_dataset, y_mean, y_var, y_pred, y_T
+
+
+
+class RobustTrainer(TeacherTrainer):
+ def __init__(
+ self,
+ model: Union[PreTrainedModel, nn.Module] = None,
+ args = None,
+ data_collator: Optional[DataCollator] = None,
+ train_dataset: Optional[Dataset] = None,
+ eval_dataset: Optional[Dataset] = None,
+ tokenizer: Optional[PreTrainedTokenizerBase] = None,
+ model_init: Callable[[], PreTrainedModel] = None,
+ compute_metrics: Optional[Callable[[EvalPrediction], Dict]] = None,
+ callbacks: Optional[List[TrainerCallback]] = None,
+ optimizers: Tuple[torch.optim.Optimizer, torch.optim.lr_scheduler.LambdaLR] = (None, None),
+ test_key: str = "accuracy"
+ ):
+ super(RobustTrainer, self).__init__(model, args, data_collator, train_dataset, eval_dataset, tokenizer, model_init, compute_metrics, callbacks, optimizers)
+ self.predict_dataset = eval_dataset
+ self.test_key = test_key
+ # if self.args.do_adv:
+ # self.fgm = FGM(self.model)
+ # for callback in callbacks:
+ # callback.trainer = self
+ self.best_metrics = OrderedDict({
+ "best_epoch": 0,
+ f"best_eval_{self.test_key}": 0,
+ })
+ self.global_step_ = 0
+
+ def robust_train(self):
+ pass
+
+
+
+class SelfTrainer(object):
+ def __init__(
+ self,
+ teacher_base_model: torch.nn.Module,
+ student_base_model: torch.nn.Module,
+ training_args,
+ semi_training_args,
+ train_dataset: Optional[Dataset]=None,
+ unlabeled_dataset: Optional[Dataset]=None,
+ eval_dataset=None,
+ compute_metrics=None,
+ tokenizer=None,
+ teacher_data_collator=None,
+ student_data_collator=None,
+ test_key=None,
+ task_type="cls",
+ num_classes=0,
+ ) -> None:
+
+ logger.info("This is a Self-trainer.")
+
+ self.teacher_base_model = teacher_base_model
+ self.student_base_model = student_base_model
+ self.training_args = training_args
+ self.semi_training_args = semi_training_args
+ self.train_dataset = train_dataset
+ self.unlabeled_dataset = unlabeled_dataset
+ self.eval_dataset = eval_dataset
+ self.compute_metrics = compute_metrics
+ self.tokenizer = tokenizer
+ self.teacher_data_collator = teacher_data_collator
+ self.student_data_collator = student_data_collator
+ self.test_key = test_key
+ self.task_type = task_type
+ self.num_classes = num_classes
+
+ # self.set_teacher_trainer()
+ # self.set_student_trainer()
+ self.training_args.per_device_train_batch_size = self.semi_training_args.unlabeled_data_batch_size
+ self.teacher_training_epoch = self.semi_training_args.teacher_training_epoch # 最初teacher模型在labeled data上训练的epoch数
+ self.teacher_tuning_epoch = self.semi_training_args.teacher_tuning_epoch # 每一轮Self-training时,teacher模型继续在labeled data上tune的epoch数
+ self.student_training_epoch = self.semi_training_args.student_training_epoch # 每一轮Self-training时,student模型在pseudo-labeled data上训练的epoch数
+ self.self_training_epoch = self.semi_training_args.self_training_epoch # Self-training迭代数
+ self.unlabeled_data_num = self.semi_training_args.unlabeled_data_num # self-training每轮迭代时,首先挑选一部分用于计算MC dropout uncertainty。-1表示全部计算uncertainty
+ self.pseudo_sample_num_or_ratio = self.semi_training_args.pseudo_sample_num_or_ratio # MC dropout后,从所有计算过uncertainty的unlabeled data上采样的样本比例/数量
+ self.student_learning_rate = self.semi_training_args.student_learning_rate
+ self.student_pre_seq_len = self.semi_training_args.student_pre_seq_len
+ self.output_dir = self.training_args.output_dir
+
+ def get_teacher_trainer(
+ self,
+ base_model: torch.nn.Module,
+ num_train_epochs: int,
+ output_dir: str = None,
+ ):
+ training_args = self.training_args
+ training_args.num_train_epochs = num_train_epochs
+ if output_dir is not None:
+ training_args.output_dir = output_dir
+ # 初始化Teacher训练器
+ teacher_trainer = TeacherTrainer(
+ model=base_model,
+ args=training_args,
+ train_dataset=self.train_dataset if self.training_args.do_train else None,
+ eval_dataset=self.eval_dataset if self.training_args.do_eval else None,
+ compute_metrics=self.compute_metrics,
+ tokenizer=self.tokenizer,
+ data_collator=self.teacher_data_collator,
+ test_key=self.test_key,
+ )
+ return teacher_trainer
+
+
+ def get_student_trainer(
+ self,
+ base_model: torch.nn.Module,
+ num_train_epochs: int,
+ student_learning_rate: float,
+ pseudo_labeled_dataset: Optional[Dataset] = None,
+ output_dir: str = None,
+ ):
+ training_args = self.training_args
+ training_args.num_train_epochs = num_train_epochs
+ training_args.learning_rate = student_learning_rate
+ if output_dir is not None:
+ training_args.output_dir = output_dir
+ # 初始化Student训练器
+ student_trainer = RobustTrainer(
+ model=base_model,
+ args=training_args,
+ train_dataset=pseudo_labeled_dataset,
+ eval_dataset=self.eval_dataset,
+ compute_metrics=self.compute_metrics,
+ tokenizer=self.tokenizer,
+ data_collator=self.student_data_collator,
+ test_key=self.test_key,
+ )
+ return student_trainer
+
+ def freeze_backbone(self, model: torch.nn.Module, use_pe: bool=False):
+ try:
+ model.freeze_backbone(use_pe=use_pe)
+ except:
+ pass
+ return model
+
+
+ def train(self, resume_from_checkpoint=None):
+ if not os.path.exists(os.path.join(self.output_dir, "iteration")):
+ os.makedirs(os.path.join(self.output_dir, "iteration"))
+
+ teacher_model = self.teacher_base_model
+ teacher_model = self.freeze_backbone(teacher_model, use_pe=False)
+ teacher_trainer: TeacherTrainer = self.get_teacher_trainer(base_model=teacher_model, num_train_epochs=self.teacher_training_epoch)
+
+ if resume_from_checkpoint is not None and (os.path.isfile(os.path.join(resume_from_checkpoint, WEIGHTS_NAME)) or os.path.isfile(
+ os.path.join(resume_from_checkpoint, WEIGHTS_INDEX_NAME))
+ ):
+ logger.info("*"*80)
+ logger.info("* Directly loading the trained teacher model from {} *".format(resume_from_checkpoint))
+ logger.info("*"*80)
+ print("*"*80)
+ logger.info("* Directly loading the trained teacher model from {} *".format(resume_from_checkpoint))
+ print("*"*80)
+ # 已有teacher模型,直接加载
+ teacher_trainer._load_from_checkpoint(resume_from_checkpoint)
+ else:
+
+ # 首先对Teacher模型在labeled data上进行full parameter fine-tuning
+ logger.info("*"*66)
+ logger.info("* Training teacher model over labeled data before self-training. *")
+ logger.info("*"*66)
+ print("*"*66)
+ print("* Training teacher model over labeled data before self-training. *")
+ print("*"*66)
+
+ teacher_trainer.train()
+ teacher_model.load_state_dict(torch.load(os.path.join(teacher_trainer.state.best_model_checkpoint, "pytorch_model.bin")))
+ teacher_trainer.model = teacher_model
+
+ # 原始的训练结果
+ metrics = teacher_trainer.evaluate()
+ convention_result = metrics["eval_{}".format(self.test_key)]
+
+ logger.info("*"*50)
+ logger.info("* Conventional fine-tuning metric: {}. *".format(convention_result))
+ logger.info("*"*50)
+ print("*"*50)
+ print("* Conventional fine-tuning metric: {}. *".format(convention_result))
+ print("*"*50)
+
+ logger.info("*"*30)
+ logger.info("* Starting Self-training ... *")
+ logger.info("*"*30)
+ print("*"*30)
+ print("* Starting Self-training ... *")
+ print("*"*30)
+
+ best_test_metric = None
+ best_self_training_iteration = None
+ best_teacher_model = None
+
+ # 多轮Teacher-Student迭代训练
+ for iter in range(self.self_training_epoch):
+
+ logger.info("*"*34)
+ logger.info("* Self-training {}-th iteration *".format(iter))
+ logger.info("*"*34)
+ print("*"*34)
+ print("* Self-training {}-th iteration *".format(iter))
+ print("*"*34)
+
+
+ # 获得Teacher模型在测试集上的效果
+ if iter > 0:
+ teacher_trainer.model = teacher_model
+ metrics = teacher_trainer.evaluate()
+ # print("metrics=", metrics)
+
+ '''
+ e.g., {'eval_loss': 0.6926815509796143, 'eval_accuracy': 0.5234657039711191, 'eval_runtime': 0.7267, 'eval_samples_per_second': 381.161, 'eval_steps_per_second': 48.161, 'epoch': 1.0}
+ '''
+ logger.info("*"*60)
+ logger.info("* The testing result of teacher model is {} result: {} *".format(self.test_key, metrics["eval_{}".format(self.test_key)]))
+ logger.info("*"*60)
+ print("*"*60)
+ print("* The testing result of teacher model is {} result: {} *".format(self.test_key, metrics["eval_{}".format(self.test_key)]))
+ print("*"*60)
+
+ if best_test_metric is None or best_test_metric < metrics["eval_{}".format(self.test_key)]:
+ best_test_metric = metrics["eval_{}".format(self.test_key)]
+ best_self_training_iteration = iter
+ best_teacher_model = teacher_model
+ logger.info("The best teacher model at {}-th self-training iteration.".format(best_self_training_iteration))
+ logger.info("The best teacher model testing result is {}.".format(best_test_metric))
+ print("The best teacher model at {}-th self-training iteration.".format(best_self_training_iteration))
+ print("The best teacher model testing result is {}.".format(best_test_metric))
+
+
+ if iter == self.self_training_epoch - 1:
+ break
+
+
+ # # Teacher模型在labeled data上进行parameter-efficient tuning
+ # if iter > 0:
+ # logger.info("*"*80)
+ # logger.info("* Tuning the teacher model on labeled data at {}-th self-training iteration. *".format(iter))
+ # logger.info("*"*80)
+ # print("*"*80)
+ # print("* Tuning the teacher model on labeled data at {}-th self-training iteration. *".format(iter))
+ # print("*"*80)
+
+ # teacher_model = self.freeze_backbone(teacher_model, use_pe=True)
+ # # teacher_trainer: TeacherTrainer = self.get_teacher_trainer(base_model=teacher_model, num_train_epochs=self.teacher_tuning_epoch)
+ # teacher_trainer.train()
+ # teacher_model.load_state_dict(torch.load(os.path.join(teacher_trainer.state.best_model_checkpoint, "pytorch_model.bin")))
+ # teacher_trainer.model = teacher_model
+
+ # Teacher模型在unlabeled data上获取pseudo-labeled data,并根据uncertainty estimation进行采样
+ logger.info("*"*72)
+ logger.info("Obtaining pseudo-labeled data and uncertainty estimation via MC dropout.")
+ logger.info("*"*72)
+ print("*"*72)
+ print("Obtaining pseudo-labeled data and uncertainty estimation via MC dropout.")
+ print("*"*72)
+
+ unlabeled_dataset, y_mean, y_var, y_pred, y_T = teacher_trainer.mc_evaluate(
+ unlabeled_dataset=self.unlabeled_dataset,
+ unlabeled_data_num=self.unlabeled_data_num,
+ T=20,
+ num_classes=self.num_classes
+ )
+
+ logger.info("*"*42)
+ logger.info("* Sampling reliable pseudo-labeled data. *")
+ logger.info("*"*42)
+ print("*"*42)
+ print("* Sampling reliable pseudo-labeled data. *")
+ print("*"*42)
+
+ X_batch, y_batch, _ = sample_by_bald_class_easiness(
+ tokenizer=self.tokenizer,
+ X=unlabeled_dataset,
+ y_mean=y_mean,
+ y_var=y_var,
+ y=y_pred,
+ num_samples=int(y_pred.shape[0] * self.pseudo_sample_num_or_ratio) if self.pseudo_sample_num_or_ratio <= 1.0 else int(self.pseudo_sample_num_or_ratio),
+ num_classes=self.num_classes,
+ y_T=y_T)
+ pseudo_labeled_examples = X_batch
+ pseudo_labeled_examples["label"] = y_batch
+
+ # 生成pseudo-labeled dataset,并与labeled data混合
+ # pseudo_labeled_dataset = DatasetDict()
+ pseudo_labeled_dataset = DatasetK.from_dict(pseudo_labeled_examples)
+ for i in range(len(self.train_dataset)):
+ pseudo_labeled_dataset = pseudo_labeled_dataset.add_item(self.train_dataset[i])
+
+ # 初始化一个新的Student模型,并让Student模型在pseudo-labeled data上进行鲁棒学习
+ logger.info("*"*56)
+ logger.info("* Training a new student model on pseudo-labeled data. *")
+ logger.info("*"*56)
+ print("*"*56)
+ print("* Training a new student model on pseudo-labeled data. *")
+ print("*"*56)
+
+ student_model = self.student_base_model
+ student_model = self.freeze_backbone(student_model, use_pe=True)
+ student_trainer: RobustTrainer = self.get_student_trainer(
+ base_model=student_model,
+ num_train_epochs=self.student_training_epoch,
+ student_learning_rate=self.student_learning_rate,
+ pseudo_labeled_dataset=pseudo_labeled_dataset,
+ output_dir=os.path.join(self.output_dir, "iteration", "student_iter_{}".format(iter))
+ )
+ student_trainer.train()
+ student_model.load_state_dict(torch.load(os.path.join(student_trainer.state.best_model_checkpoint, "pytorch_model.bin")))
+
+ # 将Student模型参数赋给Teacher,作为下一轮训练的Teacher初始化
+ logger.info("*"*64)
+ logger.info("* Initializing a new teacher model from trained student model. *")
+ logger.info("*"*64)
+ print("*"*64)
+ print("* Initializing a new teacher model from trained student model. *")
+ print("*"*64)
+ teacher_model = student_model
+ # teacher_trainer = student_trainer
+ teacher_trainer: TeacherTrainer = self.get_teacher_trainer(
+ base_model=student_model,
+ num_train_epochs=self.teacher_tuning_epoch,
+ output_dir=os.path.join(self.output_dir, "iteration", "teacher_iter_{}".format(iter))
+ )
+
+
+
+
+ logger.info("********** Finishing Self-training **********")
+ logger.info("The best teacher model at {}-th self-training iteration.".format(best_self_training_iteration))
+ logger.info("The best teacher model testing result is {}.".format(best_test_metric))
+ print("********** Finishing Self-training **********")
+ print("The best teacher model at {}-th self-training iteration.".format(best_self_training_iteration))
+ print("The best teacher model testing result is {}.".format(best_test_metric))
+
+
+ # 根据当前最好的Teacher模型,在全部的unlabeled data上打伪标签,并进行mc dropout(样本数量最多不超过50000)
+ if self.semi_training_args.post_student_train:
+
+ logger.info("********** Post training **********")
+ print("********** Post training **********")
+
+ teacher_trainer: TeacherTrainer = self.get_teacher_trainer(
+ base_model=best_teacher_model,
+ num_train_epochs=self.teacher_tuning_epoch,
+ output_dir=os.path.join(self.output_dir, "teacher_iter_post")
+ )
+
+ unlabeled_dataset, y_mean, y_var, y_pred, y_T = teacher_trainer.mc_evaluate(
+ unlabeled_dataset=self.unlabeled_dataset,
+ unlabeled_data_num=20480,
+ T=5,
+ num_classes=self.num_classes
+ )
+
+ post_sample_num = int(y_pred.shape[0] * 0.5)
+
+ X_batch, y_batch, _ = sample_by_bald_class_easiness(
+ tokenizer=self.tokenizer,
+ X=unlabeled_dataset,
+ y_mean=y_mean,
+ y_var=y_var,
+ y=y_pred,
+ num_samples=post_sample_num,
+ num_classes=self.num_classes,
+ y_T=y_T)
+ pseudo_labeled_examples = X_batch
+ pseudo_labeled_examples["label"] = y_batch
+ # 生成pseudo-labeled dataset
+ # pseudo_labeled_dataset = DatasetDict()
+ pseudo_labeled_dataset = DatasetK.from_dict(pseudo_labeled_examples)
+
+
+ # 初始化一个新的Student模型,并让Student模型在pseudo-labeled data上进行鲁棒学习
+ logger.info("*"*56)
+ logger.info("* Training a new student model on pseudo-labeled data. *")
+ logger.info("*"*56)
+ print("*"*56)
+ print("* Training a new student model on pseudo-labeled data. *")
+ print("*"*56)
+
+ student_model = self.student_base_model
+ student_model = self.freeze_backbone(student_model, use_pe=True)
+ student_trainer: RobustTrainer = self.get_student_trainer(
+ base_model=student_model,
+ num_train_epochs=self.student_training_epoch if len(pseudo_labeled_dataset) <= 4096 else int(self.student_training_epoch / 2),
+ student_learning_rate=self.student_learning_rate,
+ pseudo_labeled_dataset=pseudo_labeled_dataset,
+ output_dir=os.path.join(self.output_dir, "student_iter_{}".format(iter))
+ )
+ student_trainer.train()
+ student_model.load_state_dict(torch.load(os.path.join(student_trainer.state.best_model_checkpoint, "pytorch_model.bin")))
+
+ metrics = student_trainer.evaluate()
+ post_metric = metrics["eval_{}".format(self.test_key)]
+
+
+ print("*"*68)
+ print("Finishing all the processes, the results are shown in the following:")
+ print("Conventional fine-tuning {} metric: {}".format(self.test_key, convention_result))
+ print("Best self-training {} metric: {}".format(self.test_key, best_test_metric))
+ if self.semi_training_args.post_student_train:
+ print("Post training {} metric: {}".format(self.test_key, post_metric))
+ print("*"*68)
+
+ return TrainOutput(teacher_trainer.state.global_step, 0.0, metrics)
\ No newline at end of file
diff --git a/training/self_trainer_copy.py b/training/self_trainer_copy.py
new file mode 100644
index 0000000..5b9094c
--- /dev/null
+++ b/training/self_trainer_copy.py
@@ -0,0 +1,583 @@
+import os
+from typing import Dict, OrderedDict
+from tqdm import tqdm
+import torch
+import torch.nn as nn
+from typing import Union, Optional, Callable, List, Tuple
+from transformers import Trainer
+import datasets
+import numpy as np
+from typing import Optional, List
+from datasets import Dataset, DatasetInfo, NamedSplit, DatasetDict
+from datasets.table import Table, list_table_cache_files
+from torch.utils.data import RandomSampler, DistributedSampler, DataLoader
+from transformers import PreTrainedModel, DataCollator, PreTrainedTokenizerBase, EvalPrediction, TrainerCallback
+from transformers.trainer_pt_utils import DistributedSamplerWithLoop, get_length_grouped_indices
+from transformers.trainer_pt_utils import DistributedLengthGroupedSampler as DistributedLengthGroupedSamplerOri
+from transformers.trainer_pt_utils import LengthGroupedSampler as LengthGroupedSamplerOri
+# from transformers.trainer_utils import has_length
+from transformers.training_args import ParallelMode
+from transformers.trainer_pt_utils import (
+ DistributedTensorGatherer,
+ SequentialDistributedSampler,
+ nested_concat,
+ )
+from transformers.utils import logging
+from transformers.trainer_utils import denumpify_detensorize, TrainOutput
+from training.sampler import sample_by_bald_class_easiness
+from training.trainer_base import BaseTrainer
+
+
+logger = logging.get_logger('Self-training')
+
+
+class DatasetK(Dataset):
+ def __init__(
+ self,
+ arrow_table: Table,
+ info: Optional[DatasetInfo] = None,
+ split: Optional[NamedSplit] = None,
+ indices_table: Optional[Table] = None,
+ fingerprint: Optional[str] = None,
+ ):
+ self.custom_cache_files = None
+ super(DatasetK, self).__init__(arrow_table, info, split, indices_table, fingerprint)
+
+
+ @property
+ def cache_files(self) -> List[dict]:
+ """The cache files containing the Apache Arrow table backing the dataset."""
+ if self.custom_cache_files:
+ return self.custom_cache_files
+ cache_files = list_table_cache_files(self._data)
+ if self._indices is not None:
+ cache_files += list_table_cache_files(self._indices)
+ return [{"filename": cache_filename} for cache_filename in cache_files]
+
+ def set_cache_files(self, custom_cache_files):
+ self.custom_cache_files = custom_cache_files
+
+
+# add by wjn
+def random_sampling(raw_datasets, num_examples_per_label: Optional[int]=16):
+ label_list = raw_datasets["label"] # [0, 1, 0, 0, ...]
+ label_dict = dict()
+ # 记录每个label对应的样本索引
+ for ei, label in enumerate(label_list):
+ if label not in label_dict.keys():
+ label_dict[label] = list()
+ label_dict[label].append(ei)
+ # 对于每个类别,随机采样k个样本
+ few_example_ids = list()
+ for label, eid_list in label_dict.items():
+ # examples = deepcopy(eid_list)
+ # shuffle(examples)
+ idxs = np.random.choice(len(eid_list), size=num_examples_per_label, replace=False)
+ selected_eids = [eid_list[i] for i in idxs]
+ few_example_ids.extend(selected_eids)
+ return few_example_ids
+
+class TeacherTrainer(BaseTrainer):
+ def __init__(
+ self,
+ model: Union[PreTrainedModel, nn.Module] = None,
+ args = None,
+ data_collator: Optional[DataCollator] = None,
+ train_dataset: Optional[Dataset] = None,
+ eval_dataset: Optional[Dataset] = None,
+ tokenizer: Optional[PreTrainedTokenizerBase] = None,
+ model_init: Callable[[], PreTrainedModel] = None,
+ compute_metrics: Optional[Callable[[EvalPrediction], Dict]] = None,
+ callbacks: Optional[List[TrainerCallback]] = None,
+ optimizers: Tuple[torch.optim.Optimizer, torch.optim.lr_scheduler.LambdaLR] = (None, None),
+ test_key: str = "accuracy"
+ ):
+ super(TeacherTrainer, self).__init__(model, args, data_collator, train_dataset, eval_dataset, tokenizer, model_init, compute_metrics, callbacks, optimizers)
+ self.predict_dataset = eval_dataset
+ self.test_key = test_key
+ # if self.args.do_adv:
+ # self.fgm = FGM(self.model)
+ # for callback in callbacks:
+ # callback.trainer = self
+ self.best_metrics = OrderedDict({
+ "best_epoch": 0,
+ f"best_eval_{self.test_key}": 0,
+ })
+ self.global_step_ = 0
+
+
+ def mc_evaluate(
+ self,
+ unlabeled_dataset: Optional[Dataset] = None,
+ unlabeled_data_num: int = -1,
+ description: str = "Evaluate on Unlabeled Data via MC Dropout Uncertainty Estimation",
+ prediction_loss_only: Optional[bool] = None,
+ ignore_keys: Optional[List[str]] = None,
+ metric_key_prefix: str = "eval",
+ T: int = 30,
+ num_classes: int = 0
+ ):
+ """
+ Prediction/evaluation loop, shared by `Trainer.evaluate()` and `Trainer.predict()`.
+
+ Works both with or without labels.
+ """
+ args = self.args
+
+ prediction_loss_only = prediction_loss_only if prediction_loss_only is not None else args.prediction_loss_only
+ is_sample = True
+ if unlabeled_data_num == -1 or unlabeled_data_num >= len(unlabeled_dataset):
+ unlabeled_data_num = len(unlabeled_dataset)
+ is_sample = False
+
+ if is_sample:
+ recalled_examples_idx_list = random_sampling(
+ raw_datasets=unlabeled_dataset,
+ num_examples_per_label=unlabeled_data_num // num_classes
+ )
+ unlabeled_dataset = unlabeled_dataset.select(recalled_examples_idx_list)
+ unlabeled_data_num = len(unlabeled_dataset)
+
+ unlabeled_dataloader = self.get_eval_dataloader(unlabeled_dataset)
+ model = self._wrap_model(self.model, training=True, dataloader=unlabeled_dataloader) # reset training to True
+
+ batch_size = unlabeled_dataloader.batch_size
+ # unlabeled_data_num = self.num_examples(unlabeled_dataloader)
+ logger.info(f"***** Running {description} *****")
+ logger.info(f" Num examples = {unlabeled_data_num}")
+ logger.info(f" Batch size = {batch_size}")
+
+ # world_size = max(1, args.world_size)
+
+ # if not prediction_loss_only:
+ # # The actual number of eval_sample can be greater than num_examples in distributed settings (when we pass
+ # # a batch size to the sampler)
+ # make_multiple_of = None
+ # if hasattr(unlabeled_dataloader, "sampler") and isinstance(unlabeled_dataloader.sampler, SequentialDistributedSampler):
+ # make_multiple_of = unlabeled_dataloader.sampler.batch_size
+
+ model.train() # 开启train模式,允许模型进行Dropout
+
+ if args.past_index >= 0:
+ self._past = None
+
+ self.callback_handler.eval_dataloader = unlabeled_dataloader
+
+ # y_T = np.zeros((T, unlabeled_data_num, num_classes))
+ y_T = list()
+
+ for i in tqdm(range(T)):
+ y_pred = []
+
+ for step, inputs in enumerate(unlabeled_dataloader):
+ _, logits, __ = self.prediction_step(model, inputs, prediction_loss_only, ignore_keys=ignore_keys)
+ y_pred.extend(logits.detach().cpu().numpy().tolist())
+ # print("y_pred.shape=", torch.Tensor(y_pred).shape) # [n, num_class]
+ predict_proba = torch.softmax(torch.Tensor(y_pred).to(logits.device), -1)
+ # print("predict_proba.shape=", predict_proba.shape) # [n, num_class]
+ # y_T[i] = predict_proba.detach().cpu().numpy().tolist()
+ y_T.append(predict_proba.detach().cpu().numpy().tolist())
+
+ y_T = np.array(y_T)
+ #compute mean
+ y_mean = np.mean(y_T, axis=0)
+ # print("y_mean.shape=", y_mean.shape) # e.g., (4095, 3) [n, class_num]
+ # print("(unlabeled_data_num, num_classes)=", (unlabeled_data_num, num_classes))
+ assert y_mean.shape == (unlabeled_data_num, num_classes)
+
+ #compute majority prediction
+ y_pred = np.array([np.argmax(np.bincount(row)) for row in np.transpose(np.argmax(y_T, axis=-1))])
+ assert y_pred.shape == (unlabeled_data_num,)
+
+ #compute variance
+ y_var = np.var(y_T, axis=0)
+ assert y_var.shape == (unlabeled_data_num, num_classes)
+
+ return unlabeled_dataset, y_mean, y_var, y_pred, y_T
+
+
+
+class RobustTrainer(TeacherTrainer):
+ def __init__(
+ self,
+ model: Union[PreTrainedModel, nn.Module] = None,
+ args = None,
+ data_collator: Optional[DataCollator] = None,
+ train_dataset: Optional[Dataset] = None,
+ eval_dataset: Optional[Dataset] = None,
+ tokenizer: Optional[PreTrainedTokenizerBase] = None,
+ model_init: Callable[[], PreTrainedModel] = None,
+ compute_metrics: Optional[Callable[[EvalPrediction], Dict]] = None,
+ callbacks: Optional[List[TrainerCallback]] = None,
+ optimizers: Tuple[torch.optim.Optimizer, torch.optim.lr_scheduler.LambdaLR] = (None, None),
+ test_key: str = "accuracy"
+ ):
+ super(RobustTrainer, self).__init__(model, args, data_collator, train_dataset, eval_dataset, tokenizer, model_init, compute_metrics, callbacks, optimizers)
+ self.predict_dataset = eval_dataset
+ self.test_key = test_key
+ # if self.args.do_adv:
+ # self.fgm = FGM(self.model)
+ # for callback in callbacks:
+ # callback.trainer = self
+ self.best_metrics = OrderedDict({
+ "best_epoch": 0,
+ f"best_eval_{self.test_key}": 0,
+ })
+ self.global_step_ = 0
+
+ def robust_train(self):
+ pass
+
+
+
+class SelfTrainer(object):
+ def __init__(
+ self,
+ base_mode: torch.nn.Module,
+ training_args,
+ semi_training_args,
+ train_dataset: Optional[Dataset]=None,
+ unlabeled_dataset: Optional[Dataset]=None,
+ eval_dataset=None,
+ compute_metrics=None,
+ tokenizer=None,
+ teacher_data_collator=None,
+ student_data_collator=None,
+ test_key=None,
+ task_type="cls",
+ num_classes=0,
+ ) -> None:
+
+ logger.info("This is a Self-trainer.")
+
+ self.base_model = base_mode
+ self.training_args = training_args
+ self.semi_training_args = semi_training_args
+ self.train_dataset = train_dataset
+ self.unlabeled_dataset = unlabeled_dataset
+ self.eval_dataset = eval_dataset
+ self.compute_metrics = compute_metrics
+ self.tokenizer = tokenizer
+ self.teacher_data_collator = teacher_data_collator
+ self.student_data_collator = student_data_collator
+ self.test_key = test_key
+ self.task_type = task_type
+ self.num_classes = num_classes
+
+ # self.set_teacher_trainer()
+ # self.set_student_trainer()
+ self.training_args.per_device_train_batch_size = self.semi_training_args.unlabeled_data_batch_size
+ self.teacher_training_epoch = self.semi_training_args.teacher_training_epoch # 最初teacher模型在labeled data上训练的epoch数
+ self.teacher_tuning_epoch = self.semi_training_args.teacher_tuning_epoch # 每一轮Self-training时,teacher模型继续在labeled data上tune的epoch数
+ self.student_training_epoch = self.semi_training_args.student_training_epoch # 每一轮Self-training时,student模型在pseudo-labeled data上训练的epoch数
+ self.self_training_epoch = self.semi_training_args.self_training_epoch # Self-training迭代数
+ self.unlabeled_data_num = self.semi_training_args.unlabeled_data_num # self-training每轮迭代时,首先挑选一部分用于计算MC dropout uncertainty。-1表示全部计算uncertainty
+ self.pseudo_sample_num_or_ratio = self.semi_training_args.pseudo_sample_num_or_ratio # MC dropout后,从所有计算过uncertainty的unlabeled data上采样的样本比例/数量
+ self.student_learning_rate = self.semi_training_args.student_learning_rate
+ self.output_dir = self.training_args.output_dir
+
+ def get_teacher_trainer(
+ self,
+ base_model: torch.nn.Module,
+ num_train_epochs: int,
+ output_dir: str = None,
+ ):
+ training_args = self.training_args
+ training_args.num_train_epochs = num_train_epochs
+ if output_dir is not None:
+ training_args.output_dir = output_dir
+ # 初始化Teacher训练器
+ teacher_trainer = TeacherTrainer(
+ model=base_model,
+ args=training_args,
+ train_dataset=self.train_dataset if self.training_args.do_train else None,
+ eval_dataset=self.eval_dataset if self.training_args.do_eval else None,
+ compute_metrics=self.compute_metrics,
+ tokenizer=self.tokenizer,
+ data_collator=self.teacher_data_collator,
+ test_key=self.test_key,
+ )
+ return teacher_trainer
+
+
+ def get_student_trainer(
+ self,
+ base_model: torch.nn.Module,
+ num_train_epochs: int,
+ student_learning_rate: float,
+ pseudo_labeled_dataset: Optional[Dataset] = None,
+ output_dir: str = None,
+ ):
+ training_args = self.training_args
+ training_args.num_train_epochs = num_train_epochs
+ training_args.learning_rate = student_learning_rate
+ if output_dir is not None:
+ training_args.output_dir = output_dir
+ # 初始化Student训练器
+ student_trainer = RobustTrainer(
+ model=base_model,
+ args=training_args,
+ train_dataset=pseudo_labeled_dataset,
+ eval_dataset=self.eval_dataset,
+ compute_metrics=self.compute_metrics,
+ tokenizer=self.tokenizer,
+ data_collator=self.student_data_collator,
+ test_key=self.test_key,
+ )
+ return student_trainer
+
+ def freeze_backbone(self, model: torch.nn.Module, use_pe: bool=False):
+ try:
+ model.freeze_backbone(use_pe=use_pe)
+ except:
+ pass
+ return model
+
+
+ def train(self, resume_from_checkpoint=None):
+ # 首先对Teacher模型在labeled data上进行full parameter fine-tuning
+ logger.info("*"*66)
+ logger.info("* Training teacher model over labeled data before self-training. *")
+ logger.info("*"*66)
+ print("*"*66)
+ print("* Training teacher model over labeled data before self-training. *")
+ print("*"*66)
+
+ teacher_model = self.base_model
+ teacher_model = self.freeze_backbone(teacher_model, use_pe=False)
+ teacher_trainer: TeacherTrainer = self.get_teacher_trainer(base_model=teacher_model, num_train_epochs=self.teacher_training_epoch)
+ if resume_from_checkpoint is not None:
+ teacher_trainer._load_from_checkpoint(resume_from_checkpoint)
+ teacher_trainer.train()
+ teacher_model.load_state_dict(torch.load(os.path.join(teacher_trainer.state.best_model_checkpoint, "pytorch_model.bin")))
+ teacher_trainer.model = teacher_model
+
+ # 原始的训练结果
+ metrics = teacher_trainer.evaluate()
+ convention_result = metrics["eval_{}".format(self.test_key)]
+
+ logger.info("*"*50)
+ logger.info("* Conventional fine-tuning metric: {}. *".format(convention_result))
+ logger.info("*"*50)
+ print("*"*50)
+ print("* Conventional fine-tuning metric: {}. *".format(convention_result))
+ print("*"*50)
+
+ logger.info("*"*30)
+ logger.info("* Starting Self-training ... *")
+ logger.info("*"*30)
+ print("*"*30)
+ print("* Starting Self-training ... *")
+ print("*"*30)
+
+ best_test_metric = None
+ best_self_training_iteration = None
+ best_teacher_model = None
+
+ # 多轮Teacher-Student迭代训练
+ for iter in range(self.self_training_epoch):
+
+ logger.info("*"*34)
+ logger.info("* Self-training {}-th iteration *".format(iter))
+ logger.info("*"*34)
+ print("*"*34)
+ print("* Self-training {}-th iteration *".format(iter))
+ print("*"*34)
+
+ # if iter == self.self_training_epoch - 1:
+ # break
+
+
+ # # Teacher模型在labeled data上进行parameter-efficient tuning
+ # if iter > 0:
+ # logger.info("*"*80)
+ # logger.info("* Tuning the teacher model on labeled data at {}-th self-training iteration. *".format(iter))
+ # logger.info("*"*80)
+ # print("*"*80)
+ # print("* Tuning the teacher model on labeled data at {}-th self-training iteration. *".format(iter))
+ # print("*"*80)
+
+ # teacher_model = self.freeze_backbone(teacher_model, use_pe=True)
+ # # teacher_trainer: TeacherTrainer = self.get_teacher_trainer(base_model=teacher_model, num_train_epochs=self.teacher_tuning_epoch)
+ # teacher_trainer.train()
+ # teacher_model.load_state_dict(torch.load(os.path.join(teacher_trainer.state.best_model_checkpoint, "pytorch_model.bin")))
+ # teacher_trainer.model = teacher_model
+
+ # Teacher模型在unlabeled data上获取pseudo-labeled data,并根据uncertainty estimation进行采样
+ logger.info("*"*72)
+ logger.info("Obtaining pseudo-labeled data and uncertainty estimation via MC dropout.")
+ logger.info("*"*72)
+ print("*"*72)
+ print("Obtaining pseudo-labeled data and uncertainty estimation via MC dropout.")
+ print("*"*72)
+
+ unlabeled_dataset, y_mean, y_var, y_pred, y_T = teacher_trainer.mc_evaluate(
+ unlabeled_dataset=self.unlabeled_dataset,
+ unlabeled_data_num=self.unlabeled_data_num,
+ T=30,
+ num_classes=self.num_classes
+ )
+
+ logger.info("*"*42)
+ logger.info("* Sampling reliable pseudo-labeled data. *")
+ logger.info("*"*42)
+ print("*"*42)
+ print("* Sampling reliable pseudo-labeled data. *")
+ print("*"*42)
+
+ X_batch, y_batch, _ = sample_by_bald_class_easiness(
+ tokenizer=self.tokenizer,
+ X=unlabeled_dataset,
+ y_mean=y_mean,
+ y_var=y_var,
+ y=y_pred,
+ num_samples=int(y_pred.shape[0] * self.pseudo_sample_num_or_ratio) if self.pseudo_sample_num_or_ratio <= 1.0 else int(self.pseudo_sample_num_or_ratio),
+ num_classes=self.num_classes,
+ y_T=y_T)
+ pseudo_labeled_examples = X_batch
+ pseudo_labeled_examples["label"] = y_batch
+
+ # 生成pseudo-labeled dataset,并与labeled data混合
+ # pseudo_labeled_dataset = DatasetDict()
+ pseudo_labeled_dataset = DatasetK.from_dict(pseudo_labeled_examples)
+ for i in range(len(self.train_dataset)):
+ pseudo_labeled_dataset = pseudo_labeled_dataset.add_item(self.train_dataset[i])
+
+ # 初始化一个新的Student模型,并让Student模型在pseudo-labeled data上进行鲁棒学习
+ logger.info("*"*56)
+ logger.info("* Training a new student model on pseudo-labeled data. *")
+ logger.info("*"*56)
+ print("*"*56)
+ print("* Training a new student model on pseudo-labeled data. *")
+ print("*"*56)
+
+ student_model = self.base_model
+ student_model = self.freeze_backbone(student_model, use_pe=True)
+ student_trainer: RobustTrainer = self.get_student_trainer(
+ base_model=self.base_model,
+ num_train_epochs=self.student_training_epoch,
+ student_learning_rate=self.student_learning_rate,
+ pseudo_labeled_dataset=pseudo_labeled_dataset,
+ output_dir=os.path.join(self.output_dir, "student_iter_{}".format(iter))
+ )
+ student_trainer.train()
+ student_model.load_state_dict(torch.load(os.path.join(student_trainer.state.best_model_checkpoint, "pytorch_model.bin")))
+
+ # 将Student模型参数赋给Teacher,作为下一轮训练的Teacher初始化
+ logger.info("*"*64)
+ logger.info("* Initializing a new teacher model from trained student model. *")
+ logger.info("*"*64)
+ print("*"*64)
+ print("* Initializing a new teacher model from trained student model. *")
+ print("*"*64)
+ teacher_model = student_model
+ teacher_trainer = student_trainer
+ # teacher_trainer: TeacherTrainer = self.get_teacher_trainer(
+ # base_model=student_model,
+ # num_train_epochs=self.teacher_tuning_epoch,
+ # output_dir=os.path.join(self.output_dir, "teacher_iter_{}".format(iter))
+ # )
+
+
+ # 获得Teacher模型在测试集上的效果
+ # teacher_trainer.model = teacher_model
+ metrics = teacher_trainer.evaluate()
+ # print("metrics=", metrics)
+ '''
+ e.g., {'eval_loss': 0.6926815509796143, 'eval_accuracy': 0.5234657039711191, 'eval_runtime': 0.7267, 'eval_samples_per_second': 381.161, 'eval_steps_per_second': 48.161, 'epoch': 1.0}
+ '''
+ logger.info("*"*60)
+ logger.info("* The testing result of teacher model is {} result: {} *".format(self.test_key, metrics["eval_{}".format(self.test_key)]))
+ logger.info("*"*60)
+ print("*"*60)
+ print("* The testing result of teacher model is {} result: {} *".format(self.test_key, metrics["eval_{}".format(self.test_key)]))
+ print("*"*60)
+
+ if best_test_metric is None or best_test_metric < metrics["eval_{}".format(self.test_key)]:
+ best_test_metric = metrics["eval_{}".format(self.test_key)]
+ best_self_training_iteration = iter
+ best_teacher_model = teacher_model
+ logger.info("The best teacher model at {}-th self-training iteration.".format(best_self_training_iteration))
+ logger.info("The best teacher model testing result is {}.".format(best_test_metric))
+ print("The best teacher model at {}-th self-training iteration.".format(best_self_training_iteration))
+ print("The best teacher model testing result is {}.".format(best_test_metric))
+
+
+ logger.info("********** Finishing Self-training **********")
+ logger.info("The best teacher model at {}-th self-training iteration.".format(best_self_training_iteration))
+ logger.info("The best teacher model testing result is {}.".format(best_test_metric))
+ print("********** Finishing Self-training **********")
+ print("The best teacher model at {}-th self-training iteration.".format(best_self_training_iteration))
+ print("The best teacher model testing result is {}.".format(best_test_metric))
+
+
+ # 根据当前最好的Teacher模型,在全部的unlabeled data上打伪标签,并进行mc dropout(样本数量最多不超过50000)
+ if self.semi_training_args.post_student_train:
+
+ logger.info("********** Post training **********")
+ print("********** Post training **********")
+
+ teacher_trainer: TeacherTrainer = self.get_teacher_trainer(
+ base_model=best_teacher_model,
+ num_train_epochs=self.teacher_tuning_epoch,
+ output_dir=os.path.join(self.output_dir, "teacher_iter_post")
+ )
+
+ unlabeled_dataset, y_mean, y_var, y_pred, y_T = teacher_trainer.mc_evaluate(
+ unlabeled_dataset=self.unlabeled_dataset,
+ unlabeled_data_num=20480,
+ T=5,
+ num_classes=self.num_classes
+ )
+
+ post_sample_num = int(y_pred.shape[0] * 0.5)
+
+ X_batch, y_batch, _ = sample_by_bald_class_easiness(
+ tokenizer=self.tokenizer,
+ X=unlabeled_dataset,
+ y_mean=y_mean,
+ y_var=y_var,
+ y=y_pred,
+ num_samples=post_sample_num,
+ num_classes=self.num_classes,
+ y_T=y_T)
+ pseudo_labeled_examples = X_batch
+ pseudo_labeled_examples["label"] = y_batch
+ # 生成pseudo-labeled dataset
+ # pseudo_labeled_dataset = DatasetDict()
+ pseudo_labeled_dataset = DatasetK.from_dict(pseudo_labeled_examples)
+
+
+ # 初始化一个新的Student模型,并让Student模型在pseudo-labeled data上进行鲁棒学习
+ logger.info("*"*56)
+ logger.info("* Training a new student model on pseudo-labeled data. *")
+ logger.info("*"*56)
+ print("*"*56)
+ print("* Training a new student model on pseudo-labeled data. *")
+ print("*"*56)
+
+ student_model = self.base_model
+ student_model = self.freeze_backbone(student_model, use_pe=True)
+ student_trainer: RobustTrainer = self.get_student_trainer(
+ base_model=self.base_model,
+ num_train_epochs=self.student_training_epoch if len(pseudo_labeled_dataset) <= 4096 else int(self.student_training_epoch / 2),
+ student_learning_rate=self.student_learning_rate,
+ pseudo_labeled_dataset=pseudo_labeled_dataset,
+ output_dir=os.path.join(self.output_dir, "student_iter_{}".format(iter))
+ )
+ student_trainer.train()
+ student_model.load_state_dict(torch.load(os.path.join(student_trainer.state.best_model_checkpoint, "pytorch_model.bin")))
+
+ metrics = student_trainer.evaluate()
+ post_metric = metrics["eval_{}".format(self.test_key)]
+
+
+ print("*"*68)
+ print("Finishing all the processes, the results are shown in the following:")
+ print("Conventional fine-tuning {} metric: {}".format(self.test_key, convention_result))
+ print("Best self-training {} metric: {}".format(self.test_key, best_test_metric))
+ if self.semi_training_args.post_student_train:
+ print("Post training {} metric: {}".format(self.test_key, post_metric))
+ print("*"*68)
+
+ return TrainOutput(teacher_trainer.state.global_step, 0.0, metrics)
\ No newline at end of file
diff --git a/training/trainer_base.py b/training/trainer_base.py
new file mode 100644
index 0000000..fc687b0
--- /dev/null
+++ b/training/trainer_base.py
@@ -0,0 +1,72 @@
+import logging
+import os
+from typing import Dict, OrderedDict
+
+from transformers import Trainer
+
+logger = logging.getLogger(__name__)
+
+_default_log_level = logging.INFO
+logger.setLevel(_default_log_level)
+
+class BaseTrainer(Trainer):
+ def __init__(self, *args, predict_dataset = None, test_key = "accuracy", **kwargs):
+ super().__init__(*args, **kwargs)
+ self.predict_dataset = predict_dataset
+ self.test_key = test_key
+ self.best_metrics = OrderedDict({
+ "best_epoch": 0,
+ f"best_eval_{self.test_key}": 0,
+ })
+
+ def log_best_metrics(self):
+ self.log_metrics("best", self.best_metrics)
+ self.save_metrics("best", self.best_metrics, combined=False)
+
+
+
+ def _maybe_log_save_evaluate(self, tr_loss, model, trial, epoch, ignore_keys_for_eval):
+ if self.control.should_log:
+ logs: Dict[str, float] = {}
+
+
+ tr_loss_scalar = self._nested_gather(tr_loss).mean().item()
+
+ # reset tr_loss to zero
+ tr_loss -= tr_loss
+
+ logs["loss"] = round(tr_loss_scalar / (self.state.global_step - self._globalstep_last_logged), 4)
+ logs["learning_rate"] = self._get_learning_rate()
+
+ self._total_loss_scalar += tr_loss_scalar
+ self._globalstep_last_logged = self.state.global_step
+ self.store_flos()
+
+ self.log(logs)
+
+ eval_metrics = None
+ if self.control.should_evaluate:
+ eval_metrics = self.evaluate(ignore_keys=ignore_keys_for_eval)
+ self._report_to_hp_search(trial, epoch, eval_metrics)
+ print("eval_metrics=", eval_metrics)
+ if eval_metrics["eval_"+self.test_key] > self.best_metrics["best_eval_"+self.test_key]:
+ self.best_metrics["best_epoch"] = epoch
+ self.best_metrics["best_eval_"+self.test_key] = eval_metrics["eval_"+self.test_key]
+
+ if self.predict_dataset is not None:
+ if isinstance(self.predict_dataset, dict):
+ for dataset_name, dataset in self.predict_dataset.items():
+ _, _, test_metrics = self.predict(dataset, metric_key_prefix="test")
+ self.best_metrics[f"best_test_{dataset_name}_{self.test_key}"] = test_metrics["test_"+self.test_key]
+ else:
+ _, _, test_metrics = self.predict(self.predict_dataset, metric_key_prefix="test")
+ self.best_metrics["best_test_"+self.test_key] = test_metrics["test_"+self.test_key]
+
+ logger.info(f"***** Epoch {epoch}: Best results *****")
+ for key, value in self.best_metrics.items():
+ logger.info(f"{key} = {value}")
+ self.log(self.best_metrics)
+
+ if self.control.should_save:
+ self._save_checkpoint(model, trial, metrics=eval_metrics)
+ self.control = self.callback_handler.on_save(self.args, self.state, self.control)
diff --git a/training/trainer_exp.py b/training/trainer_exp.py
new file mode 100644
index 0000000..cbbc1aa
--- /dev/null
+++ b/training/trainer_exp.py
@@ -0,0 +1,502 @@
+import logging
+import os
+import random
+import sys
+
+from typing import Any, Dict, List, Optional, OrderedDict, Tuple, Union
+import math
+import random
+import time
+import warnings
+import collections
+
+from transformers.debug_utils import DebugOption, DebugUnderflowOverflow
+from transformers.trainer_callback import TrainerState
+from transformers.trainer_pt_utils import IterableDatasetShard
+from transformers.trainer_utils import (
+ HPSearchBackend,
+ ShardedDDPOption,
+ TrainOutput,
+ get_last_checkpoint,
+ set_seed,
+ speed_metrics,
+)
+from transformers.file_utils import (
+ CONFIG_NAME,
+ WEIGHTS_NAME,
+ is_torch_tpu_available,
+)
+
+import torch
+from torch import nn
+from torch.utils.data import DataLoader
+from torch.utils.data.distributed import DistributedSampler
+
+from training.trainer_base import BaseTrainer, logger
+
+
+class ExponentialTrainer(BaseTrainer):
+ def __init__(self, *args, **kwargs):
+ super().__init__(*args, **kwargs)
+
+ def create_scheduler(self, num_training_steps: int, optimizer: torch.optim.Optimizer = None):
+ if self.lr_scheduler is None:
+ self.lr_scheduler = torch.optim.lr_scheduler.ExponentialLR(self.optimizer, gamma=0.95, verbose=True)
+ return self.lr_scheduler
+
+
+ def train(
+ self,
+ resume_from_checkpoint: Optional[Union[str, bool]] = None,
+ trial: Union["optuna.Trial", Dict[str, Any]] = None,
+ ignore_keys_for_eval: Optional[List[str]] = None,
+ **kwargs,
+ ):
+ """
+ Main training entry point.
+ Args:
+ resume_from_checkpoint (:obj:`str` or :obj:`bool`, `optional`):
+ If a :obj:`str`, local path to a saved checkpoint as saved by a previous instance of
+ :class:`~transformers.Trainer`. If a :obj:`bool` and equals `True`, load the last checkpoint in
+ `args.output_dir` as saved by a previous instance of :class:`~transformers.Trainer`. If present,
+ training will resume from the model/optimizer/scheduler states loaded here.
+ trial (:obj:`optuna.Trial` or :obj:`Dict[str, Any]`, `optional`):
+ The trial run or the hyperparameter dictionary for hyperparameter search.
+ ignore_keys_for_eval (:obj:`List[str]`, `optional`)
+ A list of keys in the output of your model (if it is a dictionary) that should be ignored when
+ gathering predictions for evaluation during the training.
+ kwargs:
+ Additional keyword arguments used to hide deprecated arguments
+ """
+ resume_from_checkpoint = None if not resume_from_checkpoint else resume_from_checkpoint
+
+ # memory metrics - must set up as early as possible
+ self._memory_tracker.start()
+
+ args = self.args
+
+ self.is_in_train = True
+
+ # do_train is not a reliable argument, as it might not be set and .train() still called, so
+ # the following is a workaround:
+ if args.fp16_full_eval and not args.do_train:
+ self._move_model_to_device(self.model, args.device)
+
+ if "model_path" in kwargs:
+ resume_from_checkpoint = kwargs.pop("model_path")
+ warnings.warn(
+ "`model_path` is deprecated and will be removed in a future version. Use `resume_from_checkpoint` "
+ "instead.",
+ FutureWarning,
+ )
+ if len(kwargs) > 0:
+ raise TypeError(f"train() received got unexpected keyword arguments: {', '.join(list(kwargs.keys()))}.")
+ # This might change the seed so needs to run first.
+ self._hp_search_setup(trial)
+
+ # Model re-init
+ model_reloaded = False
+ if self.model_init is not None:
+ # Seed must be set before instantiating the model when using model_init.
+ set_seed(args.seed)
+ self.model = self.call_model_init(trial)
+ model_reloaded = True
+ # Reinitializes optimizer and scheduler
+ self.optimizer, self.lr_scheduler = None, None
+
+ # Load potential model checkpoint
+ if isinstance(resume_from_checkpoint, bool) and resume_from_checkpoint:
+ resume_from_checkpoint = get_last_checkpoint(args.output_dir)
+ if resume_from_checkpoint is None:
+ raise ValueError(f"No valid checkpoint found in output directory ({args.output_dir})")
+
+ if resume_from_checkpoint is not None:
+ if not os.path.isfile(os.path.join(resume_from_checkpoint, WEIGHTS_NAME)):
+ raise ValueError(f"Can't find a valid checkpoint at {resume_from_checkpoint}")
+
+ logger.info(f"Loading model from {resume_from_checkpoint}).")
+
+ if os.path.isfile(os.path.join(resume_from_checkpoint, CONFIG_NAME)):
+ config = PretrainedConfig.from_json_file(os.path.join(resume_from_checkpoint, CONFIG_NAME))
+ checkpoint_version = config.transformers_version
+ if checkpoint_version is not None and checkpoint_version != __version__:
+ logger.warn(
+ f"You are resuming training from a checkpoint trained with {checkpoint_version} of "
+ f"Transformers but your current version is {__version__}. This is not recommended and could "
+ "yield to errors or unwanted behaviors."
+ )
+
+ if args.deepspeed:
+ # will be resumed in deepspeed_init
+ pass
+ else:
+ # We load the model state dict on the CPU to avoid an OOM error.
+ state_dict = torch.load(os.path.join(resume_from_checkpoint, WEIGHTS_NAME), map_location="cpu")
+ # If the model is on the GPU, it still works!
+ self._load_state_dict_in_model(state_dict)
+
+ # release memory
+ del state_dict
+
+ # If model was re-initialized, put it on the right device and update self.model_wrapped
+ if model_reloaded:
+ if self.place_model_on_device:
+ self._move_model_to_device(self.model, args.device)
+ self.model_wrapped = self.model
+
+ # Keeping track whether we can can len() on the dataset or not
+ train_dataset_is_sized = isinstance(self.train_dataset, collections.abc.Sized)
+
+ # Data loader and number of training steps
+ train_dataloader = self.get_train_dataloader()
+
+ # Setting up training control variables:
+ # number of training epochs: num_train_epochs
+ # number of training steps per epoch: num_update_steps_per_epoch
+ # total number of training steps to execute: max_steps
+ total_train_batch_size = args.train_batch_size * args.gradient_accumulation_steps * args.world_size
+ if train_dataset_is_sized:
+ num_update_steps_per_epoch = len(train_dataloader) // args.gradient_accumulation_steps
+ num_update_steps_per_epoch = max(num_update_steps_per_epoch, 1)
+ if args.max_steps > 0:
+ max_steps = args.max_steps
+ num_train_epochs = args.max_steps // num_update_steps_per_epoch + int(
+ args.max_steps % num_update_steps_per_epoch > 0
+ )
+ # May be slightly incorrect if the last batch in the training datalaoder has a smaller size but it's
+ # the best we can do.
+ num_train_samples = args.max_steps * total_train_batch_size
+ else:
+ max_steps = math.ceil(args.num_train_epochs * num_update_steps_per_epoch)
+ num_train_epochs = math.ceil(args.num_train_epochs)
+ num_train_samples = len(self.train_dataset) * args.num_train_epochs
+ else:
+ # see __init__. max_steps is set when the dataset has no __len__
+ max_steps = args.max_steps
+ # Setting a very large number of epochs so we go as many times as necessary over the iterator.
+ num_train_epochs = sys.maxsize
+ num_update_steps_per_epoch = max_steps
+ num_train_samples = args.max_steps * total_train_batch_size
+
+ if DebugOption.UNDERFLOW_OVERFLOW in self.args.debug:
+ if self.args.n_gpu > 1:
+ # nn.DataParallel(model) replicates the model, creating new variables and module
+ # references registered here no longer work on other gpus, breaking the module
+ raise ValueError(
+ "Currently --debug underflow_overflow is not supported under DP. Please use DDP (torch.distributed.launch)."
+ )
+ else:
+ debug_overflow = DebugUnderflowOverflow(self.model) # noqa
+
+ delay_optimizer_creation = self.sharded_ddp is not None and self.sharded_ddp != ShardedDDPOption.SIMPLE
+ if args.deepspeed:
+ deepspeed_engine, optimizer, lr_scheduler = deepspeed_init(
+ self, num_training_steps=max_steps, resume_from_checkpoint=resume_from_checkpoint
+ )
+ self.model = deepspeed_engine.module
+ self.model_wrapped = deepspeed_engine
+ self.deepspeed = deepspeed_engine
+ self.optimizer = optimizer
+ self.lr_scheduler = lr_scheduler
+ elif not delay_optimizer_creation:
+ self.create_optimizer_and_scheduler(num_training_steps=max_steps)
+
+ self.state = TrainerState()
+ self.state.is_hyper_param_search = trial is not None
+
+ # Activate gradient checkpointing if needed
+ if args.gradient_checkpointing:
+ self.model.gradient_checkpointing_enable()
+
+ model = self._wrap_model(self.model_wrapped)
+
+ # for the rest of this function `model` is the outside model, whether it was wrapped or not
+ if model is not self.model:
+ self.model_wrapped = model
+
+ if delay_optimizer_creation:
+ self.create_optimizer_and_scheduler(num_training_steps=max_steps)
+
+ # Check if saved optimizer or scheduler states exist
+ self._load_optimizer_and_scheduler(resume_from_checkpoint)
+
+ # important: at this point:
+ # self.model is the Transformers Model
+ # self.model_wrapped is DDP(Transformers Model), Deepspeed(Transformers Model), etc.
+
+ # Train!
+ num_examples = (
+ self.num_examples(train_dataloader) if train_dataset_is_sized else total_train_batch_size * args.max_steps
+ )
+
+ logger.info("***** Running training *****")
+ logger.info(f" Num examples = {num_examples}")
+ logger.info(f" Num Epochs = {num_train_epochs}")
+ logger.info(f" Instantaneous batch size per device = {args.per_device_train_batch_size}")
+ logger.info(f" Total train batch size (w. parallel, distributed & accumulation) = {total_train_batch_size}")
+ logger.info(f" Gradient Accumulation steps = {args.gradient_accumulation_steps}")
+ logger.info(f" Total optimization steps = {max_steps}")
+
+ self.state.epoch = 0
+ start_time = time.time()
+ epochs_trained = 0
+ steps_trained_in_current_epoch = 0
+ steps_trained_progress_bar = None
+
+ # Check if continuing training from a checkpoint
+ if resume_from_checkpoint is not None and os.path.isfile(
+ os.path.join(resume_from_checkpoint, TRAINER_STATE_NAME)
+ ):
+ self.state = TrainerState.load_from_json(os.path.join(resume_from_checkpoint, TRAINER_STATE_NAME))
+ epochs_trained = self.state.global_step // num_update_steps_per_epoch
+ if not args.ignore_data_skip:
+ steps_trained_in_current_epoch = self.state.global_step % (num_update_steps_per_epoch)
+ steps_trained_in_current_epoch *= args.gradient_accumulation_steps
+ else:
+ steps_trained_in_current_epoch = 0
+
+ logger.info(" Continuing training from checkpoint, will skip to saved global_step")
+ logger.info(f" Continuing training from epoch {epochs_trained}")
+ logger.info(f" Continuing training from global step {self.state.global_step}")
+ if not args.ignore_data_skip:
+ logger.info(
+ f" Will skip the first {epochs_trained} epochs then the first {steps_trained_in_current_epoch} "
+ "batches in the first epoch. If this takes a lot of time, you can add the `--ignore_data_skip` "
+ "flag to your launch command, but you will resume the training on data already seen by your model."
+ )
+ if self.is_local_process_zero() and not args.disable_tqdm:
+ steps_trained_progress_bar = tqdm(total=steps_trained_in_current_epoch)
+ steps_trained_progress_bar.set_description("Skipping the first batches")
+
+ # Update the references
+ self.callback_handler.model = self.model
+ self.callback_handler.optimizer = self.optimizer
+ self.callback_handler.lr_scheduler = self.lr_scheduler
+ self.callback_handler.train_dataloader = train_dataloader
+ self.state.trial_name = self.hp_name(trial) if self.hp_name is not None else None
+ if trial is not None:
+ assignments = trial.assignments if self.hp_search_backend == HPSearchBackend.SIGOPT else trial
+ self.state.trial_params = hp_params(assignments)
+ else:
+ self.state.trial_params = None
+ # This should be the same if the state has been saved but in case the training arguments changed, it's safer
+ # to set this after the load.
+ self.state.max_steps = max_steps
+ self.state.num_train_epochs = num_train_epochs
+ self.state.is_local_process_zero = self.is_local_process_zero()
+ self.state.is_world_process_zero = self.is_world_process_zero()
+
+ # tr_loss is a tensor to avoid synchronization of TPUs through .item()
+ tr_loss = torch.tensor(0.0).to(args.device)
+ # _total_loss_scalar is updated everytime .item() has to be called on tr_loss and stores the sum of all losses
+ self._total_loss_scalar = 0.0
+ self._globalstep_last_logged = self.state.global_step
+ model.zero_grad()
+
+ self.control = self.callback_handler.on_train_begin(args, self.state, self.control)
+
+ # Skip the first epochs_trained epochs to get the random state of the dataloader at the right point.
+ if not args.ignore_data_skip:
+ for epoch in range(epochs_trained):
+ # We just need to begin an iteration to create the randomization of the sampler.
+ for _ in train_dataloader:
+ break
+
+
+ for epoch in range(epochs_trained, num_train_epochs):
+ if isinstance(train_dataloader, DataLoader) and isinstance(train_dataloader.sampler, DistributedSampler):
+ train_dataloader.sampler.set_epoch(epoch)
+ elif isinstance(train_dataloader.dataset, IterableDatasetShard):
+ train_dataloader.dataset.set_epoch(epoch)
+
+ if is_torch_tpu_available():
+ parallel_loader = pl.ParallelLoader(train_dataloader, [args.device]).per_device_loader(args.device)
+ epoch_iterator = parallel_loader
+ else:
+ epoch_iterator = train_dataloader
+
+ # Reset the past mems state at the beginning of each epoch if necessary.
+ if args.past_index >= 0:
+ self._past = None
+
+ steps_in_epoch = (
+ len(epoch_iterator) if train_dataset_is_sized else args.max_steps * args.gradient_accumulation_steps
+ )
+ self.control = self.callback_handler.on_epoch_begin(args, self.state, self.control)
+
+ step = -1
+ for step, inputs in enumerate(epoch_iterator):
+
+ # Skip past any already trained steps if resuming training
+ if steps_trained_in_current_epoch > 0:
+ steps_trained_in_current_epoch -= 1
+ if steps_trained_progress_bar is not None:
+ steps_trained_progress_bar.update(1)
+ if steps_trained_in_current_epoch == 0:
+ self._load_rng_state(resume_from_checkpoint)
+ continue
+ elif steps_trained_progress_bar is not None:
+ steps_trained_progress_bar.close()
+ steps_trained_progress_bar = None
+
+ if step % args.gradient_accumulation_steps == 0:
+ self.control = self.callback_handler.on_step_begin(args, self.state, self.control)
+
+ if (
+ ((step + 1) % args.gradient_accumulation_steps != 0)
+ and args.local_rank != -1
+ and args._no_sync_in_gradient_accumulation
+ ):
+ # Avoid unnecessary DDP synchronization since there will be no backward pass on this example.
+ with model.no_sync():
+ tr_loss_step = self.training_step(model, inputs)
+ else:
+ tr_loss_step = self.training_step(model, inputs)
+
+ if (
+ args.logging_nan_inf_filter
+ and not is_torch_tpu_available()
+ and (torch.isnan(tr_loss_step) or torch.isinf(tr_loss_step))
+ ):
+ # if loss is nan or inf simply add the average of previous logged losses
+ tr_loss += tr_loss / (1 + self.state.global_step - self._globalstep_last_logged)
+ else:
+ tr_loss += tr_loss_step
+
+ self.current_flos += float(self.floating_point_ops(inputs))
+
+ # Optimizer step for deepspeed must be called on every step regardless of the value of gradient_accumulation_steps
+ if self.deepspeed:
+ self.deepspeed.step()
+
+ if (step + 1) % args.gradient_accumulation_steps == 0 or (
+ # last step in epoch but step is always smaller than gradient_accumulation_steps
+ steps_in_epoch <= args.gradient_accumulation_steps
+ and (step + 1) == steps_in_epoch
+ ):
+ # Gradient clipping
+ if args.max_grad_norm is not None and args.max_grad_norm > 0 and not self.deepspeed:
+ # deepspeed does its own clipping
+
+ if self.do_grad_scaling:
+ # AMP: gradients need unscaling
+ self.scaler.unscale_(self.optimizer)
+
+ if hasattr(self.optimizer, "clip_grad_norm"):
+ # Some optimizers (like the sharded optimizer) have a specific way to do gradient clipping
+ self.optimizer.clip_grad_norm(args.max_grad_norm)
+ elif hasattr(model, "clip_grad_norm_"):
+ # Some models (like FullyShardedDDP) have a specific way to do gradient clipping
+ model.clip_grad_norm_(args.max_grad_norm)
+ else:
+ # Revert to normal clipping otherwise, handling Apex or full precision
+ nn.utils.clip_grad_norm_(
+ amp.master_params(self.optimizer) if self.use_apex else model.parameters(),
+ args.max_grad_norm,
+ )
+
+ # Optimizer step
+ optimizer_was_run = True
+ if self.deepspeed:
+ pass # called outside the loop
+ elif is_torch_tpu_available():
+ xm.optimizer_step(self.optimizer)
+ elif self.do_grad_scaling:
+ scale_before = self.scaler.get_scale()
+ self.scaler.step(self.optimizer)
+ self.scaler.update()
+ scale_after = self.scaler.get_scale()
+ optimizer_was_run = scale_before <= scale_after
+ else:
+ self.optimizer.step()
+
+ if optimizer_was_run and not self.deepspeed and (step + 1) == steps_in_epoch:
+ self.lr_scheduler.step()
+
+ model.zero_grad()
+ self.state.global_step += 1
+ self.state.epoch = epoch + (step + 1) / steps_in_epoch
+ self.control = self.callback_handler.on_step_end(args, self.state, self.control)
+
+ self._maybe_log_save_evaluate(tr_loss, model, trial, epoch, ignore_keys_for_eval)
+ else:
+ self.control = self.callback_handler.on_substep_end(args, self.state, self.control)
+
+ if self.control.should_epoch_stop or self.control.should_training_stop:
+ break
+ if step < 0:
+ logger.warning(
+ f"There seems to be not a single sample in your epoch_iterator, stopping training at step"
+ f" {self.state.global_step}! This is expected if you're using an IterableDataset and set"
+ f" num_steps ({max_steps}) higher than the number of available samples."
+ )
+ self.control.should_training_stop = True
+
+ self.control = self.callback_handler.on_epoch_end(args, self.state, self.control)
+ self._maybe_log_save_evaluate(tr_loss, model, trial, epoch, ignore_keys_for_eval)
+
+ if DebugOption.TPU_METRICS_DEBUG in self.args.debug:
+ if is_torch_tpu_available():
+ # tpu-comment: Logging debug metrics for PyTorch/XLA (compile, execute times, ops, etc.)
+ xm.master_print(met.metrics_report())
+ else:
+ logger.warning(
+ "You enabled PyTorch/XLA debug metrics but you don't have a TPU "
+ "configured. Check your training configuration if this is unexpected."
+ )
+ if self.control.should_training_stop:
+ break
+
+
+ if args.past_index and hasattr(self, "_past"):
+ # Clean the state at the end of training
+ delattr(self, "_past")
+
+ logger.info("\n\nTraining completed. Do not forget to share your model on huggingface.co/models =)\n\n")
+ if args.load_best_model_at_end and self.state.best_model_checkpoint is not None:
+ # Wait for everyone to get here so we are sur the model has been saved by process 0.
+ if is_torch_tpu_available():
+ xm.rendezvous("load_best_model_at_end")
+ elif args.local_rank != -1:
+ dist.barrier()
+
+ logger.info(
+ f"Loading best model from {self.state.best_model_checkpoint} (score: {self.state.best_metric})."
+ )
+
+ best_model_path = os.path.join(self.state.best_model_checkpoint, WEIGHTS_NAME)
+ if os.path.exists(best_model_path):
+ # We load the model state dict on the CPU to avoid an OOM error.
+ state_dict = torch.load(best_model_path, map_location="cpu")
+ # If the model is on the GPU, it still works!
+ self._load_state_dict_in_model(state_dict)
+ else:
+ logger.warn(
+ f"Could not locate the best model at {best_model_path}, if you are running a distributed training "
+ "on multiple nodes, you should activate `--save_on_each_node`."
+ )
+
+ if self.deepspeed:
+ self.deepspeed.load_checkpoint(
+ self.state.best_model_checkpoint, load_optimizer_states=False, load_lr_scheduler_states=False
+ )
+
+ # add remaining tr_loss
+ self._total_loss_scalar += tr_loss.item()
+ train_loss = self._total_loss_scalar / self.state.global_step
+
+ metrics = speed_metrics("train", start_time, num_samples=num_train_samples, num_steps=self.state.max_steps)
+ self.store_flos()
+ metrics["total_flos"] = self.state.total_flos
+ metrics["train_loss"] = train_loss
+
+ self.is_in_train = False
+
+ self._memory_tracker.stop_and_update_metrics(metrics)
+
+ self.log(metrics)
+
+ self.control = self.callback_handler.on_train_end(args, self.state, self.control)
+
+
+ return TrainOutput(self.state.global_step, train_loss, metrics)
diff --git a/training/trainer_qa.py b/training/trainer_qa.py
new file mode 100644
index 0000000..65f36b5
--- /dev/null
+++ b/training/trainer_qa.py
@@ -0,0 +1,159 @@
+# coding=utf-8
+# Copyright 2020 The HuggingFace Team All rights reserved.
+#
+# Licensed under the Apache License, Version 2.0 (the "License");
+# you may not use this file except in compliance with the License.
+# You may obtain a copy of the License at
+#
+# http://www.apache.org/licenses/LICENSE-2.0
+#
+# Unless required by applicable law or agreed to in writing, software
+# distributed under the License is distributed on an "AS IS" BASIS,
+# WITHOUT WARRANTIES OR CONDITIONS OF ANY KIND, either express or implied.
+# See the License for the specific language governing permissions and
+# limitations under the License.
+"""
+A subclass of `Trainer` specific to Question-Answering tasks
+"""
+
+from transformers import Trainer, is_torch_tpu_available
+from transformers.trainer_utils import PredictionOutput
+from training.trainer_exp import ExponentialTrainer, logger
+from typing import Dict, OrderedDict
+
+if is_torch_tpu_available():
+ import torch_xla.core.xla_model as xm
+ import torch_xla.debug.metrics as met
+
+
+class QuestionAnsweringTrainer(ExponentialTrainer):
+ def __init__(self, *args, eval_examples=None, post_process_function=None, **kwargs):
+ super().__init__(*args, **kwargs)
+ self.eval_examples = eval_examples
+ self.post_process_function = post_process_function
+ self.best_metrics = OrderedDict({
+ "best_epoch": 0,
+ "best_eval_f1": 0,
+ "best_eval_exact_match": 0,
+ })
+
+ def evaluate(self, eval_dataset=None, eval_examples=None, ignore_keys=None, metric_key_prefix: str = "eval"):
+ eval_dataset = self.eval_dataset if eval_dataset is None else eval_dataset
+ eval_dataloader = self.get_eval_dataloader(eval_dataset)
+ eval_examples = self.eval_examples if eval_examples is None else eval_examples
+
+ # Temporarily disable metric computation, we will do it in the loop here.
+ compute_metrics = self.compute_metrics
+ self.compute_metrics = None
+ eval_loop = self.prediction_loop if self.args.use_legacy_prediction_loop else self.evaluation_loop
+ try:
+ output = eval_loop(
+ eval_dataloader,
+ description="Evaluation",
+ # No point gathering the predictions if there are no metrics, otherwise we defer to
+ # self.args.prediction_loss_only
+ prediction_loss_only=True if compute_metrics is None else None,
+ ignore_keys=ignore_keys,
+ )
+ finally:
+ self.compute_metrics = compute_metrics
+
+ if self.post_process_function is not None and self.compute_metrics is not None:
+ eval_preds = self.post_process_function(eval_examples, eval_dataset, output.predictions)
+ metrics = self.compute_metrics(eval_preds)
+
+ # Prefix all keys with metric_key_prefix + '_'
+ for key in list(metrics.keys()):
+ if not key.startswith(f"{metric_key_prefix}_"):
+ metrics[f"{metric_key_prefix}_{key}"] = metrics.pop(key)
+
+ self.log(metrics)
+ else:
+ metrics = {}
+
+ if self.args.tpu_metrics_debug or self.args.debug:
+ # tpu-comment: Logging debug metrics for PyTorch/XLA (compile, execute times, ops, etc.)
+ xm.master_print(met.metrics_report())
+
+ self.control = self.callback_handler.on_evaluate(self.args, self.state, self.control, metrics)
+ return metrics
+
+ def predict(self, predict_dataset, predict_examples, ignore_keys=None, metric_key_prefix: str = "test"):
+ predict_dataloader = self.get_test_dataloader(predict_dataset)
+
+ # Temporarily disable metric computation, we will do it in the loop here.
+ compute_metrics = self.compute_metrics
+ self.compute_metrics = None
+ eval_loop = self.prediction_loop if self.args.use_legacy_prediction_loop else self.evaluation_loop
+ try:
+ output = eval_loop(
+ predict_dataloader,
+ description="Prediction",
+ # No point gathering the predictions if there are no metrics, otherwise we defer to
+ # self.args.prediction_loss_only
+ prediction_loss_only=True if compute_metrics is None else None,
+ ignore_keys=ignore_keys,
+ )
+ finally:
+ self.compute_metrics = compute_metrics
+
+ if self.post_process_function is None or self.compute_metrics is None:
+ return output
+
+ predictions = self.post_process_function(predict_examples, predict_dataset, output.predictions, "predict")
+ metrics = self.compute_metrics(predictions)
+
+ # Prefix all keys with metric_key_prefix + '_'
+ for key in list(metrics.keys()):
+ if not key.startswith(f"{metric_key_prefix}_"):
+ metrics[f"{metric_key_prefix}_{key}"] = metrics.pop(key)
+
+ return PredictionOutput(predictions=predictions.predictions, label_ids=predictions.label_ids, metrics=metrics)
+
+ def _maybe_log_save_evaluate(self, tr_loss, model, trial, epoch, ignore_keys_for_eval):
+ if self.control.should_log:
+ logs: Dict[str, float] = {}
+
+
+ tr_loss_scalar = self._nested_gather(tr_loss).mean().item()
+
+ # reset tr_loss to zero
+ tr_loss -= tr_loss
+
+ logs["loss"] = round(tr_loss_scalar / (self.state.global_step - self._globalstep_last_logged), 4)
+ logs["learning_rate"] = self._get_learning_rate()
+
+ self._total_loss_scalar += tr_loss_scalar
+ self._globalstep_last_logged = self.state.global_step
+ self.store_flos()
+
+ self.log(logs)
+
+ eval_metrics = None
+ if self.control.should_evaluate:
+ eval_metrics = self.evaluate(ignore_keys=ignore_keys_for_eval)
+ self._report_to_hp_search(trial, epoch, eval_metrics)
+
+ if eval_metrics["eval_f1"] > self.best_metrics["best_eval_f1"]:
+ self.best_metrics["best_epoch"] = epoch
+ self.best_metrics["best_eval_f1"] = eval_metrics["eval_f1"]
+ if "eval_exact_match" in eval_metrics:
+ self.best_metrics["best_eval_exact_match"] = eval_metrics["eval_exact_match"]
+ if "eval_exact" in eval_metrics:
+ self.best_metrics["best_eval_exact_match"] = eval_metrics["eval_exact"]
+
+
+ logger.info(f"\n***** Epoch {epoch}: Best results *****")
+ for key, value in self.best_metrics.items():
+ logger.info(f"{key} = {value}")
+ self.log(self.best_metrics)
+
+ if self.control.should_save:
+ self._save_checkpoint(model, trial, metrics=eval_metrics)
+ self.control = self.callback_handler.on_save(self.args, self.state, self.control)
+
+ def log_best_metrics(self):
+ best_metrics = OrderedDict()
+ for key, value in self.best_metrics.items():
+ best_metrics[f"best_{key}"] = value
+ self.log_metrics("best", best_metrics)
\ No newline at end of file