-
Notifications
You must be signed in to change notification settings - Fork 186
New issue
Have a question about this project? Sign up for a free GitHub account to open an issue and contact its maintainers and the community.
By clicking “Sign up for GitHub”, you agree to our terms of service and privacy statement. We’ll occasionally send you account related emails.
Already on GitHub? Sign in to your account
Dgmr #813
Merged
Merged
Dgmr #813
Changes from 23 commits
Commits
Show all changes
34 commits
Select commit
Hold shift + click to select a range
6ee9b31
add bubble datafile test=develop
liaoxin2 5c637f5
add bubble code test=develop
liaoxin2 bb6f197
add bubble code test=develop
liaoxin2 b6f4f09
add bubble code test=develop
liaoxin2 6f0ae70
add bubble code
liaoxin2 7a09656
add bubble data
liaoxin2 f1da4e3
add bubble code
liaoxin2 c51f544
add some code for chip
liaoxin2 752719d
add some code for DGMR
liaoxin2 7660b62
add some code for DGMR
liaoxin2 ff619e4
add some code for DGMR
liaoxin2 2e05dae
add some code for DGMR
liaoxin2 63db897
add some dgmr code
liaoxin2 0760db2
add some dgmr code
liaoxin2 cb202a9
add some dgmr code
liaoxin2 1d06768
add some dgmr code
liaoxin2 a772306
add some dgmr code
liaoxin2 7802eca
add some dgmr code
liaoxin2 a5c524b
add some dgmr code
liaoxin2 ac227a7
add some dgmr code
liaoxin2 d81a68f
add some dgmr code
liaoxin2 cf7645b
add some dgmr code
liaoxin2 5f90e57
add some dgmr code
liaoxin2 239b37a
add some dgmr code
liaoxin2 4940b9f
add some dgmr code
liaoxin2 fba8ca0
add some dgmr code
liaoxin2 cba2e8a
add some dgmr code
liaoxin2 f451fa3
add some dgmr code
liaoxin2 01d2184
add some dgmr code
liaoxin2 2f01f33
add some dgmr code
liaoxin2 ee21c3d
add some dgmr code
liaoxin2 cf6c8be
add some dgmr code
liaoxin2 4a67425
add some dgmr code
liaoxin2 086e670
add some dgmr code
liaoxin2 File filter
Filter by extension
Conversations
Failed to load comments.
Loading
Jump to
Jump to file
Failed to load files.
Loading
Diff view
Diff view
There are no files selected for viewing
This file contains bidirectional Unicode text that may be interpreted or compiled differently than what appears below. To review, open the file in an editor that reveals hidden Unicode characters.
Learn more about bidirectional Unicode characters
This file contains bidirectional Unicode text that may be interpreted or compiled differently than what appears below. To review, open the file in an editor that reveals hidden Unicode characters.
Learn more about bidirectional Unicode characters
This file contains bidirectional Unicode text that may be interpreted or compiled differently than what appears below. To review, open the file in an editor that reveals hidden Unicode characters.
Learn more about bidirectional Unicode characters
Original file line number | Diff line number | Diff line change |
---|---|---|
|
@@ -24,6 +24,7 @@ | |
- USCNN | ||
- NowcastNet | ||
- HEDeepONets | ||
- DGMR | ||
- ChipDeepONets | ||
- AutoEncoder | ||
show_root_heading: true | ||
|
This file contains bidirectional Unicode text that may be interpreted or compiled differently than what appears below. To review, open the file in an editor that reveals hidden Unicode characters.
Learn more about bidirectional Unicode characters
Original file line number | Diff line number | Diff line change |
---|---|---|
|
@@ -23,4 +23,5 @@ | |
- MeshCylinderDataset | ||
- RadarDataset | ||
- build_dataset | ||
- DGMRDataset | ||
show_root_heading: true |
This file contains bidirectional Unicode text that may be interpreted or compiled differently than what appears below. To review, open the file in an editor that reveals hidden Unicode characters.
Learn more about bidirectional Unicode characters
Original file line number | Diff line number | Diff line change |
---|---|---|
@@ -0,0 +1,178 @@ | ||
# DGMR(deep generative models of radar) | ||
|
||
=== "模型训练命令" | ||
|
||
暂无 | ||
|
||
=== "模型评估命令" | ||
|
||
``` sh | ||
# Download data from Huggingface | ||
mkdir openclimatefix/nimrod-uk-1km/20200718/valid/subsampled_tiles_256_20min_stride | ||
cd openclimatefix/nimrod-uk-1km/20200718/valid/subsampled_tiles_256_20min_stride | ||
git lfs install | ||
git lfs pull --include="seq-24-*-of-00033.tfrecord.gz" | ||
|
||
python dgmr.py mode=eval EVAL.pretrained_model_path=https://paddle-org.bj.bcebos.com/paddlescience/models/dgmr/dgmr_pretrained.pdparams | ||
``` | ||
|
||
| 预训练模型 | 指标 | | ||
|:--| :--| | ||
| [dgmr_pretrained.pdparams](https://paddle-org.bj.bcebos.com/paddlescience/models/dgmr/dgmr_pretrained.pdparams) | d_loss: 130.5056<br>g_loss: 61.4635<br>grid_loss: 1.7939 | | ||
|
||
## 1. 背景简介 | ||
|
||
短临降水预报是对未来两小时内的降水进行高分辨率预测,支持了许多依赖于天气决策的实际社会经济需求。最先进的运行即时预报方法通常利用基于雷达的风估计对降水场进行平流,但往往难以捕捉重要的非线性事件,如对流的发生。最近引入的深度学习方法利用雷达直接预测未来的降雨率,摆脱了物理约束。虽然它们能够准确预测低强度降雨,但由于缺乏约束,在更长的前瞻时间内产生模糊的即时预报,导致对中到大雨事件的性能较差,其运行实用性受到限制。为了解决这些挑战,我们提出了一种用于基于雷达的降水概率即时预报的深度生成模型。我们的模型在范围为1536 km × 1280 km的区域内,能够在5-90分钟的前瞻时间内生成逼真且时空一致的预测。通过英国气象局的五十多位专家预报员进行的系统评估显示,我们的生成模型在88%的情况下在准确性和实用性方面排名第一,超过了两种竞争方法,表明了其在决策价值和向现实世界专家提供物理洞察力方面的能力。在定量验证方面,这些即时预报具有良好的技能而无需进行模糊处理。我们展示了生成式即时预报可以提供改进预报价值并支持运行实用性的概率性预测,在分辨率和前瞻时间方面,替代方法存在困难的情况下尤其如此。 | ||
|
||
短临降水预报在工程和科学领域具有多方面的重要性,主要体现在以下几个方面: | ||
|
||
- 社会影响: 短临降水预报对各行各业的实际决策都有着直接的社会影响。例如,农业、水资源管理、城市防汛和交通运输等领域都需要准确的降水预报来做出相应的应对措施,以减少可能的损失和风险。 | ||
- 安全保障: 短临降水预报对于保障公众安全至关重要。例如,预警系统可以根据短临降水预报提前通知人们可能发生的暴雨、洪涝、泥石流等灾害,从而及时采取避险措施,减少人员伤亡和财产损失。 | ||
- 生态环境保护: 对降水的准确预报有助于生态环境的保护和管理。例如,预测降水量可以帮助决策者及时调整水利工程的运行,保障生态系统的健康运行,并为植被生长提供必要的水资源。 | ||
- 科学研究: 短临降水预报也是气象科学研究的重要组成部分。通过对降水过程的研究和预测,可以更好地理解大气环境中的水循环过程,为气象学、气候学等相关领域的研究提供重要数据和支撑。 | ||
- 工程规划和设计: 在城市规划、土木工程、农业灌溉等领域,准确的短临降水预报对工程的规划和设计至关重要。例如,在城市排水系统设计中,需要考虑未来短时间内可能发生的降水情况,以保证排水系统的正常运行和城市的防洪能力。 | ||
|
||
总的来说,短临降水预报在工程和科学领域的重要性体现在保障社会安全、促进科学研究、支持生态环境保护和推动工程发展等多个方面,对于各行业的发展和社会的可持续发展都具有重要意义。 | ||
|
||
## 2. 模型原理 | ||
|
||
### 2.1 模型结构 | ||
|
||
DGMR是在条件生成对抗网络的算法框架中构建的。以过去的雷达数据为基础,对未来的雷达做出详细和可信的预测。也就是说在给定的时间点 $T$,使用基于雷达的地表降水估计值 $X_T$,基于过去 $M$ 个雷达场预测未来 $N$ 个雷达场。即: | ||
|
||
$$ | ||
P\left(X_{M+1: M+N} \mid X_{1: M}\right)=\int P\left(X_{M+1: M+N} \mid \mathrm{Z}, X_{1: M}, \boldsymbol{\theta}\right) P\left(\mathrm{Z} \mid X_{1: M}\right) d \mathrm{Z}. | ||
$$ | ||
|
||
其中 $Z$ 为随机向量,$\theta$ 为生成模型的参数。 | ||
|
||
- 等式左边是条件概率,给定过去$M$个时刻的雷达降水,预报之后$N$个时刻的雷达降水。 | ||
- 右边则将概率写为集合预报的积分形式: | ||
- 给定随机抽样 $Z$ 和生成网络参数 $\theta$,在过去 $M$ 个时刻的雷达降水约束下预报之后 $N$ 个时刻的雷达降水; | ||
- 计算随机抽样 $Z$ 在过去 $M$ 个时刻的雷达降水约束下的条件概率; | ||
- 两者相乘,为该结果的出现概率,积分后得到多次抽样下的集合预报。 | ||
|
||
|
||
对随机向量 $Z$ 的积分确保了模型产生的预测具有空间相关性。DGMR 专门用于降水预测问题。四个连续的雷达观测数据(前20分钟)被用作生成器的输入,该生成器允许对未来降水的多个实现进行抽样,每个实现包含18帧(90分钟)。模型架构示意图如图所示。 | ||
|
||
<figure markdown> | ||
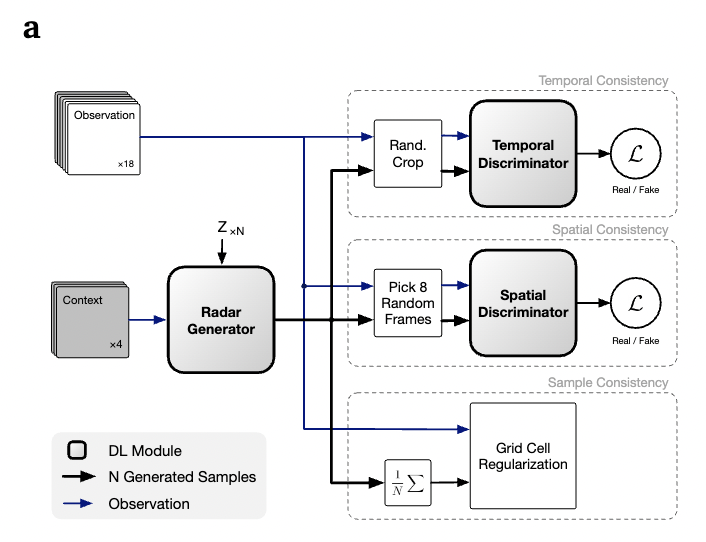{ loading=lazy style="height:80%;width:80%" align="center" } | ||
<figcaption> 模型架构示意图。</figcaption> | ||
</figure> | ||
|
||
DGMR 是一个使用两个判别器和一个附加正则化项进行训练的生成器。下图显示了生成模型和判别器的详细示意图: | ||
|
||
<figure markdown> | ||
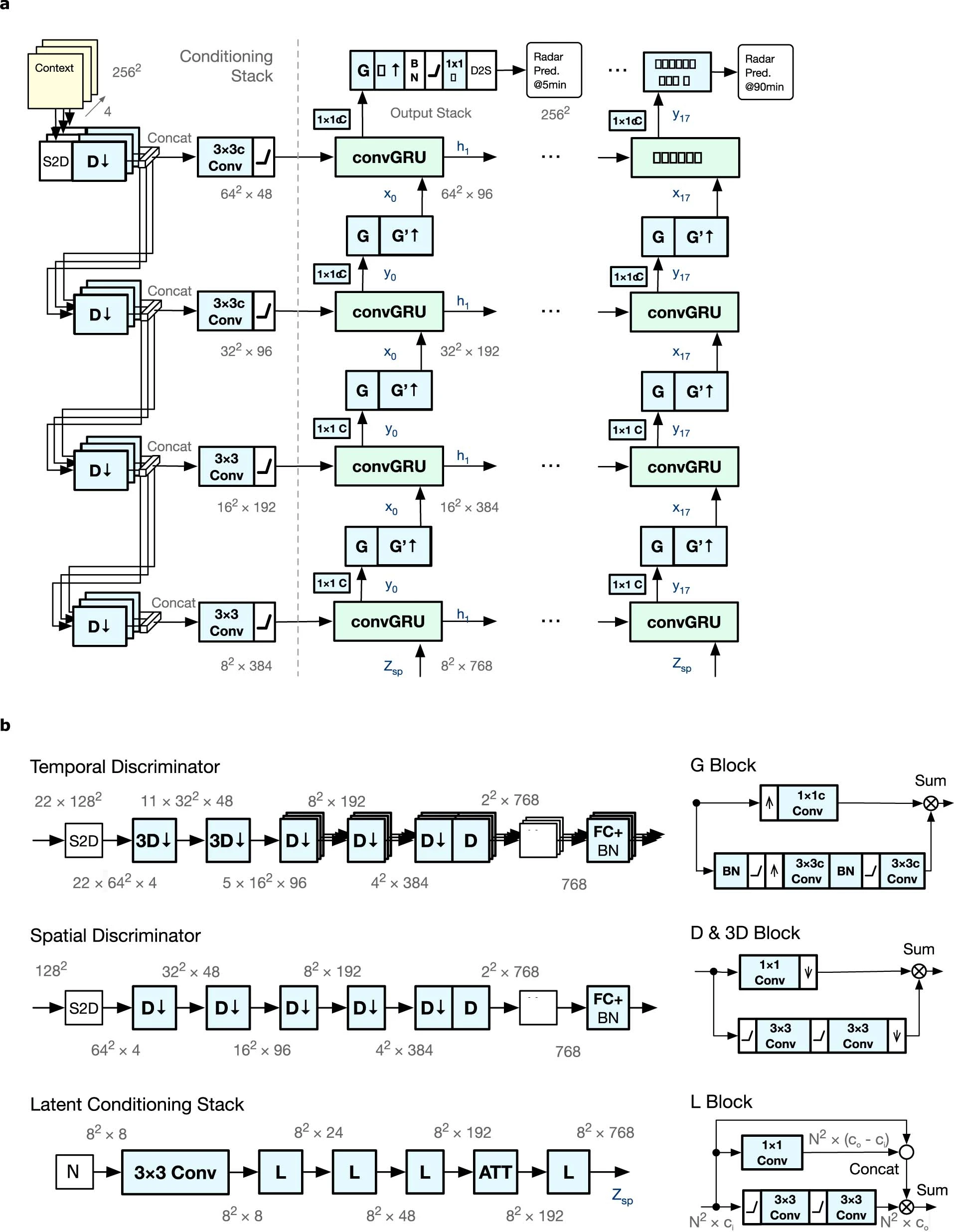{ loading=lazy style="height:80%;width:80%" align="center" } | ||
<figcaption> a、生成器架构。b,生成器的时间鉴别器架构(左上)、空间鉴别器(左中)和潜在条件堆栈(左下)。右侧是 G 块(上)、D 和 3D 块(中)以及 L 块(右)的架构。</figcaption> | ||
</figure> | ||
|
||
### 2.2 目标函数 | ||
|
||
生成器通过两个鉴别器的损失和一个网格单元正则化项(记为 $\mathcal{L}_R(\theta)$ )进行训练。空间鉴别器 $D\phi$ 具有参数 $\phi$,时间鉴别器 $T_\psi$ 具有参数 $\psi$,生成器 $G_\theta$ 具有参数 $\theta$。我们使用符号 $\{X ; G\}$ 表示两个字段的串联。最大化的生成器损失如下: | ||
|
||
$$ | ||
\begin{gathered} | ||
\mathcal{L}_G(\theta)=\mathbb{E}_{X_{1: M+N}}\left[\mathbb{E}_Z\left[D\left(G_\theta\left(Z ; X_{1: M}\right)\right)+T\left(\left\{X_{1: M} ; G_\theta\left(Z ; X_{1: M}\right)\right\}\right)\right]-\lambda \mathcal{L}_R(\theta)\right] ; \\ | ||
\mathcal{L}_R(\theta)=\frac{1}{H W N}\left\|\left(\mathbb{E}_Z\left[G_\theta\left(Z ; X_{1: M}\right)\right]-X_{M+1: M+N}\right) \odot w\left(X_{M+1: M+N}\right)\right\|_1 . | ||
\end{gathered} | ||
$$ | ||
|
||
我们在上面公式中对潜变量 $\mathrm{Z}$ 的期望使用 Carlo 估计。这些估计是使用每个输入 $X_{1: M}$ 的六个样本计算的,其中包括 $M=4$ 个雷达观测数据。网格单元正则化项确保平均预测保持接近真实值,并在高度 $H$、宽度 $W$ 和提前时间 $N$ 轴上对所有网格单元进行平均。它通过函数 $w(y)=\max (y+1,24)$ 加权至更高的降雨目标,该函数对输入向量进行逐元素操作,并在 24 处截断以提高对雷达中异常大值的鲁棒性。GAN 空间鉴别器损失 $\mathcal{L}_D(\phi)$ 和时间鉴别器损失 $\mathcal{L}_T(\psi)$ 分别相对于参数 $\phi$ 和 $\psi$ 最小化。鉴别器损失采用铰链损失公式: | ||
|
||
$$ | ||
\begin{aligned} | ||
& \mathcal{L}_D(\phi)=\mathbb{E}_{X_{1: M+N}, Z}\left[\operatorname{ReLU}\left(1-D_\phi\left(X_{M+1: M+N}\right)\right)+\operatorname{ReLU}\left(1+D_\phi\left(G\left(Z ; X_{1: M}\right)\right)\right)\right], \\ | ||
& \mathcal{L}_T(\psi)=\mathbb{E}_{X_{1: M+N}, Z}\left[\operatorname{ReLU}\left(1-T_\psi\left(X_{1: M+N}\right)\right)+\operatorname{ReLU}\left(1+T_\psi\left(\left\{X_{1: M} ; G\left(Z ; X_{1: M}\right)\right\}\right)\right)\right], | ||
\end{aligned} | ||
$$ | ||
|
||
其中 $\operatorname{ReLU} = \max(0,x)$. 更多详细的理论推导请参考 [Skillful Precipitation Nowcasting using Deep Generative Models of Radar](https://arxiv.org/pdf/2104.00954.pdf)。 | ||
|
||
## 3. 问题求解 | ||
|
||
接下来开始讲解如何将该问题一步一步地转化为 PaddleScience 代码,用 DGMR 来预测短临降水。为了快速理解 PaddleScience,接下来仅对模型构建、约束构建等关键步骤进行阐述,而其余细节请参考[API文档](../api/arch.md)。 | ||
|
||
### 3.1 数据集介绍 | ||
|
||
为了训练和评估英国的临近预报模型,DGMR 使用了英国气象局 RadarNet4 网络中的雷达复合数据。使用 2016 年 1 月 1 日至 2019 年 12 月 31 日期间每五分钟收集一次的雷达数据。我们使用以下数据分割进行模型开发。将 2016 年至 2018 年每月第一天的字段分配给验证集。2016 年至 2018 年的所有其他日期都分配给训练集。最后,使用2019年的数据作为测试集,防止数据泄露和分布泛化测试。开源的英国训练数据集已镜像到 [HuggingFace 数据集](https://huggingface.co/datasets/openclimatefix/nimrod-uk-1km),用户可以自行下载使用。 | ||
|
||
在该模型的PaddleScience 代码中,我们可以调用`ppsci.data.dataset.DGMRDataset` 来加载数据集,代码如下: | ||
|
||
``` py linenums="198" | ||
--8<-- | ||
examples/dgmr/dgmr.py:198:200 | ||
--8<-- | ||
``` | ||
|
||
### 3.2 模型构建 | ||
|
||
在 DGMR 模型中,输入过去四个雷达场数据,对 18 个未来雷达场(接下来的 90 分钟)进行预测。DGMR 网络可以表示为 $X_{1:4}$ 到输出 $X_{5:22}$ 的映射函数 $f$,即: | ||
|
||
$$ | ||
X_{5:22} = f(X_{1:4}),\\ | ||
$$ | ||
|
||
上式中 $f$ 代表 DGMR 模型。我们定义 PaddleScience 内置的 DGMR 模型类,并调用,PaddleScience 代码表示如下 | ||
|
||
``` py linenums="196" | ||
--8<-- | ||
examples/dgmr/dgmr.py:196:197 | ||
--8<-- | ||
``` | ||
|
||
这样我们就实例化出了一个 DGMR 模型,模型参数设置相关内容请参考文献 [Skillful Precipitation Nowcasting using Deep Generative Models of Radar](https://arxiv.org/pdf/2104.00954.pdf)。 | ||
|
||
### 3.3 模型评估、可视化 | ||
|
||
模型构建和加载数据后,将上述实例化的对象按顺序传递给 `ppsci.solver.Solver`,然后启动评估和可视化。首先我们初始化模型: | ||
|
||
``` py linenums="201" | ||
--8<-- | ||
examples/dgmr/dgmr.py:201:206 | ||
--8<-- | ||
``` | ||
|
||
然后自定义评估方式和损失函数,代码如下: | ||
|
||
``` py linenums="86" | ||
--8<-- | ||
examples/dgmr/dgmr.py:86:188 | ||
--8<-- | ||
``` | ||
|
||
最后对数据中的每个 batch 进行遍历评估,同时对预测结果进行可视化。 | ||
|
||
``` py linenums="208" | ||
--8<-- | ||
examples/dgmr/dgmr.py:208:231 | ||
--8<-- | ||
``` | ||
|
||
## 4. 完整代码 | ||
|
||
``` py linenums="1" title="dgmr.py" | ||
--8<-- | ||
examples/dgmr/dgmr.py | ||
--8<-- | ||
``` | ||
|
||
## 5. 结果展示 | ||
|
||
如图所示为分别在 $T+5, T+10, \cdots, T+45$ 分钟的气象降水预测,与真实的降水情况相比可以看出,该模型可以给出比较好的短临降水预测。 | ||
|
||
<figure markdown> | ||
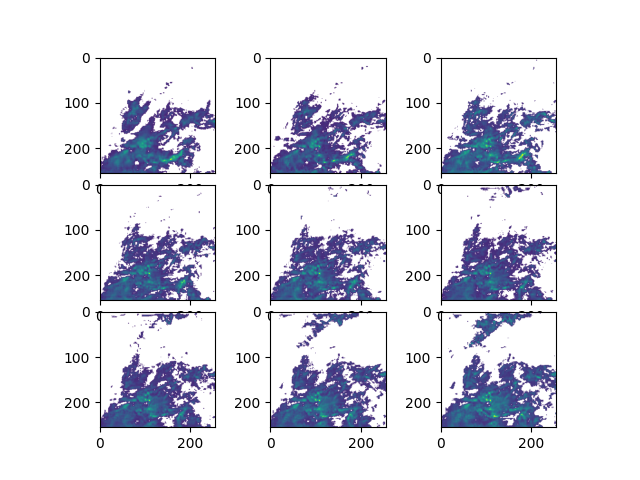{ loading=lazy style="height:80%;width:80%" align="center" } | ||
<figcaption> 模型预测的降水情况</figcaption> | ||
</figure> | ||
|
||
<figure markdown> | ||
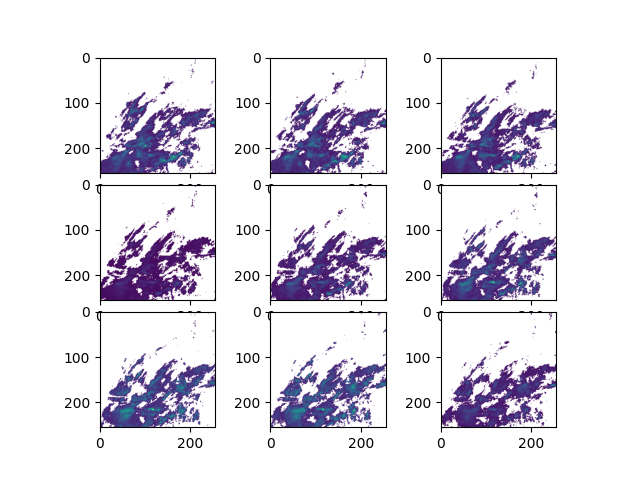{ loading=lazy style="height:80%;width:80%" align="center" } | ||
<figcaption> 真实降水情况</figcaption> | ||
</figure> | ||
|
||
## 6. 参考资料 | ||
|
||
参考文献: [Skillful Precipitation Nowcasting using Deep Generative Models of Radar](https://arxiv.org/pdf/2104.00954.pdf) | ||
|
||
参考代码: [DGMR](https://github.com/openclimatefix/skillful_nowcasting) |
This file contains bidirectional Unicode text that may be interpreted or compiled differently than what appears below. To review, open the file in an editor that reveals hidden Unicode characters.
Learn more about bidirectional Unicode characters
Original file line number | Diff line number | Diff line change |
---|---|---|
@@ -0,0 +1,60 @@ | ||
hydra: | ||
run: | ||
# dynamic output directory according to running time and override name | ||
dir: outputs_dgmr/${now:%Y-%m-%d}/${now:%H-%M-%S}/${hydra.job.override_dirname} | ||
job: | ||
name: ${mode} # name of logfile | ||
chdir: false # keep current working direcotry unchaned | ||
config: | ||
override_dirname: | ||
exclude_keys: | ||
- TRAIN.checkpoint_path | ||
- TRAIN.pretrained_model_path | ||
- EVAL.pretrained_model_path | ||
- mode | ||
- output_dir | ||
- log_freq | ||
callbacks: | ||
init_callback: | ||
_target_: ppsci.utils.callbacks.InitCallback | ||
sweep: | ||
# output directory for multirun | ||
dir: ${hydra.run.dir} | ||
subdir: ./ | ||
|
||
# general settings | ||
mode: eval # running mode: train/eval | ||
seed: 42 | ||
output_dir: ${hydra:run.dir} | ||
|
||
# dataset settings | ||
DATASET: | ||
input_keys: 'input_frames' | ||
label_keys: 'target_frames' | ||
split: validation # train or validation | ||
num_input_frames: 4 | ||
num_target_frames: 18 | ||
dataset_path: openclimatefix/nimrod-uk-1km | ||
number: 1000 | ||
|
||
# model settings | ||
MODEL: | ||
input_keys: 'input_frames' | ||
output_keys: 'future_images' | ||
forecast_steps: 18 | ||
input_channels: 1 | ||
output_shape: 256 | ||
gen_lr: 5e-05 | ||
disc_lr: 0.0002 | ||
conv_type: 'standard' | ||
num_samples: 6 | ||
grid_lambda: 20.0 | ||
beta1: 0.0 | ||
beta2: 0.999 | ||
latent_channels: 768 | ||
context_channels: 384 | ||
generation_steps: 6 | ||
|
||
# evaluation settings | ||
EVAL: | ||
pretrained_model_path: https://paddle-org.bj.bcebos.com/paddlescience/models/dgmr/dgmr_pretrained.pdparams |
Oops, something went wrong.
Add this suggestion to a batch that can be applied as a single commit.
This suggestion is invalid because no changes were made to the code.
Suggestions cannot be applied while the pull request is closed.
Suggestions cannot be applied while viewing a subset of changes.
Only one suggestion per line can be applied in a batch.
Add this suggestion to a batch that can be applied as a single commit.
Applying suggestions on deleted lines is not supported.
You must change the existing code in this line in order to create a valid suggestion.
Outdated suggestions cannot be applied.
This suggestion has been applied or marked resolved.
Suggestions cannot be applied from pending reviews.
Suggestions cannot be applied on multi-line comments.
Suggestions cannot be applied while the pull request is queued to merge.
Suggestion cannot be applied right now. Please check back later.
There was a problem hiding this comment.
Choose a reason for hiding this comment
The reason will be displayed to describe this comment to others. Learn more.
参考其他配置文件,加上callback字段