-
Notifications
You must be signed in to change notification settings - Fork 5.6k
New issue
Have a question about this project? Sign up for a free GitHub account to open an issue and contact its maintainers and the community.
By clicking “Sign up for GitHub”, you agree to our terms of service and privacy statement. We’ll occasionally send you account related emails.
Already on GitHub? Sign in to your account
paddle 推理完之后内存不释放 #43346
Comments
您好,我们已经收到了您的问题,会安排技术人员尽快解答您的问题,请耐心等待。请您再次检查是否提供了清晰的问题描述、复现代码、环境&版本、报错信息等。同时,您也可以通过查看官网API文档、常见问题、历史Issue、AI社区来寻求解答。祝您生活愉快~ Hi! We've received your issue and please be patient to get responded. We will arrange technicians to answer your questions as soon as possible. Please make sure that you have posted enough message to demo your request. You may also check out the API,FAQ,Github Issue and AI community to get the answer.Have a nice day! |
另外,在tensorflow和torch的python代码里面都有限制GPU内存的命令,即限制GPU使用多少的内存。在paddle里面有没有类似的python代码或命令? |
同样的问题,每个predictor.run() 之后调用了上述clearxxx和shrink之后显存占用的确可以了。但是时常出现一些内存/显存相关的错误: C++ Traceback (most recent call last):0 paddle::AnalysisPredictor::ZeroCopyRun() Error Message Summary:NotFoundError: The memory block is not found in cache |
跑24小时差不多必现。有时是如下一种报错: ***** SIGNAL SIGABRT(6) ******* STACKDUMP *******
|
@hp03 是不是上述clearxxx和shrink在每个predictor.run之后都必须调用?如果一些predictor.run之后调用这个,一些不调用,会不会出现上述问题?还是其他原因? |
+1: ***** SIGNAL SIGABRT(6) ******* STACKDUMP *******
|
terminate called after throwing an instance of 'paddle::platform::EnforceNotMet' Compile Traceback (most recent call last):
C++ Traceback (most recent call last):0 paddle::AnalysisPredictor::ZeroCopyRun() Error Message Summary:ExternalError: CUDNN error(8), CUDNN_STATUS_EXECUTION_FAILED. |
有新的进展么? |
内存问题,我的方案:1、控制输入大小,对数据裁剪控制最大限制,比如图片统一压缩到128*128 2、控制批量大小 |
楼上的大哥们, 怎么解决的? |
issue的意思是,一直推理无法释放内存,但是压缩图片只是增加模型能推理的图片数量而已吧,好像没解决内存问题阿? |
我这个是控制住内存的上限,不释放无所谓,因为要持续推理使用; |
明白了,我以为是每次推理都会增加显存,刚测试了只要达到上限就行。 |
@Tian14267 @Lanme @2742195759 @anexplore 大家好 我是Paddle的产品经理 雷青 ,大家现在这个问题解决了嘛? 备注:这个issue应该问题说的应该是显存问题哈。我看贴图也都是显存的信息。 【显存释放问题】释放显存通常是在后续没有推理任务(即Predict后面不用了);如果后面还要继续推理,频繁释放显存会造成推理模型频繁的加载到显存里面。 【指定固定显存问题】Paddle现在还有没可以手动设定显存的功能,如果大家有改需求,欢迎大家给FastDeploy仓库提需求。 【其他部署需求】大家在部署中有其他部署需求,也欢迎随时给FastDeploy仓库提需求 |
为什么处理过程中显示一直增加。paddle很不稳定还总是coredump |
这个 ISSUE 有最新进展么?使用 CPU 推理也会遇到同样问题:内存占用随着推理图片调用次数增加而持续增加。 |
CPU推理关闭MKLDNN加速可以解决 |
我也遇到类似的问题,但是不是在inference环节,training的环节就出现显存消耗一直增加。 Paddle有没有一个语句可以实时的返回消耗的显存额度?我好排查一下是在哪一步一直吃显存的 |
请提出你的问题 Please ask your question
你好,我遇到一个问题,paddle模型预测万之后内存不释放的情况。详细情况如下:
服务:使用Websocket 进行模型加载和部署
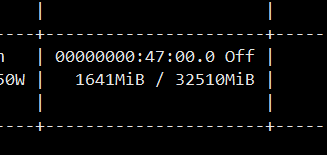
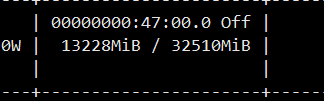
详情:
1:初始加载模型和服务的时候,**会消耗GPU大概1.7G内存(如图):
2:加载完之后进行语音合成的时候内存会涨上去。整个合成任务过程中,内存能够涨到13G左右(如图),任务结束之后,这个内存无法释放。即,无法从13G回到1.7G。
请问paddle有没有内存释放机制,能够在任务结束之后,再不关闭服务的前提下,把多出来的内存释放了?
https://github.com/PaddlePaddle/PaddleSpeech/issues/2024
The text was updated successfully, but these errors were encountered: