-
Notifications
You must be signed in to change notification settings - Fork 99
New issue
Have a question about this project? Sign up for a free GitHub account to open an issue and contact its maintainers and the community.
By clicking “Sign up for GitHub”, you agree to our terms of service and privacy statement. We’ll occasionally send you account related emails.
Already on GitHub? Sign in to your account
Processing large Whole Slide Images with MESMER- Potential memory issues? #582
Comments
Hi rjesud, One notable difference between your data and the benchmarks described in #553 is that you specify
|
There's indeed a post-processing step that operates across the entire image. I'm guessing for something this large, it will make more sense to generate segmentation predictions on large patches (say 10k x 10k pixels), and then stitch them together into a single image. This isn't something that's currently supported by the |
Thank you for the suggestion.
@ngreenwald, tile/patch-wise post processing would be great! Can we add this as a feature request? |
Absolutely, feel free to open a separate detailing the requirements and what an ideal interface would look like. No promises on having something immediately ready. In the meantime, something like this should work for you:
|
@ngreenwald Thanks for this snippet! I'll give it a try. We'll probably have to get fancy with tile overlaps to solve the border cell issue. |
Yes, although with 10k x 10k crops, the number of cells at the border would quite a small overall percentage of the image, might be fine to just leave them as is. Up to you of course. |
Hi, I have a follow up question. Is the predict function executing normalization of the image array prior to inference? If so, is it possible to override this step and perform it outside of the function. This would be the preferred method to ensure each tile is using the same normalization scaling. |
Yes, you can pass kwarg to the function to control whether normalization happens or not, see here. However, I would verify that you see a decrease in performance before substituting your own normalization. The normalization we're using, CLAHE, already adjusts across the image to some degree, so I don't know that it will necessarily cause issues to have it applied to different tiles, especially tiles as large as 10k x 10k. The only area where I've seen issues before is if entire tiles are devoid of signal, and it's just normalizing background to background. |
Hi,
Thanks for developing this useful tool! I’m eager to apply it in our research.
I am encountering what I believe is an out of memory crash when processing a large digital pathology whole slide image (WSI). This specific image Is 76286 x 44944 (XY) at .325 microns/pixel. While this is large, it is not atypical in our WSI datasets. Other images in our dataset can be larger.
I have done benchmarking with the data and workflow described in another ticket here: #553. I can confirm results and processing time (6min) as shown here: #553 (comment). So it appears things are working as expected.
So, I am not sure where I am hitting an issue with my image. I assumed that the image was being sent to GPU in memory-efficient batches. Perhaps this is occurring at the pre-processing or post-processing stage? Should I explore a different workflow for Mesmer usage with images of this size?
I am using:
Deep-cell 0.11.0
Tensorflow 2.5.1
Cudnn 8.2.1
Cudotoolkit 11.3.1
GPU: Quadro P6000 computeCapability: 6.1
coreClock: 1.645GHz coreCount: 30 deviceMemorySize: 23.88GiB deviceMemoryBandwidth: 403.49GiB/s
CPU: 16 cores, 128 GB RAM
Seems to be talking to GPU:
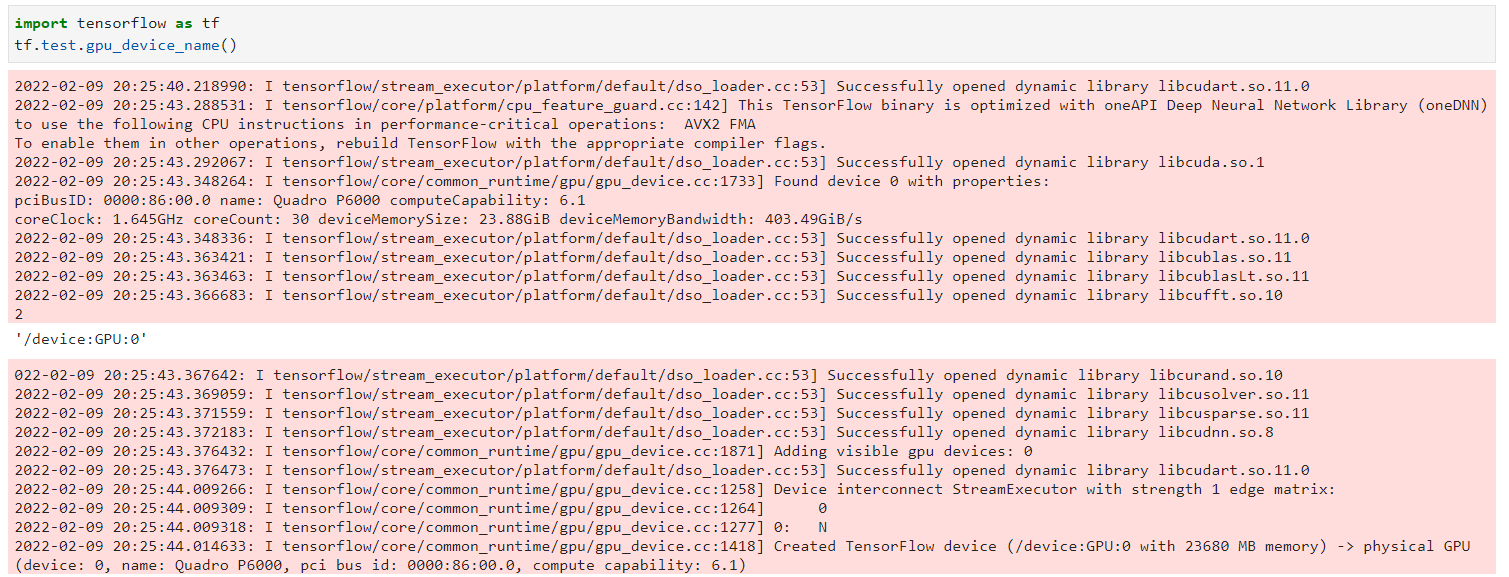
Unfortunately, does not complete:
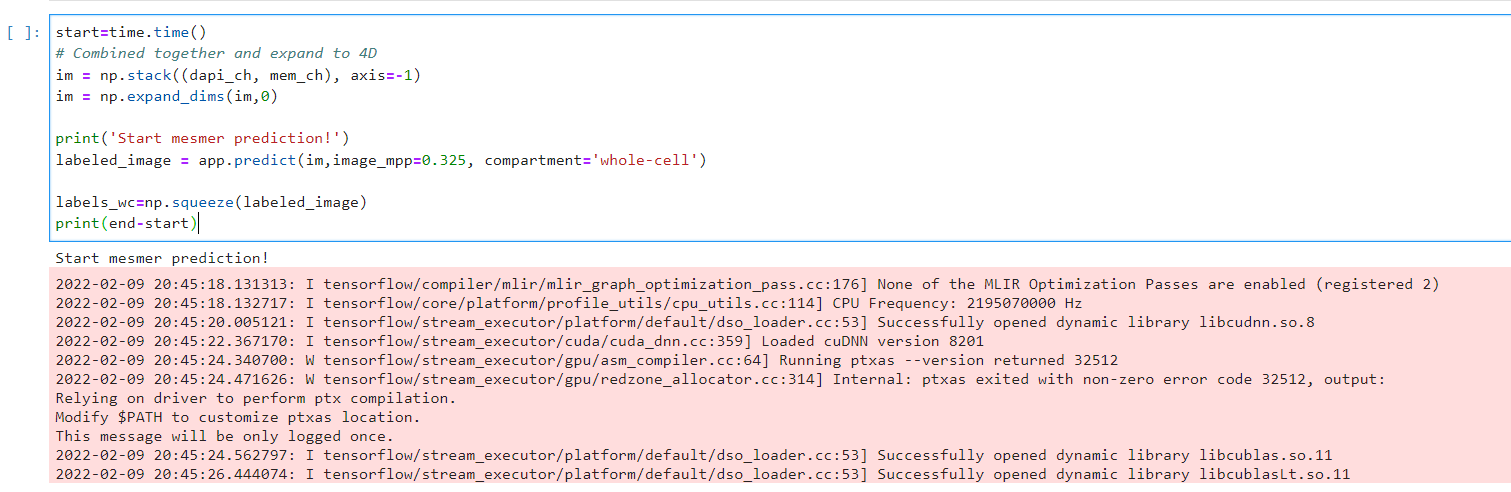
The text was updated successfully, but these errors were encountered: