This repository is based on ultralytics/yolov5. As per the Official Readme file from Ultralytics, YOLOV5 is a family of object detectors with the following major differences from YOLOV3:
- Darknet-csp backbone instead of vanilla Darknet. Reduces complexity by 30%.
- PANet feature extractor instead of FPN.
- Better box-decoding technique
- Genetic algorithm based anchor-box selection.
- Several new augmentation techniques. E.g. Mosaic augmentation
-
YOLOV5-ti-lite is a version of YOLOV5 from TI for efficient edge deployment. This naming convention is chosen to avoid conflict with future release of YOLOV5-lite models from Ultralytics.
-
Here is a brief description of changes that were made to get yolov5-ti-lite from yolov5:
- YOLOV5 introduces a Focus layer as the very first layer of the network. This replaces the first few heavy convolution layers that are present in YOLOv3. It reduces the complexity of the n/w by 7% and training time by 15%. However, the slice operations in Focus layer are not embedded friendly and hence we replace it with a light-weight convolution layer. Here is a pictorial description of the changes from YOLOv3 to YOLOv5 to YOLOv5-ti-lite:
-
SiLU activation is not well-supported in embedded devices. it's not quantization friendly as well because of it's unbounded nature. This was observed for hSwish activation function while quantizing efficientnet. Hence, SiLU activation is replaced with ReLU.
-
SPP module with maxpool(k=13, s=1), maxpool(k=9,s=1) and maxpool(k=5,s=1) are replaced with various combinations of maxpool(k=3,s=1).Intention is to keep the receptive field and functionality same. This change will cause no difference to the model in floating-point.
- maxpool(k=5, s=1) -> replaced with two maxpool(k=3,s=1)
- maxpool(k=9, s=1) -> replaced with four maxpool(k=3,s=1)
- maxpool(k=13, s=1)-> replaced with six maxpool(k=3,s=1) as shown below:
-
Variable size inference is replaced with fixed size inference as preferred by edge devices. E.g. tflite models are exported with a fixed i/p size.
- Training any model using this repo will take the above changes by default. Same commands as the official one can be used for training models from scartch. E.g.
python train.py --data coco.yaml --cfg yolov5s6.yaml --weights '' --batch-size 64 yolov5m6.yaml
- Yolov5-l6-ti-lite model is finetuned for 100 epochs from the official ckpt. To replicate the results for yolov5-l6-ti-lite, download the official pre-trained weights for yolov5-l6 and set the lr to 1e-3 in hyp.scratch.yaml
python train.py --data coco.yaml --cfg yolov5l6.yaml --weights 'yolov5l6.pt' --batch-size 40
- Pretrained model checkpoints along with onnx and prototxt files are kept inside pretrained_models.
- Run the following command to replicate the accuracy number on the pretrained checkpoints:
python val.py --data coco.yaml --img 640 --conf 0.001 --iou 0.65 --weights pretrained_models/yolov5s6_640_ti_lite/weights/best.pt yolov5m6_640_ti_lite yolov5l6_640_ti_lite
Dataset | Model Name | Input Size | GFLOPS | AP[0.5:0.95]% | AP50% | Notes |
---|---|---|---|---|---|---|
COCO | Yolov5s6_ti_lite_640 | 640x640 | 17.48 | 37.4 | 56.0 | |
COCO | Yolov5s6_ti_lite_576 | 576x576 | 14.16 | 36.6 | 55.7 | (Train@ 640, val@576) |
COCO | Yolov5s6_ti_lite_512 | 512x512 | 11.18 | 35.3 | 54.3 | (Train@ 640, val@512) |
COCO | Yolov5s6_ti_lite_448 | 448x448 | 8.56 | 34.0 | 52.3 | (Train@ 640, val@448) |
COCO | Yolov5s6_ti_lite_384 | 384x384 | 6.30 | 32.8 | 51.2 | (Train@ 384, val@384) |
COCO | Yolov5s6_ti_lite_320 | 320x320 | 4.38 | 30.3 | 47.6 | (Train@ 384, val@320) |
COCO | Yolov5m6_ti_lite_640 | 640x640 | 52.5 | 44.1 | 62.9 | |
COCO | Yolov5m6_ti_lite_576 | 576x576 | 42.52 | 43.0 | 61.9 | (Train@ 640, val@576) |
COCO | Yolov5m6_ti_lite_512 | 512x512 | 32.16 | 42.0 | 60.5 | (Train@ 640, val@512) |
COCO | Yolov5l6_ti_lite_640 | 640x640 | 117.84 | 47.1 | 65.6 | This model is fintuned from the official ckpt for 100 epochs |
There are three models in the pretrained_models. All other results are generated for these model on a different resolution. In order to generate the accuracy number at 512x512, run the following:
python test.py --data coco.yaml --img 512 --conf 0.001 --iou 0.65 --weights pretrained_models/yolov5s6_640_ti_lite/weights/best.pt
yolov5m6_640_ti_lite
yolov5l6_640_ti_lite
- Run the following command to export the entire models including the detection part,
python export.py --weights pretrained_models/yolov5s6_640_ti_lite/weights/best.pt --img 640 --batch 1 --simplify --export-nms --opset 11 # export at 640x640 with batch size 1
- Apart from exporting the complete ONNX model, above script will generate a prototxt file that contains information of the detection layer. This prototxt file is required to deploy the moodel on TI SoC.
[1] Official YOLOV5 repository
[2] yolov5-improvements-and-evaluation, Roboflow
[3] Focus layer in YOLOV5
[4] CrossStagePartial Network
[5] Chien-Yao Wang, Hong-Yuan Mark Liao, Yueh-Hua Wu, Ping-Yang Chen, Jun-Wei Hsieh, and I-Hau Yeh. CSPNet: A new backbone that can enhance learning capability of
cnn. Proceedings of the IEEE Conference on Computer Vision and Pattern Recognition Workshop (CVPR Workshop),2020.
[6]Shu Liu, Lu Qi, Haifang Qin, Jianping Shi, and Jiaya Jia. Path aggregation network for instance segmentation. In Proceedings of the IEEE Conference on Computer Vision and Pattern Recognition (CVPR), pages 8759–8768, 2018
[7] Efficientnet-lite quantization
[8] [YOLOv5 Training video from Texas Instruments] (https://training.ti.com/process-efficient-object-detection-using-yolov5-and-tda4x-processors)
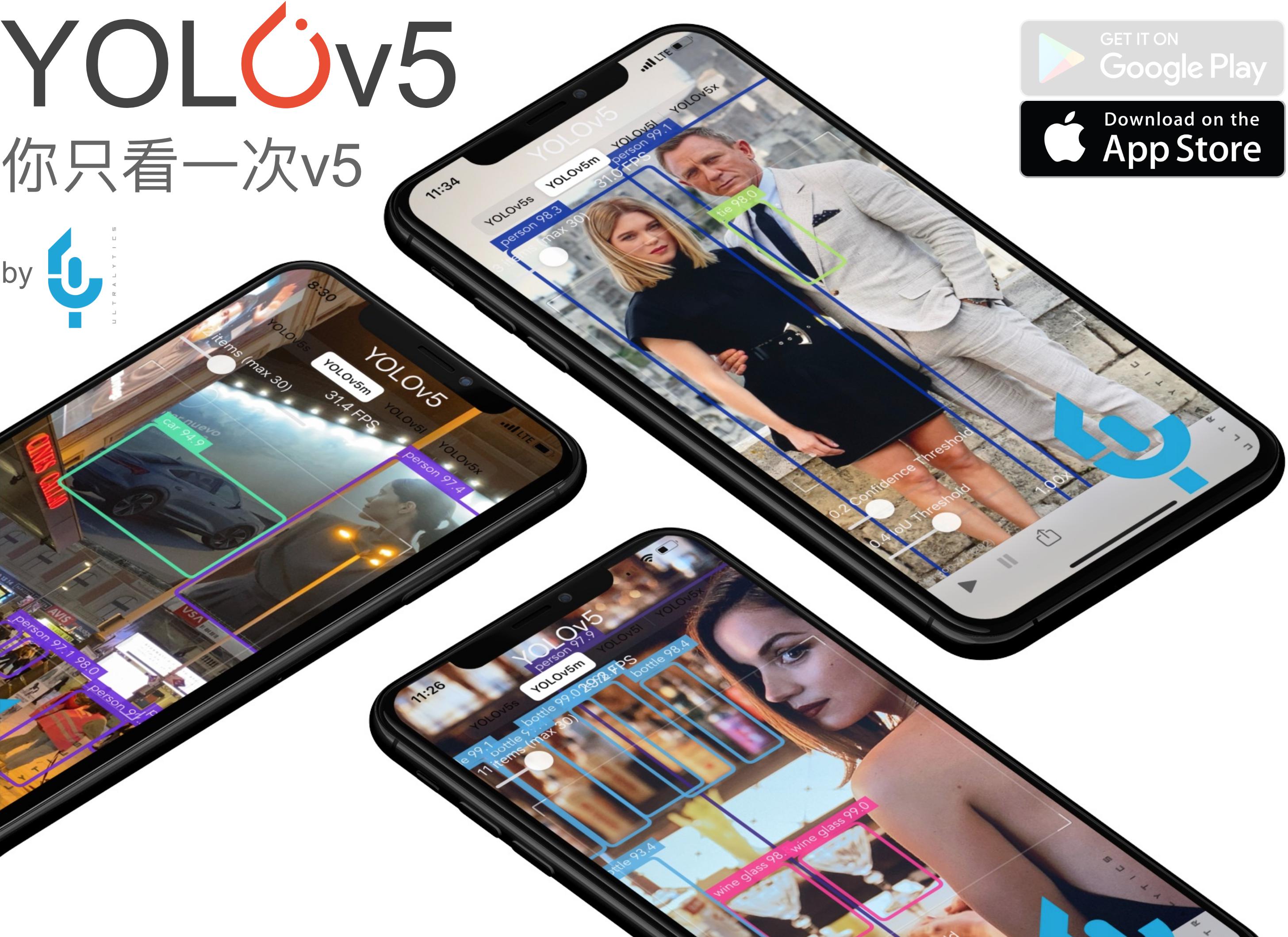
This repository represents Ultralytics open-source research into future object detection methods, and incorporates lessons learned and best practices evolved over thousands of hours of training and evolution on anonymized client datasets. All code and models are under active development, and are subject to modification or deletion without notice. Use at your own risk.
Figure Notes (click to expand)
- GPU Speed measures end-to-end time per image averaged over 5000 COCO val2017 images using a V100 GPU with batch size 32, and includes image preprocessing, PyTorch FP16 inference, postprocessing and NMS.
- EfficientDet data from google/automl at batch size 8.
- Reproduce by
python test.py --task study --data coco.yaml --iou 0.7 --weights yolov5s6.pt yolov5m6.pt yolov5l6.pt yolov5x6.pt
- April 11, 2021: v5.0 release: YOLOv5-P6 1280 models, AWS, Supervise.ly and YouTube integrations.
- January 5, 2021: v4.0 release: nn.SiLU() activations, Weights & Biases logging, PyTorch Hub integration.
- August 13, 2020: v3.0 release: nn.Hardswish() activations, data autodownload, native AMP.
- July 23, 2020: v2.0 release: improved model definition, training and mAP.
Model | size (pixels) |
mAPval 0.5:0.95 |
mAPtest 0.5:0.95 |
mAPval 0.5 |
Speed V100 (ms) |
params (M) |
FLOPS 640 (B) |
|
---|---|---|---|---|---|---|---|---|
YOLOv5s | 640 | 36.7 | 36.7 | 55.4 | 2.0 | 7.3 | 17.0 | |
YOLOv5m | 640 | 44.5 | 44.5 | 63.1 | 2.7 | 21.4 | 51.3 | |
YOLOv5l | 640 | 48.2 | 48.2 | 66.9 | 3.8 | 47.0 | 115.4 | |
YOLOv5x | 640 | 50.4 | 50.4 | 68.8 | 6.1 | 87.7 | 218.8 | |
YOLOv5s6 | 1280 | 43.3 | 43.3 | 61.9 | 4.3 | 12.7 | 17.4 | |
YOLOv5m6 | 1280 | 50.5 | 50.5 | 68.7 | 8.4 | 35.9 | 52.4 | |
YOLOv5l6 | 1280 | 53.4 | 53.4 | 71.1 | 12.3 | 77.2 | 117.7 | |
YOLOv5x6 | 1280 | 54.4 | 54.4 | 72.0 | 22.4 | 141.8 | 222.9 | |
YOLOv5x6 TTA | 1280 | 55.0 | 55.0 | 72.0 | 70.8 | - | - |
Table Notes (click to expand)
- APtest denotes COCO test-dev2017 server results, all other AP results denote val2017 accuracy.
- AP values are for single-model single-scale unless otherwise noted. Reproduce mAP by
python test.py --data coco.yaml --img 640 --conf 0.001 --iou 0.65
- SpeedGPU averaged over 5000 COCO val2017 images using a GCP n1-standard-16 V100 instance, and includes FP16 inference, postprocessing and NMS. Reproduce speed by
python test.py --data coco.yaml --img 640 --conf 0.25 --iou 0.45
- All checkpoints are trained to 300 epochs with default settings and hyperparameters (no autoaugmentation).
- Test Time Augmentation (TTA) includes reflection and scale augmentation. Reproduce TTA by
python test.py --data coco.yaml --img 1536 --iou 0.7 --augment
Python 3.8 or later with all requirements.txt dependencies installed, including torch>=1.7
. To install run:
$ pip install -r requirements.txt
- Train Custom Data 🚀 RECOMMENDED
- Tips for Best Training Results ☘️ RECOMMENDED
- Weights & Biases Logging 🌟 NEW
- Supervisely Ecosystem � NEW
- Multi-GPU Training
- PyTorch Hub ⭐ NEW
- ONNX and TorchScript Export
- Test-Time Augmentation (TTA)
- Model Ensembling
- Model Pruning/Sparsity
- Hyperparameter Evolution
- Transfer Learning with Frozen Layers ⭐ NEW
- TensorRT Deployment
YOLOv5 may be run in any of the following up-to-date verified environments (with all dependencies including CUDA/CUDNN, Python and PyTorch preinstalled):
- Google Colab and Kaggle notebooks with free GPU:
- Google Cloud Deep Learning VM. See GCP Quickstart Guide
- Amazon Deep Learning AMI. See AWS Quickstart Guide
- Docker Image. See Docker Quickstart Guide
detect.py
runs inference on a variety of sources, downloading models automatically from the latest YOLOv5 release and saving results to runs/detect
.
$ python detect.py --source 0 # webcam
file.jpg # image
file.mp4 # video
path/ # directory
path/*.jpg # glob
'https://youtu.be/NUsoVlDFqZg' # YouTube video
'rtsp://example.com/media.mp4' # RTSP, RTMP, HTTP stream
To run inference on example images in data/images
:
$ python detect.py --source data/images --weights yolov5s.pt --conf 0.25
Namespace(agnostic_nms=False, augment=False, classes=None, conf_thres=0.25, device='', exist_ok=False, img_size=640, iou_thres=0.45, name='exp', project='runs/detect', save_conf=False, save_txt=False, source='data/images/', update=False, view_img=False, weights=['yolov5s.pt'])
YOLOv5 v4.0-96-g83dc1b4 torch 1.7.0+cu101 CUDA:0 (Tesla V100-SXM2-16GB, 16160.5MB)
Fusing layers...
Model Summary: 224 layers, 7266973 parameters, 0 gradients, 17.0 GFLOPS
image 1/2 /content/yolov5/data/images/bus.jpg: 640x480 4 persons, 1 bus, Done. (0.010s)
image 2/2 /content/yolov5/data/images/zidane.jpg: 384x640 2 persons, 1 tie, Done. (0.011s)
Results saved to runs/detect/exp2
Done. (0.103s)
Inference with YOLOv5 and PyTorch Hub:
import torch
# Model
model = torch.hub.load('ultralytics/yolov5', 'yolov5s')
# Image
img = 'https://github.com/ultralytics/yolov5/raw/master/data/images/zidane.jpg'
# Inference
results = model(img)
results.print() # or .show(), .save()
Run commands below to reproduce results on COCO dataset (dataset auto-downloads on first use). Training times for YOLOv5s/m/l/x are 2/4/6/8 days on a single V100 (multi-GPU times faster). Use the largest --batch-size
your GPU allows (batch sizes shown for 16 GB devices).
$ python train.py --data coco.yaml --cfg yolov5s.yaml --weights '' --batch-size 64
yolov5m 40
yolov5l 24
yolov5x 16
Ultralytics is a U.S.-based particle physics and AI startup with over 6 years of expertise supporting government, academic and business clients. We offer a wide range of vision AI services, spanning from simple expert advice up to delivery of fully customized, end-to-end production solutions, including:
- Cloud-based AI systems operating on hundreds of HD video streams in realtime.
- Edge AI integrated into custom iOS and Android apps for realtime 30 FPS video inference.
- Custom data training, hyperparameter evolution, and model exportation to any destination.
For business inquiries and professional support requests please visit us at https://www.ultralytics.com.
Issues should be raised directly in the repository. For business inquiries or professional support requests please visit https://www.ultralytics.com or email Glenn Jocher at glenn.jocher@ultralytics.com.